Plasma Neurofilament Light: A Marker of Neurodegeneration in Mild Behavioral Impairment
Abstract
Background:
Assessing neuropsychiatric symptoms (NPS) in older adults is important for determining dementia risk. Mild behavioral impairment (MBI) is an at-risk state for cognitive decline and dementia, characterized by emergent NPS in later life. MBI has significantly higher dementia incidence than late life psychiatric conditions. However, its utility as a proxy for neurodegeneration has not been demonstrated. Plasma neurofilament light (NfL) is a validated biomarker of axonal damage, and has been shown to associate with hallmarks of neurodegeneration.
Objective:
The purpose of this investigation was to identify associations between NfL rate of change and the presence of MBI symptomatology.
Methods:
We evaluated the association of MBI with changes in NfL in a cohort (n = 584; MBI + n = 190, MBI– n = 394) of non-demented participants from the Alzheimer’s Disease Neuroimaging Initiative. MBI was determined by transforming Neuropsychiatric Inventory Questionnaire items using a published algorithm. Change in NfL was calculated over 2 years.
Results:
Time*MBI status was the only significant interaction to predict change in NfL concentrations (F(1,574) = 4.59, p = 0.032), even after controlling for age, mild cognitive impairment, and demographics. Analyses reclassifying 64 participants with new onset MBI over 2 years similarly demonstrated greater increases in NfL (F(1,574) = 5.82, p = 0.016).
Conclusion:
These findings suggest MBI is a clinical proxy of early phase neurodegeneration with putative utility in identifying those at dementia risk. MBI can be used as a case ascertainment approach to capture those at high risk for cognitive decline and dementia, and is an important construct for clinicians dealing with cognitive and neuropsychiatric symptomatology in older adults.
INTRODUCTION
Alzheimer’s disease (AD) is the most widespread form of dementia. It is a neurodegenerative disease characterized by progressive cognitive impairment and functional decline, as well as neuropsychiatric symptoms (NPS) [1]. Worldwide, approximately 47 million individuals are living with dementia with an expected 131 million projected by 2050 [2]. Functional connectivity changes and dementia proteinopathies can occur 20 years in advance of memory symptoms [3]; however, AD clinical trials have been unsuccessful in finding a disease-modifying drug, with poor recruitment and retention of participants in the early phase of disease being cited as a primary reason [4]. The initial patient with dementia described by Alois Alzheimer over 100 years ago, Auguste D, presented to hospital not with cognitive symptoms, but with NPS of emotional dysregulation and suspiciousness, followed by cognitive symptoms. Yet, this message has been lost over time, with the embrace of a cognocentric model of dementia. Incorporating a systematic approach to case ascertainment using NPS may improve clinical trial design [5], and offer more insight into the nature of later life psychiatric symptoms for appropriate risk assessment and treatment.
Although NPS have traditionally been underappreciated in advance of dementia, emerging research has consistently demonstrated the relevance of NPS for predicting future cognitive decline in pre-dementia populations [6–9]. Mild behavioral impairment (MBI) is a validated neurobehavioral syndrome that describes later-life onset of sustained NPS as an at-risk state for incident cognitive decline and dementia [10–12]. MBI may even be the earliest manifestation of a neurodegenerative disease in some, emerging before cognitive decline. Within the MBI diagnostic framework [10], relevant NPS are categorized into five domains, namely: decreased drive and motivation (apathy) [13], emotional dysregulation (mood and anxiety symptoms) [14], impulse dyscontrol (agitation, aggression, impulsivity, abnormal reinforcement, and reward) [15], social inappropriateness (impaired social cognition) [16], and abnormal perception or thought content (psychotic symptoms, i.e., hallucinations and delusions) [17], which are examined independently and collectively for risk assessment. MBI is relatively simple to capture using a brief informant rated scale for NPS [18]. To determine the biological correlates of this clinical syndrome, additional biomarker identification of MBI is required. Specifically, there is a need to identify minimally-invasive biomarkers that can validate MBI as a way to capture the disease early, and track progression and neurodegeneration. Cerebrospinal fluid (CSF) biomarkers, positron emission tomography (PET), and detailed neuropsychological testing are scientifically valid approaches to early detection, but are not feasible at a population level. Straightforward cost-effective approaches are urgently needed [5]. Recent investigations have revealed blood-based biomarkers as a potential target to fill this void [19–22].
