Neural Correlates of Mild Behavioral Impairment: A Functional Brain Connectivity Study Using Resting-State Functional Magnetic Resonance Imaging
Abstract
Background:
Mild behavioral impairment (MBI) is associated with accelerated cognitive decline and greater risk of dementia. However, the neural correlates of MBI have not been completely elucidated.
Objective:
The study aimed to investigate the correlation between cognitively normal participants and participants with amnestic mild cognitive impairment (aMCI) using resting-state functional magnetic resonance imaging.
Methods:
The study included 30 cognitively normal participants and 13 participants with aMCI (20 men and 23 women; mean age, 76.9 years). The MBI was assessed using the MBI checklist (MBI-C). Region of interest (ROI)-to-ROI analysis was performed to examine the correlation between MBI-C scores and functional connectivity (FC) of the default mode network, salience network, and frontoparietal control network (FPCN). Age, Mini-Mental State Examination score, sex, and education were used as covariates. A p-value of 0.05, with false discovery rate correction, was considered significant.
Results:
A negative correlation was observed between the MBI-C total score and FC of the left posterior parietal cortex with the right middle frontal gyrus. A similar result was obtained for the MBI-C affective dysregulation domain score.
Conclusion:
FPCN dysfunction was detected as a neural correlate of MBI, especially in the affective dysregulation domain. This dysfunction may be associated with cognitive impairment in MBI and conversion of MBI to dementia; however, further longitudinal data are needed to examine this relationship.
INTRODUCTION
The neural correlates of neuropsychiatric symptoms (NPS) have not been completely elucidated. For example, the results for neural correlates of NPS in Alzheimer’s disease (AD) are inconsistent; however, the involvement of the anterior and posterior cingulate, frontal lobes, hippocampus, insula, and amygdala has been suggested [1, 2]. These inconsistencies could be explained, in certain cases, when the NPS are not caused by damage to specific brain regions but rather due to dysfunction of certain regions within the specific brain networks. There are three important brain networks in this regard: the default mode network (DMN), salience network (SN), and fronto-parietal control network (FPCN). The DMN includes the medial prefrontal cortex, posterior cingulate cortex, and bilateral inferior parietal cortex, and is activated in the resting state [3]; while the FPCN, which is similar to the central executive network (CEN), includes the dorsolateral prefrontal cortex, anterior prefrontal cortex, anterior inferior parietal lobule, and lateral intraparietal sulcus, and supports the decision-making processes and cognitive control [4, 5]. The SN includes the anterior cingulate cortex and orbital frontoinsula and is involved in interoceptive autonomic processing [4]. The right frontoinsula within the SN plays an important role in switching between the DMN and the CEN [6].
The neural correlates of NPS using functional magnetic resonance imaging (fMRI) have demonstrated the association of impairment in the DMN with apathy in mild cognitive impairment (MCI) [7], multiple NPS in MCI and AD [8], and hyperactivity in AD [9]. Changes in the SN have been correlated with agitation [10] and hyperactivity syndrome [11] in AD, while greater affective symptoms and apathy in MCI have been associated with FPCN [7, 12]. These results indicate the importance of the DMN, SN, and FPCN as neural correlates of NPS.
Since NPS in older individuals is considered a risk factor for dementia, new criteria for mild behavioral impairment (MBI) were proposed by the International Society to Advance Alzheimer’s Research and Treatment (ISAART) [13]. According to these criteria, MBI comprises five domains: decreased motivation, affective dysregulation, impulse dyscontrol, social inappropriateness, and abnormal thoughts and perception. MBI has been shown to accelerate cognitive decline [14, 15] and increase the risk of dementia [15–17]; however, the neural correlates of MBI have not been completely elucidated. Based on the MRI, MBI in Parkinson’s disease (PD) has been related to the right middle temporal cortex [18], and with the DMN, SN, and FPCN using fMRI [19, 20]. However, the neural correlates of MBI in the AD continuum may differ from those of the MBI in PD. Very recently, an MRI study in a memory clinic on patients with subjective cognitive decline (SCD) and MCI detected correlations between MBI and entorhinal and hippocampal atrophy [21]. MBI has also been associated with a greater increase in plasma neurofilament light levels in cognitively normal individuals and individuals with MCI, suggesting a link with accelerated neuropathological changes or faster or greater neurodegeneration [22]. Therefore, revealing the neural correlates of MBI might demonstrate how MBI is involved in the cognitive decline and increased risk of dementia, thereby adding to the very scant literature, which is in its infancy. This study aimed to investigate these neural correlates in cognitively normal participants and participants with amnestic MCI (aMCI) using resting-state fMRI.
METHODS
Participants
Volunteers or outpatients at the Center for Diagnosis of Dementia, Kyoto Prefectural University of Medicine were recruited. The inclusion criteria were age≥60 years and maintenance of activities of daily living. The exclusion criteria were brain injury, dementia, anti-dementia drug treatment, inability to undergo an MRI scan, history of mental illness, intellectual disability, drug or alcohol abuse, serious impairment of vision or hearing, and use of both hands. Using these criteria, 43 older adults were enrolled in the study, including 30 cognitively normal participants and 13 participants with aMCI. Cognitively normal was defined as a clinical dementia rating (CDR) [23] of 0. aMCI was diagnosed using a combination of a CDR of 0.5, CDR memory score of 0.5 or 1, and Petersen criteria [24]. The assessments were reviewed by a psychiatrist and psychologists, and the diagnosis was made by the psychiatrist. This study was approved by the ethics committee of the Kyoto Prefectural University of Medicine (ERB-C-853-5). Informed consent was obtained from all the participants. Of the 43 participants, 27 were included in a previous report [25]; however, the aim of the previous study, which dealt with interoceptive accuracy, differed from that of the current study.
Assessments
The MBI checklist (MBI-C, available at http://www.MBItest.org) [26] was used to assess the MBI. The MBI-C consists of 34 items (decreased motivation, 6 items; affective dysregulation, 6 items; impulse dyscontrol, 12 items; social inappropriateness, 5 items; and abnormal thoughts and perception, 5 items). These five domains were supported by factor analysis [27]. In each item, the score was 0 if the symptom was absent, did not represent a change from a longstanding pattern of behavior, or did not persist for at least 6 months. If the symptom is endorsed, the score is 1 (mild: noticeable but not a significant change); 2 (moderate: significant but not a dramatic change); or 3 (severe: marked or prominent, a dramatic change). Therefore, the MBI-C total score is calculated by summing up the five domain scores (total score range, 0 to 102) with a higher score representing a more severe symptomatology.
The MBI-C was translated into Japanese with the participation of the developer, following a standard forward and back-translation procedure to confirm the semantic equivalence of the Japanese version with the original English version. The initial Japanese MBI-C version was preliminarily administered to five participants with MCI. Minor modifications were then made to develop the final version. In the current study, the MBI-C was self-reported by the participants. The Mini-Mental State Examination (MMSE) [28] was also administered to evaluate cognitive function. The assessments and MRI scans were performed on the same day.
Image acquisition
MRI was performed using a 3T Philips Achieva scanner (Philips, Best, The Netherlands). T1-weighted three-dimensional magnetization-prepared rapid gradient-echo (MPRAGE) images were obtained with repetition time, 6.8 ms; echo time, 3.1 ms; field of view, 256×256 mm2; flip angle, 9°; matrix, 256×256; resolution, 1.0×1.0×1.2 mm; and number of sagittal slices, 170. A 10 min resting-state fMRI scan was also performed using T2*-weighted echo planar sequence with repetition time, 2500 ms; echo time, 30 ms; field of view, 212×212 mm2; flip angle, 80°; matrix, 64×64; resolution, 3.3×3.3×3.2 mm; number of axial slices, 40; 0.8 mm gaps, and interleaved collection, during which the participants glanced fixedly at a black cross in the center of a white screen.
