Mortality Risk Models for Persons with Dementia: A Systematic Review
Abstract
Background:
Persons with dementia have higher mortality than the general population. Objective, standardized predictions of mortality risk in persons with dementia could help with planning resources for care close to the end of life.
Objective:
To systematically review prediction models for risk of death in persons with dementia.
Methods:
The Medline and PsycInfo databases were searched on November 29, 2020, for prediction models estimating the risk of death in persons with dementia. Study quality was assessed using the Prediction model Risk Of Bias ASsessment Tool.
Results:
The literature search identified 2,828 studies, of which 18 were included. These studies described 16 different prediction models with c statistics mostly ranging from 0.67 to 0.79. Five models were externally validated, of which four were applicable. There were two models that were both applicable and had reasonably low risk of bias. One model predicted risk of death at six months in persons with advanced dementia residing in a nursing home. The other predicted risk of death at three years in persons seen in primary care practice or a dementia specialty clinic, derived from a nationwide registry in Sweden but not externally validated.
Conclusion:
Valid, applicable models with low risk of bias were found in two settings: advanced dementia in a nursing home and outpatient practices. The outpatient model requires external validation. Better models are needed for persons with mild to moderate dementia in nursing homes, a common demographic. These models may be useful for educating persons living with dementia and care partners and directing resources for end of life care.
Registration:
The study protocol is registered on PROSPERO as RD4202018076.
INTRODUCTION
In most cases dementia is caused by incurable, progressive neurodegenerative diseases that will lead to death. The average duration of survival with dementia is seven to ten years [1]. Shorter survival has been associated with older age, male sex, lower cognition, neuropsychiatric symptoms, greater impairments in function, and more comorbidities [2]. Patients and care partners are usually aware that dementia will shorten the lifespan, and often ask their clinicians how long the patient has to live. The answer to this question can help persons living with dementia and their care partners understand the illness and what the future holds, motivate care planning for the future, and, in later stages, determine eligibility for palliative care services. Additionally, an emerging application during the Coronavirus Disease in 2019 (COVID-19) pandemic may be to identify patients with limited remaining lifespan that may not warrant triage to critical care resources when such resources are scarce [3].
Our objective was to determine whether there are validated risk scores that predict the risk of death in persons with dementia, suitable for application in either the general population, a primary care practice, a specialty dementia clinic, or in a nursing home.
METHODS
The CHecklist for critical Appraisal and data extraction for systematic Reviews of prediction Modelling Studies (CHARMS) [4] was used to guide the definition of the research question and design of the systematic review. The objective was to systematically identify prognostic models that would allow the user to calculate an estimated probability of death at 6 months or later in persons with dementia. Models that estimated median survival were also considered potentially applicable. The required setting was a nursing home, outpatient practice, or in the general population. The study protocol is registered on PROSPERO as CRD42020180760.
Study selection criteria required that all participants had dementia at inception, that the dementia was either caused by Alzheimer’s disease or included all types of dementia, that outcomes were assessed at 6 months or later, and that either all-cause mortality or duration of survival was reported as an outcome. Studies were required to report a multivariable-adjusted risk score or a multivariable model from which the user could make individualized predictions of 6-month, 1-year, or longer-term estimated percent mortality or estimated survival. Studies were excluded if they predicted mortality only in non-Alzheimer’s causes of dementias (e.g., Lewy body dementia) or in subpopulations based on risk factors (e.g., hypertension), acute conditions (e.g., pneumonia) or other factors; if they used longitudinal information (e.g., rate of change on test scores) as a predictor; or if they analyzed risk factors for mortality without developing or validating a prognostic model to predict risks in individuals. The included settings were a population-based study, family practice, dementia specialty practice, or long-term care home. Studies of model development, external validation, or comparison were included.
The Medline and PsycInfo databases were searched on November 29, 2020, using keywords and medical subject headings for dementia, risk, prediction, death, and mortality (full search terms are provided in Supplementary Table 1). There were no restrictions on study dates or language. Two reviewers (ES and ZI) screened each abstract and read each full text article for eligibility. The Covidence website (http://www.covidence.org) was used to organize the screening. Reasons for excluding full text articles were recorded in these pre-specified categories: unable to retrieve, letter or abstract only, wrong population, review article, duplicate, wrong study design, wrong setting, wrong outcomes, pediatric population.
Data were extracted independently by two reviewers (ES and ZI) with discrepancies resolved by consensus. If consensus was not achieved, the data element were described as unclear and the reasons for the lack of clarity was recorded. These data were extracted: year of publication, years of data collection, setting, sample size, number of outcomes (deaths), country of origin, population selection criteria, percent women, age (central tendency and distribution), type of model (development, validation, or both), candidate predictors, final predictors, analysis methods, measures of discrimination, measures of calibration, internal validation, external validation, other significant results, and limitations. Studies were considered to undergo external validation only when tested in an independent dataset, not when the original sample was split.