Neurofilament light (NfL) is an axoskeletal protein that is involved in maintaining the shape and structure of neurons [23]. NfL is highly expressed in large-caliber myelinated axons and is released into the brain interstitial fluid, following axonal injury [24]. Historically obtained from CSF, NfL has recently been identified as a biomarker of neurodegeneration that can be obtained minimally-invasively from plasma samples [19, 25]. In a recently published study, plasma NfL measured using the ultra-sensitive single molecule array (Simoa) assay [26] was particularly high in patients with mild cognitive impairment (MCI) and patients with AD dementia with Aβ pathologic features. Additionally, high plasma NfL correlated with poor cognition and AD-related atrophy (baseline and longitudinally) and brain hypometabolism (longitudinally) [19]. The objective of this study was to determine the association of baseline MBI symptoms and plasma NfL change over a period of two years. Our cohort consisted of pre-dementia participants from the Alzheimer’s Disease Neuroimaging Initiative (ADNI). We hypothesized that non-demented participants with MBI symptomatology at baseline (MBI+) would have increased rates of NfL change, reflecting faster NfL accumulation due to neurodegeneration, when compared to those without baseline MBI symptomatology (MBI–).
MATERIALS AND METHODS
Alzheimer’s disease neuroimaging initiative participants
The ADNI is a public-private partnership launched in 2003 with the goal of testing whether biological markers, clinical, and neuropsychological assessments can be combined to monitor progression of MCI and early AD. Data from ADNI1, ADNIGO, and ADNI2 cohorts were used in this study. Quantified NfL data, diagnostic status, demographic information, and Neuropsychiatric Inventory Questionnaire (NPI-Q) scores were extracted for 584 pre-dementia participants.
Participants were between 55 and 93 years of age at their first NfL visit, English or Spanish speakers, and consented to their data being included in the study. Cognitive classification into MCI or normal cognition (NC) was based upon the ADNI criteria (found at http://adni.loni.usc.edu/wp-content/uploads/2010/09/ADNI_GeneralProceduresManual.pdf).
MBI status
The MBI checklist (MBI– C) is the case ascertainment instrument developed to capture MBI [18]. However, it is a relatively new scale not yet incorporated into ADNI. Thus, MBI status was approximated by a transformation of NPI-Q scores using a published algorithm [27]. Ten NPS domains from the NPI-Q were used to operationalize the five ISTAART-AA MBI domains of decreased motivation (NPI-Q apathy/indifference); emotional/ affective dysregulation (NPI-Q depression/dysphoria, anxiety, elation/euphoria); impulse dyscontrol (NPI-Q agitation/aggression, irritability/lability, aberrant motor behavior); social inappropriateness (NPI-Q disinhibition); and abnormal perception or through content (NPI-Q delusions, hallucinations). To obtain the MBI total score, these five transformed domains were added together.
NPI-Q scores obtained within six months from the first available NfL reading were used in this approximation. An MBI score was calculated from the sum of the five transformed MBI domains. Participants with an MBI total score >1 were considered MBI+ and participants with an MBI total score of zero were considered MBI–. Participants with a baseline MBI total score of 1 were excluded from the study due to diagnostic uncertainty. Figure 1 outlines how the sample population was obtained from the ADNI longitudinal plasma NfL dataset. Participants were excluded from this analysis if: 1) the NPI-Q was not administered within six months of the baseline NfL value; 2) an NfL value was not available two years after the first visit, and; 3) there was no baseline cognitive status determined at the NPI-Q visit.
Fig.1
Flowchart depicting how the sample population was obtained from the ADNI database, stratified by MBI status at baseline. Those with a transformed MBI score of greater than 1 were considered MBI+, whereas those with an MBI score of 0 were considered MBI–. 1Participant identified as having a suspected NfL data entry error at the initial visit was removed (n = 1). 2Participants with AD at baseline were removed (n = 230). 3Participants who had an MBI score of 1 (n = 99) were removed.
