A Systems Biology Approach for Hypothesizing the Effect of Genetic Variants on Neuroimaging Features in Alzheimer’s Disease
Abstract
Background:
Neuroimaging markers provide quantitative insight into brain structure and function in neurodegenerative diseases, such as Alzheimer’s disease, where we lack mechanistic insights to explain pathophysiology. These mechanisms are often mediated by genes and genetic variations and are often studied through the lens of genome-wide association studies. Linking these two disparate layers (i.e., imaging and genetic variation) through causal relationships between biological entities involved in the disease’s etiology would pave the way to large-scale mechanistic reasoning and interpretation.
Objective:
We explore how genetic variants may lead to functional alterations of intermediate molecular traits, which can further impact neuroimaging hallmarks over a series of biological processes across multiple scales.
Methods:
We present an approach in which knowledge pertaining to single nucleotide polymorphisms and imaging readouts is extracted from the literature, encoded in Biological Expression Language, and used in a novel workflow to assist in the functional interpretation of SNPs in a clinical context.
Results:
We demonstrate our approach in a case scenario which proposes KANSL1 as a candidate gene that accounts for the clinically reported correlation between the incidence of the genetic variants and hippocampal atrophy. We find that the workflow prioritizes multiple mechanisms reported in the literature through which KANSL1 may have an impact on hippocampal atrophy such as through the dysregulation of cell proliferation, synaptic plasticity, and metabolic processes.
Conclusion:
We have presented an approach that enables pinpointing relevant genetic variants as well as investigating their functional role in biological processes spanning across several, diverse biological scales.
INTRODUCTION
As aging populations continue to grow, age-ass-ociated disorders such as Alzheimer’s disease (AD) have become increasingly prevalent [1, 2]. AD is a slow-progressing, complex, idiopathic disorder in which early diagnosis is challenging because patients do not initially present symptoms [3]. Emerging neuroimaging techniques are a versatile, non-invasive approach for the high-resolution, in vivo investigation of the underlying pathophysiology of AD that may provide an opportunity for earlier detection and therapeutic intervention.
Neuroimaging techniques quantitatively measure markers of brain structure and function that are considered as endophenotypes, measurable intermediate phenotypes that link molecular changes to organ-spe-cific pathophysiological contexts [4]. One of the numerous neuroanatomical markers considered as an endophenotype is medial temporal atrophy. This well-established AD marker is an intermediate phenotype that implicates the aggregation of hyperphosphorylated tau protein (a well-known molecular change) as a causative biological process of memory decline [5]. The diversity of markers prompted the cataloging and organizing of their information in order to better link clinical readouts to underlying molecular changes. As a first attempt in addressing this need, Iyappan et al. curated the terms used in the literature to describe structural and functional brain information in the Neuroimaging Feature Terminology (NIFT) [6].
Elucidating the effect of genes and genetic variations (e.g., single nucleotide polymorphisms (SNPs)) on brain structure and function often begins with genome-wide association studies (GWASs). However, this type of study only calculates statistical associa-tions between SNPs and traits and ignores mechanistic insights. More robust approaches aimed at addressing the mechanistic shortcomings of GWAS are referred to as imaging genetics [7]. For example, Wachinger et al. [8] studied genetic influences on neuroanatomical shape asymmetries associated with AD progression. Although their findings on the association of genetic variants (i.e., BIN1, CD2AP, ZCWPW1, and ABCA7 genes) to neuroanatomical structures had been reported in previous studies [9–12], here the authors were able to provide an exp-lanation for the observed effect, specifically that alterations in the expression level of the aforementioned genes can affect cellular homeostasis, thus leading to changes in brain symmetry. A common issue facing many imaging genetics approaches is small sample size, which leads to a lack of statistical power, limited replicability, and stratification effects [13, 14]. Alternatively, Stefanovski et al. [15] studied the connection between molecular changes and neuronal population dynamics using differential equations. For example, this study provided a possible mechanistic explanation of how local amyloid beta-mediated synaptic function disinhibition leads to diminishing neural signaling. However, such mathematical models thus far fail to handle the number of variables that are necessary to represent the pathophysiological phenomenon involved in a multifactorial disorder such as AD.
The limitations and lack of mechanistic insights provided by these previously mentioned techniques prompted us to develop a new approach to interpret how a particular genetic variant may have an impact on neuroimaging feature changes through sequences of molecular causalities in the context of AD. Our approach captures knowledge from the literature pertaining to SNPs and imaging readouts in a causal model encoded in Biological Expression Language (BEL) [16] to support the functional interpretation of SNPs in a clinical context. In a case scenario, we propose KANSL1 as a candidate gene mediating the connection between the genetic variants and hippocampal atrophy. We then hypothesized that variants of this gene dysregulate biological processes related to cell proliferation, synaptic plasticity, and energy metabolism that ultimately leads to hippocampal atrophy. These dysregulated biological processes are early events in AD, and they have been posited as attractive therapeutic targets for pharmaceutical intervention [17, 18]. Thus, by garnering these mechanistic insights, it may be possible to reveal novel therapeutic options in the future.
MATERIALS AND METHODS
In order to support the interpretation of the fun-ctional impact of SNPs on the alteration of neuroimaging features, associations between SNPs and imaging readouts were extracted using natural language processing. Linkage disequilibrium (LD) analysis was used to identify co-occurring SNPs and their corresponding or associated genes. These genes were then ranked by how often they appear in the literature in the context of AD. This workflow is described in Fig. 1A.
Fig. 1
The two workflows developed for (A) gene prioritization and for (B) generating the mechanistic knowledge assembly around the effect of genetic variants on neuroimaging features in AD. In workflow A, the first step involves the selection of a corpus of relevant scientific literature. Next, the SNPs extracted from this corpus were subjected to LD block analysis and the subsequently obtained SNPs were mapped to their corresponding or associated genes. KANSL1, a novel AD gene, was selected from this pool of mapped genes for further investigation. In workflow B, corpus for the selected gene is extracted and translated into BEL to generate a knowledge assembly model for hypothesis generation.