Quality was assessed using the Prediction model Risk Of Bias ASsessment Tool (PROBAST) [5], following guidance for its use [6]. Risk of bias and applicability was rated by two reviewers, using the recommended categories of Yes, Probably Yes, No, Probably No, or No Information. For risk of bias, the number of Yes and Probably Yes responses for 20 signaling questions were tabulated, and reported as ≥18, 15–17, and <17. Studies were considered overall good quality if they were applicable to the study question, reported both discrimination and calibration, and had a quality score of ≥18. Publication bias was suspected for studies that reported high discrimination with small samples (less than 20 events per predictor).
The research question was developed based on the work of a taskforce on COVID-19 and dementia sponsored by the Alzheimer Society of Canada, a charitable organization working on behalf of persons with dementia. A subcommittee of this taskforce has published guidance for triaging persons with dementia for critical care, including recommendations that survival from underlying dementia should be estimated based on objective, evidence-based models [7].
RESULTS
The search returned 3,070 articles, of which 73 were read in full and 18 were ultimately selected for inclusion (Fig. 1). One study [8] retrospectively analyzed administrative health data and provided a figure showing mortality rates stratified by age, sex and organ failure; however, the authors wrote that they “did not aim to build a prediction model” and therefore the paper was excluded.
Fig. 1
Study selection.
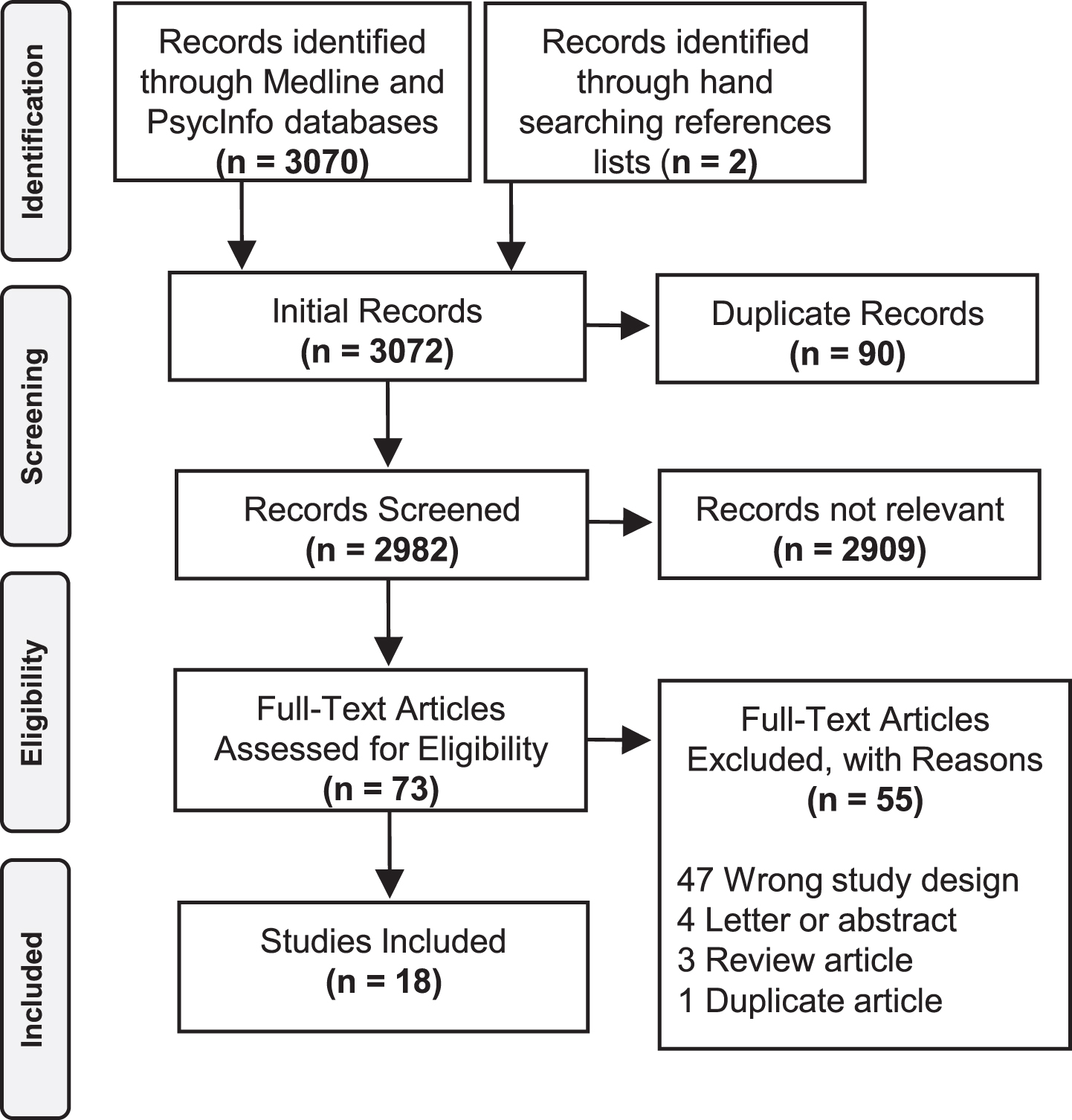
Characteristics of the studies are shown in Table 1. Sixteen studies included development of a model of which three also included an external validation in the same paper, while two reported external validation of a previously published model. The models were developed in nursing home populations (4 studies), in administrative or electronic health record databases of claims (3), dementia specialty clinics (2), geriatrics inpatient units or day programs (2), convenience samples from the community (2), incident dementia cases in a population-based study (1), prevalent dementia in a community survey (1), and participants in a randomized controlled trial of case management (1). The mean age of participants ranged from 76 to 86 years. The proportion that were women ranged from 53 to 82%.