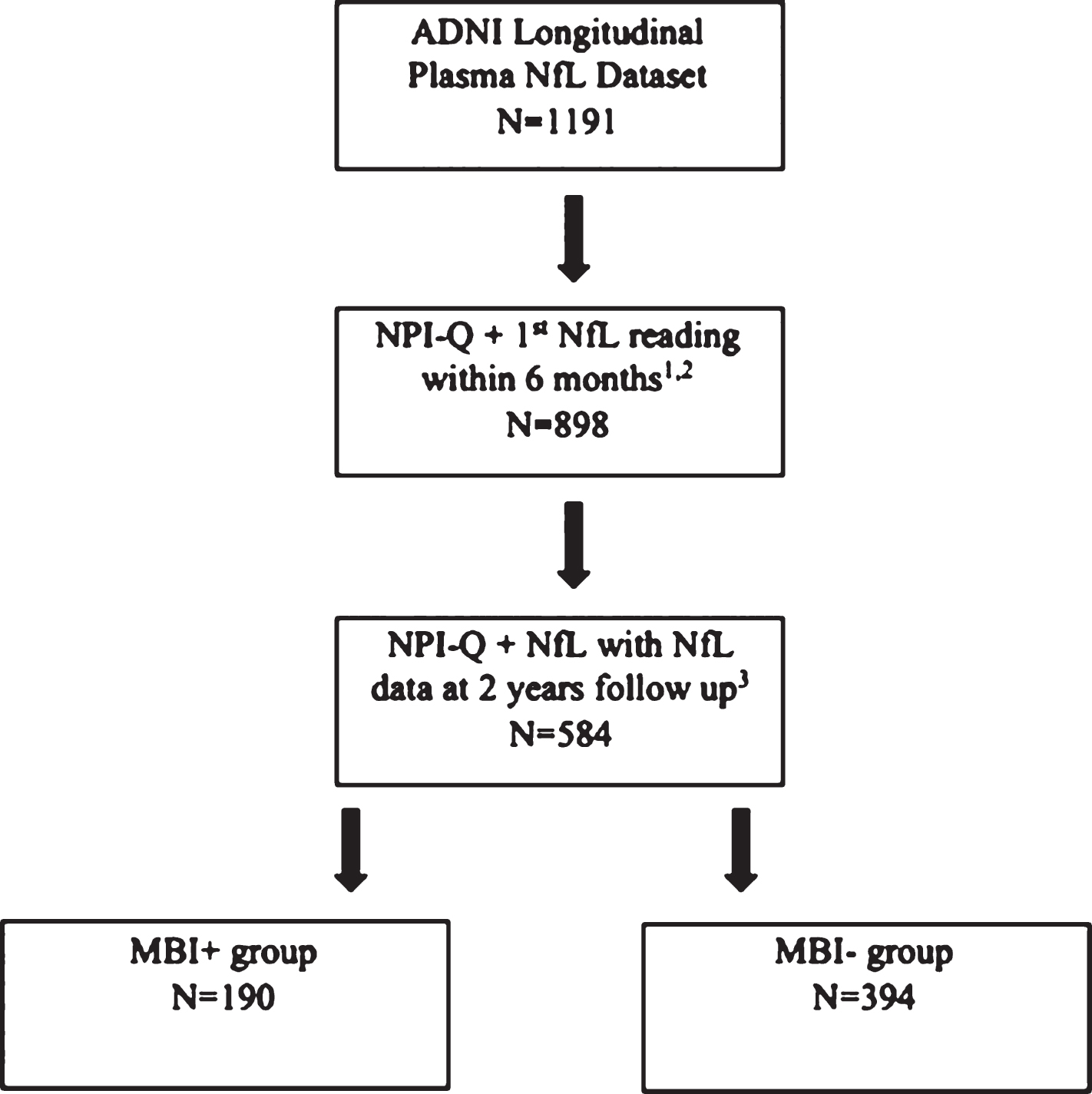
NfL quantification
One board certified laboratory technician conducted ultrasensitive enzyme linked immunosorbent assays on a single molecule array (SIMOA) platform at the University of Gothenburg, Sweden, to quantify NfL levels [19]. Further details of the procedure can be found at http://adni.loni.usc.edu.
