Analysis and Identification Genetic Effect of SARS-CoV-2 Infections to Alzheimer’s Disease Patients by Integrated Bioinformatics
Abstract
Background:
COVID-19 pandemic is a global crisis which results in millions of deaths and causes long-term neurological sequelae, such as Alzheimer’s disease (AD).
Objective:
We aimed to explore the interaction between COVID-19 and AD by integrating bioinformatics to find the biomarkers which lead to AD occurrence and development with COVID-19 and provide early intervention.
Methods:
The differential expressed genes (DEGs) were found by GSE147507 and GSE132903, respectively. The common genes between COVID-19 and AD were identified. Gene Ontology (GO), Kyoto Encyclopedia of Genes and Genomes (KEGG), and protein–protein interactions (PPI) network analysis were carried out. Hub genes were found by cytoscape. A multivariate logistic regression model was constructed. NetworkAnalyst was used for the analysis of TF-gene interactions, TF-miRNA coregulatory network, and Protein-chemical Interactions.
Results:
Forty common DEGs for AD and COVID-19 were found. GO and KEGG analysis indicated that the DEGs were enriched in the calcium signal pathway and other pathways. A PPI network was constructed, and 5 hub genes were identified (ITPR1, ITPR3, ITPKB, RAPGEF3, MFGE8). Four hub genes (ITPR1, ITPR3, ITPKB, RAPGEF3) which were considered as important factors in the development of AD that were affected by COVID-19 were shown by nomogram. Utilizing NetworkAnalyst, the interaction network of 4 hub genes and TF, miRNA, common AD risk genes, and known compounds is displayed, respectively.
Conclusion:
COVID-19 patients are at high risk of developing AD. Vaccination is required. Four hub genes can be considered as biomarkers for prediction and treatment of AD development caused by COVID-19. Compounds with neuroprotective effects can be used as adjuvant therapy for COVID-19 patients.
INTRODUCTION
Ever since cases of COVID-19 were first reported on 31 December 2019 in China, COVID-19 has rapidly spread through the whole world and caused millions of deaths [1, 2]. Several studies showed that COVID-19 can impair the central nervous system and result in long-term neurological sequelae, like Alzheimer’s disease (AD) [3, 4]. As we know, the therapy and management of AD patients impose a substantial burden on society and families. In addition, research indicated that AD patients were more susceptible to severe acute respiratory syndrome coronavirus 2 (SARS-CoV-2), and COVID-19 would cause their AD condition to worsen [3, 5].
Our paper studies the interaction between COVID-19 and AD in order to explore the effective control strategy for managing the diseases. We aimed to explain the central nervous system damage caused by COVID-19 in the population by using integrated bioinformatics analysis, especially concentrating on the potential molecular biological functions and pathways that cause and aggravate AD, thus benefiting future exploration of intervention and treatment strategies.
We screened differential expressed genes (DEGs) by using GSE147507 [6] and GSE132903 [7], respectively, and found the common DEGs between COVID-19 and AD. Then we carried out a series of bioinformatics analysis such as Gene Ontology (GO), Kyoto Encyclopedia of Genes and Genomes (KEGG), protein–protein interactions (PPI), hub gene identification, etc.
We found that common DEGs were enriched in calcium signaling and other pathways, among which the imbalance of calcium ion homeostasis is one of the most important pathogenesis of AD. We found 4 hub genes (ITPR1, ITPR3, ITPKB, RAPGEF3) that may play an important role in the occurrence and development of AD in COVID-19 patients through the identification of hub genes and the validation of the multivariate logistic regression model. The 4 hub genes can be considered as biomarkers for the occurrence and development of AD caused by COVID-19. In addition, we utilized the common hub genes of the two diseases to construct clinical diagnostic model (ROC_AUC = 0.757) in order to provide effective strategy and tools for early screening risk of AD. This study also indicated that vaccination is a relatively safe and economic intervention to achieve universal immunization. Finally, compounds with neuroprotective effects can be used as adjuvant therapy for COVID-19 patients.
METHODS
Dataset collections
Dataset (GSE147507: https://www.ncbi.nlm.nih.gov/geo/query/acc.cgi?acc=GSE147507) [6] was selected for SARS-CoV-2 infection in human at transcriptional levels and dataset (GSE132903: https://www.ncbi.nlm.nih.gov/geo/query/acc.cgi?acc=GSE132903) [7] was selected for the gene expression of AD analysis. Both datasets were acquired from GEO database (https://www.ncbi.nlm.nih.gov/geo/). Samples of lung biopsy for healthy negative control and lung sample from postmortem COVID-19 patient were selected from GSE147507 for analysis. Samples which composed of temporal gyrus samples from AD patients (AD = 97) and non-dementia controls (ND = 98) was selected from GSE132903. The study flow is shown as in Fig. 1.
Fig. 1
Study flowchart. AD, Alzheimer disease; DEGs, differential expressed genes; GO, Gene Ontology; KEGG, Kyoto Encyclopedia of Genes and Genomes.
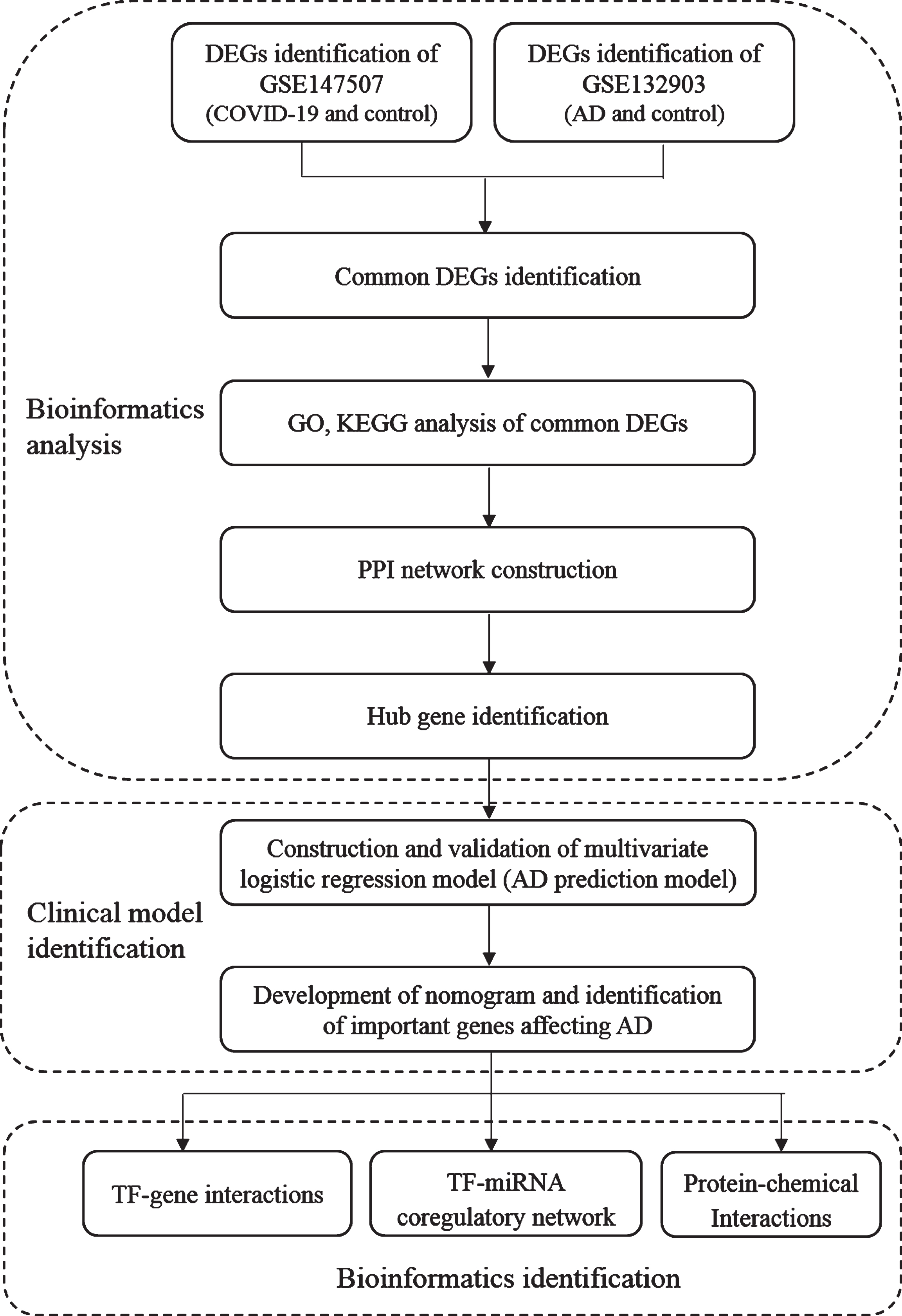
DEGs identification and common gene identification between COVID-19 and AD
DESeq2 [8] and limma [9] package in R software (version 4.0.2) with P-value < 0.05 and log2|FC|> 1 were used for DEGs identification between COVID-19 patients and health control from GSE147507. And limma packages in R software with log2|FC|> 0.5, adj.p.val < 0.01 were used to obtain DEGs from GSE132903. The common DEGs between COVID-19 and AD was obtained by using R software.
GO and KEGG analysis
GO and KEGG enrichment analysis were carried out with the “clusterProfiler R” [10] package (v3.16.1) of R software.
PPI network analysis and hub genes identification
Common DEGs were used to construct a PPI network by STRING (https://string-db.org/) with a confidence score of ≥0.4. Hub genes of the PPI network were identified using degree algorithm from cytoHubba [11], a plugin in Cytoscape, and visualized using Cytoscape (v3.7.2).
Model construction
Sex, age, and hub gene expression profiles from GSE132903 were integrated to analyze the correlation between these factors and AD using univariate logistic regression. The baseline data was shown as in Table 1. Then the data was divided into a training set (70%) and a test set (30%), the difference between the two sets was then verified. In the training set, a multivariate logistic regression model was constructed by incorporating the features of p < 0.05 in the results of univariate logistic regression analysis. ROC_AUC was performed to validate the model in the test set.
Table 1
Baseline data of AD and the control in GSE132903
AD | ND | p | |
NO. | 97 | 98 | |
ITPR1 (mean(sd)) | 9.39 (0.92) | 10.03 (0.82) | 6.30E-07 |
ITPR3 (mean(sd)) | 8.63 (0.61) | 8.04 (0.55) | 1.40E-10 |
ITPKB (mean(sd)) | 9.88 (0.82) | 8.98 (0.74) | 7.90E-14 |
RAPGEF3 (mean(sd)) | 8.34 (0.77) | 7.52 (0.53) | 5.10E-14 |
MFGE8 (mean(sd)) | 10.42 (0.6) | 9.89 (0.54) | 2.40E-09 |
Age (mean(sd)) | 85.02 (6.75) | 84.98 (6.9) | 0.97 |
Sex (%) | N = 97 (100(%)) | ||
Female | 48 (49.48%) | 48 (48.98%) | |
Male | 49 (50.52%) | 50 (51.02%) | 1 |
AD_diagnosis (%) | N = 97 (100(%)) | ||
AD | 97 (100%) | 0 (0%) | |
Normal | 0 (0%) | 98 (100%) | 0 |
p < 0.05 is considered to be statistically significant.