Statistical analyses
Independent group t-tests and chi-square tests were performed to compare the two groups using the SPSS 24 software (IBM Corp., Armonk, NY, USA). Statistical significance was set at p < 0.05.
The structural and functional images were preprocessed using CONN Toolbox ver.18.b [29] running in MATLAB R2019b (MathWorks, Inc., Natick, MA, USA). After discarding the initial ten functional images, functional realignment, subject-motion estimation and correction, slice timing correction, segmentation, and normalization to Montreal Neurological Institute (MNI) atlas space were performed. Finally, the normalized images were smoothed with an 8 mm full width at half maximum (FWHM) Gaussian kernel. Artifact detection tools-based identification of outlier scans for scrubbing was used to detect functional outliers in preprocessing in CONN. In the first-level analysis, realignment parameter, quality assurance timeseries, and scrubbing parameter were used as covariates. Denoising tool in CONN was also used to eliminate the effects of motion, white matter (WM) and cerebrospinal fluid (CSF) noise, and other physiological noise. After creating the ROI-to-ROI and voxel-to-voxel connectivity matrices for each participant at the first-level analysis, general linear model was conducted using the data acquired at the first level to obtain group-level estimates at the second-level analysis [29].
Region of interest (ROI)-to-ROI analysis was performed as described in our previous study [25]. Seeds were selected from within the DMN, SN, and FPCN. The anatomical locations were the medial prefrontal cortex, bilateral lateral parietal, and posterior cingulate cortex for the DMN seeds, the bilateral rostral prefrontal cortex, anterior cingulate cortex, bilateral anterior insular cortex, and bilateral supramarginal gyrus for the SN seeds, and the bilateral lateral prefrontal cortex and bilateral posterior parietal cortex for the FPCN seeds. ROIs were a set of whole brain 164 ROIs provided by the CONN software. In the second-level analysis, one-sample general linear model analyses were performed to examine the regression between MBI-C scores and functional connectivity (FC) of the DMN, SN, and FPCN. Age, sex, education, and MMSE scores were used as covariates. A p-value of 0.05, with false discovery rate (FDR) correction, was considered significant.
To identify the FC correlates with the MBI-C score, group-level independent component analysis (ICA) was performed using the CONN toolbox ver.18.b, as in our previous study [25]. The number of independent components to be estimated was set to 40, and the dimensionality reduction was set to 64. The summary option in the ICA networks’ second-level results was used to search the estimated ICA networks. Spatial correlations between each component and the CONN default network file were calculated using the “compute spatial match to template” option using the ICA tools. Components associated with the DMN, SN, and FPCN were determined by visual inspection. In the second-level analysis, the determined ICA networks were entered into a general linear model, which was used to examine the correlations with the MBI-C score using age, sex, education, and MMSE score as covariates. Statistical thresholds were set to uncorrected p < 0.001 at the voxel level and to FDR corrected p < 0.05 at the cluster level.
Voxel-based morphometry (VBM) was performed using Statistical Parametric Mapping (SPM) 12 (Wellcome Department of Cognitive Neurology, University College, London, UK) in MATLAB R2019b. MPRAGE images were segmented into gray matter (GM), WM, and CSF with bias correction. The template for diffeomorphic anatomical registration through exponentiated lie algebra (DARTEL) was created using data acquired during the segmentation process. Next, each segmented GM image was spatially warped to the MNI space using the DARTEL algorithm with the created template. Finally, the images were smoothed using an 8 mm FWHM filter. In image analysis, multiple regression analysis was performed to examine the relationship between the MBI score and regional GM volume. Age, sex, education, MMSE score, and total intracranial volume (sum of the GM, WM, and CSF volumes) were used as covariates. Thresholds were set to a p-value of 0.001 (uncorrected) at the voxel level and to a p-value of 0.05 (FDR corrected) at the cluster level.
RESULTS
Participants
The clinical characteristics of the 43 participants are shown in Table 1. Using a cut-off score of 6.5 [30], 5/43 total participants (12%), 3/30 cognitive normal participants (10%), and 2/13 participants with MCI (15%) had MBI. Eight participants (19%) were taking psychotropic drugs, including suvorexant (n = 2), zolpidem (n = 2), flunitrazepam (n = 2), triazolam (n = 1), brotizolam (n = 1), etizolam (n = 1), and duloxetine (n = 1). The characteristics of the participants taking and not taking these drugs were not significantly different.
Table 1
Clinical characteristics of the participants
Characteristic | All participants (n = 43) | Cognitive normal participants (n = 30) | Participants with MCI (n = 13) |
Age, y | 76.9±5.7 | 76.1±5.7 | 78.8±5.4 |
Sex, male/female | 20/23 | 12/18 | 8/5 |
Education, y | 13.3±2.4 | 13.2±2.3 | 13.6±2.8 |
MMSE score | 27.6±2.5 | 28.3±2.2 | 26.1±2.6 |
CDR, 0/0.5 | 30/13 | 30/0 | 0/13 |
MBI-C total (range) | 2.7±4.2 (0–20) | 2.7±4.9 (0–20) | 2.6±2.5 (0–7) |
MBI-C total score≥1, number (%) | 29 (67) | 19 (63) | 10 (77) |
MBI-C decreased motivation (range) | 0.7±1.4 (0–6) | 0.6±1.4 (0–6) | 1.0±1.2 (0–3) |
MBI-C decreased motivation score≥1, number (%) | 14 (33) | 7 (23) | 7 (54) |
MBI-C affective dysregulation (range) | 1.0±1.6 (0–8) | 1.1±1.8 (0–8) | 0.8±1.0 (0–3) |
MBI-C affective dysregulation score≥1, number (%) | 19 (44) | 13 (43) | 6 (46) |
MBI-C impulse dyscontrol (range) | 0.8±1.5 (0–7) | 0.9±1.7 (0–7) | 0.5±1.0 (0–3) |
MBI-C impulse dyscontrol score≥1, number (%) | 17 (40) | 13 (43) | 4 (31) |
MBI-C social inappropriateness (range) | 0.1±0.5 (0–3) | 0.1±0.3 (0–1) | 0.3±0.9 (0–3) |
MBI-C social inappropriateness score≥1, number (%) | 4 (9.3) | 2 (6.7) | 2 (15) |
MBI-C abnormal thought and perception (range) | 0.0±0.2 (0–1) | 0.0±0.2 (0–1) | 0.0±0.0 (0) |
MBI-C abnormal thought and perception score≥1, number (%) | 1 (2.3) | 1 (3.3) | 0 (0) |
CDR, Clinical Dementia Rating; MBI-C, mild behavioral impairment checklist; MCI, mild cognitive impairment; MMSE, Mini- Mental State Examination. Data are shown as the mean±standard deviation, except for sex and CDR.
ROI-to-ROI analysis
A negative correlation was observed between the MBI-C total score and FC of the left posterior parietal cortex with the right middle frontal gyrus (t [37] = –4.39, FDR-corrected p = 0.015) (Fig. 1). The MBI-C affective dysregulation score was also negatively correlated with FC between the left posterior parietal cortex and right middle frontal gyrus (t [37] = –4.14, FDR-corrected p = 0.032). MBI-C scores for decreased motivation and impulse dyscontrol were not significantly correlated with FC of seeds within the DMN, SN, and FPCN using whole-brain ROIs. MBI-C scores for social inappropriateness and abnormal thoughts and perceptions were not analyzed because these characteristics were only found in a few participants.