Table 1
Study Characteristics
Study author, year [Ref] | Model type | Data source | Years collected | Age | Women |
Carlson, 2001 [9] | Development | Single long term care home | 1994–1996 | 79.4 (7.8) | 72.1% |
Cheng, 2019 [19] | Development | Taiwan claims database | 2002–2013 | 79.01 | 52.7% |
Delva, 2013 [28] | Development and validation | Incident dementia within pop.f-based cohort studies | 1999–2013 | 86.4 (5.5) | 67.4% |
Haaksma, 2019 [20] | Development | Newly diagnosed dementia from three specialty clinics | 2010–2018 | 76.2, 67.4–83.0 | 54.7% |
Haaksma, 2020 [18] | Development | National dementia clinic registry | 2007–2016 | 81.6, 76.5–86.0 | 59.4% |
Mitchell, 2004 [22] | Development and validation | Advanced dementia* in all nursing homes in NY (derivation) and Michigan (validation) | 1994–2000 | Median 83 | 66.8% |
Mitchell, 2010 [17] | Development | Advanced dementia* with admission or annual assessment in all nursing homes in USA | 2002-2003 | 84.5 (7.5) | 77.0% |
Mitchell, 2010 [25] | Validation | Advanced dementia* in nursing home, prospectively consented | 2007–2009 | Mode 85–90 | 81.9% |
Newcomer, 2003 [10] | Development | Participants in RCT of outpatient dementia case management | 1989–1994 | Mode 70–79 | 60.5% |
Paradise, 2009 [14] | Development | Mild-moderate dementia (MMSE≥10) recruited from community | NR | 81.0 (7.1) | 70.9% |
Pilotto, 2009 [13] | Development | Hospitalized in a geriatrics unit | 2004–2007 | 80.8 (6.7) | 65.6% |
Stern, 1997 [15] | Development and validation | Outpatients with mild dementia (defined as modified MMSE< =30 out of 100) | NR | 73.1 (8.9) | 59.3% |
Sultana, 2018 [11] | Development | Primary care registry | 2000–2012 | 79.3 (6.2) | 35.0% |
van der Steen, 2007 [24] | Validation | Netherlands cohort: Advanced dementia* in nursing home; USA cohort: Nursing home with lower respiratory tract infection | 2004–2006 | Netherlands: 56.6%> 83, Missouria 62.8%> 83 | 74.7% |
van der Vorst, 2020 [12] | Development | Admitted to hospital or attending a hospital day clinic | 2000–2010 | Mode 80–89 | 61.3% |
van Dijk, 1996 [21] | Development | Single nursing home | 1982–1990 | 80.8 (6.8) | 72.1% |
Wang, 2010 [23] | Development | Diagnosed with dementia in community survey (10/66) | 2004–2009 | 79.1 (6.9) | 62.0% |
Wang, 2019 [16] | Development | Diagnosis of dementia in health system electronic health record | 2011–2017 | 74.8 (13.2) | 60.4% |
“Development” refers to model development without external validation, while “Validation” refers to external validation. RCT, randomized controlled trial. *Defined as Cognitive Performance Score 5 or 6.
Study quality according to PROBAST criteria is shown in Table 2. Eight of the studies had concerns about applicability, either because they did not provide a usable probability table or provided only a simple cut-off with sensitivity and specificity (3 studies) [9–11], included hospitalized inpatients (2) [12, 13], or used predictors that were difficult to obtain, poorly defined, subjective, or non-standardized (3) [14–16].