Statistical analysis
All statistical analyses were carried out in SPSS v24. Baseline continuous variables were analyzed with Student’s t-test or Mann-Whitney U test and dichotomous variables were analyzed with chi-square tests. We utilized repeated measures ANOVA to determine whether the change in NfL concentration over two years was significantly different between MBI+ and MBI– subjects, and then repeated the analyses including statistically significant clinical and demographic differences between MBI+ and MBI– groups as covariates in the model (specifically, age, sex, education, and MCI status). Huynh-Feldt correction was applied. Alpha was set at <0.05.
Data availability
Data used in this study is available from ADNI.
RESULTS
Demographic and clinical variables are detailed in Table 1. The MBI+ group was significantly younger, had fewer years of education, more males, and was more likely to have a diagnosis of MCI at baseline than the MBI– group.
The repeated measures ANOVA without covariates identified statistically significant contributions from Time (F(1,582) = 29.66, p < 0.001) and Time*MBI status (F(1,582) = 5.37, p = 0.021). A full-factorial repeated measures ANOVA was then performed including significant demographic and clinical differences as covariates. The results of these analyses are detailed in Table 2 and Fig. 2A. Main effects for age and MCI were observed, with no other main effects. Time*MBI status was the only significant interaction associated with change in NfL concentrations (F(1,574 = 4.59, p = 0.032) at 2 years. This corresponds to an average increase in NfL of 2.42±16.16 ng/L in the MBI– group and 6.01±20.12ng/L in the MBI+ group.
Table 1
Baseline demographic information stratified by MBI status at baseline (N = 584)
Item | MBI– (n = 394) | MBI+ (n = 190) | Test Statistic | p |
Mean ± SD or n (%) | Mean ± SD or n (%) | |||
Age | 74.39±7.37 | 72.93±7.37 | t(582) = 2.25 | 0.025 |
Female, N (%) | 199 (50.5) | 70 (36.8) | χ2 = 9.63 | 0.002 |
Years of education | 16.56±2.70 | 16.03±2.65 | t(582) = 2.23 | 0.026 |
Mild cognitive impairment | 174 (44.2) | 156 (82.1) | χ2 = 75.08 | <0.001 |
NfL concentration (ng/L) | 39.05±22.38 | 36.43±18.25 | t(582) = 1.40 | 0.161 |
Table 2
Multivariate repeated measures ANOVA model results with baseline definition of MBI
Model term | F(1,574) | p | Partial Eta Squared |
Between-Subjects Main Effects | |||
Age | 212.75 | <0.001 | 0.103 |
Sex | 1.11 | 0.890 | 0.002 |
Education | 0.39 | 0.530 | 0.001 |
MBI– Baseline | 0.19 | 0.890 | 0.000 |
MCI | 10.11 | 0.002 | 0.017 |
Within-Subjects Contrasts | |||
Time | 0.004 | 0.951 | 0.000 |
Time*Age | 1.52 | 0.217 | 0.003 |
Time*Sex | 0.20 | 0.653 | 0.000 |
Time*Education | 0.86 | 0.352 | 0.002 |
Time*MBI– Baseline | 4.59 | 0.032 | 0.008 |
Time*MCI | 0.00 | 0.958 | 0.000 |
Time*MBI– Baseline*Sex | 0.26 | 0.610 | 0.000 |
Time*MBI– Baseline*MCI | 0.09 | 0.762 | 0.000 |
Time*MCI*Sex | 1.66 | 0.198 | 0.000 |
Time*Sex*MBI– Baseline*MCI | 0.46 | 0.494 | 0.001 |
Between subjects parameters included: MBI, MCI, and sex. Covariates included: age and years of education.
Fig.2
Estimated marginal NfL concentration means from the full factorial model including covariates by MBI status. Error bars indicate ± standard error of the mean. Figure 2a is stratified by MBI status at baseline, while Fig. 2b combines participants with emergent MBI + during the follow-up period with the baseline MBI positive participants.