Fig. 1
Brain regions showing a significant correlation of seed functional connectivity with the mild behavioral impairment checklist (MBI-C) total score. The connectivity value between the left posterior parietal cortex and right middle frontal gyrus was negatively correlated with the MBI-C total score.
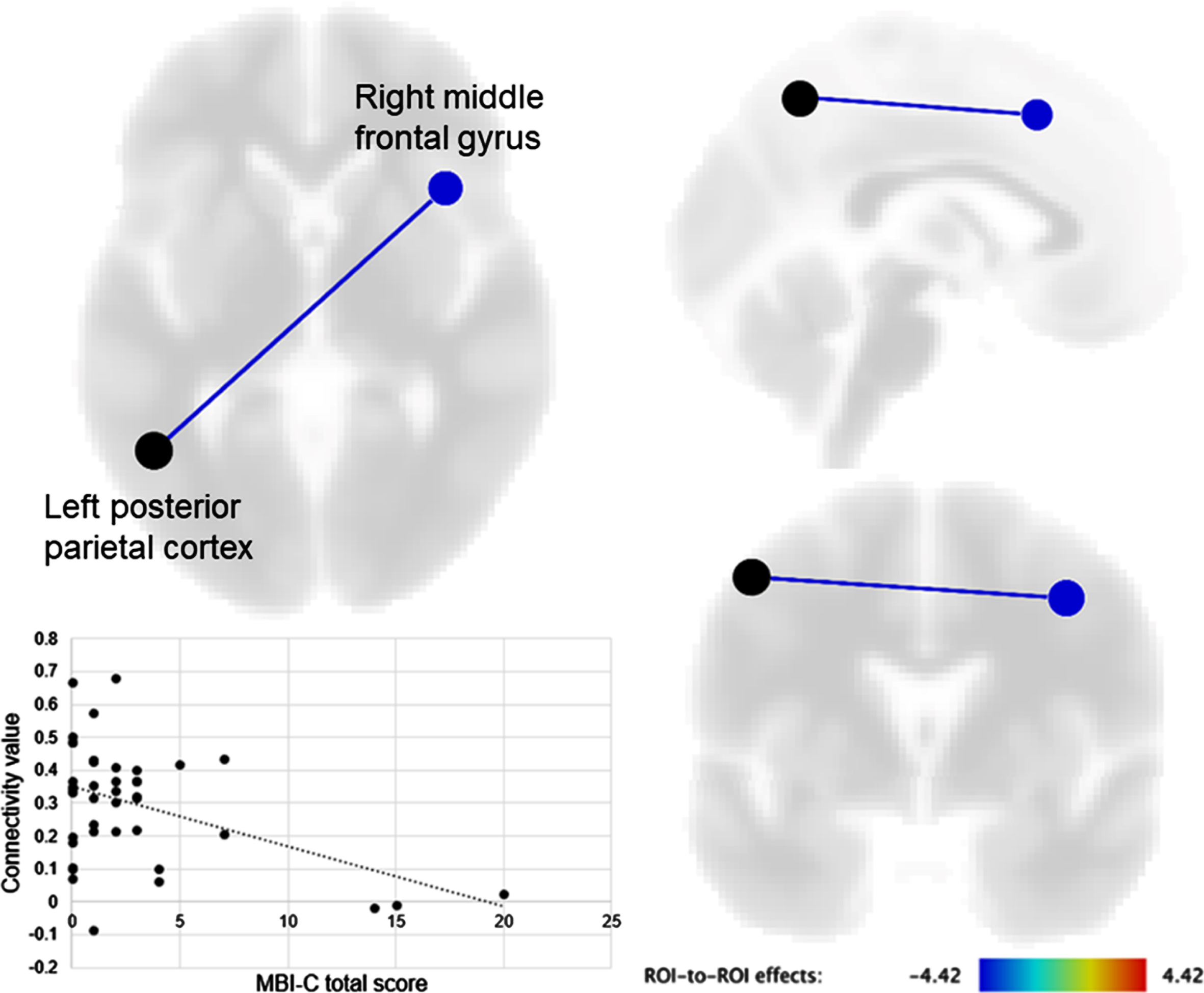
ICA analysis
In the component associated with the FPCN, the MBI-C total score was negatively correlated with the voxel-based FC in the left frontal pole and superior frontal gyrus, and positively correlated with the voxel-based FC in the right precentral gyrus (Fig. 2, Table 2). No brain regions were significantly correlated with the MBI-C total scores in the components associated with the DMN and SN. The MBI-C affective dysregulation score was also negatively correlated with the voxel-based FC in the left frontal pole and superior frontal gyrus (Fig. 3, Table 2); the MBI-C impulse dyscontrol score was positively correlated with the voxel-based FC in the bilateral precentral gyri, and negatively correlated with the left superior frontal gyrus, left frontal pole, and right cerebellum in the component associated with the FPCN (Fig. 3, Table 2). The MBI-C decreased motivation score did not show a significant correlation with components associated with the DMN, SN, and FPCN.
Fig. 2
Brain regions with a significant positive correlation (red) and a significant negative correlation (blue) of voxel-based functional connectivity and the mild behavioral impairment checklist (MBI-C) total score. The left frontal pole and superior frontal gyrus were negatively correlated with the MBI-C total score, and the right precentral gyrus was positively correlated with the MBI-C total score.