Table 2
Study Quality
Risk of bias | Applicability | Overall | ||||||
Participants | Predictors | Analysis | Participants | Predictors | Outcome | ROB | Applicability | |
Carlson, 2001 [9] | + | + | – | + | – | – | – | – |
Cheng, 2019 [19] | + | + | – | + | + | + | – | + |
Delva, 2013 [28] | + | + | – | + | + | + | – | + |
Haaksma, 2019 [20] | + | + | – | + | + | + | – | + |
Haaksma, 2020 [18] | + | + | + | + | + | + | + | + |
Mitchell, 2004 [22] | + | + | – | + | + | + | – | + |
Mitchell, 2010 [17] | + | + | + | + | + | + | + | + |
Mitchell, 2010 [25] | + | + | + | + | + | + | + | + |
Newcomer, 2003 [10] | + | + | – | + | + | – | – | – |
Paradise, 2009 [14] | + | + | – | + | – | + | – | – |
Pilotto, 2009 [13] | – | + | – | – | + | + | – | – |
Stern, 1997 [15] | + | + | – | + | – | + | – | – |
Sultana, 2018 [11] | – | – | – | + | – | – | – | – |
van der Steen, 2007 [24] | + | + | + | + | + | + | – | + |
van der Vorst, 2020 [12] | + | + | – | – | + | + | – | – |
van Dijk, 1996 [21] | + | + | – | + | + | + | – | + |
Wang, 2010 [23] | + | + | – | + | + | + | – | + |
Wang, 2019 [16] | – | – | – | + | – | + | – | – |
+ indicates low risk of bias (ROB)/low concern regarding applicability; –indicates high ROB/high concern regarding applicability. Risk of bias for determining the outcome (death) was deemed to be low for all studies, and therefore is not shown.
Most studies were deemed to be at some risk for bias, usually for analytic reasons. Common reasons included lack of information on discrimination and calibration, lack of internal validation, insufficient numbers of events, lack of accounting for missing data or using complete case analysis, and selecting final candidates based on the results of univariate p value testing (more details available in Supplementary Table 2). Of the 16 studies reporting model development, 2 had low risk of bias for ≥18 PROBAST signaling questions [17, 18], 7 had low risk of bias for 15–17 signaling questions[9, 10, 12, 19–21], and 7 had low risk of bias for <15 signaling questions [11, 13–16, 22, 23]. The two highest quality studies were the Advanced Dementia Prognostic Tool (ADEPT) [17], derived to predict risk of death at 6 months among persons with advanced dementia in nursing homes, and a prognostic model for risk of death at 6 months among persons with dementia seen in outpatient primary care or dementia specialty clinics contributing data to a nationwide registry [18].
Results of model development are shown in Table 3. C statistics ranged from 0.68 to 0.79, with the exception of a paper that described machine learning-based models with very high c statistics of ≥0.94 [16]. However, that machine learning paper was deemed to be at high risk of bias due to overfitting because of the large number of predictors (all information in the electronic health records) and was of low applicability to the research question because data from all medical encounters were used rather than data from a single inception point. Model calibration was generally reported to be good, when it was assessed. However, many studies only reported the Homer-Lemeshow test, rather than tabulating or plotting observed versus expected deaths as recommended by recent guidelines [6].
Table 3
Results of Model Development
Study author, year [Ref] | Size (n) | Final predictors | Timing (y) | C statistic | Calibration |
Carlson, 2001 [9] | 132 (60) | BEHAVE AD delusions, General Medical Heath Rating | 5 | NR | NR |
Cheng, 2019 [19] | 6,556 (2,543) | Age, sex, COPD, CHF, diabetes, recent (< 3 month) lower respiratory infection, recent urinary tract infection, chronic kidney disease, myocardial infarction, cancer, recent ng tube insertion | 0.5, 1, 2, 3, 5 | 1 y 0.7792 y 0.7253 y 0.7215 y 0.703 | Hosmer-Lemeshow |
Delva, 2013 [28] | 454 (319) | Male sex, age, number of restricted ADLs | 5 | 0.754 | Hosmer-Lemeshow |
Haaksma, 2019 [20] | 331 | Cumulative Illness Rating Scale for Geriatrics, Frailty Index | 1, 3, 6 | 0.72 | NR |
Haaksma, 2020 [18] | 50,076 (20,828) | Memory clinic: age, sex, MMSE, Charlson comorbidity index, dementia subtype; Primary care: Age, sex, MMSE, Charlson comorbidity index | 3 | Memory clinic: men 0.71, women 0.72 Primary care: men 0.70, women 0.71 | O-E plot |
Mitchell, 2004 [22] | 6,799 (1,922) | Completely dependent for ADLs, male, cancer, oxygen therapy, CHF, SOB,< 25%food eaten, unstable medical condition, bowel incontinence, bedfast, age above median, not awake most of day | 0.5 | 0.74 | NR |