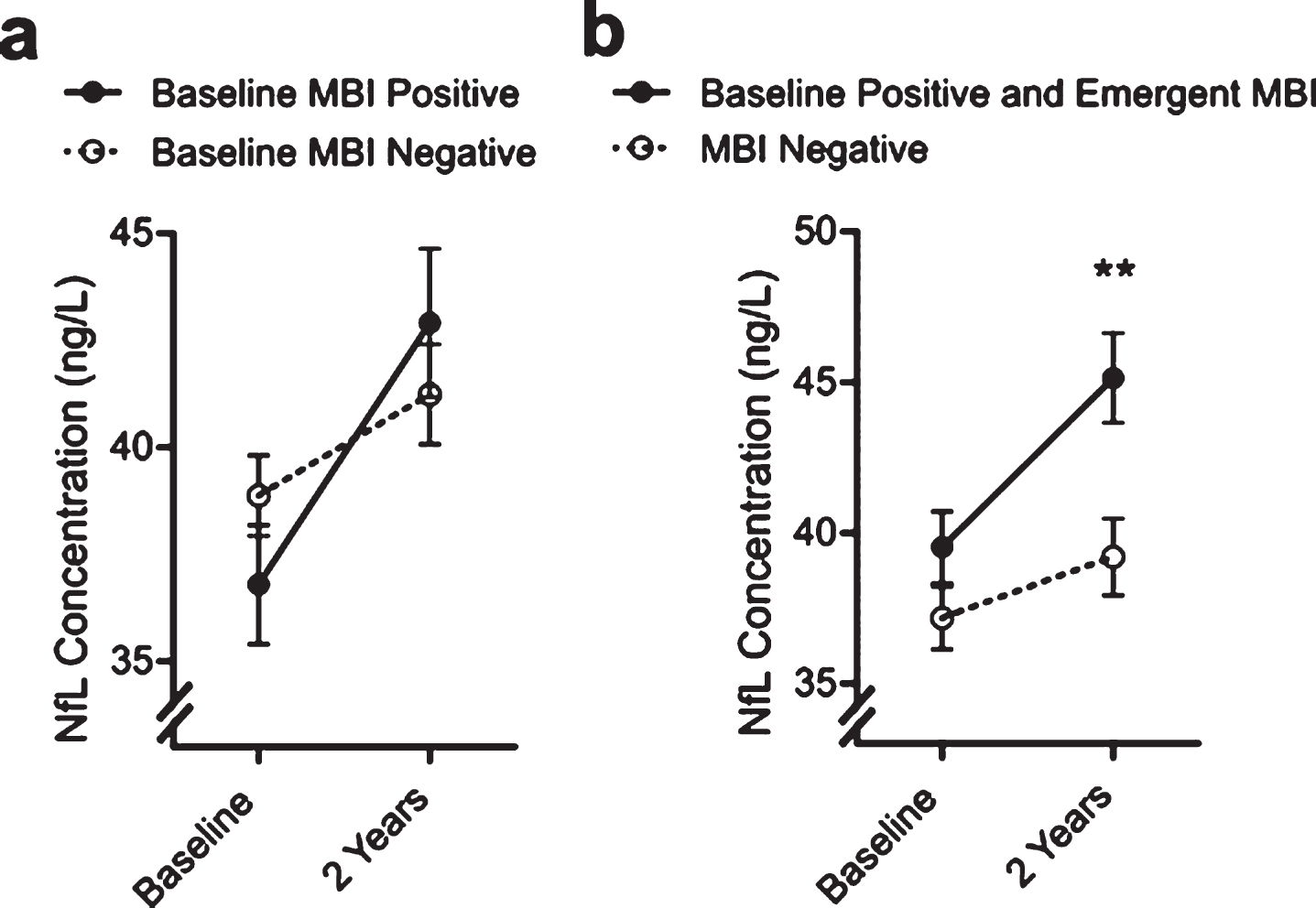
Over the 2-year follow-up period, an additional 64 participants who were classified as MBI– at baseline went on to develop emergent MBI symptomology (MBI score ≥ 1). Compared to individuals who were MBI– and did not develop NPS over the follow-up period, these individuals had a significantly higher NfL concentration at baseline (42.74 ± 26.30 versus 37.92 ± 20.28; Mann-Whitney U = 2.23, p = 0.025). We pooled the baseline MBI+ and emergent MBI cases into a single group and repeated the full-factorial model (see Table 3 and Fig. 2B). This identified a statistically significant contribution by MBI(Baseline + Emergent) status (F(1,574) = 4.83, p = 0.028), Time (F(1,582 = 27.24, p <0.001) Time*MBI(Baseline + Emergent) status (F(1,574) = 5.82, p = 0.016), and Time*MBI(Baseline + Emergent)*Sex (F(1,574) = 4.34, p = 0.038). Tukey’s post-hoc tests revealed separation between MBI(Baseline + Emergent) and MBI negative participants at 2 years, and while the effect of sex did not reach significance the effect appeared to be driven by increases in NfL in females.