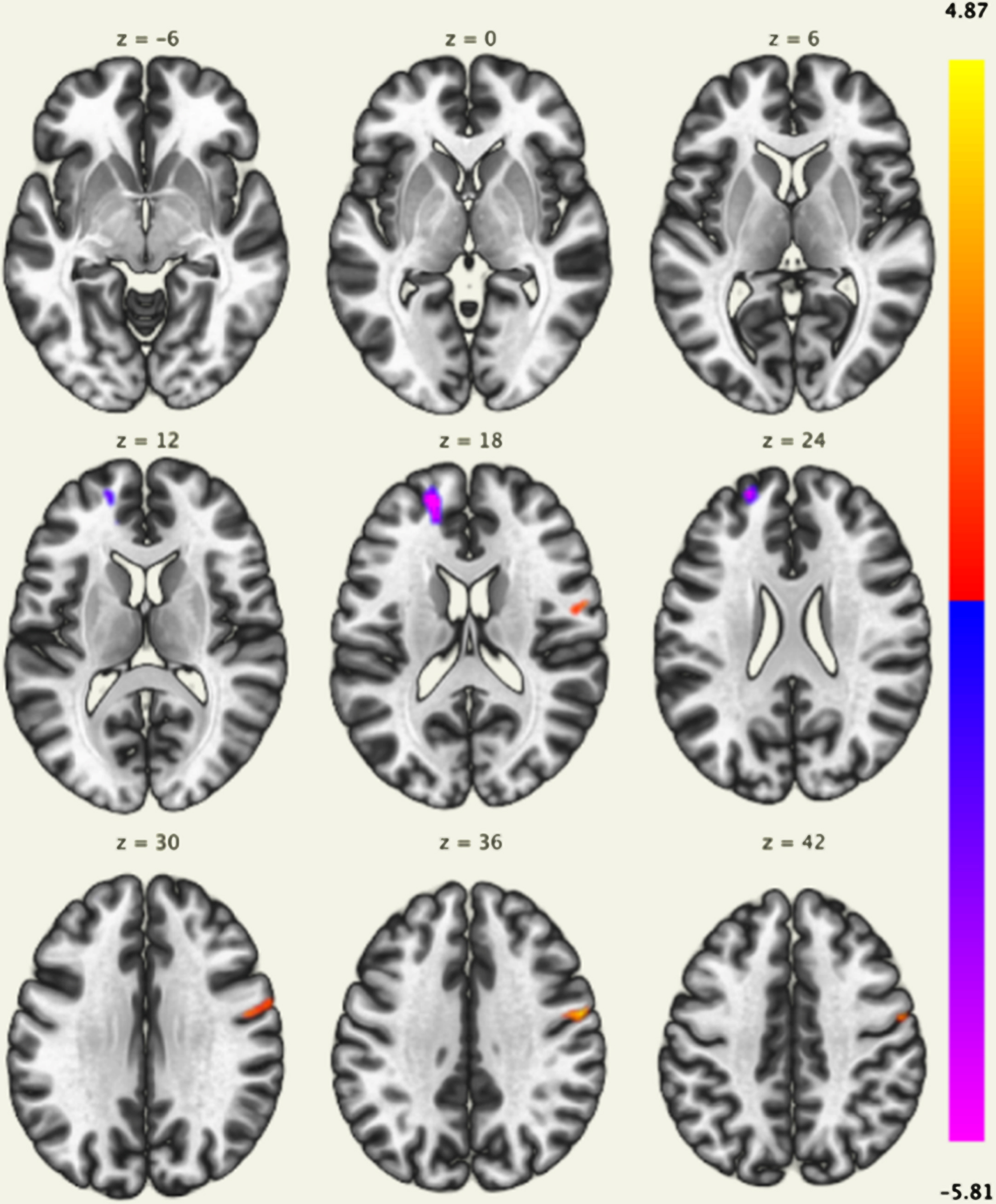
Table 2
Results of independent component analysis
Brain area | MNI coordinates at the peak voxel | Cluster size | Cluster p (FDR-corrected) | ||
X | Y | Z | |||
MBI-C total | |||||
Left frontal pole | –18 | 52 | 18 | 315 | 0.008 |
Right precentral gyrus | 56 | –4 | 36 | 257 | 0.012 |
Left superior frontal gyrus | –18 | 18 | 60 | 191 | 0.034 |
MBI-C affective dysregulation | |||||
Left frontal pole | –22 | 56 | 22 | 414 | 0.001 |
Left superior frontal gyrus | –6 | 34 | 36 | 292 | 0.007 |
Left superior frontal gyrus | –18 | 18 | 58 | 268 | 0.007 |
MBI-C impulse dyscontrol | |||||
Right precentral gyrus | 12 | –16 | 62 | 901 | < 0.001 |
Right precentral gyrus | 64 | 2 | 28 | 663 | < 0.001 |
Left precentral gyrus | –60 | –2 | 24 | 545 | < 0.001 |
Left superior frontal gyrus | 12 | 36 | 46 | 203 | 0.022 |
Left frontal pole | –18 | 52 | 18 | 158 | 0.041 |
Right cerebellum | 44 | –80 | –42 | 158 | 0.041 |
FDR, false discovery rate; MBI-C, mild behavioral impairment checklist; MNI, Montreal Neurological Institute.
Fig. 3
Brain regions with a significant positive correlation (red) and a significant negative correlation (blue) of voxel-based functional connectivity and the mild behavioral impairment checklist (MBI-C) affective dysregulation score (a) and impulse dyscontrol score (b). The left frontal pole and superior frontal gyrus were negatively correlated with the MBI-C affective dysregulation score (a). The bilateral precentral gyri were positively correlated with the MBI-C impulse dyscontrol score, and the left superior frontal gyrus, left frontal pole, and right cerebellum were negatively correlated with the MBI-C impulse dyscontrol score (b).
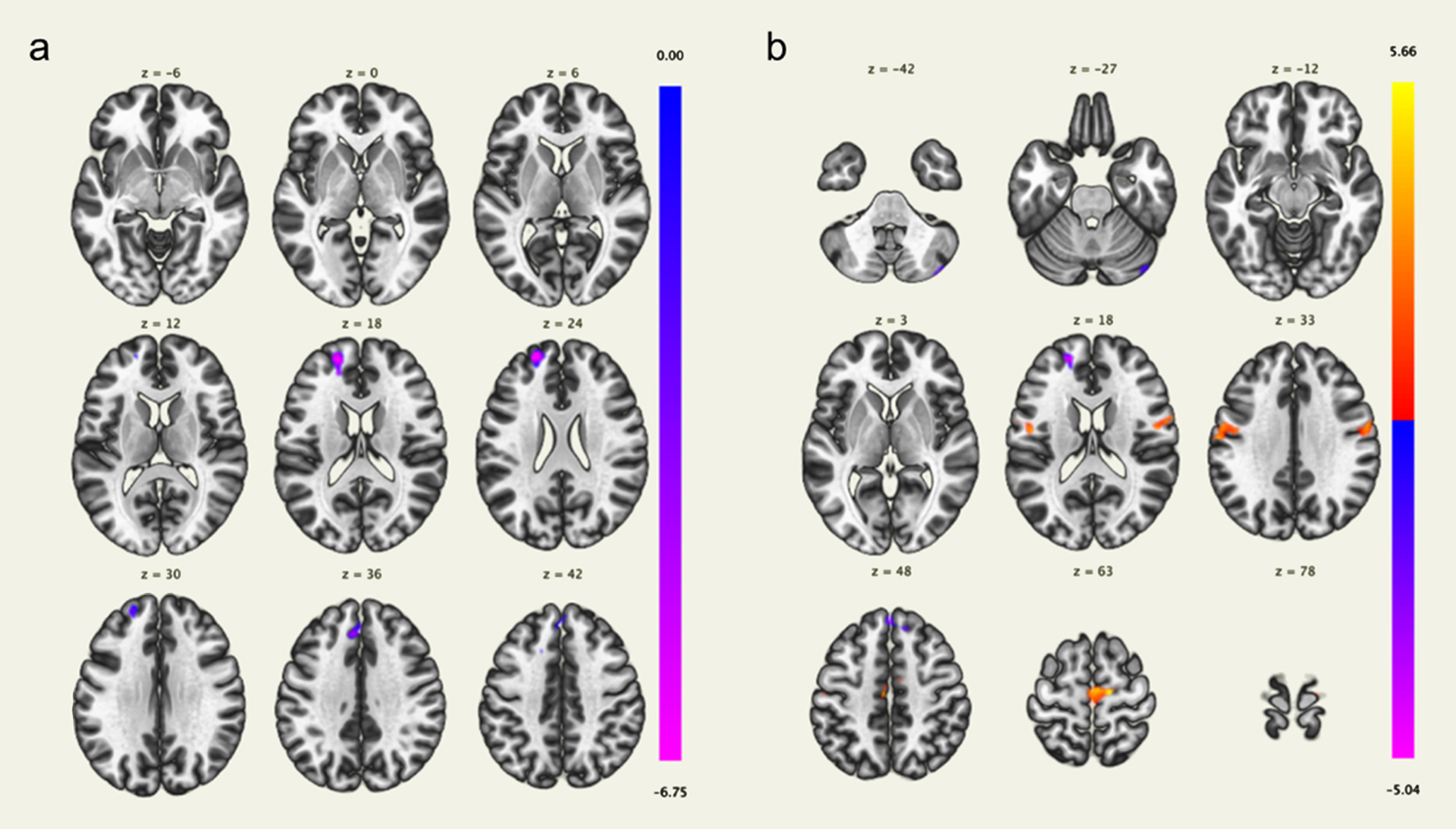
VBM analysis
VBM analysis did not detect any brain regions that were significantly correlated with the MBI-C total, decreased motivation, or affective dysregulation scores. The MBI-C impulse dyscontrol score was negatively correlated with the regional brain volume in the left superior temporal gyrus (cluster size 547; FDR-corrected p = 0.033, MNI coordinates of peak voxels -51/-20/9) (Fig. 4).