Development and validation of a multigene containing nomogram
Nomograms include several lines corresponding to certain clinical parameters and have been widely used to predict the incidence of patients in a clinical environment [12]. A multigene containing nomogram was constructed according to the multivariate logistic regression model in the training set.
Validation of hub genes by Alzdata
The hub gene expression profiles between AD and control brain tissues were determined using AlzData (http://www.alzdata.org). AlzData is a database that provides human brain gene expression profiling [13, 14].
TF-gene interactions
NetworkAnalyst [15] (https://www.networkanalyst.ca/) was used to find the TF-gene interaction with identified hub genes. TF-gene interaction analysis with integration of common AD risk genes [16, 17] (APP, PSEN1, PSEN2, APOE, SORL1, ABCA7, TREM2, PLCG2, BDNF) and hub genes was also performed by NetworkAnalyst.
TF-miRNA coregulatory network
TF-miRNA coregulatory network was constructed with the identified hub genes using NetworkAnalyst tool [15].
Protein-chemical interactions
An important component of the study also included using NetworkAnalyst to identify compounds that interact with hub genes [15].
RESULTS
DEGs identification and common DEGs identification between COVID-19 and AD
We obtained 805 DEGs from GSE147507 and 704 DEGs from GSE132903. Then we took the intersection of 805 DEGs for COVID-19 and 704 DEGs for AD and determined 40 common DEGs, this was then visualized with a Venn diagram (Fig. 2).
Fig. 2
Common DEGs are represented by a Venn diagram. 40 genes were found as common DEGs from 805 DEGs of COVID-19 and 704 DEGs of AD patients.
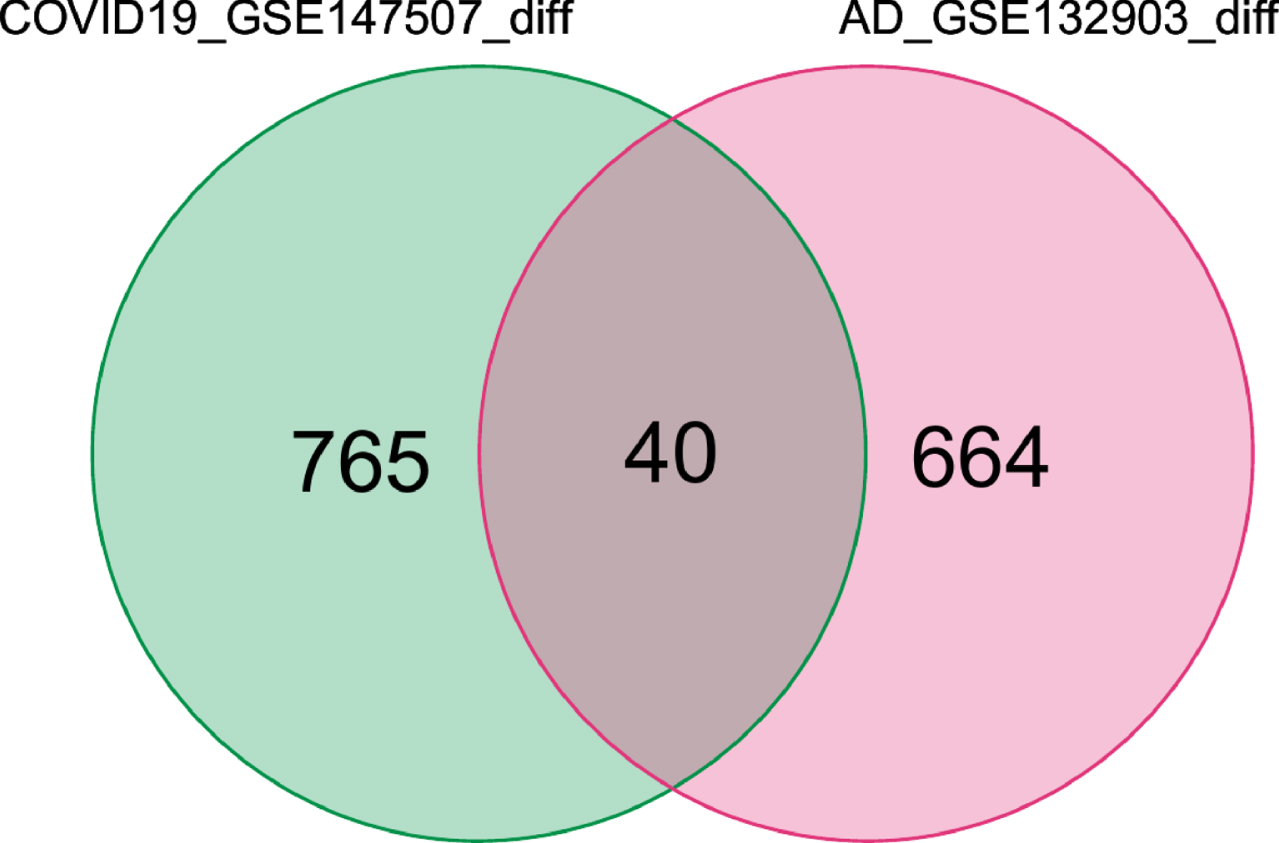
GO and KEGG analysis
The 40 common DEGs between AD and COVID-19 were used for GO and KEGG analysis. GO analysis showed that genes were enriched in molecular function of inositol 1,4,5 trisphosphate binding and calcium-release channel activity (Fig. 3). KEGG analysis demonstrated the genes were enriched on pathways of apoptosis, long-term potentiation, calcium signaling, cellular senescence, glutamatergic synapse, AD, etc. (Fig. 4).
Fig. 3
GO enrichment analysis for the common DEGs. BP, biological process of GO analysis; CC, cellular component of GO analysis; MF, molecular function of GO analysis.
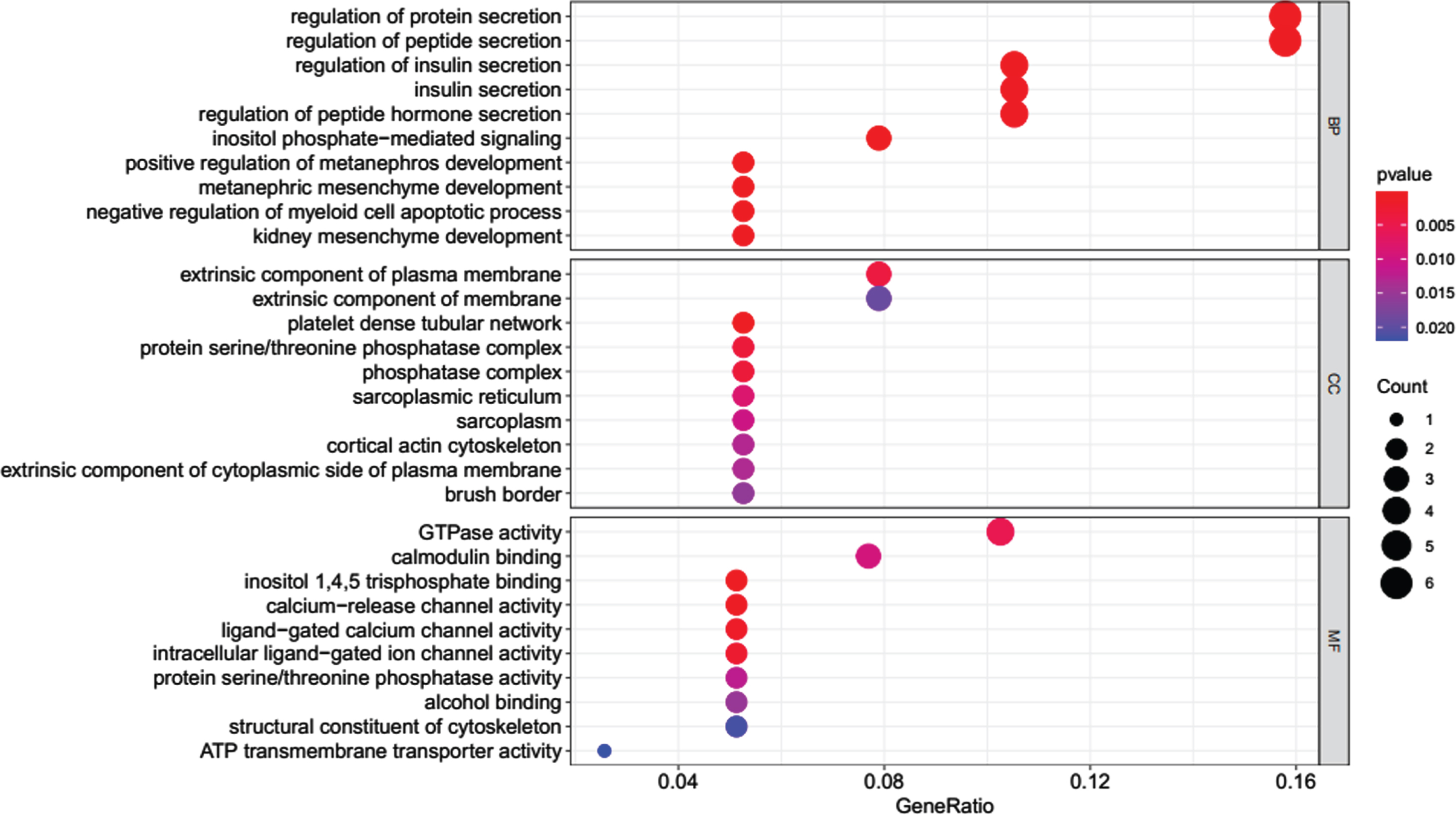
Fig. 4
KEGG enrichment analysis for the common DEGs.