Mitchell, 2010 [17] | 222,405 (90,324) | Length of stay, age, male, dyspnea, pressure ulcers, total functional dependence, bedfast, insufficient intake, bowel incontinence, BMI, weight loss, CHF | 1 | 0.68 | O-E plot |
Newcomer, 2003 [10] | 3858 (derivation) and 1559 (validation) | Age, sex, number ADLs needing help, caregiver relationship, inpatient stays in last 12 months, CHF or cardiac condition, COPD, cancer (except minor skin), diabetes | 1 | 0.69 | O-E table |
Paradise, 2009 [14] | 158 (derivation) and 241 (validation) | Age, constructional apraxia, gait apraxia | 1, 2, 3, 3.5 | NR | NR |
Pilotto, 2009 [13] | 262 (49) | Age, sex, MPI | 0.5, 1 | 0.5 y 0.79 1 y 0.78 | O-E table |
Stern, 1997 [15] | 236 (99) | Sex, extrapyramidal signs, mMMS score, duration in years | Est’d months to death | NR | O-E plot |
Sultana, 2018 [11] | Overall: 15,300 (1,656) With accommodation 1174 (286) With mobility 1497 (286) With dressing 143 (28) | Age, sex, QOF comorbidity score; then subpopulations with either accommodation, mobility or dressing data | 1 | Overall 0.66, mobility 0.59, dressing 0.69. | Calibration slope, O-E |
van der Vorst, 2020 [12] | 50,993 (17.923) | Age, Charlson comorbidity, setting type | 1, 3 | 1 y 0.71 3 y 0.72 | Hosmer-Lemeshow |
van Dijk, 1996 [21] | 606 (394) | Age, sex, pulmonary infection and stroke, cancer, atrial fibrillation, pressure sores, Parkinsonism, pulmonary infection without stroke, heart failure, diabetes mellitus, urinary incontinence, visual problems | 2 | NR | O-E plot |
Wang, 2010 [23] | 137 (91) | Age, substantial disability (WHO-DAS II > = 45), comorbidity, CDR | 5 | NR | NR |
Wang, 2019 [16] | 29613 (12385) | All EHR data | 0.5, 1 year, 2 year | 0.5 y 0.98 1 y 0.96 2 y 0.94 | NR |
NR, not reported; O-E, plot or table comparing observed versus expected. If studies reported split samples the total size and total number of deaths is reported. If studies reported a derivation and external validation cohort, the size of the derivation cohort is shown (details of external validation are shown in Table 4). ADEPT, Advanced Dementia Prognostic Tool; ADL, activity of daily living; BEHAVE-AD, Behavioral Pathology in Alzheimer’s Disease; BMI, body mass index; COPD, chronic obstructive pulmonary disease; mMMS, modified Mini-Mental State Examination; MMSE, Folstein Mini-Mental State Examination; SOB, shortness of breath; WHO-DAS II, World Health Organization Disability Assessment Schedule.
The results of external model validation are shown in Table 4. There were five studies that validated four models. The most extensively validated model was developed by Mitchell and colleagues to predict death at 6 months in persons with advanced dementia on admission to a nursing home [22]. The candidate predictors were taken from the Minimum Data Set, which is collected by regulation on admission to a nursing home and at regular intervals, at minimum every year thereafter. It was validated externally in 4,631 persons in the original derivation paper [22], and then subsequently in data from two other multicenter nursing home studies (n = 288 and n = 289) [24]. The C statistics from external validation (0.70, 0.65, 0.64) were somewhat lower than the original derivation cohort (0.74). The model was re-derived, and re-named ADEPT, in a 2010 publication that included persons already admitted to the nursing home, with a new variable for newly admitted versus existing resident [17]. In a prospective cohort of 606 persons recruited to validate ADEPT, the validation c-statistic (0.67) [25] was essentially the same as in the original derivation cohort (0.68). The two other external validation studies validated models derived from incident dementia cases in a population-based study and in outpatients with dementia.
Table 4
Model external validation
Study author, year [Ref] | Model | Population | Size (n) | C statistic | Calibration |
Delva, 2013 [28] | Ref 6 | Population-based 3C study | 267 (108) | 0.700 | Hosmer-Lemeshow |
Mitchell, 2004 [22] | Ref 9 | 440 nursing homes in Michigan | 4631 (1626) | 0.70 | NR |
Mitchell, 2010 [25] | Advanced Dementia Prognostic Tool [17] | Prospective cohort from 21 nursing homes | 606 (111) | 0.67 | O-E table |
Stern, 1997 [15] | Ref 15 | Prospective epidemiological study | 105 (NR) | NR | O-E plot |
van der Steen, 2007 [24] | Ref 9 | Netherlands: 6 nursing homes | Netherlands 288 (70) | Netherlands 0.65 | O-E table |
Missouri: Nursing home lower respiratory tract infection study in nursing homes | Missouri 269 (99) | Missouri 0.64 |
NR, not reported; O-E, plot or table comparing observed versus expected.