Table 3
Multivariate Repeated Measures ANOVA Model Results with Baseline and Emergent Definition of MBI
Model term | F(1,574) | p | Partial Eta Squared |
Between-Subjects Main Effects | |||
Age | 213.62 | <0.001 | 0.271 |
Sex | 2.07 | 0.150 | 0.004 |
Education | 1.12 | 0.290 | 0.002 |
MBI– Baseline & Emergent | 4.83 | 0.028 | 0.008 |
MCI | 6.36 | 0.012 | 0.011 |
Within-Subjects Contrasts | |||
Time | 0.002 | 0.968 | 0.000 |
Time*Age | 1.46 | 0.227 | 0.003 |
Time*Sex | 0.07 | 0.779 | 0.000 |
Time*Education | 0.98 | 0.322 | 0.002 |
Time*MBI– Baseline & Emergent | 5.82 | 0.016 | 0.010 |
Time*MCI | 0.30 | 0.862 | 0.000 |
Time*MBI– Baseline & Emergent*Sex | 4.34 | 0.038 | 0.008 |
Time*MBI– Baseline*MCI | 0.04 | 0.828 | 0.000 |
Time*MCI*Sex | 0.36 | 0.545 | 0.001 |
Time*Sex* MBI– Baseline & Emergent*MCI | 0.009 | 0.923 | 0.000 |
Between subjects parameters included: MBI, MCI, and sex. Covariates included: age and years of education.
DISCUSSION
To the best of our knowledge, the relationship between NfL and MBI has never been longitudinally characterized in a pre-dementia sample. Our data support the hypothesis that MBI is a valid risk marker for neurodegeneration, as indicated by greater increase in NfL over two years in individuals with MBI. By studying participants with normal cognition and MCI, this study was able to increase our understanding of the development of MCI and dementia, which will be extremely valuable in enhancing early detection.
MBI represents the neurobehavioral axis of pre-dementia risk states, as a complement to the neurocognitive axis represented by subjective cognitive decline (SCD) and MCI. Thus far, clinical and imaging/biomarker studies have validated MBI as a risk state and marker of early disease. Cross-sectional studies have associated MBI with a specific cognitive profile in pre-dementia [28] and Parkinson’s disease [29], and longitudinal data have demonstrated faster cognitive decline in the presence of MBI [12, 28, 30, 31]. Neurobiological correlates of MBI are also emerging. First genetic studies suggest an association of MBI with AD risk genes [32]. Neural correlates of MBI impulse dyscontrol have been associated with micro-structural changes using diffusion tensor MRI [33]. Using the National Institute of Aging-Alzheimer’s Association Amyloid Tau Neurodegeneration (ATN) research framework [34], MBI has been validated as a preclinical dementia syndrome, associated with amyloid positivity, in advance of tau and neurodegeneration in older adults with normal cognition, irrespective of the presence or absence of subjective cognitive complaints [35].