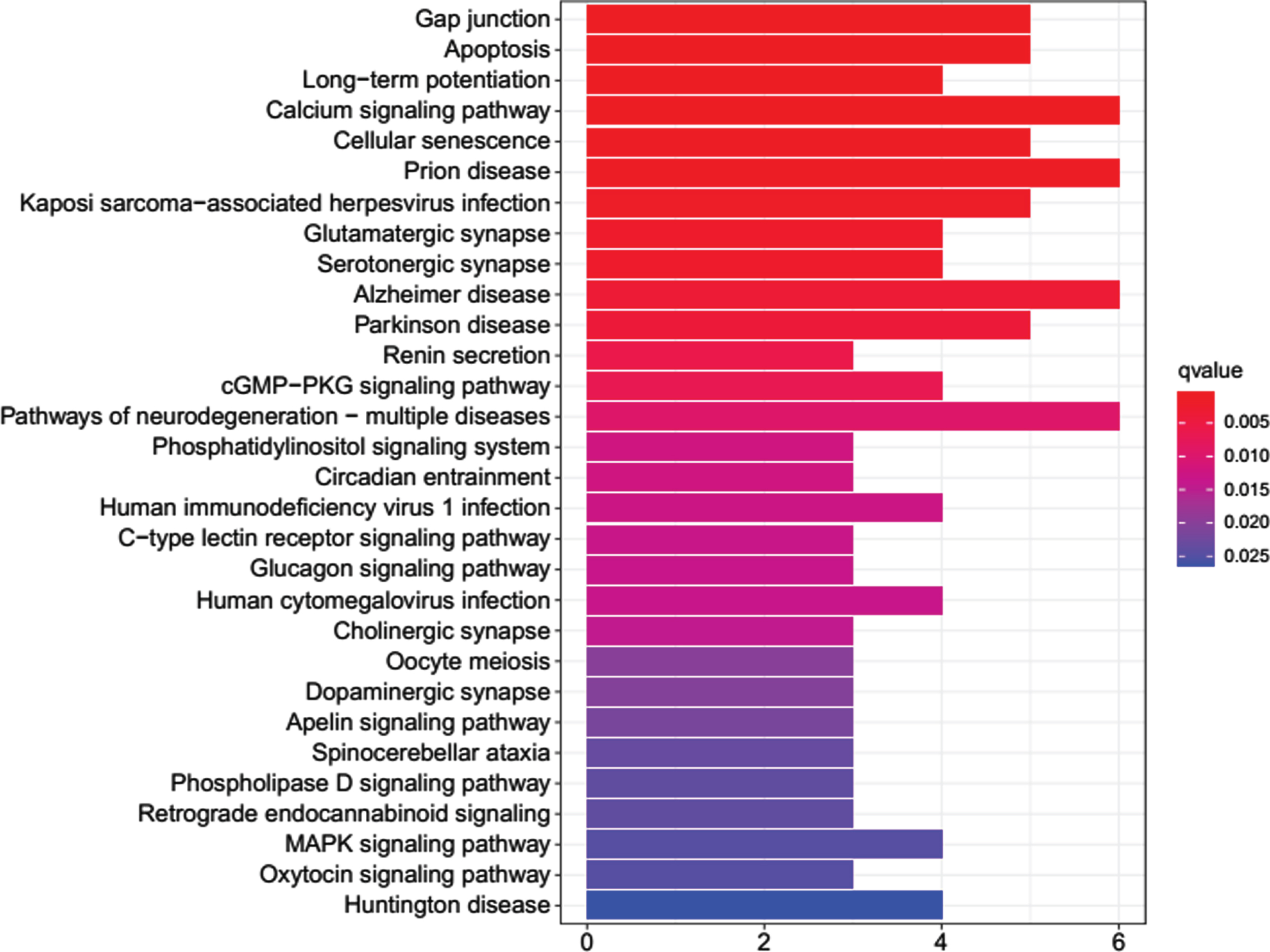
PPI network analysis and hub genes identification
We entered the 40 common DEGs into STRING and imported generated files into Cytoscape (https://cytoscape.org/) for the visualization of PPI network analysis (Fig. 5). Then we used cytoHubba to identify the 5 hub genes ITPR1, ITPR3, ITPKB, RAPGEF3, and MFGE8 (Fig. 6).
Fig. 5
PPI network for common DEGs which are shared by COVID-19 and AD.
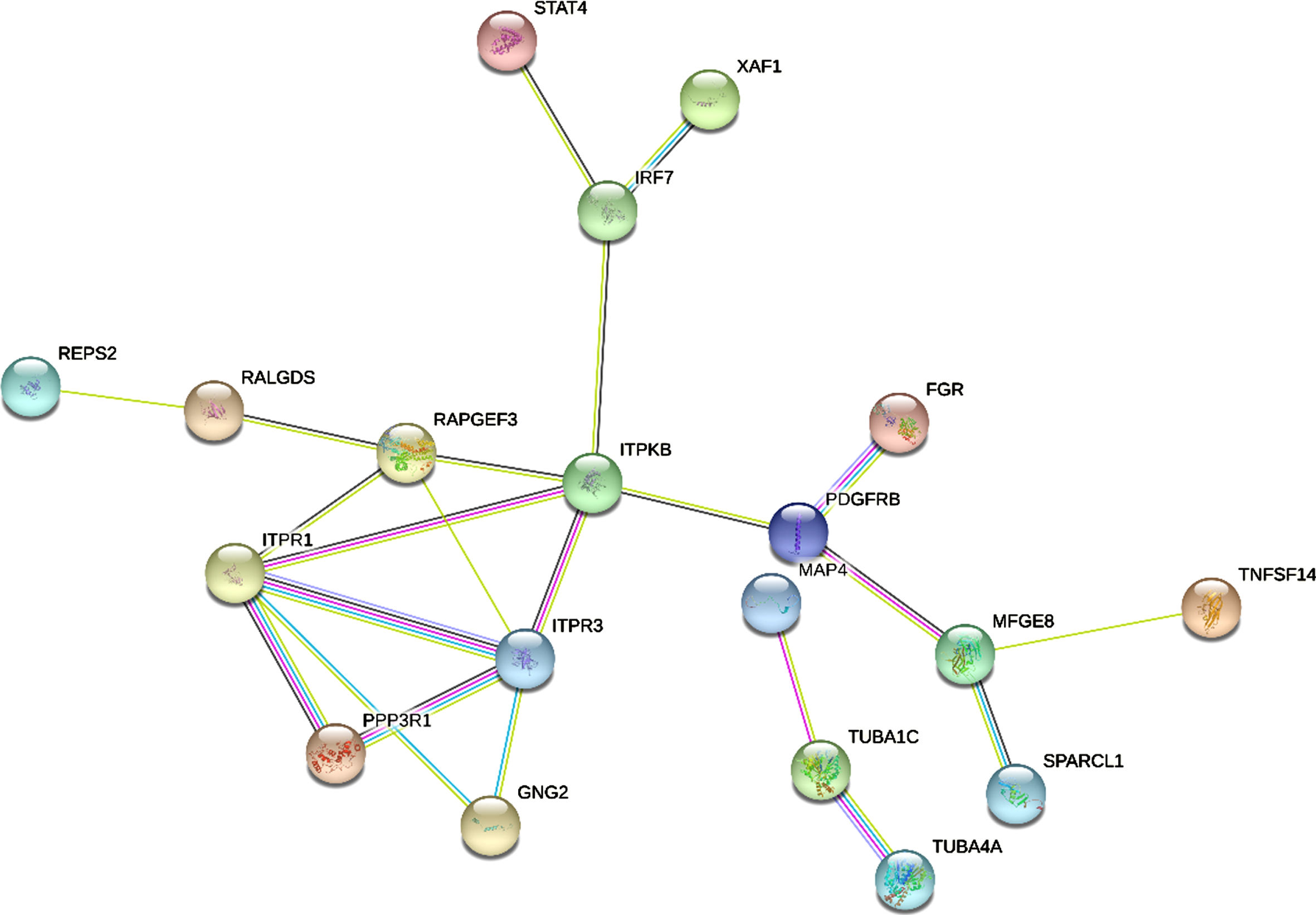
Fig. 6
Identification of hub gene from the PPI network. According to the degree value, the highlighted 5 genes (ITPR1, ITPR3, ITPKB, RAPGEF3, MFGE8) are considered as hub genes.
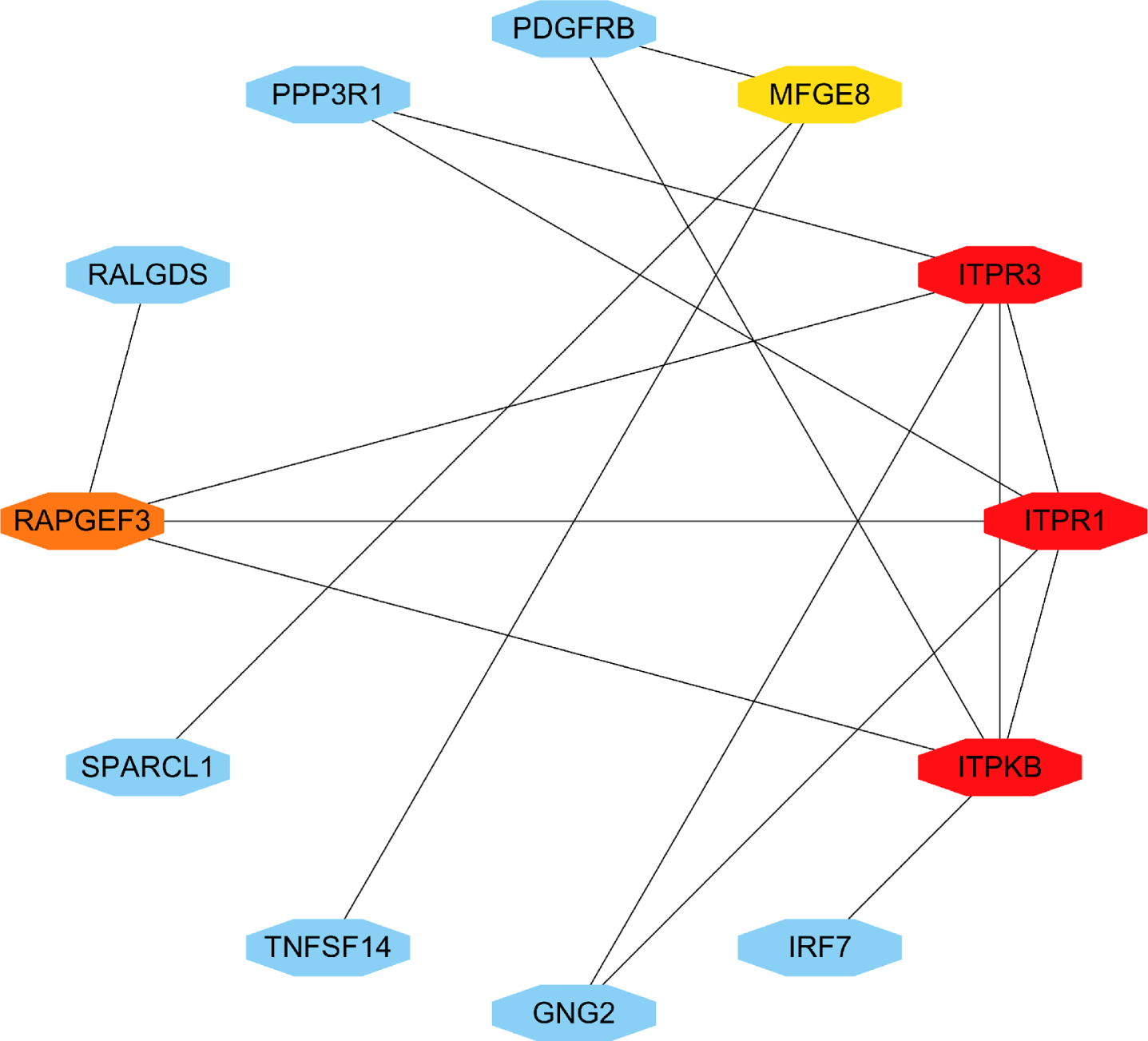
Model construction and validation
The training set and test set was verified, and there was no significant difference between the two sets (Supplementary Table 1). Univariate logistic regression was used to analyze features which would affect the occurrence of AD, including ITPR1, ITPR3, ITPKB, RAPGEF3, and MFGE8 (p < 0.05) (Table 2). Multivariate regression analysis indicated that 4 hub genes (ITPR1, ITPR3, ITPKB, RAPGEF3) were important factors affecting AD (p < 0.05) (Table 3). The result was validated using ROC_AUC with a score of 0.757 in the test set (Fig. 7).
Table 2
Univariate logistic regression of risk factors in AD
characteristics | R val | P val | |
1 | ITPR1 | –0.82 | 0 |
2 | ITPR3 | 1.69 | 0 |
3 | ITPKB | 1.42 | 0 |
4 | RAPGEF3 | 1.97 | 0 |
5 | MFGE8 | 1.57 | 0 |
6 | Age | 0 | 0.97 |
7 | Sex | –0.02 | 0.94 |
p < 0.05 is considered to be statistically significant.