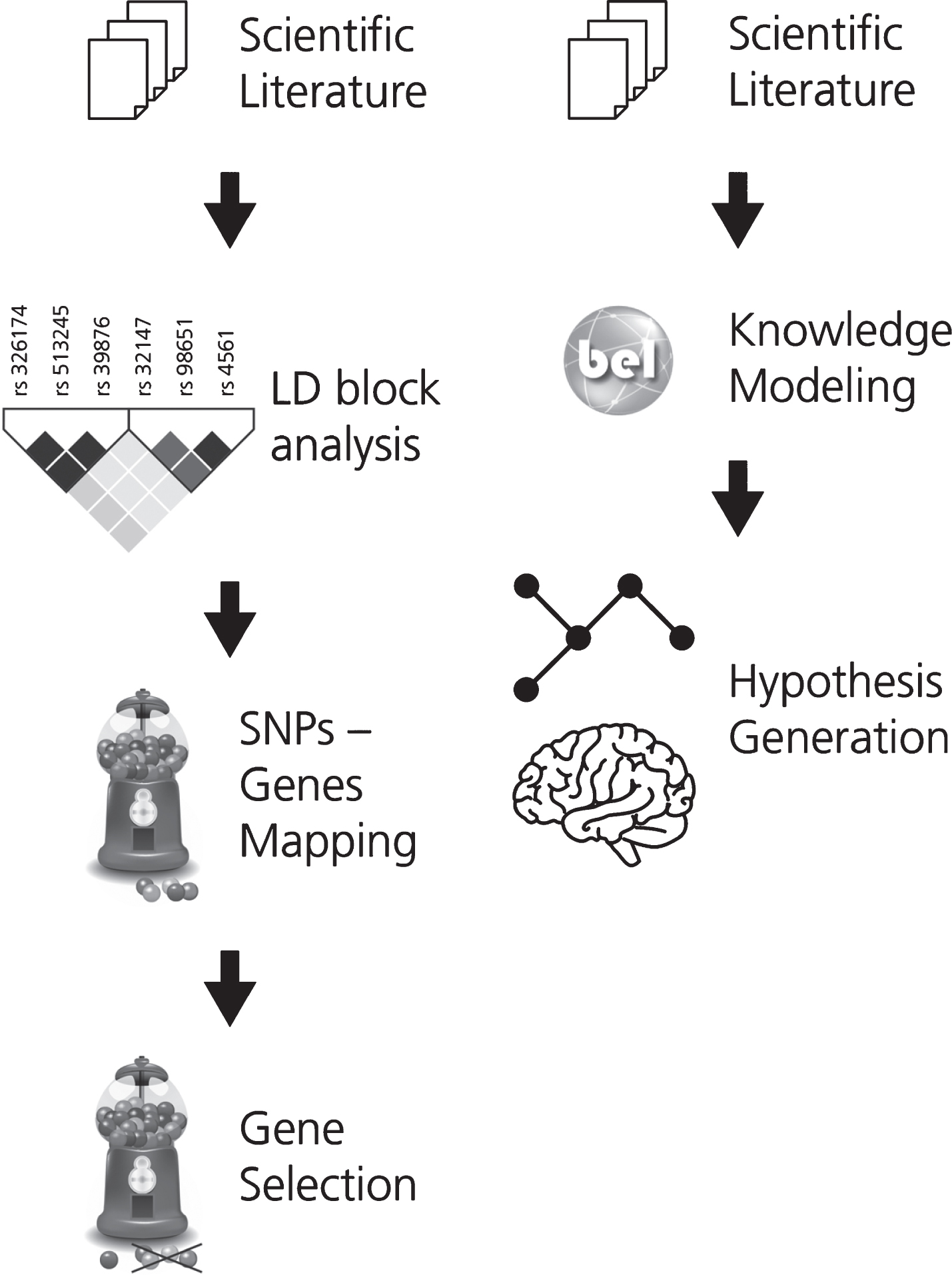
Based on this analysis, one gene (KANSL1) was selected for further investigation and a corpus explaining its role in AD was enriched with knowledge pertaining to multi-scale biological processes. To enable computer-aided reasoning, manually-extracted relations from this corpus were encoded in BEL. The resulting KANSL1 knowledge assembly was validated using PyBEL [19] and integrated into NeuroMMSig [20]. Finally, NeuroMMSig was then used to investigate the putative role of KANSL1 in neuroimaging feature alteration, namely hippoc-ampal atrophy. For the sake of reproducibility, we have made the workflow publicly available through GitHub (https://github.com/sepehrgolriz/GeVa_NeIF) under the MIT License. This workflow is described in Fig. 1B. Additionally, to investigate the concordance of knowledge around the KANSL1 gene, pathways from three well-known pathway databases were queried to determine those in which the gene is implicated.
Generation of a SNP-neuroimaging corpus
A corpus enriched with neuroimaging features and SNPs in the context of AD was generated using SCAIView v0.3.3 (https://academia.scaiview.com) on MEDLINE using the following query: “(([MeSH Disease: “Alzheimer Disease ”]) AND [Neuroimaging Feature]) AND [SNP]))”. The corpus comprised 568 documents with a total of 2215 SNP-neuroima-ging feature associations (Supplementary Table 1), including 126 unique neuroimaging features (Supplementary Table 2) and 745 unique SNPs (Supplementary Table 3).
Identification of related SNPs via linkage disequilibrium blocks
Over time, dependencies between genetic variants are developed across populations [21]. This phenomenon, described as LD, implies that correlations between genetic variants and traits are caused by the aggregated effect of multiple variants [22, 23]. However, SNP-trait associations identified in the literature are obtained by analyzing thousands of SNPs individually (the “single-marker” approach). Therefore, we performed LD block analysis using HaploReg v4.1 [24] to identify a total of 6,070 SNPs that occur with the SNPs extracted from the literature and further mapped them to their corresponding or associated genes (Supplementary Table 4).
Gene selection
DisGeNET [25] was used to identify diseases associated with the genes obtained from HaploReg v4.1. After filtering out genes not associated with AD, the remaining genes were categorized as either well-known risk variants (supported by a minimum of 5 literature evidence which are enriched with observational studies, such as case-control studies) or as emerging genetic biomarkers (those supported by few or no published evidence) (Supplementary Table 5). Since the involvement of well-known risk variants has been sufficiently described in the literature, this study investigated novel genes which may contribute to AD development.
The study of genetics in the context of multiple phenotypes, such as physiological traits or diseases, can provide a holistic overview of gene functions in a biological system. For this reason, DisGeNET was used to investigate gene-disease associations of genes that are not clearly linked to AD [26]. Although the genes are associated with a broad range of diseases, from autoimmune disorders to different types of cancer, we focused on enriching the mechanistic context surrounding genes linked to conditions, such as Parkinson’s disease (PD), which have substantial genetic, pathological, and clinical overlap with AD [27]. While it is believed that cancer and autoimmune diseases are less prevalent in AD patients, 25 to 33 percent of AD patients show concomitant PD pathology [28, 29]. Of the 25 PD-associated genes acquired, we selected KANSL1 for further study of its putative pathogenic role in AD as it had the highest number of literature evidence and its functionality can thus be better understood [30, 31] (Supplementary Table 6).