NfL has been validated as a biomarker of neurodegeneration, and change in NfL is associated with other well-established correlates of AD [19]. Indeed, NfL change is associated with low fluorodeoxyglucose PET uptake, expansion of ventricular volume, and reduction in cognitive test scores. Additionally, in the MCI population, a change in NfL has been associated with white matter lesions and CSF p/t-tau, which was not observed in cognitively normal and AD groups. Another very recent study from the Mayo Clinic Study of Aging compared CSF and plasma NfL in a sample of 79 participants with disease severity not exceeding an MCI state. No cross-sectional associations between NfL and imaging or cognitive markers were found, but higher baseline plasma and CSF NfL levels were longitudinally associated with worsened outcomes for global cognition and most neuroimaging measures of neurodegeneration [36]. Relevant to the importance of appropriate assessment of later life psychiatric symptoms, two recent studies have assessed differences in serum NfL in behavioral variant FTD (bvFTD) and primary psychiatric conditions (PPC). A German study assessed cross-sectional differences in serum NfL levels between bvFTD and PPC (including depression, bipolar disorder, and schizophrenia). In this small-sample study, no significant changes were observed when comparing psychiatric patients with the control group, but elevated Simoa serum NfL levels (>23.7 pg/ml) in bvFTD had 85% sensitivity and 78% specificity in distinguishing of bvFTD from all psychiatric disorders as a combined group [37]. Similarly, a Finnish study comparing 91 participants with FTD and 34 with PPC, demonstrated discriminative utility of the Simoa assay with 79% sensitivity and 85% specificity (AUC = 0.830) [38]. Cross sectional studies have also suggested large-scale axonal degradation to occur in pre-disease states through investigation of plasma and CSF NfL [23, 39].
Our data extend this body of literature suggesting that NfL is sensitive to domains beyond cognition, to include NPS, which are also associated with neurodegeneration. As these can be among the first noticeable indicators of an impending neurodegenerative disorder, our findings suggest that the NPS clinical phenotype described by MBI constitutes a subtype at risk of accelerated disease progression, captured by NfL rate of change. Using NfL, early disease sensitivity has been suggested by other studies that identified elevated serum, plasma, and CSF levels prior to cognitive symptom onset [39, 40]. Combined, the behavioral aspect communicated by MBI and biological validation provided by NfL could produce a sensitive method to track early phase disease.
Neither age, gender, education, nor baseline cognitive status (NC versus MCI) were significantly associated with the NfL rate of change in this dataset. Consistent with the literature [19], when stratified based on cognitive status alone (MCI versus NC), there were also no significant differences between the two groupings in NfL rate of change. This highlights the sensitivity that MBI provides in identifying non-demented populations at risk of cognitive decline, irrespective of their cognitive status (normal cognition, subjective cognitive decline, MCI). It also helps to confirm the validity of MBI as a marker of not only impending cognitive decline, but of neurodegeneration as well, reinforcing the interpretation that late life NPS are a feature of neurodegeneration. Indeed, the later in life the onset of psychiatric symptomatology, the more likely it is a manifestation of early neurodegenerative disease [14]. This is one of the core constructs of the MBI syndrome, which mandates symptoms be emergent in later life. Additional biological studies also support the relationship between dementia proteinopathies and NPS. Striatal amyloid binding has been associated with anxiety [41], and the early neurodegeneration-associated loss of biogenic amine nuclei can potentially manifest as psychiatric symptomatology [42]. More recently, longitudinal data have demonstrated that cortical amyloid moderates the association between worsening depressive symptoms and declining cognition in older adults [43]. Our study adds to the evidence base that NPS can be a core symptom of neurodegenerative disease, seen in advance of or in concert with cognitive decline, advancing our biological understanding of neuropsychiatric symptomatology in older adults.
It is unclear at this point what are the exact temporal and neurobiological relationships between plasma NfL accumulation and MBI. In the MBI (emergent + baseline) grouping, there was an elevated rate of NfL accumulation alongside the main effect of MBI that was not present in the MBI (baseline) only group. By observing a global, MBI related elevation in plasma NfL only in the group that included emergent MBI symptomology participants, it can be speculated that the degeneration occurred prior to the onset of MBI symptoms. This is reinforced by the separation in plasma NfL concentrations presented at the two-year follow-up visit. In the MBI (emergent + baseline) group, there was a significantly higher plasma NfL concentration at follow-up than the MBI– grouping, which was not observed when stratifying based only on baseline MBI symptomology. As such, the results of the present study suggest that axonal degradation indicated by NfL accumulation may precede the onset of MBI symptomology in time. We believe these findings with NfL add substantial weight to the notion that MBI has a biological basis and is a manifestation of dementia, in contrast to the reverse causality perspective that NPS may cause cognitive decline and dementia. Alternatively or additionally, there may be a third factor that contributes to both NfL accumulation and MBI symptomology (e.g., dementia proteinopathies). Further studies are required to explore these temporal and neurobiological relationships.