Table 3
Multivariate logistic regression of Risk Factors in AD
Intercept and Variable | β | Odds Ratio (95CI) | p | |||
(Intercept) | –62.8404185 | 1.24E-05 | ||||
ITPR1 | 2.2525753 | 9.51E + 00 | (2.95E + 00 | to | 3.54E + 01) | 0.000341 |
ITPR3 | 1.9249126 | 6.85E + 00 | (1.63E + 00 | to | 3.24E + 01) | 0.010611 |
ITPKB | 0.9699832 | 2.64E + 00 | (1.06E + 00 | to | 6.86E + 00) | 0.0398 |
RAPGEF3 | 3.5058907 | 3.33E + 01 | (7.21E + 00 | to | 2.03E + 02) | 3.14E-05 |
MFGE8 | –1.1648198 | 3.12E-01 | (7.38E-02 | to | 1.22E + 00) | 0.099675 |
p<0.05 is considered to be statistically significant.
Fig. 7
ROC-AUC of the multivariate logistic regression model for AD prediction (test set)
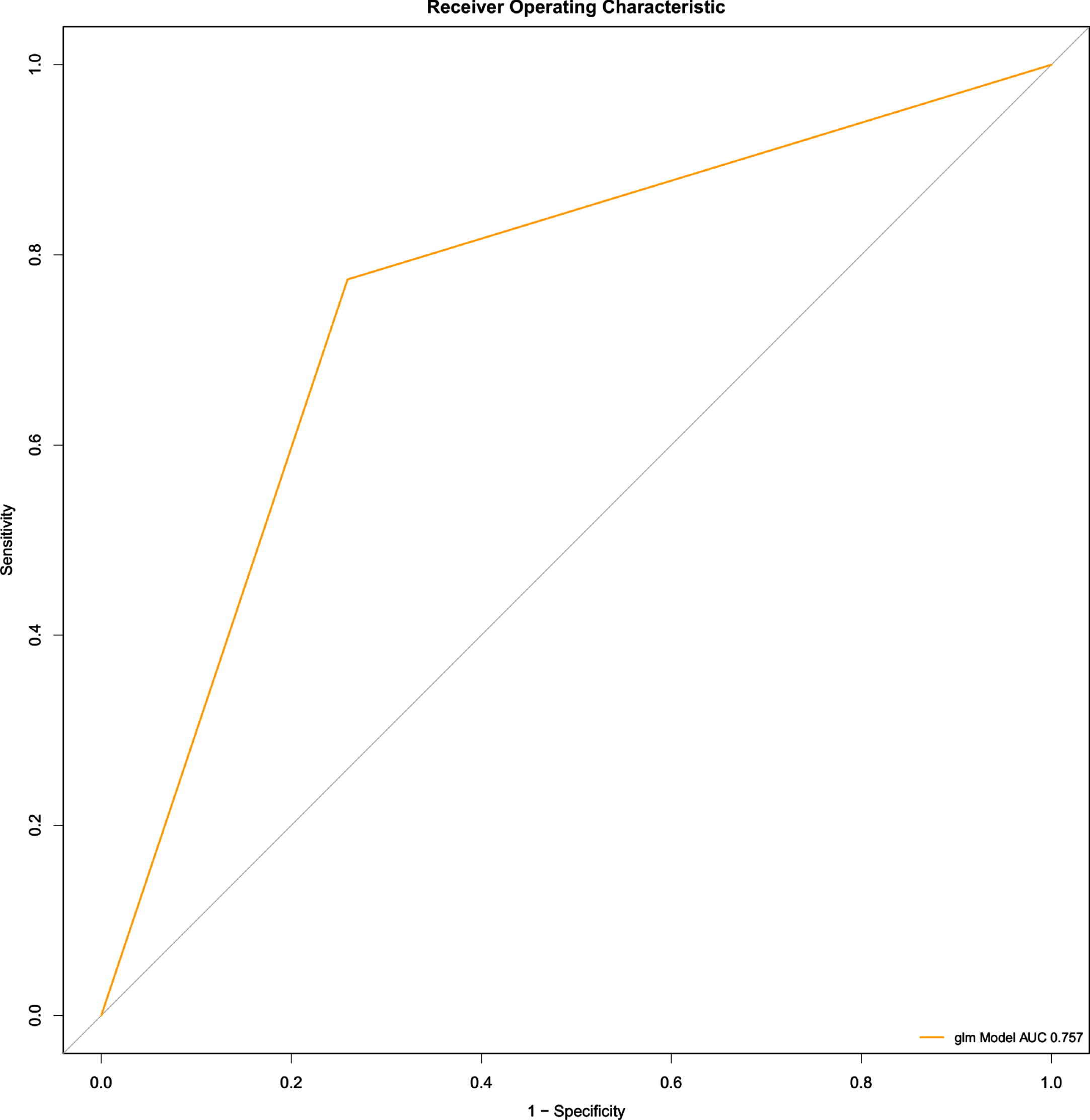
Development of a multigene containing nomogram
A multigene containing nomogram was constructed according to the multivariate logistic regression model in the training set. For example, a sample (GSM3896060) from GSE132903 has an AD incidence probability of 0.883 and requires active treatment (Fig. 8).
Fig. 8
Multigene based nomogram by 4 hub genes predicting the probability with Alzheimer’s disease (GSE132903).
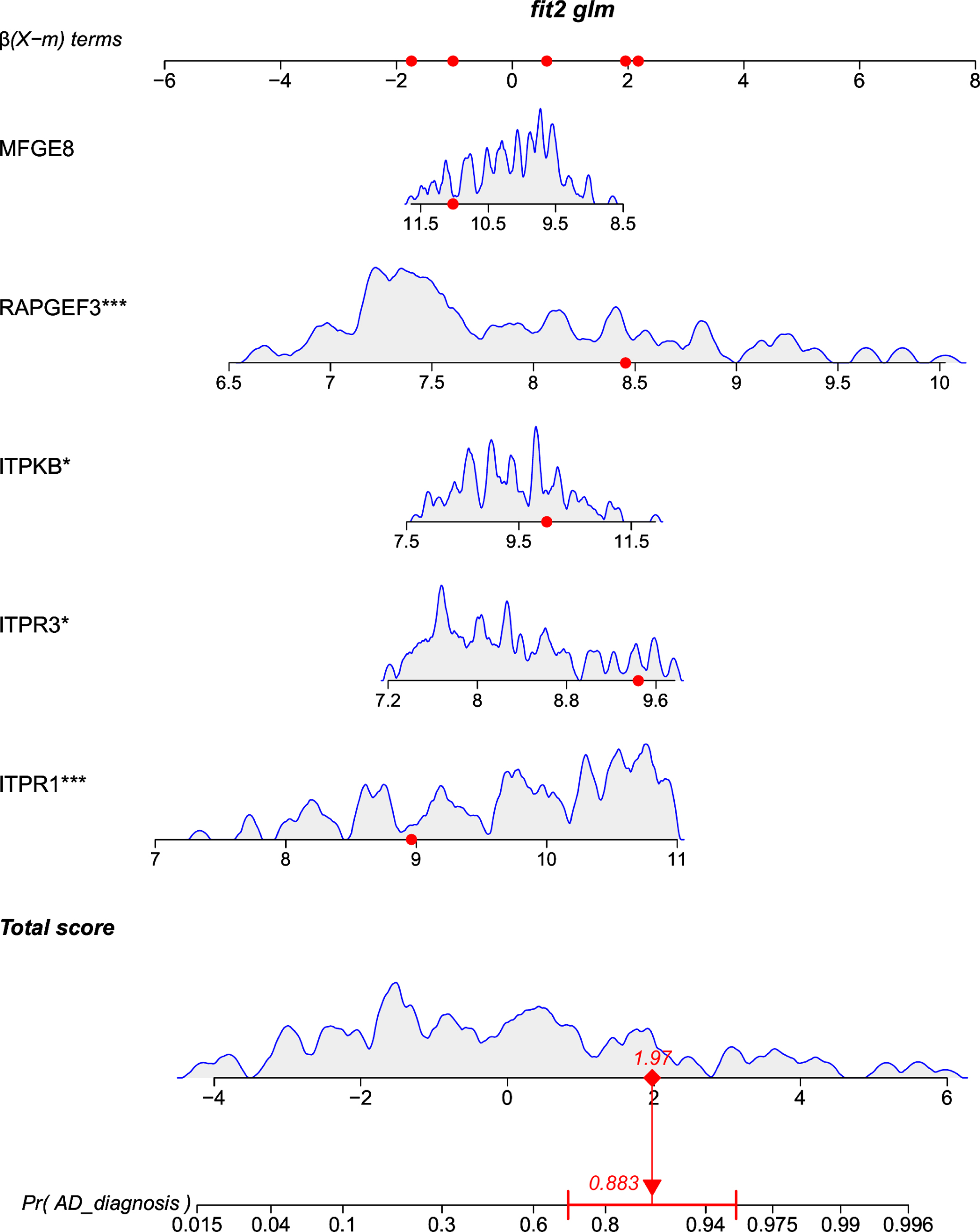
Validation of hub genes by Alzdata
The 4 genes ITPR1, ITPR3, ITPKB, and RAPGEF3 have significant differences in gene expression between AD and normal groups (Fig. 9). RAPGEF3 has a high correlation with Aβ and tau, while ITPR3 has a correlation with Aβ. RAPGEF3 and APP and APOE interact in the PPI network (Supplementary Table 2).