Corpus generation, relation extraction, and mechanism enrichment for KANSL1
Using the same strategy and resources as the pre-vious corpus, a new corpus describing the role of KANSL1 in the context of AD was generated using the following SCAIView query: “(([Human Genes/Proteins:“KANSL1”]) AND [MeSH Disease: “Alz-heimer Disease”]) AND [Neuroimaging Features]”. The resulting gene-neuroimaging interaction information was then enriched with further causal relations from the literature using manual relation extraction in order to bridge the knowledge gap between genetics and clinical endpoints.
Knowledge modeling
Manually generating mechanistic hypotheses by linking genetic variants to neuroimaging markers is a daunting task. Therefore, in order to empower com-puter-aided reasoning, the extracted knowledge ass-embly was encoded in BEL. Both the syntax and semantics of BEL encoded in the knowledge assembly were validated using the PyBEL framework.
Knowledge was extracted from the selected cor-pus using the official BEL curation guidelines from https://biological-expression-language.github.io as well as additional guidelines from https://github.com/pharmacome/curation.
Evidence from the selected corpus was manually translated into BEL statements together with their contextual information (e.g., brain regions, brain cell types). For instance, the evidence “BDNF infusion led to rapid phosphorylation of the mitogen-activated protein (MAP) in the adult hippocampus” corresponds to the following BEL statement:
SET MeSHAnatomy=“Hippocampus”
p(HGNC:BDNF) - - p(HGNCGENEFAMILY: “Mitogen-activated protein kinases”, pmod(Ph))
The resulting knowledge assembly was then int-egrated into NeuroMMSig, a web server for mechanism enrichment that allows querying over genes, SNPs, and neuroimaging features in the context of a specific disease. Finally, NeuroMMSig was used to identify the mechanistic model representing the putative role of KANSL1 in hippocampal atrophy.
Comparison of the mechanistic model to pathway knowledge
Several manually curated and highly-cited pathway databases are available to deduce biologically relevant pathways. We used three major ones, namely KEGG [32], Reactome, [33] and WikiPathways [34], in order to determine whether knowledge on the KANSL1 gene has yet to be integrated into these resources. Hence, we queried KANSL1 as well as all other proteins from our mechanistic model in pathways from the three databases.
RESULTS
While KANSL1 has been associated with changes in gene expression levels in the hippocampus [35], its mechanism of action remains elusive. In order to better understand KANSL1’s involvement in hippocampal dysfunction, we queried NeuroMMSig to investigate the downstream effects of this gene. Then, reasoning over the knowledge assembly led us to the interpretation described below. Finally, we report the results of querying KANSL1 and other genes from our mechanistic model in pathways from three major pathway databases to determine which pathways the gene may be implicated in.
The putative role of KANSL1 in hippocampal atrophy
The transcription and expression of the genes promoting cell proliferation (e.g., BTG2) and synaptic plasticity (e.g., BDNF) as well as metabolic proces-ses (e.g., cell energy production) are both of para-mount importance for hippocampal function [36] (Fig. 3). KANSL1 is a protein-coding gene involved in chromatin modification through histone acetylation [37, 38], one of the mechanisms orchestrating gene transcription and expression [39–44]. While histone acetylation transforms the condensed structure of the chromatin into a relaxed architecture enhancing RNA transcription and gene expression, its hypoacetylation causes it to behave adversely [45–47].
Fig. 2
This figure shows the results obtained from the LD block analysis and gene mapping. The generation of the SNP-Neuroimaging corpus yielded 745 SNPs. Following LD block analysis, 6,070 SNPs that occur with the SNPs extracted from the literature were identified and located on 136 unique AD associated genes. These genes were then classified according to the number of evidences which are available in the scientific literature. The first group, incorporating 78 AD associated genes, comprises well-known genes characterized by a high number of publications in the AD context. The second group, that includes 58 AD associated genes, comprises emerging genes in the context of AD. From the latter group, KANSL1 was selected.

Fig. 3
The putative role of KANSL1 in hippocampal atrophy. A) KANSL1 role in hippocampal neurogenesis. B) KANSL1 function in hippocampal metabolic processes. C) KANSL1 role in hippocampal synaptic plasticity. [https://nbviewer.jupyter.org/github/sepehrgolriz/GeVa_NeIF/blob/master/Semi_automatic_developed_pipeline/Exploring% 20KANSL1% 20putative% 20role% 20graph% 20in% 20hippocampal% 20atrophy.ipynb].
![The putative role of KANSL1 in hippocampal atrophy. A) KANSL1 role in hippocampal neurogenesis. B) KANSL1 function in hippocampal metabolic processes. C) KANSL1 role in hippocampal synaptic plasticity. [https://nbviewer.jupyter.org/github/sepehrgolriz/GeVa_NeIF/blob/master/Semi_automatic_developed_pipeline/Exploring% 20KANSL1% 20putative% 20role% 20graph% 20in% 20hippocampal% 20atrophy.ipynb].](https://ip.ios.semcs.net:443/media/jad/2021/80-2/jad-80-2-jad201397/jad-80-jad201397-g003.jpg)
KANSL1 and hippocampal neurogenesis
KANSL1 is required for the acetylation of p53 [41], a transcription factor modulating BTG2 expression and a vital protein for hippocampal neurogenesis (i.e., while KANSL1-dependent p53 acetylation induces BTG2 expression, p53 hyperacetylation leads to the overexpression of BTG2) [42, 48, 49]. BTG2 negatively controls the cell cycle since its overexpression results in cell growth rate decline [42, 50]. Through BTG2 binding to Ras (the signaling event mediator), the Ras/MAPK signaling cascade is activated, leading to tau hyperphosphorylation [48]. Tau is a microtubule-associated protein that promotes the assembly and stabilization of cytoskeleton microtubules, both of which are required for cell di-vision (i.e., mitosis). However, tau hyperphosphoryl-ation reduces its capability to bind the microtubules, giving rise to dynamic instability, mitosis impairment, cell cycle deterioration, elimination of proli-ferating newborn neurons, and ultimately to apoptotic processes [51]. In summary, KANSL1 dysfunction disturbs the expression of cell cycle regulatory genes, leading to the perturbation of cell proliferation processes [46, 50] (Fig. 3A).