Fig. 4
Brain regions correlated with the mild behavioral impairment checklist (MBI-C) impulse dyscontrol score. The gray matter volume of the left superior temporal gyrus was negatively correlated with the MBI-C impulse dyscontrol score.
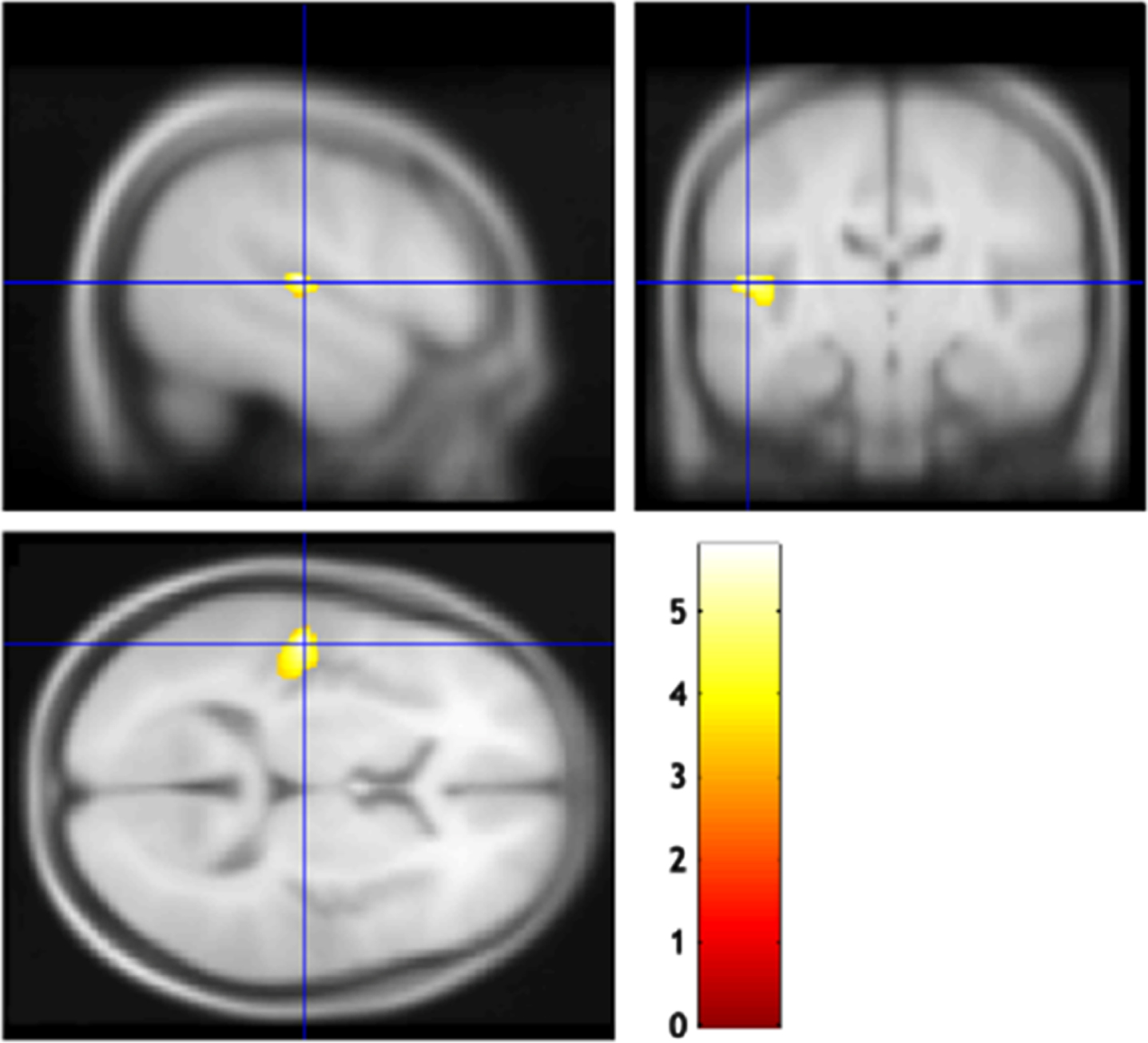
DISCUSSION
The results of the study demonstrated that MBI, especially affective dysregulation, correlated with decreased FC between the left posterior parietal cortex and right middle frontal gyrus. Decreased FCs in the left frontal pole and superior frontal gyrus are also involved in MBI. Therefore, dysfunction of FPCN might be linked to MBI.
Affective and emotional dysregulation are common symptoms in preclinical and prodromal dementia [17, 31], and decreased connectivity in the FPCN has been associated with subthreshold depressive symptoms in the general population [32] and affective symptoms in MCI [12]. A meta-analysis of resting-state FC found associations between major depressive disorder and hypoconnectivity within the FPCN and hyperconnectivity within the DMN [33]. Another meta-analysis showed involvement of the right middle frontal gyrus in the FPCN [34]. These results suggest that the FPCN might be an important network as a neural correlate of MBI, especially for symptoms comprising the affective dysregulation domain.
The FPCN plays an important role in working memory, executive function, and general task-switching processes [4, 35]. Previous studies have examined the association between MBI and impairment of executive function, working memory, and attention. Thus, comparison of cognitive function between MBI without cognitive complaints and MCI showed poorer language and executive function in MBI, but worse memory in MCI [16]. In a study at the National Alzheimer Coordinating Center, participants with MBI had greater impairment of attention, executive function, and episodic memory, compared to participants without MBI [36]; measurement of MBI with the MBI-C in a large sample of cognitively normal community-dwelling elderly people demonstrated a decline in working memory and attention over one year [14]. Therefore, FPCN dysfunction could result in impairment of executive function in MBI; indeed, impairment of set shifting in PD with MBI has been associated with such dysfunction [20]. Thus, the findings of the current study link networks associated with cognitive impairment, in advance of overt cognitive impairment, and support the utility of MBI for identification of the risk group for incident cognitive decline and dementia.
MBI manifests with early symptoms of various types of dementia, including AD, frontotemporal dementia (FTD), PD dementia, dementia with Lewy bodies, and vascular dementia [13]. We previously reported an increased risk of dementia in participants with affective dysregulation symptoms of MBI [17], which was the most common symptom in the current study. Since our sample consisted of cognitively normal participants and participants with aMCI, these symptoms and their association with the FPCN could represent early symptoms of AD. Previous studies have shown relationships between MBI and biomarkers for AD, including brain amyloid deposition [37], a high tau-PET signal in early Braak stages, elevated CSF P-tau181 [38], Alzheimer’s genetic loci [39, 40], and plasma neurofilament light [22]. More recently, in a comparable Alzheimer’s Disease Neuroimaging Initiative sample of persons with normal cognition or MCI, MBI was associated with low plasma Aβ42/Aβ40, a signal driven by the affective dysregulation domain [41].