Fig. 9
Differential expression of Cross platform normalized data (AlzData)
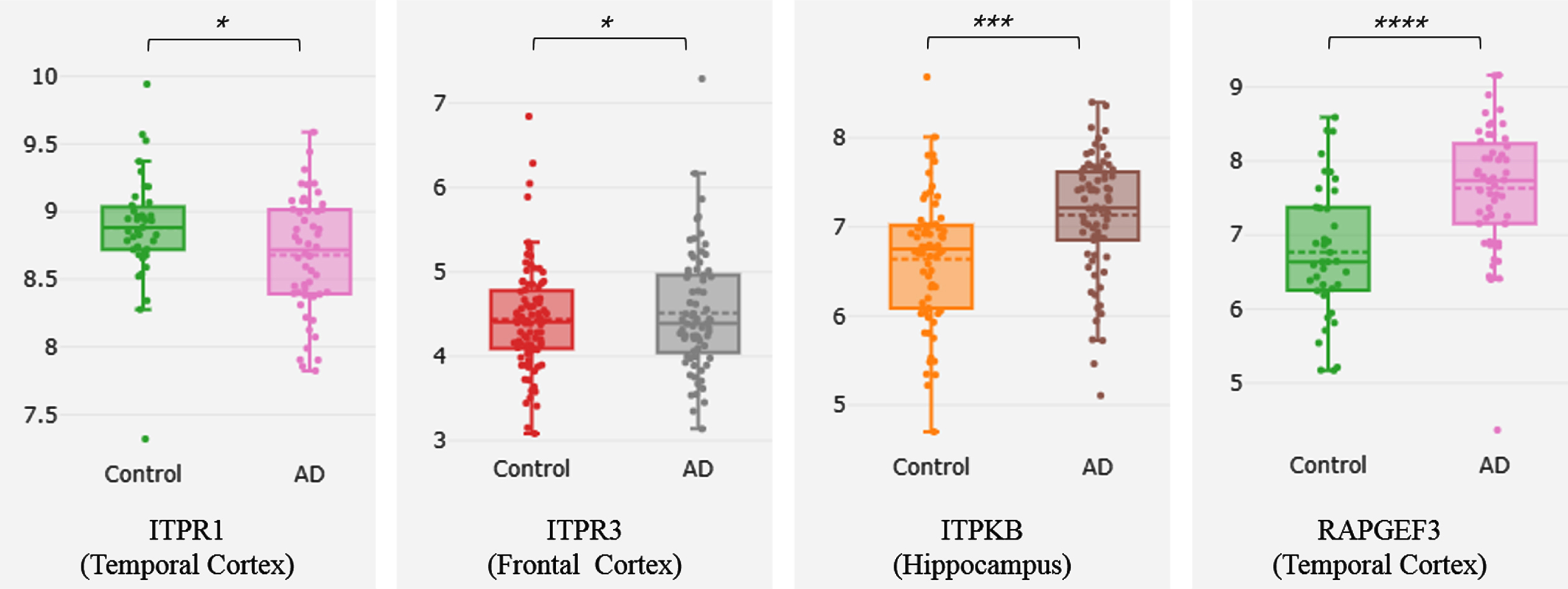
TF-gene interactions
TF-gene interactions showed the interaction of TF genes and 4 hub genes (Fig. 10, Supplementary Table 3). ITPR1, ITPR3, ITPKB, and RAPGEF3 were regulated by 43, 28, 14, and 30 TF genes, respectively. A TF-gene regulatory network of 4 hub genes and common AD risk genes was constructed. Figure 11 and Supplementary Table 4 represent the interaction network between the 7 AD risk genes (ABCA7, APOE, PSEN1, PSEN2, SORL1, PLCG2, BDNF) and the 4 hub genes (ITPR1, ITPR3, ITPKB, RAPGEF3).
Fig. 10
Network of TF-gene interactions with 4 hub genes. The red node represents the hub genes, yellow node represents TF-genes.
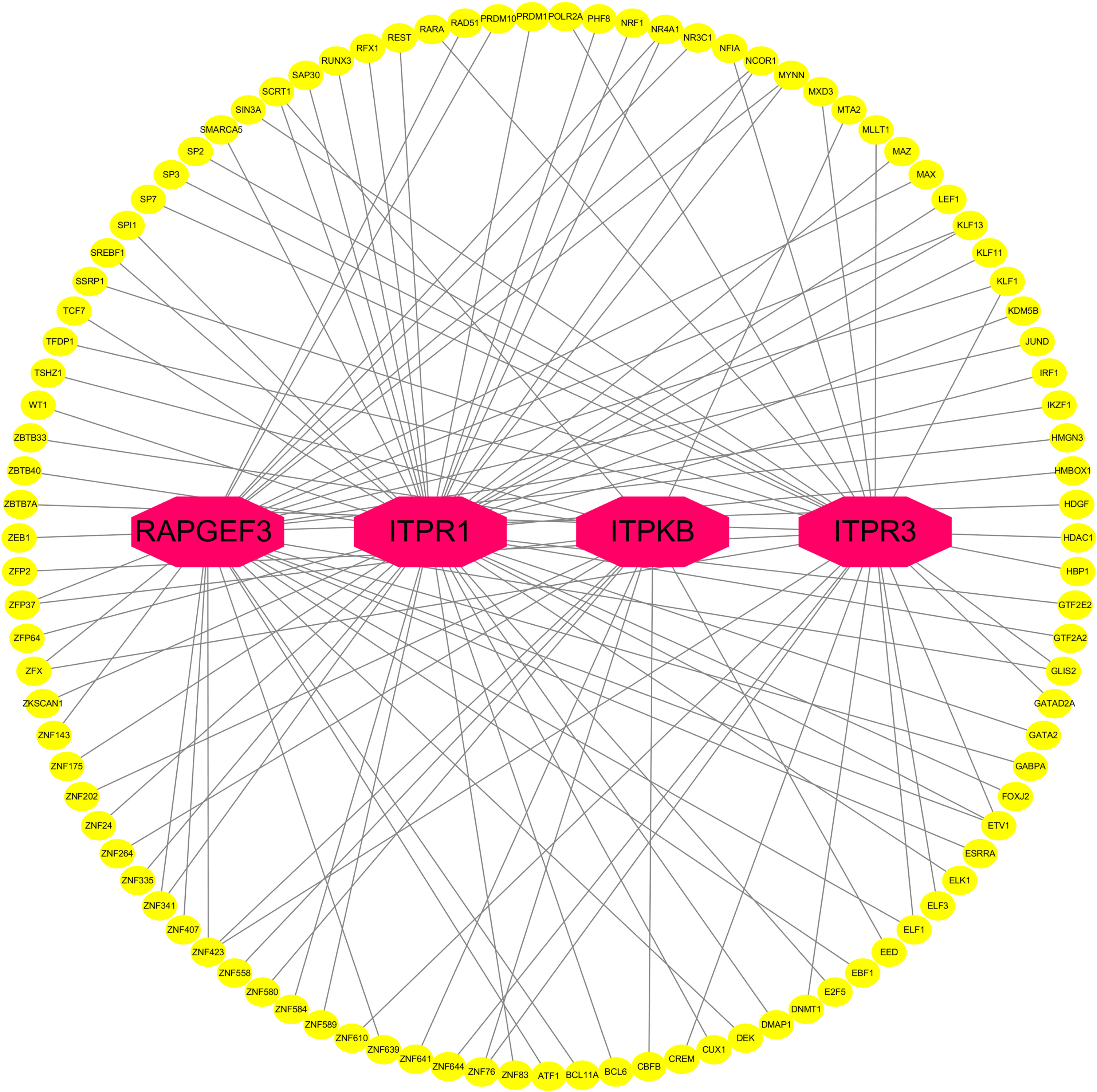
Fig. 11
Network of TF-gene interactions with 4 hub genes and common AD risk genes. AD risk genes: PSEN1, PSEN2, APOE, SORL1, ABCA7, PLCG2, BDNF. Hub genes: ITPR1, ITPR3, ITPKB, RAPGEF3. The red node represents the AD risk genes and hub genes, yellow node represents TF-genes.
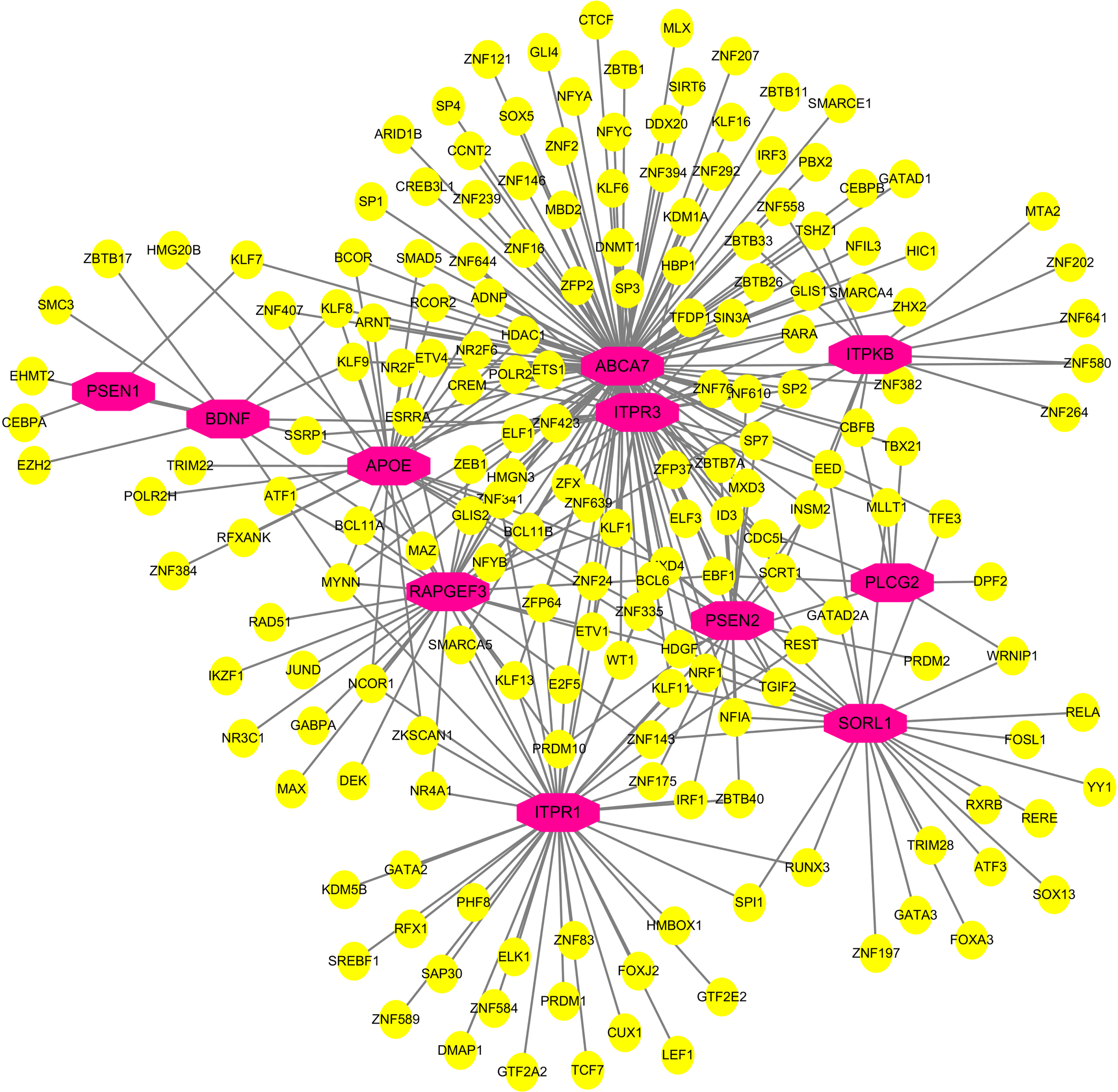
TF-miRNA coregulatory network
TF-miRNA coregulatory network showed the interaction of TF genes and miRNAs with 4 hub genes (Fig. 12, Supplementary Table 5).
Fig. 12
TF-miRNA coregulatory network. The network presents the interactions between TF-genes, miRNA and 4 hub genes. The red color nodes represent the hub genes, yellow nodes are TF-genes and blue nodes indicate miRNA.