DISCUSSION
This review identified two models at reasonably low risk of bias that were applicable to the research question. The ADEPT score can be used to predict risk of death at 6 months in persons with advanced dementia residing in a nursing home [17], and has been externally validated [25]. A prognostic model derived from the Swedish Dementia Registry can be used to predict risk of death at 3 years in persons with dementia seen in specialty clinics or primary care practice. It was derived with good quality methods but has not yet been externally validated. Another 14 prognostic models were returned by the search but suffered from either methodological limitations that conferred higher risk of bias, or had concerns about applicability, or both.
The best validated prognostic model was the ADEPT score for predicting risk of death in persons with dementia in a nursing home [17]. The intended purpose for the model was to help identify persons meeting United States Medicare rules for eligibility for hospice care, which requires a life expectancy of less than 6 months. The ADEPT score was closely based on a previous model derived and externally validated in 2004 by the same author group [22]. ADEPT uses 12 predictor variables derived from the Minimum Data Set, a component of the federally mandated Resident Assessment Instrument (RAI) which is collected on nursing home residents on admission and annually each year thereafter. It displayed good discrimination and calibration in a cohort of 606 patients prospectively collected to test its validity [25]. A limitation of ADEPT is that, although deemed applicable to our research question, it is only valid for persons with very advanced dementia. It was derived and validated in persons with a RAI Cognitive Performance Score of 5 or 6, indicating very severe loss of function including eating problems. Most people with this level of impairment have a Folstein Mini-Mental State Exam (MMSE) score of 5 or less [26]. Within this population, ADEPT seems valid for continued use although additional new research on the calibration of the score would be welcome, as it was calibrated ten years ago and may not reflect contemporary trends in medical care and preferences regarding palliative care.
A prognostic model from the Swedish Dementia Registry was applicable to predicting death in patients with dementia attending either a primary care practice or a dementia specialty clinic. The score was derived from a nationwide registry including all dementia specialty clinics and about 75%of primary care practices in Sweden, with a large sample size. In the primary care setting, three-year risk of death was predicted by age, sex, MMSE, and Charlson comorbidity score, while in the specialty clinic setting cause of dementia was another predictor (classified as Alzheimer’s disease; vascular, mixed dementia, or other; or Lewy Body disease, Parkinson’s disease dementia, or frontotemporal dementia). The inclusion of dementia diagnosis in the specialty clinic model presumably reflects better clinical characterization by specialists, although the discrimination was similar in primary care (c statistic 0.70 for men and 0.71 for women) as in specialty care (c statistic 0.71 for men and 0.72 for women). This model seems suitable for use in the outpatient setting, although more research is required to externally validate it. Internal validation by bootstrapping was promising, with low shrinkage factors and little evidence of overoptimism [18].
Thirteen other models were identified in the systematic review, but eight were deemed not applicable to the research question and five others were deemed applicable but to be at high risk of bias due to various methodological shortcomings.
Among all the models, C statistics ranged from 0.67 to 0.79 (with one overly optimistic exception), suggesting that it is possible to predict risk of death in persons with dementia with sufficiently good discrimination to use this information to make individual prognoses.
There are several potential uses for prognostic model of death in persons with dementia. Patients and their care partners typically understand that dementia is irreversible and progressive. They deserve to have the best evidenced-based prognosis of survival and risk of death in this condition. This information can help reinforce the need for advanced planning for medical care, finances, and designating power of attorney. It could also be useful for determining eligibility for palliative care services, such as hospice, close to the end of life.
An additional prognostic need has emerged in the context of the COVID-19 pandemic. When access to ICUs and mechanical ventilation is limited by scarce resources, eligibility for ICU care should be determined by objective evidence of likelihood of survival. One perspective recommends giving priority to patients with “reasonable life expectancy” [27]. However, all persons living with dementia deserve good general medical care—including, where appropriate, palliative care—even if intensive care, which can be burdensome, is scarce. Guiding principles for triaging persons with dementia state that dementia is a heterogenous condition with a wide range of prognoses and recommends basing decisions on objective evidence of estimated survival rather than speculations about quality of life, which are more subjective and prone to bias [7]. The prognostic models systematically reviewed in this paper have some utility for this triaging application, but also some limitations. The ADEPT model [17] predicts risk of death from dementia in an appropriate time frame for triage decisions but only in persons with very advanced dementia, where functional status alone probably conveys sufficient information for triage. The Swedish Dementia Registry model [18] includes outpatient and milder dementia patients but predicts death over a longer time period (3 years) that may not be as relevant for immediate triage decisions. However, it does clarify that younger patients (age 65 or 75) with dementia without other comorbidities generally have greater than 80%chance of surviving to three years and beyond, such that mortality risk from underlying dementia alone seems insufficient to justify a blanket exclusion from ICU care during the pandemic. What is absent from the published literature are models that predict the risk of death in persons with mild to moderate dementia residing in nursing homes.