KANSL1 and hippocampal metabolic processes
The functional crosstalk between KANSL1 and the metabolic processes occurring in the mitochondria (e.g., oxidative phosphorylation) is key for the regulation of hippocampal synaptic plasticity [52–55]. KANSL1 is highly expressed in the mitochondria, where it regulates mitochondrial DNA (mtDNA) transcription and the subsequent translation of genes involved in Oxidative phosphorylation—a set of complex mechanistic processes that form adenosine triphosphate (cell energy currency) by oxidizing nutrients [36, 55, 56]. Oxidative phosphorylation produces potentially harmful reactive oxygen species whose production and detoxification are balanced in normal mitochondria [57]. However, KANSL1 deficiency promotes the downregulation of mtDNA transcription and translation of genes involved in Oxidative phosphorylation, causing reactive oxygen species accumulation. Oxidative stress then occurs, leading to cholesterol metabolism perturbation [58]. Cholesterol homeostasis dysregulation increases cholesterol concentration in cells, leading to synaptic plasticity impairment and ultimately hippocampal shrinkage [59–63] (Fig. 3C).
KANSL1 and hippocampal synaptic plasticity
Long-term potentiation (LTP) is one of the major cellular processes involved in memory formation [64]. BDNF, a member of the neurotrophin family of growth factors, plays a role in LTP [65–67]. One of the mechanisms governing the regulation of BDNF expression is histone acetylation, where KANSL1 contributes significantly as a histone acetyltransferase complex. KANSL1 deficiency might severely affect BDNF expression, which further promotes long-term potentiation impairment and synaptic plasticity. Both are considered to play an important role in memory formation [68] (Fig. 3B).
Pathways implicating genes from mechanistic model
The investigation on the presence, or lack thereof, of KANSL1 in pathways from KEGG, Reactome, and WikiPathways revealed that the KANSL1 gene is largely absent in the major pathway databases. While KANSL1 does participate in the “Chromatin Organization (Homo Sapiens)” and “Pathways Affected in Adenoid Cystic Carcinoma (Homo sapiens)” pathways from WikiPathways, no interaction information for this gene is provided. Moreover, KANSL1 is altogether absent in pathways from KEGG and Reactome. Similarly, we queried pathways from the three databases for all other genes from our mechanistic model (Supplementary Table 7). Unsurprisingly, well-studied genes yielded a higher number of pathways which they participate in (e.g., BDNF was found in 33 pathways across KEGG, Reactome, and WikiPathways), while genes with fewer literature evidence were scarcely present (e.g., KAT8 was found in one pathway across KEGG, Reactome and WikiPathways, however lacked interaction information). Furthermore, these pathway resources do not yet capture SNPs nor image features. Accordingly, the mechanism by which KANSL1 may be implicated in hippocampal atrophy can thus far only be inferred through dedicated modeling approaches, such as the one we have presented in this work.
Assessment of putative KANSL1-mediated mechanism with experimental databases
The putative KANSL1-dependent hippocampal atrophy mechanisms identified through systematically harvested knowledge is based on qualitative information. To further support the mechanisms of action exerted by KANSL1 in the nervous system, we screened evidence from experimental databases containing data sets on knockout mouse models in the Mouse Genome Informatics database [69]. In this database, we queried for KANSL1 and nervous system and found two knockout mice studies which investigated how KANSL1/MAPT dysregulation may cause hippocampal shrinkage [70, 71]. These studies associated tau hyperphosphorylation coupled with impaired microtubule binding of tau with reduction in synaptic transmission and altered synaptic plasticity. Furthermore, the authors argue that these mechanisms may lead to neuronal apoptosis and hippocampal shrinkage (Fig. 3A).
Additionally, with respect to SNPs that occur in the non-coding regions of the gene, we used RegulomeDB [72] to functionally annotate the 60 SNPs associated with KANSL1. RegulomeDB scores SNPs based on transcription factor binding sites, position weight matrix for transcription factor binding, DNase footprinting, open chromatin and chromatin states, expression quantitative trait loci (eQTL), and validated functional SNPs. Moreover, it calculates a score that represents the probability of being a regulatory variant based on functional genomics features along with continuous values such as ChIP-seq signal, DNase-seq signal, information content change, and DeepSEA scores for each SNPs [73]. From the 60 SNPs, our analysis suggested that 11 of them are located in the functional region of KANSL1 (Supplementary Table 8).
DISCUSSION
While the exact mechanism of action of KANSL1 remains obscure, the proposed methodology was able to identify the mechanisms through which it may have an impact on hippocampal atrophy. This demonstrates how the mechanism enrichment approach offers improved interpretation of molecular mechanisms involved in disease pathobiology. Ultimately, the hypotheses derived from such approaches can foster research by identifying unexplored links that have not been validated in the laboratory.
We observed that the information pertaining to different biological scales is not equally distributed in the literature. For example, there is a paucity of results reported at the phenotypic level, compared to those at the molecular or organ level. Shortcomings in knowledge representation at different scales are also reflected in pathway databases which currently do not contain information on SNPs or neuroimaging features. Consequently, linking molecular mechanisms to clinical readouts is one of the great challenges in biomedical informatics.
The results presented in this work are hypotheses that require further investigation. We have shown that despite the scarcity of knowledge from the literature around KANSL1, our approach was able to reveal interesting hypotheses. This sparsity of information surrounding KANSL1 combined with its manifestation as a novel AD associated gene motivates future updates of the knowledge assembly as new information becomes available. Furthermore, in our attempt to validate our hypothesis, we did not find any of the genetic variations of KANSL1 in major AD cohorts, such as Alzheimer’s Disease Neuroimaging Initiative and AddNeuroMed [74, 75]. Thus, future work can include measurements of these genetic variations as well as their expression in these and other independent cohorts. Using these quantitative measurements, if available, several tools can be employed to elucidate pathway signatures in disease as well drug-perturbed states, which can then be used to prioritize drug candidates relevant to the particular disease under investigation when these signatures are anti-correlated [76]. Similarly, gene expression measurements paired with a network containing prior knowledge on drug-disease data can also be used for drug candidate identification [77]. Finally, looking ahead, the presented strategy can be applied to other AD genes or across disease domains such as psychiatric diseases.
ACKNOWLEDGMENTS
The authors would like to acknowledge the financial support from the B-IT foundation.
Authors’ disclosures available online (https://www.j-alz.com/manuscript-disclosures/20-1397r2).
SUPPLEMENTARY MATERIAL
[1] The supplementary material is available in the electronic version of this article: https://dx.doi.org/10.3233/JAD201397.