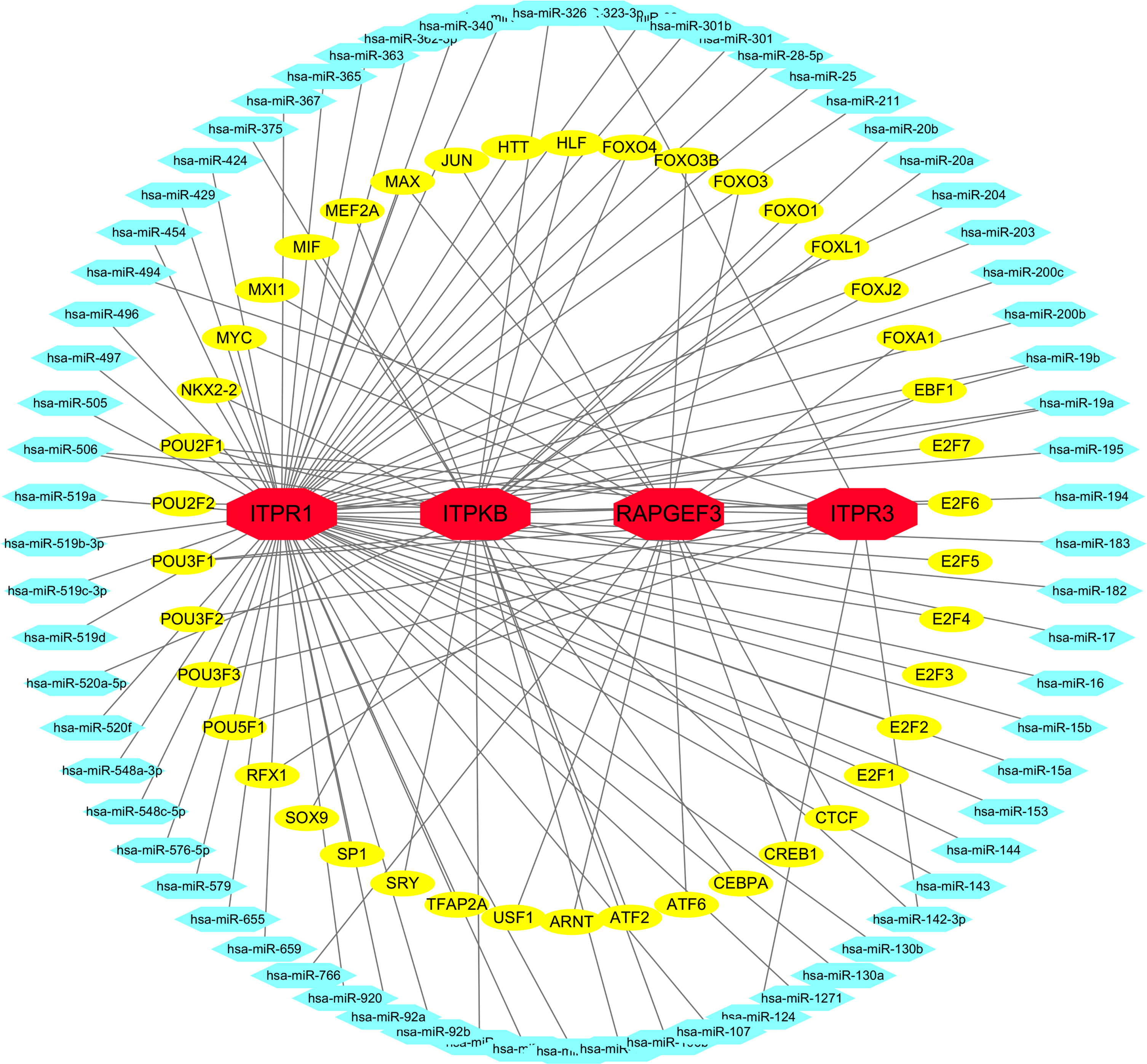
Protein-chemical interactions
We found that resveratrol, genistein, and quercetin were compounds that could interact with most hub genes. Since these compounds were detected against common hub genes, these compounds represented common compounds in COVID-19 and AD (Fig. 13, Supplementary Table 6). Top 20 of the compounds which interact hub genes were listed in Table 4.
Fig. 13
Protein-chemical Interactions by NetworkAnalyst. Suggested compounds that interact with hub genes.
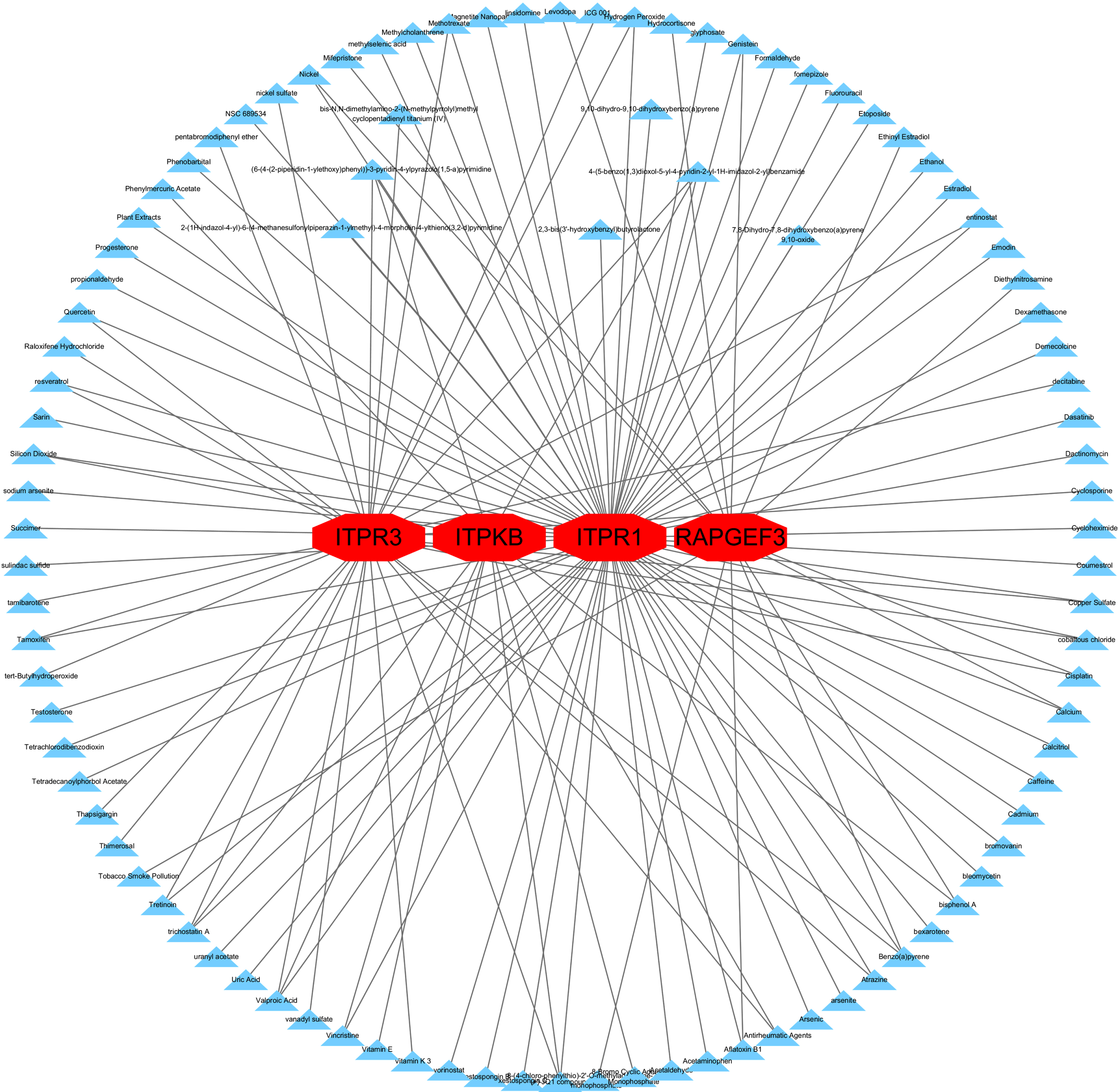
Table 4
Suggested top 20 compounds for AD
Id | Compound | Degree | Betweenness | Genes |
D001564 | Benzo(a)pyrene | 3 | 239.74 | ITPR1, ITPR3, RAPGEF3 |
C459179 | 4-(5-benzo(1,3)dioxol-5-yl-4-pyridin-2-yl-1H-imidazol-2-yl)benzamide | 3 | 110.7 | ITPR1, ITPR3, ITPKB |
C516138 | (6-(4-(2-piperidin-1-ylethoxy)phenyl))-3-pyridin-4-ylpyrazolo(1,5-a)pyrimidine | 3 | 110.7 | ITPR1, ITPR3, ITPKB |
C561695 | (+)-JQ1 compound | 3 | 110.7 | ITPR1, ITPR3, ITPKB |
C012589 | trichostatin A | 3 | 110.7 | ITPR1, ITPR3, ITPKB |
D014635 | Valproic Acid | 3 | 110.7 | ITPR1, ITPR3, ITPKB |
D016604 | Aflatoxin B1 | 2 | 106.21 | ITPR1, RAPGEF3 |
D002118 | Calcium | 2 | 106.21 | ITPR1, RAPGEF3 |
D019833 | Genistein | 2 | 106.21 | ITPR1, RAPGEF3 |
D009532 | Nickel | 2 | 106.21 | ITPR1, RAPGEF3 |
D002945 | Cisplatin | 2 | 80.61 | ITPR3, RAPGEF3 |
D001280 | Atrazine | 2 | 52.91 | ITPR1, ITPR3 |
C018021 | cobaltous chloride | 2 | 52.91 | ITPR1, ITPR3 |
D019327 | Copper Sulfate | 2 | 52.91 | ITPR1, ITPR3 |
C118739 | entinostat | 2 | 52.91 | ITPR1, ITPR3 |
D006861 | Hydrogen Peroxide | 2 | 52.91 | ITPR1, ITPR3 |
D008727 | Methotrexate | 2 | 52.91 | ITPR1, ITPR3 |
D011794 | Quercetin | 2 | 52.91 | ITPR1, ITPR3 |
C059514 | resveratrol | 2 | 52.91 | ITPR1, ITPR3 |
D013629 | Tamoxifen | 2 | 52.91 | ITPR1, ITPR3 |
DISCUSSION
COVID-19 could lead to neurologic sequelae such as AD [3]. Since many studies have reported the pathogenesis of AD in association with COVID-19 [3, 18, 19], AD is of particular concern. We tried to explore the common molecular biology functions and pathways between COVID-19 and AD in order to find biomarkers of AD progression by COVID-19 and provide early intervention.
In this study, we have identified the DEGs of COVID-19 and AD, respectively. Then we found forty common DEGs of AD and COVID-19 and performed bioinformatics analysis. GO indicated that common DEGs were enriched in molecular function of inositol 1,4,5 trisphosphate binding and calcium-release channel activity. KEGG showed that common DEGs were enriched in pathways of apoptosis, long-term potentiation, calcium signaling, cellular senescence, glutamatergic synapse, and AD. The inositol 1,4,5-trisphosphate receptor (IP3R) can mediate calcium-release channel activity and dysfunction of IP3R may play a role in the pathogenesis of AD [20]. The calcium signaling pathway involved in the analysis of KEGG is consistent with the current studies which have confirmed calcium ionic homeostasis imbalance as one of the key mechanisms of AD pathogenesis [21–24]. Common DEGs enriched in pathways of long-term potentiation [25, 26] and glutamatergic synapse [27] may explain to some extent the cause of memory loss in some COVID-19 patients [28], as memory loss is widely known as a clinical manifestation of AD. Then we performed PPI network analysis on common DEGs and identified 5 hub genes (ITPR1, ITPR3, ITPKB, RAPGEF3, MFGE8).