In conclusion, this systematic review identified two useful prognostic models for mortality risk in persons with dementia, in nursing home residents with advanced dementia and outpatients with dementia attending primary care or seen in a specialty clinic. However, there remain many questions to be answered by future research. Both models would benefit from further validation, including the first external validation for the latter model in the outpatient setting. There are no validated, applicable, high quality models for patients residing in nursing homes with mild to moderate dementia, which is a common demographic. Models in this population should be derived and validated. For all models, research is needed on how they can be implemented in clinical practice. Patients, and their care partners, deserve individualized prognoses based on high quality, objective evidence. The impact of obtaining this information—on their knowledge, personal choices, clinical care, and quality of life—should be investigated.
-4pt
DISCLOSURE STATEMENT
Authors’ disclosures available online (https://www.j-alz.com/manuscript-disclosures/20-1364r1).
SUPPLEMENTARY MATERIAL
[1] The supplementary material is available in the electronic version of this article: https://dx.doi.org/10.3233/JAD-201364.
REFERENCES
[1] | Todd S , Barr S , Roberts M , Passmore AP ((2013) ) Survival in dementia and predictors of mortality: A review. Int J Geriatr Psychiatry 28: , 1109–1124. |
[2] | Brown MA , Sampson EL , Jones L , Barron AM ((2013) ) Prognostic indicators of 6-month mortality in elderly people with advanced dementia: A systematic review. Palliat Med 27: , 389–400. |
[3] | Truog RD , Mitchell C , Daley GQ ((2020) ) The toughest triage - allocating ventilators in a pandemic. N Engl J Med 382: , 1973–1975. |
[4] | Moons KG , de Groot JA , Bouwmeester W , Vergouwe Y , Mallett S , Altman DG , Reitsma JB , Collins GS ((2014) ) Critical appraisal and data extraction for systematic reviews of prediction modelling studies: The CHARMS checklist. PLoS Med 11: , e1001744. |
[5] | Wolff RF , Moons KGM , Riley RD , Whiting PF , Westwood M , Collins GS , Reitsma JB , Kleijnen J , Mallett S , Groupdagger P ((2019) ) PROBAST: A tool to assess the risk of bias and applicability of prediction model studies. Ann Intern Med 170: , 51–58. |
[6] | Moons KGM , Wolff RF , Riley RD , Whiting PF , Westwood M , Collins GS , Reitsma JB , Kleijnen J , Mallett S ((2019) ) PROBAST: A tool to assess risk of bias and applicability of prediction model studies: Explanation and elaboration. Ann Intern Med 170: , W1–W33. |
[7] | Smith EE , Couillard P , Fisk JD , Ismail Z , Montero-Odasso M , Robillard JM , Vedel I , Sivananthan S , Gauthier S ((2020) ) Pandemic dementia scarce resource allocation. Can Geriatr J 23: , 216–218. |
[8] | Huyer G , Brown CRL , Spruin S , Hsu AT , Fisher S , Manuel DG , Bronskill SE , Qureshi D , Tanuseputro P ((2020) ) Five-year risk of admission to long-term care home and death for older adults given a new diagnosis of dementia: A population-based retrospective cohort study. CMAJ 192: , E422–E430. |
[9] | Carlson MC , Brandt J , Steele C , Baker A , Stern Y , Lyketsos CG ((2001) ) Predictor index of mortality in dementia patients upon entry into long-term care. J Gerontol A Biol Sci Med Sci 56: , M567–570. |
[10] | Newcomer R , Covinsky KE , Clay T , Yaffe K ((2003) ) Predicting 12-month mortality for persons with dementia. J Gerontol B Psychol Sci Soc Sci 58: , S187–198. |
[11] | Sultana J , Fontana A , Giorgianni F , Basile G , Patorno E , Pilotto A , Molokhia M , Stewart R , Sturkenboom M , Trifiro G ((2018) ) Can information on functional and cognitive status improve short-term mortality risk prediction among community-dwelling older people? A cohort study using a UK primary care database. Clin Epidemiol 10: , 31–39. |
[12] | van de Vorst IE , Goluke NMS , Vaartjes I , Bots ML , Koek HL ((2020) ) A prediction model for one- and three-year mortality in dementia: Results from a nationwide hospital-based cohort of 50,993 patients in the Netherlands. Age Ageing 49: , 361–367. |