Functional neuroimaging implicated the frontal cortex [42], precuneus [42, 43], and temporoparietal cortex [44] in predicting the conversion of MCI to AD. Participants with AD had FC reduction not only in the DMN, but also in the CEN and FPCN [45]. Attention deficits in AD have been associated with less activation in the parietal and frontal lobes [46]. Therefore, affective dysregulation and FPCN dysfunction could be predictors of conversion to AD. On the other hand, the impairment of executive function and theory of mind, and focal frontal atrophy have been detected as predictors of conversion from MBI to dementia, especially the behavioral variant of FTD (bvFTD) [47]; the SN is involved in the symptoms of bvFTD [10]. Thus, MBI in PD associated with the DMN, SN, and FPCN [19, 20] could be an early symptom of PD dementia. These results suggest a dependence of neural correlates of MBI on the neuropathological background, and further longitudinal neuroimaging studies are needed to identify the predictors of conversion of MBI to dementia.
The current study demonstrated the involvement of the bilateral precentral gyri, left superior frontal gyrus, left frontal pole, right cerebellum, and left superior temporal gyrus with impulse dyscontrol symptoms of MBI. These symptoms include agitation, aggression, rigidity, stubbornness, impulsivity, and abnormal reward salience [13, 26]. A recent network analysis of impulse dyscontrol in MCI and SCD identified irritability, agitation, and rigidity as the central symptoms in the network [48]. More recently, in a mixed sample of normal cognition, MCI, and AD, impulse dyscontrol was associated with changes in fractional anisotropy and/or radial diffusivity in the fornix, superior fronto-occipital fasciculus, cingulum, and uncinate fasciculus, and grey matter atrophy in the parahippocampal gyrus [49]. In fMRI studies, the SN and DMN have been associated with hyperactivity syndrome, including irritability, agitation, euphoria, aberrant motor behavior, and disinhibition in AD [9, 11], and reduced connectivity in the olfactory cortex and posterior cingulate cortex has been linked to agitation and irritability in cognitively normal participants and those with aMCI and AD [50]. However, an association between impulse dyscontrol symptoms of MBI and SN and DMN was not detected in the current study. We were unable to detect the neural correlates of decreased motivation, social inappropriateness, and abnormal thoughts and perception due to the low severity and prevalence of these symptoms in the participants. In the current study, the neural correlates of the MBI-C total score and MBI-C affective dysregulation score were similar. This could be because the MBI-C affective dysregulation score represented a large portion of MBI-C total score. On the other hand, the neural correlates between affective dysregulation and impulse dyscontrol symptoms of MBI were partially different. Since the neural correlates of MBI may differ in each MBI domain, further neuroimaging studies are warranted to examine these correlates in each domain. However, total MBI-C score identifies the global risk of incident cognitive decline and dementia, of which individual domains may not be endorsed frequently enough to do. Further, there are no published cut off scores for individual domain positivity, and individual items may load onto different domains based on the sample and the psychometric properties of the specific translation. Thus, considering the state of the field at present, the global MBI burden remains the best-validated approach to risk assessment, and the neural correlates of this burden may still represent a distinct risk marker.
The characteristics of the MBI in this study were consistent with those of previous studies. Various prevalence estimates have been reported with differences based on setting (primary care population, community population, psychiatric clinic, and neurology clinic), and on case ascertainment (neuropsychiatric inventory, MBI-C, semi-structured interview/chart review). These prevalences have ranged from 5.8 to 75.5%for SCD, and 14.2 to 85.3%for MCI [17, 30, 51–53] with the most conservative prevalence generated using the MBI-C (which most accurately measures the MBI). The affective dysregulation domain has been the most common [17, 27, 51, 52], and the mean MBI-C total score ranges from 1.94 to 5.08 [30, 37, 53]. Using a cut-off score of 0/1, 45%of the participants self-reported MBI-C symptoms [27]. A prevalence of MBI of 14.2%has been found in primary care participants with MCI, and a cut-off of 6.5 for the MBI-C total score best distinguished patients with and without MBI [30]. Therefore, the Japanese version of the MBI-C could accurately assess the MBI symptoms.
This study has certain limitations. First, the MBI-C was self-reported by the participants, and while this method has been shown to be associated with 1-year cognitive decline in a cognitively normal community sample [14], the self- and informant-rated MBI-C were weakly correlated and could possess different psychometric properties [27]. MBI-C scoring by informants tends to be higher than that by the participants [27]; however, the self-reported MBI-C is also significant since many older adults who are cognitively normal or have MCI do not have informants or have subtle symptoms that could be better recognized by themselves before others notice these symptoms. Second, MBI severity was low since the number of participants was small; 70%of the participants were cognitively normal. Moreover, only a few participants endorsed social inappropriateness and abnormal thoughts and perceptions, which precluded analysis of these domains. It is possible that the loss of insight could have resulted in underestimation of these symptoms by self-report. Previous research has demonstrated that self-rated scores for impulse dyscontrol and social inappropriateness were lower than the informant scores in a sample of cognitively normal people [27]. Third, results of the validation study of the Japanese MBI-C version have not yet been published. However, this translation demonstrates satisfactory concurrent validity and reliability (data not shown). Fourth, the assessment of cognitive function could be insufficient since it was evaluated using only the MMSE and CDR. Fifth, the current study was cross-sectional, and thus, does not provide information on the changes in MBI, cognition, or FC over time. These limitations should be taken into account when interpreting our results, and further investigations are clearly warranted to confirm the preliminary findings in the present study.
In conclusion, FPCN dysfunction was detected as a neural correlate of MBI, especially in the affective dysregulation domain. This dysfunction may be associated with cognitive impairment in MBI and conversion of MBI to dementia, and longitudinal neuroimaging studies in the future are warranted for further examination of MBI.
ACKNOWLEDGMENTS
This study is based on the work supported by the National Institution of Information and Communications Technology (18701) and a JSPS Grant-in-Aid for Scientific Research (C) (21K07505).
Authors’ disclosures available online (https://www.j-alz.com/manuscript-disclosures/21-0628r1).