REFERENCES
[1] | Heemels MT ((2016) ) Neurodegenerative diseases. Nature 539: , 179. |
[2] | Ahmad K , Hassan Baig M , Mushtaq G , Amjad Kamal M , Greig NH , Choi I ((2017) ) Commonalities in biological pathways, genetics, and cellular mechanism between Alzheimer’s disease and other neurodegenerative diseases: An in silico-updated overview. Curr Alzheimer Res 14: , 1190–1197. |
[3] | Rajput AH , Rozdilsky B , Rajput A ((1993) ) Alzheimer’s disease and idiopathic Parkinson’s disease coexistence. J Geriatr Psychiatry Neurol 6: , 170–176. |
[4] | Braskie MN , Ringman J M , Thompson PM ((2011) ) Neuroimaging measures as endophenotypes in Alzheimer’s disease. Int J Alzheimers Dis 2011: , 490140. |
[5] | Scheltens P , van de Pol L ((2012) ) Atrophy of medial temporal lobes on MRI in “probable” Alzheimer’s disease and normal ageing: Diagnostic value and neuropsychological correlates. J Neurol Neurosurg Psychiatry 83: , 1038–1040. |
[6] | Iyappan A , Younesi E , Redolfi A , Vrooman H , Khanna S , Frisoni GB , Hofmann-Apitius M ((2017) ) Neuroimaging feature terminology: A controlled terminology for the annotation of brain imaging features. J Alzheimers Dis 59: , 1153–1169. |
[7] | Meyer-Lindenberg A , Nicodemus KK , Egan MF , Callicott JH , Mattay V , Weinberger DR ((2008) ) False positives in imaging genetics. Neuroimage 40: , 655–661. |
[8] | Wachinger C , Nho K , Saykin AJ , Reuter M , Rieckmann A , Alzheimer’s Disease Neuroimaging Initiative ((2018) ) A longitudinal imaging genetics study of neuroanatomical asymmetry in Alzheimer’s disease. Biol Psychiatry 84: , 522–530. |
[9] | Biffi A , Anderson CD , Desikan RS , Sabuncu M , Cortellini L , Schmansky N , Salat D , Rosand J ; Alzheimer’s Disease Neuroimaging Initiative (ADNI) ((2010) ) Genetic variation and neuroimaging measures in Alzheimer disease. Arch Neurol 67: , 677–685. |
[10] | Lambert JC , Ibrahim-Verbaas CA , Harold D , Naj AC , Sims R , Bellenguez C , DeStafano AL , Bis JC , Beecham GW , Grenier-Boley B , Russo G , Thorton-Wells TA , Jones N , Smith AV , Chouraki V , Thomas C , Ikram MA , Zelenika D , Vardarajan BN , Kamatani Y , Lin CF , Gerrish A , Schmidt H , Kunkle B , Dunstan ML , Ruiz A , Bihoreau MT , Choi SH , Reitz C , Pasquier F , Cruchaga C , Craig D , Amin N , Berr C , Lopez OL , De Jager PL , Deramecourt V , Johnston JA , Evans D , Lovestone S , Letenneur L , Morón FJ , Rubinsztein DC , Eiriksdottir G , Sleegers K , Goate AM , Fiévet N , Huentelman MW , Gill M , Brown K , Kamboh MI , Keller L , Barberger-Gateau P , McGuiness B , Larson EB , Green R , Myers AJ , Dufouil C , Todd S , Wallon D , Love S , Rogaeva E , Gallacher J , St George-Hyslop P , Clarimon J , Lleo A , Bayer A , Tsuang DW , Yu L , Tsolaki M , Bossú P , Spalletta G , Proitsi P , Collinge J , Sorbi S , Sanchez-Garcia F , Fox NC , Hardy J , Deniz Naranjo MC , Bosco P , Clarke R , Brayne C , Galimberti D , Mancuso M , Matthews F ; European Alzheimer’s Disease Initiative (EADI); Genetic and Environmental Risk in Alzheimer’s Disease; Alzheimer’s Disease Genetic Consortium; Cohorts for Heart and Aging Research in Genomic Epidemiology, Moebus S , Mecocci P , Del Zompo M , Maier W , Hampel H , Pilotto A , Bullido M , Panza F , Caffarra P , Nacmias B , Gilbert JR , Mayhaus M , Lannefelt L , Hakonarson H , Pichler S , Carrasquillo MM , Ingelsson M , Beekly D , Alvarez V , Zou F , Valladares O , Younkin SG , Coto E , Hamilton-Nelson KL , Gu W , Razquin C , Pastor P , Mateo I , Owen MJ , Faber KM , Jonsson PV , Combarros O , O’Donovan MC , Cantwell LB , Soininen H , Blacker D , Mead S , Mosley TH Jr , Bennett DA , Harris TB , Fratiglioni L , Holmes C , de Bruijn RF , Passmore P , Montine TJ , Bettens K , Rotter JI , Brice A , Morgan K , Foroud TM , Kukull WA , Hannequin D , Powell JF , Nalls MA , Ritchie K , Lunetta KL , Kauwe JS , Boerwinkle E , Riemenschneider M , Boada M , Hiltuenen M , Martin ER , Schmidt R , Rujescu D , Wang LS , Dartigues JF , Mayeux R , Tzourio C , Hofman A , Nöthen MM , Graff C , Psaty BM , Jones L , Haines JL , Holmans PA , Lathrop M , Pericak-Vance MA , Launer LJ , Farrer LA , van Duijn CM , Van Broeckhoven C , Moskvina V , Seshadri S , Williams J , Schellenberg GD , Amouyel P ((2013) ) Meta-analysis of 74,046 individuals identifies 11 new susceptibility loci for Alzheimer’s disease. Nature Genet 45: , 1452. |
[11] | Ruiz A , Heilmann S , Becker T , Hernández I , Wagner H , Thelen M , Mauleón A , Rosende-Roca M , Bellenguez C , Bis JC , Harold D , Gerrish A , Sims R , Sotolongo-Grau O , Espinosa A , Alegret M , Arrieta JL , Lacour A , Leber M , Becker J , Lafuente A , Ruiz S , Vargas L , Rodríguez O , Ortega G , Dominguez MA ; IGAP, Mayeux R , Haines JL , Pericak-Vance MA , Farrer LA , Schellenberg GD , Chouraki V , Launer LJ , van Duijn C , Seshadri S , Antúnez C , Breteler MM , Serrano-Ríos M , Jessen F , Tárraga L , Nöthen MM , Maier W , Boada M , Ramírez A ((2014) ) Follow-up of loci from the International Genomics of Alzheimer’s Disease Project identifies TRIP4 as a novel susceptibility gene. Transl Psychiatry 4: , e358. |
[12] | Chan S L , Kim W S , Kwok J B , Hill A F , Cappai R , Rye K A , Garner B ((2008) ) ATP binding cassette transporter A7 regulates processing of amyloid precursor protein in vitro. J Neurochem 106: , 793–804. |