By integrated the gender, age and the expression profiles of 5 hub genes in GSE132903 which included AD patients and the control group, we established a multivariate logistic regression model and found 4 hub genes (ITPR1, ITPR3, ITPKB, RAPGEF3) that were the most important factors affecting AD. ITPR1 (Inositol 1,4,5-Trisphosphate Receptor Type 1) takes part in regulating calcium homeostasis in the endoplasmic reticulum and induces Ca2 + release into the cytosol and may be a potential target for treatment of AD [20, 29]. One study indicated that ITPR3 (Inositol 1,4,5-Trisphosphate Receptor Type 3) is particularly important at mitochondrial calcium and apoptosis modulation [30, 31]. In SH-SY5Y cells, knockout of STIM1 can downregulate the expression of ITPR3 which leads to the reduce of free calcium ion concentration of mitochondria, and finally results in energy metabolism disorders [32]. ITPKB (Inositol-Trisphosphate 3-Kinase B) is necessary for mature T cell and B cell functions [33, 34]. And in AD mouse models, inhibition of ITPKB can reduce the neuroinflammation in microglia [35]. It was also reported that melatonin can attenuate scopolamine-induced memory loss by rescuing EPACs/miR-124/Egr1 pathway [36], with EPAC being an alias for RAPGEF3 gene. EPAC activation in neuronal cells has been confirmed to promote apoptosis [37]. EPAC is also involved in secretion of an amyloid precursor protein which is widely known to be an important mechanism leading to AD [38].
Our bioinformatics analysis and current literature suggests that imbalance in calcium homeostasis would be a shared mechanism to COVID-19 and AD. We speculate that the imbalance of calcium homeostasis in COVID-19 patients may eventually lead to the occurrence and development of AD as calcium homeostasis plays an important role in AD pathogenesis [39]. Recent studies suggested the sequelae of the nervous system in COVID-19 may be due to SARS-CoV-2 infection of central nervous system [40]. The latest study demonstrated virus-induced senescence can be the pathogenic trigger of COVID-19-related cytokine escalation and organ damage [41]. Another research demonstrated COVID-mediated cytokine storm can cause systemic inflammation, including neuroinflammation [42]. Neuroinflammation can then cause an increase in cytokines and reactive oxygen species by activating microglia, and then imbalance of calcium homeostasis in neurons will lead to neuronal necrosis and apoptosis, with a clinical symptom of memory loss [43]. Activation of microglia by calcium homeostasis dysfunction exacerbated disease progression [44]. Researchers found that the interaction between neuroinflammation and neuronal calcium dysregulation may synergistically lead to memory deficits [43, 45]. Since ABCA7 is involved in the phagocytosis and clearance of amyloid-β by microglia [46], the interaction of ABCA7 with ITPR1, ITPR3, and ITPKB shown in Fig. 11 confirms the relationship between neuroinflammation and calcium homeostasis with the development of AD from the perspective of bioinformatics.
In addition, studies have found that since COVID-19 may exacerbate neuroinflammation and calcium homeostasis dysregulation in AD patients, COVID-19 infection can worsen AD conditions [3]. Recent studies showed that APOE4 can aggravate the synaptic loss and neurodegeneration of brain organoids derived from iPSC in AD patients [47], which means that AD patients carrying APOE4 are prone to disease progression after being infected by SARS-CoV-2. The latest research shows that neuronal APOE4 can drive the occurrence and development of AD pathology by influencing immune response genes [48], and the inflammation and immune response that occur in COVID-19 infection are likely to become the inducement for APOE to participate in the pathogenesis of AD. In other words, even in the normal population, COVID-19 infection may induce AD due to the imbalance of calcium homeostasis and neuroinflammation. We speculate that the normal population carrying APOE4 may be more susceptible to AD. Figure 11 showed a network interaction relationship between APOE and other genes such as ABCA7, ITPR1, ITPR3, and ITPKB, which also indicates that APOE is involved in the occurrence and development of AD. This study indicated that APOE is one of the important factors driving AD among the normal population and AD patients with COVID-19 infection. This may also explain to some extent why some COVID-19 patients are more likely to develop AD, and find a reasonable explanation for why the APOE4 genotype population is more likely to suffer from AD.
In this study, we developed an AD risk diagnosis model (ROC_AUC = 0.757) using the common hub genes of COVID-19 and AD. This provides effective strategies and tools for early screening AD risk in COVID-19 patients. which is valuable for the prognosis of COVID-19. We found that the 4 hub genes are not only the DEG of COVID-19, but also participate in the pathogenesis of AD. Therefore, these 4 genes can be considered as biomarkers for predicting the occurrence and development of AD in COVID-19 patients.
Finally, we found the interaction between several compounds and hub genes by NetworkAnalyst, among which resveratrol, genistein, and quercetin were already confirmed to have a neuroprotective effect on AD [49-54]. These compounds could be considered for the prevention and treatment of patients with COVID-19. But we also believe that taking individual protection and vaccination to avoid SARS-CoV-2 infection is the best strategy for individuals.
Conclusion
This paper developed an AD risk diagnosis model based on the common hub gene of COVID-19 patients and AD patients. According to the model and current research, COVID-19 patients are at high risk to develop AD. We highlighted that vaccination is effective and economic for preventing COVID-19. In addition, we found 4 hub genes (ITPR1, ITPR3, ITPKB, RAPGEF3) that can be considered as biomarkers to predict the occurrence and development of AD in COVID-19 patients. This model provides valuable strategies and tools for the prevention and treatment of COVID-19 and AD. Compounds with neuroprotective effects can be used as adjuvant therapy for COVID-19 patients.
ACKNOWLEDGMENTS
This work was supported by the National Key Research and Development Program of China (2016YFC1305900), National Natural Science Foundation of China (82001155, 52007087), Natural Science Foundation of Ningbo (2019A610295), Planning Research Subject of Zhejiang Provincial Education Sciences (2020SCG351), Ningbo Science and Technology plan project (202002N3165), and Natural Science Foundation of Zhejiang province (No. LQ19H090001).
Authors’ disclosures available online (https://www.j-alz.com/manuscript-disclosures/21-5086r2).
SUPPLEMENTARY MATERIAL
[1] The supplementary material is available in the electronic version of this article: https://dx.doi.org/10.3233/JAD-215086.
REFERENCES
[1] | Zou L , Ruan F , Huang M , Liang L , Huang H , Hong Z , Yu J , Kang M , Song Y , Xia J , Guo Q , Song T , He J , Yen HL , Peiris M , Wu J ((2020) ) SARS-CoV-2 viral load in upper respiratory specimens of infected patients, N Engl J Med 382: , 1177–1179. |
[2] | Kannan S , Shaik Syed Ali P , Sheeza A , Hemalatha K ((2020) ) COVID-19 (Novel Coronavirus 2019) - recent trends, Eur Rev Med Pharmacol Sci 24: , 2006–2011. |
[3] | Wang F , Kream RM , Stefano GB ((2020) ) Long-term respiratory and neurological sequelae of COVID-19, Med Sci Monit 26: , e928996. |
[4] | Mao L , Jin H , Wang M , Hu Y , Chen S , He Q , Chang J , Hong C , Zhou Y , Wang D , Miao X , Li Y , Hu B ((2020) ) Neurologic manifestations of hospitalized patients with coronavirus disease 2019 in Wuhan, China, JAMA Neurol 77: , 683–690. |
[5] | Xiong N , Schiller MR , Li J , Chen X , Lin Z ((2021) ) Severe COVID-19 in Alzheimer’s disease: APOE4’s fault again? Alzheimers Res Ther 13: , 111. |
[6] | Blanco-Melo D , Nilsson-Payant BE , Liu WC , Uhl S , Hoagland D , Moller R , Jordan TX , Oishi K , Panis M , Sachs D , Wang TT , Schwartz RE , Lim JK , Albrecht RA , tenOever BR ((2020) ) Imbalanced host response to SARS-CoV-2 drives development of COVID-19, Cell 181: , 1036–1045 e1039. |
[7] | Piras IS , Krate J , Delvaux E , Nolz J , Mastroeni DF , Persico AM , Jepsen WM , Beach TG , Huentelman MJ , Coleman PD ((2019) ) Transcriptome changes in the Alzheimer’s disease middle temporal gyrus: Importance of RNA metabolism and mitochondria-associated membrane genes, J Alzheimers Dis 70: , 691–713. |
[8] | Love MI , Huber W , Anders S ((2014) ) Moderated estimation of fold change and dispersion for RNA-seq data with DESeq2, Genome Biol 15: , 550. |
[9] | Ritchie ME , Phipson B , Wu D , Hu Y , Law CW , Shi W , Smyth GK ((2015) ) limma powers differential expression analyses for RNA-sequencing and microarray studies, Nucleic Acids Res 43: , e47. |
[10] | Yu G , Wang LG , Han Y , He QY ((2012) ) clusterProfiler: An R package for comparing biological themes among gene clusters, OMICS 16: , 284–287. |
[11] | Chin CH , Chen SH , Wu HH , Ho CW , Ko MT , Lin CY ((2014) ) cytoHubba: Identifying hub objects and sub-networks from complex interactome, BMC Syst Biol 8 Suppl 4: , S11. |
[12] | Balachandran VP , Gonen M , Smith JJ , DeMatteo RP ((2015) ) Nomograms in oncology: More than meets the eye, Lancet Oncol 16: , e173–180. |
[13] | Xu M , Zhang DF , Luo R , Wu Y , Zhou H , Kong LL , Bi R , Yao YG ((2018) ) A systematic integrated analysis of brain expression profiles reveals YAP1 and other prioritized hub genes as important upstream regulators in Alzheimer’s disease, Alzheimers Dement 14: , 215–229. |
[14] | Zhang DF , Fan Y , Xu M , Wang G , Wang D , Li J , Kong LL , Zhou H , Luo R , Bi R , Wu Y , Li GD , Alzheimer’s Disease Neuroimaging I, Li M , Luo XJ , Jiang HY , Tan L , Zhong C , Fang Y , Zhang C , Sheng N , Jiang T , Yao YG ((2019) ) Complement C7 is a novel risk gene for Alzheimer’s disease in Han Chinese, Natl Sci Rev 6: , 257–274. |