[13] | Pilotto A , Sancarlo D , Panza F , Paris F , D’Onofrio G , Cascavilla L , Addante F , Seripa D , Solfrizzi V , Dallapiccola B , Franceschi M , Ferrucci L ((2009) ) The Multidimensional Prognostic Index (MPI), based on a comprehensive geriatric assessment predicts short- and long-term mortality in hospitalized older patients with dementia. J Alzheimers Dis 18: , 191–199. |
[14] | Paradise M , Walker Z , Cooper C , Blizard R , Regan C , Katona C , Livingston G ((2009) ) Prediction of survival in Alzheimer’s disease–the LASER-AD longitudinal study. Int J Geriatr Psychiatry 24: , 739–747. |
[15] | Stern Y , Tang MX , Albert MS , Brandt J , Jacobs DM , Bell K , Marder K , Sano M , Devanand D , Albert SM , Bylsma F , Tsai WY ((1997) ) Predicting time to nursing home care and death in individuals with Alzheimer disease. JAMA 277: , 806–812. |
[16] | Wang L , Sha L , Lakin JR , Bynum J , Bates DW , Hong P , Zhou L ((2019) ) Development and validation of a deep learning algorithm for mortality prediction in selecting patients with dementia for earlier palliative care interventions. JAMA Netw Open 2: , e196972. |
[17] | Mitchell SL , Miller SC , Teno JM , Davis RB , Shaffer ML ((2010) ) The advanced dementia prognostic tool: A risk score to estimate survival in nursing home residents with advanced dementia. J Pain Symptom Manage 40: , 639–651. |
[18] | Haaksma ML , Eriksdotter M , Rizzuto D , Leoutsakos JS , Olde Rikkert MGM , Melis RJF , Garcia-Ptacek S ((2020) ) Survival time tool to guide care planning in people with dementia. Neurology 94: , e538–e548. |
[19] | Cheng CM , Chang WH , Chiu YC , Chen MH , Liao WT , Yang CH , Hwang JP , Li CT , Bai YM , Tsai SJ , Chen TJ , Tsai CF ((2019) ) Risk score for predicting mortality in people with dementia: A nationwide, population-based cohort study in Taiwan with 11 years of follow-up. J Clin Psychiatry 80: , 18m12629. |
[20] | Haaksma ML , Rizzuto D , Ramakers I , Garcia-Ptacek S , Marengoni A , van der Flier WM , Verhey FRJ , Olde Rikkert MGM , Melis RJF ((2019) ) The impact of frailty and comorbidity on institutionalization and mortality in persons with dementia: A prospective cohort study. J Am Med Dir Assoc 20: , 165–170 e162. |
[21] | Van Dijk PT , Dippel DW , Van Der Meulen JH , Habbema JD ((1996) ) Comorbidity and its effect on mortality in nursing home patients with dementia. J Nerv Ment Dis 184: , 180–187. |
[22] | Mitchell SL , Kiely DK , Hamel MB , Park PS , Morris JN , Fries BE ((2004) ) Estimating prognosis for nursing home residents with advanced dementia. JAMA 291: , 2734–2740. |
[23] | Wang Y , Huang Y , Liu Z , Zhuo C , Li S , Prince M ((2010) ) A five-year community-based longitudinal survival study of dementia in Beijing, China: A 10/66 Dementia Research Group population-based study.. Int Psychogeriatr 22: , 761–768. |
[24] | van der Steen JT , Mitchell SL , Frijters DH , Kruse RL , Ribbe MW ((2007) ) Prediction of 6-month mortality in nursing home residents with advanced dementia: Validity of a risk score. J Am Med Dir Assoc 8: , 464–468. |
[25] | Mitchell SL , Miller SC , Teno JM , Kiely DK , Davis RB , Shaffer ML ((2010) ) Prediction of 6-month survival of nursing home residents with advanced dementia using ADEPT vs hospice eligibility guidelines. JAMA 304: , 1929–1935. |
[26] | Morris JN , Fries BE , Mehr DR , Hawes C , Phillips C , Mor V , Lipsitz LA ((1994) ) MDS Cognitive Performance Scale. J Gerontol 49: , M174–182. |
[27] | Emanuel EJ , Persad G , Upshur R , Thome B , Parker M , Glickman A , Zhang C , Boyle C , Smith M , Phillips JP ((2020) ) Fair allocation of scarce medical resources in the time of Covid-19. N Engl J Med 382: , 2049–2055. |
[28] | Delva F , Pimouguet C , Helmer C , Peres K , Brechat B , Le Goff M , Jacqmin-Gadda H , Amieva H , Orgogozo JM , Auriacombe S , Berr C , Tzourio C , Barberger-Gateau P , Dartigues JF ((2013) ) A simple score to predict survival with dementia in the general population. Neuroepidemiology 41: , 20–28. |