REFERENCES
[1] | Boublay N , Schott AM , Krolak-Salmon P ((2016) ) Neuroimaging correlates of neuropsychiatric symptoms in Alzheimer’s disease: A review of 20 years of research. Eur J Neurol 23: , 1500–1509. |
[2] | Wolinsky D , Drake K , Bostwick J ((2018) ) Diagnosis and management of neuropsychiatric symptoms in Alzheimer’s disease. Curr Psychiatry Rep 20: , 117. |
[3] | Greicius MD , Krasnow B , Reiss AL , Menon V ((2003) ) Functional connectivity in the resting brain: A network analysis of the default mode hypothesis. Proc Natl Acad Sci U S A 100: , 253–258. |
[4] | Seeley WW , Menon V , Schatzberg AF , Keller J , Glover GH , Kenna H , Reiss AL , Greicius MD ((2007) ) Dissociable intrinsic connectivity networks for salience processing and executive control. J Neurosci 27: , 2349–2356. |
[5] | Vincent JL , Kahn I , Snyder AZ , Raichle ME , Buckner RL ((2008) ) Evidence for a frontoparietal control system revealed by intrinsic functional connectivity. J Neurophysiol 100: , 3328–3342. |
[6] | Sridharan D , Levitin DJ , Menon V ((2008) ) A critical role for the right fronto-insular cortex in switching between central-executive and default-mode networks. Proc Natl Acad Sci U S A 105: , 12569–12574. |
[7] | Joo SH , Lee CU , Lim HK ((2017) ) Apathy and intrinsic functional connectivity networks in amnestic mild cognitive impairment. Neuropsychiatr Dis Treat 13: , 61–67. |
[8] | Wang X , Ren P , Mapstone M , Conwell Y , Porsteinsson AP , Foxe JJ , Raizada RDS , Lin F , the Alzheimer’s Disease Neuroimaging Initiative ((2019) ) Identify a shared neural circuit linking multiple neuropsychiatric symptoms with Alzheimer’s pathology. Brain Imaging Behav 13: , 53–64. |
[9] | Lee JS , Kim JH , Lee SK ((2020) ) The relationship between neuropsychiatric symptoms and default-mode network connectivity in Alzheimer’s disease. Psychiatry Investig 17: , 662–666. |
[10] | Rosenberg PB , Nowrangi MA , Lyketsos CG ((2015) ) Neuropsychiatric symptoms in Alzheimer’s disease: What might be associated brain circuits? Mol Aspects Med 43-44: , 25–37. |
[11] | Balthazar ML , Pereira FR , Lopes TM , da Silva EL , Coan AC , Campos BM , Duncan NW , Stella F , Northoff G , Damasceno BP , Cendes F ((2014) ) Neuropsychiatric symptoms in Alzheimer’s disease are related to functional connectivity alterations in the salience network. Hum Brain Mapp 35: , 1237–1246. |
[12] | Munro CE , Donovan NJ , Guercio BJ , Wigman SE , Schultz AP , Amariglio RE , Rentz DM , Johnson KA , Sperling RA , Marshall GA ((2015) ) Neuropsychiatric symptoms and functional connectivity in mild cognitive impairment. J Alzheimers Dis 46: , 727–735. |
[13] | Ismail Z , Smith EE , Geda Y , Sultzer D , Brodaty H , Smith G , Agüera-Ortiz L , Sweet R , Miller D , Lyketsos CG , ISTAART Neuropsychiatric Symptoms Professional Interest Area ((2016) ) Neuropsychiatric symptoms as early manifestations of emergent dementia: Provisional diagnostic criteria for mild behavioral impairment. Alzheimers Dement 12: , 195–202. |
[14] | Creese B , Brooker H , Ismail Z , Wesnes KA , Hampshire A , Khan Z , Megalogeni M , Corbett A , Aarsland D , Ballard C ((2019) ) Mild behavioral impairment as a marker of cognitive decline in cognitively normal older adults. Am J Geriatr Psychiatry 27: , 823–834. |
[15] | Ismail Z , McGirr A , Gill S , Hu S , Forkert ND , Smith EE ((2021) ) Mild behavioral impairment and subjective cognitive decline predict cognitive and functional decline. J Alzheimers Dis 80: , 459–469. |
[16] | Taragano FE , Allegri RF , Heisecke SL , Martelli MI , Feldman ML , Sánchez V , García VA , Tufro G , Castro DM , Leguizamón PP , Guelar V , Ruotolo E , Zegarra C , Dillon C ((2018) ) Risk of conversion to dementia in a mild behavioral impairment group compared to a psychiatric group and to a mild cognitive impairment group. J Alzheimers Dis 62: , 227–238. |
[17] | Matsuoka T , Ismail Z , Narumoto J ((2019) ) Prevalence of mild behavioral impairment and risk of dementia in a psychiatric outpatient clinic. J Alzheimers Dis 70: , 505–513. |
[18] | Yoon EJ , Ismail Z , Hanganu A , Kibreab M , Hammer T , Cheetham J , Kathol I , Sarna JR , Martino D , Furtado S , Monchi O ((2019) ) Mild behavioral impairment is linked to worse cognition and brain atrophy in Parkinson disease. Neurology 93: , e766–e777. |
[19] | Lang S , Yoon EJ , Kibreab M , Kathol I , Cheetham J , Hammer T , Sarna J , Ismail Z , Monchi O ((2020) ) Mild behavioral impairment in Parkinson’s disease is associated with altered corticostriatal connectivity. Neuroimage Clin 26: , 102252. |
[20] | Yoon EJ , Ismail Z , Kathol I , Kibreab M , Hammer T , Lang S , Ramezani M , Auclair-Ouellet N , Sarna JR , Martino D , Furtado S , Monchi O ((2021) ) Patterns of brain activity during a set-shifting task linked to mild behavioral impairment in Parkinson’s disease. Neuroimage Clin 30: , 102590. |
[21] | Matuskova V , Ismail Z , Nikolai T , Markova H , Cechova K , Nedelska Z , Laczo J , Wang M , Hort J , Vyhnalek M ((2021) ) Mild behavioral impairment is associated with atrophy of entorhinal cortex and hippocampus in a memory clinic cohort. Front Aging Neurosci 13: , 236. |
[22] | Naude JP , Gill S , Hu S , McGirr A , Forkert ND , Monchi O , Stys PK , Smith EE , Ismail Z , Alzheimer’s Disease Neuroimaging Initiative ((2020) ) Plasma neurofilament light: A marker of neurodegeneration in mild behavioral impairment. J Alzheimers Dis 76: , 1017–1027. |
[23] | Hughes CP , Berg L , Danziger WL , Coben LA , Martin RL ((1982) ) A new clinical scale for the staging of dementia. Br J Psychiatry 140: , 566–572. |
[24] | Petersen RC ((2004) ) Mild cognitive impairment as a diagnostic entity. J Intern Med 256: , 183–194. |
[25] | Ueno D , Matsuoka T , Kato Y , Ayani N , Maeda S , Takeda M , Narumoto J ((2020) ) Individual differences in interoceptive accuracy are correlated with salience network connectivity in older adults. Front Aging Neurosci 12: , 592002. |
[26] | Ismail Z , Agüera-Ortiz L , Brodaty H , Cieslak A , Cummings J , Fischer CE , Gauthier S , Geda YE , Herrmann N , Kanji J , Lanctôt KL , Miller DS , Mortby ME , Onyike CU , Rosenberg PB , Smith EE , Smith GS , Sultzer DL , Lyketsos C , NPS Professional Interest Area of the International Society of to Advance Alzheimer’s Research and Treatment (NPS-PIA of ISTAART) ((2017) ) The Mild Behavioral Impairment Checklist (MBI-C): A rating scale for neuropsychiatric symptoms in pre-dementia populations. J Alzheimers Dis 56: , 929–938. |
[27] | Creese B , Griffiths A , Brooker H , Corbett A , Aarsland D , Ballard C , Ismail Z ((2020) ) Profile of mild behavioral impairment and factor structure of the Mild Behavioral Impairment Checklist in cognitively normal older adults. Int Psychogeriatr 32: , 705–717. |