[13] | Casey BJ , Soliman F , Bath KG , Glatt CE ((2010) ) Imaging genetics and development: Challenges and promises. Hum Brain Mapp 31: , 838–851. |
[14] | Turner BO , Paul EJ , Miller MB , Barbey AK ((2018) ) Small sample sizes reduce the replicability of task-based fMRI studies. Commun Biol 1: , 62. |
[15] | Stefanovski L , Triebkorn P , Spiegler A , Diaz-Cortes MA , Solodkin A , Jirsa V , McIntosh AR , Ritter P ; Alzheimer’s Disease Neuroimaging Initiative ((2019) ) Linking molecular pathways and large-scale computational modeling to assess candidate disease mechanisms and pharmacodynamics in Alzheimer’s Disease. Front Comput Neurosci 13: , 54. |
[16] | SlaterT ((2014) ) Recent advances in modeling languages for pathway maps and computable biological networks. Drug Discov Today 19: , 193–198. |
[17] | Neve RL , McPhie DL ((2006) ) The cell cycle as a therapeutic target for Alzheimer’s disease. Pharmacol Ther 111: , 99–113. |
[18] | Cenini G , Voos W ((2019) ) Mitochondria as potential targets in Alzheimer’s disease therapy: An Update. Front Pharmacol 10: , 192. |
[19] | Hoyt CT , Konotopez A , Ebeling C ((2018) ) PyBEL: A computational framework for Biological Expression Language. Bioinformatics 34: , 703–704. |
[20] | Domingo-Fernández D , Kodamullil AT , Iyappan A , Naz M , Emon MA , Raschka T , Karki R , Springstubbe S , Ebeling C , Hofmann-Apitius M ((2017) ) Multimodal mechanistic signatures for neurodegenerative diseases (NeuroMMSig): A web server for mechanism enrichment. Bioinformatics 33: , 3679–3681. |
[21] | Li H , Roossinck MJ ((2004) ) Genetic bottlenecks reduce population variation in an experimental RNA virus population. J Virol 78: , 10582–10587. |
[22] | Lewis C M , Knight J ((2012) ) Introduction to genetic association studies. Cold Spring Harb Protoc 2012: , 297–306. |
[23] | Lee SH , Yang J , Goddard ME , Visscher PM , Wray NR ((2012) ) Estimation of pleiotropy between complex diseases using single-nucleotide polymorphism-derived genomic relationships and restricted maximum likelihood. Bioinformatics 28: , 2540–2542. |
[24] | Ward LD , Kellis M ((2012) ) HaploReg: A resource for exploring chromatin states, conservation, and regulatory motif alterations within sets of genetically linked variants. Nucleic Acids Res 40: , 930–934. |
[25] | Piñero J , Bravo Á , Queralt-Rosinach N , Gutiérrez-Sacristán A , Deu-Pons J , Centeno E , García-García J , Sanz F , Furlong LI ((2017) ) DisGeNET: A comprehensive platform integrating information on human disease-associated genes and variants. Nucleic Acids Res 45: , 833–839. |
[26] | Tyler AL , Crawford DC , Pendergrass SA ((2016) ) The detection and characterization of pleiotropy: Discovery, progress, and promise. Brief Bioinform 17: , 13–22. |
[27] | Nussbaum RL , Ellis CE ((2003) ) Alzheimer’s disease and Parkinson’s disease. N Engl J Med 348: , 1356–1364. |
[28] | Catalá-López F , Crespo-Facorro B , Vieta E , Valderas JM , Valencia A , Tabarés-Seisdedos R ((2014) ) Alzheimer’s disease and cancer: Current epidemiological evidence for a mutual protection. Neuroepidemiology 42: , 121–122. |
[29] | Rosen AR , Steenland NK , Hanfelt J , Factor SA , Lah JJ , Levey AI ((2007) ) Evidence of shared risk for Alzheimer’s disease and Parkinson’s disease using family history. Neurogenetics 8: , 263–70. |
[30] | Vacic V , Ozelius LJ , Clark LN , Bar-Shira A , Gana-Weisz M , Gurevich T , Gusev A , Kedmi M , Kenny EE , Liu X , Mejia-Santana H , Mirelman A , Raymond D , Saunders-Pullman R , Desnick RJ , Atzmon G , Burns ER , Ostrer H , Hakonarson H , Bergman A , Barzilai N , Darvasi A , Peter I , Guha S , Lencz T , Giladi N , Marder K , Pe’er I , Bressman SB , Orr-Urtreger A ((2014) ) Genome-wide mapping of IBD segments in an Ashkenazi PD cohort identifies associated haplotypes. Hum Mol Genet 23: , 4693–4702. |
[31] | Lill CM , Roehr JT , McQueen MB , Kavvoura FK , Bagade S , Schjeide BM , Schjeide LM , Meissner E , Zauft U , Allen NC , Liu T , Schilling M , Anderson KJ , Beecham G , Berg D , Biernacka JM , Brice A , DeStefano AL , Do CB , Eriksson N , Factor SA , Farrer MJ , Foroud T , Gasser T , Hamza T , Hardy JA , Heutink P , Hill-Burns EM , Klein C , Latourelle JC , Maraganore DM , Martin ER , Martinez M , Myers RH , Nalls MA , Pankratz N , Payami H , Satake W , Scott WK , Sharma M , Singleton AB , Stefansson K , Toda T , Tung JY , Vance J , Wood NW , Zabetian CP ; 23andMe Genetic Epidemiology of Parkinson’s Disease Consortium; International Parkinson’s Disease Genomics Consortium; Parkinson’s Disease GWAS Consortium; Wellcome Trust Case Control Consortium, Young P , Tanzi RE , Khoury MJ , Zipp F , Lehrach H , Ioannidis JP , Bertram L ((2012) ) Comprehensive research synopsis and systematic meta-analyses in Parkinson’s disease genetics: The PDGene database. PLoS Gene 8: , e1002548. |
[32] | Kanehisa M , Furumichi M , Tanabe M , Sato Y , Morishima K ((2016) ) KEGG: New perspectives on genomes, pathways, diseases and drugs. Nucleic Acids Res 45: , 353–361. |
[33] | Fabregat A , Sidiropoulos K , Garapati P , Gillespie M , Hausmann K , Haw R , Jassal B , Jupe S , Korninger F , McKay S , Matthews L , May B , Milacic M , Rothfels K , Shamovsky V , Webber M , Weiser J , Williams M , Wu G , Stein L , Hermjakob H , D’Eustachio P ((2018) ) The reactome pathway knowledgebase. Nucleic Acids Res 46: , 649–655. |