[15] | Zhou G , Soufan O , Ewald J , Hancock REW , Basu N , Xia J ((2019) ) NetworkAnalyst 3.0: A visual analytics platform for comprehensive gene expression profiling and meta-analysis, Nucleic Acids Res 47: , W234–W241. |
[16] | Choi SH , Bylykbashi E , Chatila ZK , Lee SW , Pulli B , Clemenson GD , Kim E , Rompala A , Oram MK , Asselin C , Aronson J , Zhang C , Miller SJ , Lesinski A , Chen JW , Kim DY , van Praag H , Spiegelman BM , Gage FH , Tanzi RE ((2018) ) Combined adult neurogenesis and BDNF mimic exercise effects on cognition in an Alzheimer’s mouse model, Science 361: , eaan8821. |
[17] | Scheltens P , De Strooper B , Kivipelto M , Holstege H , Chetelat G , Teunissen CE , Cummings J , van der Flier WM ((2021) ) Alzheimer’s disease, Lancet 397: , 1577–1590. |
[18] | Pezzini A , Padovani A ((2020) ) Lifting the mask on neurological manifestations of COVID-19, Nat Rev Neurol 16: , 636–644. |
[19] | Rahman MA , Islam K , Rahman S , Alamin M ((2021) ) Neurobiochemical cross-talk between COVID-19 and Alzheimer’s disease, Mol Neurobiol 58: , 1017–1023. |
[20] | Egorova PA , Bezprozvanny IB ((2018) ) Inositol 1,4,5-trisphosphate receptors and neurodegenerative disorders, FEBS J 285: , 3547–3565. |
[21] | Tong BC , Wu AJ , Li M , Cheung KH ((2018) ) Calcium signaling in Alzheimer’s disease & therapies, Biochim Biophys Acta Mol Cell Res 1865: , 1745–1760. |
[22] | Gibson GE , Thakkar A ((2017) ) Interactions of mitochondria/metabolism and calcium regulation in Alzheimer’s disease: A calcinist point of view, Neurochem Res 42: , 1636–1648. |
[23] | O’Neill C , Cowburn RF , Bonkale WL , Ohm TG , Fastbom J , Carmody M , Kelliher M ((2001) ) Dysfunctional intracellular calcium homoeostasis: A central cause of neurodegeneration in Alzheimer’s disease, Biochem Soc Symp, pp. 177–194. |
[24] | Supnet C , Bezprozvanny I ((2010) ) The dysregulation of intracellular calcium in Alzheimer disease, Cell Calcium 47: , 183–189. |
[25] | Shetty MS , Sharma M , Sajikumar S ((2017) ) Chelation of hippocampal zinc enhances long-term potentiation and synaptic tagging/capture in CA1 pyramidal neurons of aged rats: Implications to aging and memory, Aging Cell 16: , 136–148. |
[26] | Prieto GA , Trieu BH , Dang CT , Bilousova T , Gylys KH , Berchtold NC , Lynch G , Cotman CW ((2017) ) Pharmacological rescue of long-term potentiation in Alzheimer diseased synapses, J Neurosci 37: , 1197–1212. |
[27] | Revett TJ , Baker GB , Jhamandas J , Kar S ((2013) ) Glutamate system, amyloid ss peptides and tau protein: Functional interrelationshis and relevance to Alzheimer disease pathology. J Psychiatry Neurosci 38: , 6–23 . |
[28] | Blomberg B , Mohn KG , Brokstad KA , Zhou F , Linchausen DW , Hansen BA , Lartey S , Onyango TB , Kuwelker K , Saevik M , Bartsch H , Tondel C , Kittang BR , Bergen C-RG , Cox RJ , Langeland N ((2021) ) Long COVID in a prospective cohort of home-isolated patients, Nat Med 27: , 1607–1613. |
[29] | Takada SH , Ikebara JM , de Sousa E , Cardoso DS , Resende RR , Ulrich H , Ruckl M , Rudiger S , Kihara AH ((2017) ) Determining the roles of inositol trisphosphate receptors in neurodegeneration: Interdisciplinary perspectives on a complex topic, Mol Neurobiol 54: , 6870–6884. |
[30] | Guerra MT , Florentino RM , Franca A , Lima Filho AC , Dos Santos ML , Fonseca RC , Lemos FO , Fonseca MC , Kruglov E , Mennone A , Njei B , Gibson J , Guan F , Cheng YC , Ananthanarayanan M , Gu J , Jiang J , Zhao H , Lima CX , Vidigal PT , Oliveira AG , Nathanson MH , Leite MF ((2019) ) Expression of the type 3 InsP3 receptor is a final common event in the development of hepatocellular carcinoma, Gut 68: , 1676–1687. |
[31] | Filadi R , Leal NS , Schreiner B , Rossi A , Dentoni G , Pinho CM , Wiehager B , Cieri D , Cali T , Pizzo P , Ankarcrona M ((2018) ) TOM70 sustains cell bioenergetics by promoting IP3R3-mediated ER to mitochondria Ca(2+) transfer, Curr Biol 28: , 369–382 e366. |
[32] | Pascual-Caro C , Orantos-Aguilera Y , Sanchez-Lopez I , de Juan-Sanz J , Parys JB , Area-Gomez E , Pozo-Guisado E , Martin-Romero FJ ((2020) ) STIM1 deficiency leads to specific down-regulation of ITPR3 in SH-SY5Y cells, Int J Mol Sci 21: , 6598. |
[33] | Thangavelu G , Du J , Paz KG , Loschi M , Zaiken MC , Flynn R , Taylor PA , Kirchmeier AK , Panoskaltsis-Mortari A , Luznik L , MacDonald KP , Hill GR , Maillard I , Munn DH , Serody JS , Murphy WJ , Miklos D , Cutler CS , Koreth J , Antin JH , Soiffer RJ , Ritz J , Dahlberg C , Miller AT , Blazar BR ((2020) ) Inhibition of inositol kinase B controls acute and chronic graft-versus-host disease, Blood 135: , 28–40. |
[34] | Schurmans S , Pouillon V , Marechal Y ((2011) ) Regulation of B cell survival, development and function by inositol 1,4,5-trisphosphate 3-kinase B (Itpkb), Adv Enzyme Regul 51: , 66–73. |
[35] | Zhang Y , Xu C , Nan Y , Nan S ((2020) ) Microglia-derived extracellular vesicles carrying miR-711 alleviate neurodegeneration in a murine Alzheimer’s disease model by binding to Itpkb, Front Cell Dev Biol 8: , 566530. |
[36] | Wang X , Wang ZH , Wu YY , Tang H , Tan L , Wang X , Gao XY , Xiong YS , Liu D , Wang JZ , Zhu LQ ((2013) ) Melatonin attenuates scopolamine-induced memory/synaptic disorder by rescuing EPACs/miR-124/Egr1 pathway, Mol Neurobiol 47: , 373–381. |
[37] | Suzuki S , Yokoyama U , Abe T , Kiyonari H , Yamashita N , Kato Y , Kurotani R , Sato M , Okumura S , Ishikawa Y ((2010) ) Differential roles of Epac in regulating cell death in neuronal and myocardial cells, J Biol Chem 285: , 24248–24259. |
[38] | ZalduaN, GastineauM, HoshinoM, Lezoualc’hF, ZugazaJL ((2007) ) Epac signaling pathway involves STEF, a guanine nucleotide exchange factor for Rac, to regulate APP processing. FEBS Lett 581: , 5814–5818. |
[39] | Calvo-Rodriguez M , Bacskai BJ ((2021) ) Mitochondria and calcium in Alzheimer’s disease: From cell signaling to neuronal cell death, Trends Neurosci 44: , 136–151. |
[40] | Roy D , Ghosh R , Dubey S , Dubey MJ , Benito-Leon J , Kanti Ray B ((2021) ) Neurological and neuropsychiatric impacts of COVID-19 pandemic, Can J Neurol Sci 48: , 9–24. |
[41] | Lee S , Yu Y , Trimpert J , Benthani F , Mairhofer M , Richter-Pechanska P , Wyler E , Belenki D , Kaltenbrunner S , Pammer M , Kausche L , Firsching TC , Dietert K , Schotsaert M , Martinez-Romero C , Singh G , Kunz S , Niemeyer D , Ghanem R , Salzer HJF , Paar C , Mulleder M , Uccellini M , Michaelis EG , Khan A , Lau A , Schonlein M , Habringer A , Tomasits J , Adler JM , Kimeswenger S , Gruber AD , Hoetzenecker W , Steinkellner H , Purfurst B , Motz R , Di Pierro F , Lamprecht B , Osterrieder N , Landthaler M , Drosten C , Garcia-Sastre A , Langer R , Ralser M , Eils R , Reimann M , Fan DNY , Schmitt CA ((2021) ) Virus-induced senescence is driver and therapeutic target in COVID-19, Nature, doi: 10.1038/s41586-021-03995-1. |
[42] | Gao YM , Xu G , Wang B , Liu BC ((2021) ) Cytokine storm syndrome in coronavirus disease 2019: A narrative review, J Intern Med 289: , 147–161. |
[43] | Hopp SC , D’Angelo HM , Royer SE , Kaercher RM , Crockett AM , Adzovic L , Wenk GL ((2015) ) Calcium dysregulation via L-type voltage-dependent calcium channels and ryanodine receptors underlies memory deficits and synaptic dysfunction during chronic neuroinflammation, J Neuroinflammation 12: , 56. |
[44] | Cheng J , Dong Y , Ma J , Pan R , Liao Y , Kong X , Li X , Li S , Chen P , Wang L , Yu Y , Yuan Z ((2021) ) Microglial Calhm2 regulates neuroinflammation and contributes to Alzheimer’s disease pathology, Sci Adv 7: , eabe3600. |
[45] | Sama DM , Norris CM ((2013) ) Calcium dysregulation and neuroinflammation: Discrete and integrated mechanisms for age-related synaptic dysfunction, Ageing Res Rev 12: , 982–995. |
[46] | Li H , Karl T , Garner B ((2015) ) Understanding the function of ABCA7 in Alzheimer’s disease, Biochem Soc Trans 43: , 920–923. |
[47] | Zhao J , Fu Y , Yamazaki Y , Ren Y , Davis MD , Liu CC , Lu W , Wang X , Chen K , Cherukuri Y , Jia L , Martens YA , Job L , Shue F , Nguyen TT , Younkin SG , Graff-Radford NR , Wszolek ZK , Brafman DA , Asmann YW , Ertekin-Taner N , Kanekiyo T , Bu G ((2020) ) APOE4 exacerbates synapse loss and neurodegeneration in Alzheimer’s disease patient iPSC-derived cerebral organoids, Nat Commun 11: , 5540. |
[48] | Zalocusky KA , Najm R , Taubes AL , Hao Y , Yoon SY , Koutsodendris N , Nelson MR , Rao A , Bennett DA , Bant J , Amornkul DJ , Xu Q , An A , Cisne-Thomson O , Huang Y ((2021) ) Neuronal ApoE upregulates MHC-I expression to drive selective neurodegeneration in Alzheimer’s disease, Nat Neurosci 24: , 786–798. |
[49] | Moussa C , Hebron M , Huang X , Ahn J , Rissman RA , Aisen PS , Turner RS ((2017) ) Resveratrol regulates neuro-inflammation and induces adaptive immunity in Alzheimer’s disease, J Neuroinflammation 14: , 1. |
[50] | Kou X , Chen N ((2017) ) Resveratrol as a natural autophagy regulator for prevention and treatment of Alzheimer’s disease, Nutrients 9: , 927. |
[51] | Yan Y , Yang H , Xie Y , Ding Y , Kong D , Yu H ((2020) ) Research progress on Alzheimer’s disease and resveratrol, Neurochem Res 45: , 989–1006. |
[52] | Devi KP , Shanmuganathan B , Manayi A , Nabavi SF , Nabavi SM ((2017) ) Molecular and therapeutic targets of genistein in Alzheimer’s disease, Mol Neurobiol 54: , 7028–7041. |
[53] | Pierzynowska K , Podlacha M , Gaffke L , Majkutewicz I , Mantej J , Wegrzyn A , Osiadly M , Myslinska D , Wegrzyn G ((2019) ) Autophagy-dependent mechanism of genistein-mediated elimination of behavioral and biochemical defects in the rat model of sporadic Alzheimer’s disease, Neuropharmacology 148: , 332–346. |
[54] | Khan H , Ullah H , Aschner M , Cheang WS , Akkol EK ((2019) ) Neuroprotective effects of quercetin in Alzheimer’s disease, Biomolecules 10: , 59. |