[28] | Folstein MF , Folstein SE , McHugh PR ((1975) ) “Mini-mental state”. A practical method for grading the cognitive state of patients for the clinician. J Psychiatr Res 12: , 189–198. |
[29] | Whitfield-Gabrieli S , Nieto-Castanon A ((2012) ) Conn: A functional connectivity toolbox for correlated and anticorrelated brain networks. Brain Connect 2: , 125–141. |
[30] | Mallo SC , Ismail Z , Pereiro AX , Facal D , Lojo-Seoane C , Campos-Magdaleno M , Juncos-Rabadán O ((2018) ) Assessing mild behavioral impairment with the mild behavioral impairment-checklist in people with mild cognitive impairment. J Alzheimers Dis 66: , 83–95. |
[31] | Ismail Z , Gatchel J , Bateman DR , Barcelos-Ferreira R , Cantillon M , Jaeger J , Donovan NJ , Mortby ME ((2018) ) Affective and emotional dysregulation as pre-dementia risk markers: Exploring the mild behavioral impairment symptoms of depression, anxiety, irritability, and euphoria. Int Psychogeriatr 30: , 185–196. |
[32] | Schultz DH , Ito T , Solomyak LI , Chen RH , Mill RD , Anticevic A , Cole MW ((2019) ) Global connectivity of the fronto-parietal cognitive control network is related to depression symptoms in the general population. Netw Neurosci 3: , 107–123. |
[33] | Kaiser RH , Andrews-Hanna JR , Wager TD , Pizzagalli DA ((2015) ) Large-scale network dysfunction in major depressive disorder: A meta-analysis of resting-state functional connectivity. JAMA Psychiatry 72: , 603–611. |
[34] | Fischer M , Moscovitch M , Alain C ((2021) ) A systematic review and meta-analysis of memory-guided attention: Frontal and parietal activation suggests involvement of fronto-parietal networks.e. Wiley Interdiscip Rev Cogn Sci 12: , 1546. |
[35] | Kim C , Cilles SE , Johnson NF , Gold BT ((2012) ) Domain general and domain preferential brain regions associated with different types of task switching: A meta-analysis. Hum Brain Mapp 33: , 130–142. |
[36] | Rouse HJ , Small BJ , Schinka JA , Loewenstein DA , Duara R , Potter H ((2020) ) Mild behavioral impairment as a predictor of cognitive functioning in older adults. Int Psychogeriatr 33: , 285–293. |
[37] | Lussier FZ , Pascoal TA , Chamoun M , Therriault J , Tissot C , Savard M , Kang MS , Mathotaarachchi S , Benedet AL , Parsons M , Qureshi MNI , Thomas ÉM , Shin M , Dion LA , Massarweh G , Soucy JP , Tsai IH , Vitali P , Ismail Z , Rosa-Neto P , Gauthier S ((2020) ) Mild behavioral impairment is associated with beta-amyloid but not tau or neurodegeneration in cognitively intact elderly individuals. Alzheimers Dement 16: , 192–199. |
[38] | Johansson M , Stomrud E , Insel PS , Leuzy A , Johansson PM , Smith R , Ismail Z , Janelidze S , Palmqvist S , van Westen D , Mattsson-Carlgren N , Hansson O ((2021) ) Mild behavioral impairment and its relation to tau pathology in preclinical Alzheimer’s disease. Transl Psychiatry 11: , 76. |
[39] | Andrews SJ , Ismail Z , Anstey KJ , Mortby M ((2018) ) Association of Alzheimer’s genetic loci with mild behavioral impairment. Am J Med Genet B Neuropsychiatr Genet 177: , 727–735. |
[40] | Creese B , Arathimos R , Brooker H , Aarsland D , Corbett A , Lewis C , Ballard C , Ismail Z ((2021) ) Genetic risk for Alzheimer’s disease, cognition, and mild behavioral impairment in healthy older adults. Alzheimers Dement (Amst) 13: , e12164. |
[41] | Miao R , Chen H-Y , Gill S , Naude J , Smith EE , Ismail Z , Alzheimer’s Disease Neuroimaging Initiative Plasma β-amyloid in mild behavioural impairment –neuropsychiatric symptoms on the Alzheimer’s continuum. J Geriatr Psychiatry Neurol, doi: 10.1177/08919887211016068 |
[42] | Hojjati SH , Ebrahimzadeh A , Khazaee A , Babajani-Feremi A , Alzheimer’s Disease Neuroimaging Initiative ((2018) ) Predicting conversion from MCI to AD by integrating rs-fMRI and structural MRI. Comput Biol Med 102: , 30–39. |
[43] | Ottoy J , Niemantsverdriet E , Verhaeghe J , De Roeck E , Struyfs H , Somers C , Wyffels L , Ceyssens S , Van Mossevelde S , Van den Bossche T , Van Broeckhoven C , Ribbens A , Bjerke M , Stroobants S , Engelborghs S , Staelens S ((2019) ) Association of short-term cognitive decline and MCI-to-AD dementia conversion with CSF, MRI, amyloid- and (18)F-FDG-PET imaging. Neuroimage Clin 22: , 101771. |
[44] | Zhou H , Jiang J , Lu J , Wang M , Zhang H , Zuo C , Alzheimer’s Disease Neuroimaging Initiative ((2018) ) Dual-Model radiomic biomarkers predict development of mild cognitive impairment progression to Alzheimer’s disease. Front Neurosci 12: , 1045. |
[45] | Zhao Q , Sang X , Metmer H , Swati ZNNK , Lu J , Alzheimer’s Disease Neuroimaging Initiative ((2019) ) Functional segregation of executive control network and frontoparietal network in Alzheimer’s disease. Cortex 120: , 36–48. |
[46] | Hao J , Li K , Li K , Zhang D , Wang W , Yang Y , Yan B , Shan B , Zhou X ((2005) ) Visual attention deficits in Alzheimer’s disease: An fMRI study. Neurosci Lett 385: , 18–23. |
[47] | Orso B , Mattei C , Arnaldi D , Massa F , Serafini G , Plantone D , Doglione E , Grafman J , Nobili F , Pardini M ((2020) ) Clinical and MRI predictors of conversion from mild behavioural impairment to dementia. Am J Geriatr Psychiatry 28: , 755–763. |
[48] | Saari T , Smith EE , Ismail Z (2021) Network analysis of impulse dyscontrol in mild cognitive impairment and subjective cognitive decline. Int Psychogeriatr, doi: 10.1017/S1041610220004123 |
[49] | Gill S , Wang M , Mouches P , Rajashekar D , Sajobi T , MacMaster FP , Smith EE , Forkert ND , Ismail Z , Alzheimer’s Disease Neuroimaging Initiative Neural correlates of the impulse dyscontrol domain of mild behavioral impairment. Int J Geriatr Psychiatry 36: , 1398–1406. |
[50] | Serra L , Bruschini M , Di Domenico C , Mancini M , Bechi Gabrielli G , Bonarota S , Caltagirone C , Cercignani M , Marra C , Bozzali M ((2020) ) Behavioral psychological symptoms of dementia and functional connectivity changes: A network-based study. Neurobiol Aging 94: , 196–206. |
[51] | Mortby ME , Ismail Z , Anstey KJ ((2018) ) Prevalence estimates of mild behavioral impairment in a population-based sample of pre-dementia states and cognitively healthy older adults. Int Psychogeriatr 30: , 221–232. |
[52] | Sheikh F , Ismail Z , Mortby ME , Barber P , Cieslak A , Fischer K , Granger R , Hogan DB , Mackie A , Maxwell CJ , Menon B , Mueller P , Patry D , Pearson D , Quickfall J , Sajobi T , Tse E , Wang M , Smith EE , PROMPT registry investigators ((2018) ) Prevalence of mild behavioral impairment in mild cognitive impairment and subjective cognitive decline, and its association with caregiver burden. Int Psychogeriatr 30: , 233–244. |
[53] | Mallo SC , Ismail Z , Pereiro AX , Facal D , Lojo-Seoane C , Campos-Magdaleno M , Juncos-Rabadán O ((2019) ) Assessing mild behavioral impairment with the mild behavioral impairment checklist in people with subjective cognitive decline. Int Psychogeriatr 31: , 231–239. |