[34] | Slenter DN , Kutmon M , Hanspers K , Riutta A , Windsor J , Nunes N , Mélius J , Cirillo E , Coort SL , Digles D , Ehrhart F , Giesbertz P , Kalafati M , Martens M , Miller R , Nishida K , Rieswijk L , Waagmeester A , Eijssen LMT , Evelo CT , Pico AR , Willighagen EL ((2018) ) WikiPathways: A multifaceted pathway database bridging metabolomics to other omics research. Nucleic Acids Res 46: , 661–667. |
[35] | Jun G , Ibrahim-Verbaas CA , Vronskaya M , Lambert JC , Chung J , Naj AC , Kunkle BW , Wang LS , Bis JC , Bellenguez C , Harold D , Lunetta KL , Destefano AL , Grenier-Boley B , Sims R , Beecham GW , Smith AV , Chouraki V , Hamilton-Nelson KL , Ikram MA , Fievet N , Denning N , Martin ER , Schmidt H , Kamatani Y , Dunstan ML , Valladares O , Laza AR , Zelenika D , Ramirez A , Foroud TM , Choi SH , Boland A , Becker T , Kukull WA , van der Lee SJ , Pasquier F , Cruchaga C , Beekly D , Fitzpatrick AL , Hanon O , Gill M , Barber R , Gudnason V , Campion D , Love S , Bennett DA , Amin N , Berr C , Tsolaki M , Buxbaum JD , Lopez OL , Deramecourt V , Fox NC , Cantwell LB , Tárraga L , Dufouil C , Hardy J , Crane PK , Eiriksdottir G , Hannequin D , Clarke R , Evans D , Mosley TH Jr , Letenneur L , Brayne C , Maier W , De Jager P , Emilsson V , Dartigues JF , Hampel H , Kamboh MI , de Bruijn RF , Tzourio C , Pastor P , Larson EB , Rotter JI , O’Donovan MC , Montine TJ , Nalls MA , Mead S , Reiman EM , Jonsson PV , Holmes C , St George-Hyslop PH , Boada M , Passmore P , Wendland JR , Schmidt R , Morgan K , Winslow AR , Powell JF , Carasquillo M , Younkin SG , Jakobsdóttir J , Kauwe JS , Wilhelmsen KC , Rujescu D , Nöthen MM , Hofman A , Jones L ; IGAP Consortium, Haines JL , Psaty BM , Van Broeckhoven C , Holmans P , Launer LJ , Mayeux R , Lathrop M , Goate AM , Escott-Price V , Seshadri S , Pericak-Vance MA , Amouyel P , Williams J , van Duijn CM , Schellenberg GD , Farrer LA ((2016) ) A novel Alzheimer’s disease locus located near the gene encoding tau protein. Mol Psychiatry 21: , 108–117. |
[36] | Todorova V , Blokland A ((2017) ) Mitochondria and synaptic plasticity in the mature and aging nervous system. Curr Neuropharmacol 15: , 166–173. |
[37] | Cai Y , Jin J , Swanson SK , Cole MD , Choi SH , Florens L , Washburn MP , Conaway JW , Conaway RC ((2010) ) Subunit composition and substrate specificity of a MOF-containing histone acetyltransferase distinct from the male-specific lethal (MSL) complex. J Biol Chem 285: , 4268–4272. |
[38] | Huang J , Wan B , Wu L , Yang Y , Dou Y , Lei M ((2012) ) Structural insight into the regulation of MOF in the male-specific lethal complex and the non-specific lethal complex. Cell Res 22: , 1078–1081. |
[39] | Gregory PD , Wagner K , Hörz W ((2001) ) Histone acetylation and chromatin remodeling. Exp Cell Res 265: , 195–202. |
[40] | Peixoto L , Abel T ((2013) ) The role of histone acetylation in memory formation and cognitive impairments. Neuropsychopharmacology 38: , 62–76. |
[41] | Bahari-Javan S , Sananbenesi F , Fischer A ((2014) ) Histone-acetylation: A link between Alzheimer’s disease and post-traumatic stress disorder?. Front Neurosci 8: , 160. |
[42] | Farioli-Vecchioli S , Saraulli D , Costanzi M , Leonardi L , Ciná I , Micheli L , Nutini M , Longone P , Oh SP , Cestari V , Tirone F ((2009) ) Impaired terminal differentiation of hippocampal granule neurons and defective contextual memory in PC3/Tis21 knockout mice. PLoS One 4: , e8339. |
[43] | Leal G , Bramham CR , Duarte CB ((2017) ) BDNF and hippocampal synaptic plasticity. Vitam Horm 104: , 153–195. |
[44] | Myers KA , McGlade A , Neubauer BA , Lal D , Berkovic SF , Scheffer IE , Hildebrand MS ((2018) ) KANSL1 variation is not a major contributing factor in self-limited focal epilepsy syndromes of childhood. PLoS One 13: , e0191546. |
[45] | Soliman ML , Smith MD , Houdek HM , Rosenberger TA ((2012) ) Acetate supplementation modulates brain histone acetylation and decreases interleukin-1β expression in a rat model of neuroinflammation. J Neuroinflammation 9: , 51. |
[46] | Vadnal J , Houston S , Bhatta S , Freeman E , McDonough J ((2012) ) Transcriptional signatures mediated by acetylation overlap with early-stage Alzheimer’s disease. Exp Brain Res 221: , 287–297. |
[47] | Kim S , Kaang BK ((2017) ) Epigenetic regulation and chromatin remodeling in learning and memory. Exp Mol Med 49: , e281. |
[48] | Moreno-Igoa M , Hernández-Charro B , Bengoa-Alonso A , Pérez-Juana-del-Casal A , Romero-Ibarra C , Nieva-Echebarria B , Ramos-Arroyo MA ((2015) ) KANSL1 gene disruption associated with the full clinical spectrum of 17q21.31 microdeletion syndrome. BMC Med Genet 16: , 68. |
[49] | Rouault JP , Falette N , Guéhenneux F , Guillot C , Rimokh R , Wang Q , Berthet C , Moyret-Lalle C , Savatier P , Pain B , Shaw P , Berger R , Samarut J , Magaud JP , Ozturk M , Samarut C , Puisieux A ((1996) ) Identification of BTG2, an antiproliferative p53-dependent component of the DNA damage cellular response pathway. Nat Genet 14: , 482–486. |
[50] | Rouault JP , Prévôt D , Berthet C , Birot AM , Billaud M , Magaud JP , Corbo L ((1998) ) Interaction of BTG1 and p53-regulated BTG2 gene products with mCaf1, the murine homolog of a component of the yeast CCR4 transcriptional regulatory complex. J Biol Chem 273: , 22563–22569. |
[51] | Terra R , Luo H , Qiao X , Wu J ((2008) ) Tissue-specific expression of B-cell translocation gene 2 (BTG2) and its function in T-cell immune responses in a transgenic mouse model. Int Immunol 20: , 317–326. |
[52] | Rossi G , Dalprá L , Crosti F , Lissoni S , Sciacca FL , Catania M , Di Fede G , Mangieri M , Giaccone G , Croci D , Tagliavini F ((2008) ) A new function of microtubule-associated protein tau: Involvement in chromosome stability. Cell Cycle 7: , 1788–1794. |
[53] | Jordán J , Galindo MF , Prehn JH , Weichselbaum RR , Beckett M , Ghadge GD , Roos RP , Leiden JM , Miller RJ ((1997) ) p53 expression induces apoptosis in hippocampal pyramidal neuron cultures. J Neurosci 17: , 1397–1405. |
[54] | Mattson MP , Liu D ((2002) ) Energetics and oxidative stress in synaptic plasticity and neurodegenerative disorders. Neuromolecular Med 2: , 215–231. |
[55] | Hroudová J , Fišar Z ((2013) ) Control mechanisms in mitochondrial oxidative phosphorylation. Neural Regen Res 8: , 363–375. |
[56] | Chatterjee A , Seyfferth J , Lucci J , Gilsbach R , Preissl S , Böttinger L , Mårtensson CU , Panhale A , Stehle T , Kretz O , Sahyoun AH , Avilov S , Eimer S , Hein L , Pfanner N , Becker T , Akhtar A ((2016) ) MOF acetyl transferase regulates transcription and respiration in mitochondria. Cell 167: , 722–738. |
[57] | Stryer L , L Tymoczko J , M Berg J ((2002) ) Biochemistry, Fifth Edition. W.H. Freeman and Company, ISBN-10:0-7167-3051-0. |
[58] | Johns P ((2016) ) Clinical Neuroscience. Churchill Livingstone, eBook ISBN: 9780702057137 |
[59] | Stepien KM , Heaton R , Rankin S , Murphy A , Bentley J , Sexton D , Hargreaves IP ((2017) ) Evidence of oxidative stress and secondary mitochondrial dysfunction in metabolic and non-metabolic disorders. J Clin Med 6: , 71. |
[60] | Koudinov AR , Koudinova NV ((2001) ) Essential role for cholesterol in synaptic plasticity and neuronal degeneration. FASEB J 15: , 1858–1860. |
[61] | Wang D , Zheng W ((2015) ) Dietary cholesterol concentration affects synaptic plasticity and dendrite spine morphology of rabbit hippocampal neurons. Brain Res 1622: , 350–360. |
[62] | Koudinov AR , Koudinova NV ((2003) ) Cholesterol, synaptic function and Alzheimer’s disease. Pharmacopsychiatry 36: (Suppl 2), S107–112. |
[63] | Cutler RG , Kelly J , Storie K , Pedersen WA , Tammara A , Hatanpaa K , Troncoso JC , Mattson MP ((2004) ) Involvement of oxidative stress-induced abnormalities in ceramide and cholesterol metabolism in brain aging and Alzheimer’s disease. Proc Natl Acad Sci U S A 101: , 2070–2075. |
[64] | Zhou Q , Homma KJ , Poo MM ((2004) ) Shrinkage of dendritic spines associated with long-term depression of hippocampal synapses. Neuron 44: , 749–57. |
[65] | Whalley K ((2007) ) Balancing LTP and LTD. Neuroscience 8: , 249–249. |
[66] | Edelmann E , Lessmann V , Brigadski T ((2014) ) Pre- and postsynaptic twists in BDNF secretion and action in synaptic plasticity. Neuropharmacology 76: , 610–627. |
[67] | Bagheri A , Habibzadeh P , Razavipour SF , Volmar CH , Chee NT , Brothers SP , Wahlestedt C , Mowla SJ , Faghihi MA ((2019) ) HDAC inhibitors induce BDNF expression and promote neurite outgrowth in human neural progenitor cells-derived neurons. Int J Mol Sci 20: , 1109. |
[68] | Malenka RC , Bear MF ((2004) ) LTP and LTD: An embarrassment of riches. Neuron 44: , 5–21. |
[69] | Geetha T , Zheng C , McGregor WC , White BD , Diaz-Meco MT , Moscat J , Babu JR ((2012) ) TRAF6 and p62 inhibit amyloid β-induced neuronal death through p75 neurotrophin receptor. Neurochem Int 61: , 1289–1293. |
[70] | Koss DJ , Robinson L , Drever BD , Plucińska K , Stoppelkamp S , Veselcic P , Riedel G , Platt B ((2016) ) Mutant Tau knock-in mice display frontotemporal dementia relevant behavior and histopathology. Neurobiol Dis 91: , 105–123. |
[71] | Rodríguez-Martín T , Pooler AM , Lau DHW , Mórotz GM , De Vos KJ , Gilley J , Coleman MP , Hanger DP ((2016) ) Reduced number of axonal mitochondria and tau hypophosphorylation in mouse P301L tau knockin neurons. Neurobiol Dis 85: , 1–10. |
[72] | Boyle AP , Hong EL , Hariharan M , Cheng Y , Schaub MA , Kasowski M , Karczewski KJ , Park J , Hitz BC , Weng S , Cherry JM , Snyder M ((2012) ) Annotation of functional variation in personal genomes using RegulomeDB. Genome Res 22: , 1790–1797. |
[73] | Dong S , Boyle AP ((2019) ) Predicting functional variants in enhancer and promoter elements using RegulomeDB. Hum Mutat 40: , 1292–1298. |
[74] | Mueller SG , Weiner MW , Thal LJ , Petersen RC , Jack C , Jagust W , Trojanowski JQ , Toga AW , Beckett L ((2005) ) The Alzheimer’s disease neuroimaging initiative. Neuroimaging Clin N Am 15: , 869–877. |
[75] | Lovestone S , Francis P , Kloszewska I , Mecocci P , Simmons A , Soininen H , Spenger C , Tsolaki M , Vellas B , Wahlund LO , Ward M ; AddNeuroMed Consortium ((2009) ) AddNeuroMed —the European collaboration for the discovery of novel biomarkers for Alzheimer’s disease. Ann N Y Acad Sci 1180: , 36–46. |
[76] | Emon MA , Domingo-Fernández D , Hoyt CT , Hofmann-Apitius M ((2020) ) PS4DR: A multimodal workflow for identification and prioritization of drugs based on pathway signatures. BMC Bioinformatics 21: , 1–21. |
[77] | Peyvandipour A , Saberian N , Shafi A , Donato M , Draghici S ((2018) ) A novel computational approach for drug repurposing using systems biology. Bioinformatics 34: , 2817–2825. |