Limitations and future directions
There were several limitations of this study. First, ADNI protocol excludes some participants with NPS due to the assumption that these symptoms represent a psychiatric disorder because of greater symptom severity. Without ascertainment of the natural history of symptoms to determine if they are longstanding/recurrent (and less linked to dementia) or new onset (which may be preclinical or prodromal dementia), potential MBI cases may be lost in ADNI. Second, the NPI-Q was developed specifically for a dementia population, and while it has been used extensively in MCI, it is unclear to what extent it captures NPS in older adults with normal cognition, let alone those that are sustained and emergent in accordance with MBI criteria, for which the MBI– C was explicitly developed. MBI criteria require a 6-month symptom duration to meet syndromic threshold, the NPI-Q has a 1-month reference range. Specifically, using the NPI-Q is an imprecise approach to capture MBI, as evidenced by additional preliminary work we have done. In a recent study of cognitive neurology patients, MBI was present in 83.5% of MCI and 76.5% of SCD using this transformation approach [27]. A similar analysis in an Australian population sample of 1,377 participants with normal cognition, pre-MCI and MCI, found MBI prevalence to be 34.1% [44]. In contrast, in a primary care MBI– C validation study, prevalence was 5.8% in SCD [45] and 14.2% in MCI [46]. Prevalence estimates of MBI using the NPI-Q have been inflated compared to those with the MBI– C due to decreased specificity associated with transient and reactive symptoms captured by a scale with a short reference range [27, 44–46]. To mitigate this loss of specificity, only those with a total MBI score greater than 1 were classified as MBI+, and those with an MBI score of 1 were excluded. While this approach may increase specificity, it may falsely categorize some participants as MBI–. However, including only those with greater severity to compensate for a shorter duration may still not accurately capture the true MBI signal which is driven by later life onset of sustained NPS.
Notwithstanding these limitations, using the approach of transforming NPI-Q items to MBI domains has shown to be effective in other studies. Using the National Alzheimer Coordinating Center dataset, transformed NPI-Q scores of 2769 NC participants were used to determine MBI status. In this study, MBI was associated with greater risk of incident cognitive decline and dementia at 3 years compared to those without MBI [31]. In another ADNI study of 341 non-demented individuals, machine learning models demonstrated that MBI score (derived from transformed NPI-Q scores) was as effective as hippocampal volume in predicting dementia diagnosis at 40 months [47]. Similarly, ADNI transformed NPI-Q scores were used to determine the neural correlates of MBI impulse dyscontrol across the cognitive spectrum, from normal cognition through MCI and dementia [33]. These findings support use of the NPI-Q transformation algorithm here, as preliminary foundational work linking MBI and known dementia markers. We believe this is a very good starting point, and a complement to recent ligand-based imaging evidence that MBI is associated with amyloid positivity in cognitive normals [35], and tau positivity in MCI [48]. Overall, the field requires a systematic approach to exploring the link between MBI and dementia biomarkers, and our study is but one step (but the first with NfL to our knowledge), which needs to be replicated in other datasets.
Future studies should also utilize the validated MBI– C [45, 46, 49, 50] to determine MBI status. Use of the MBI– C would also allow appropriate examination in pre-dementia populations of MBI domain specific associations with NfL. The MBI– C is being incorporated into some cohorts (COMPASS-ND Canada [51], PROTECT- UK [12], CatchCog – Netherlands [52], BHR – USA [53], CobTek – France [54], Czech Brain Aging Study-Czech Republic [55], Swedish BioFINDER2 [56]), although data will only emerge slowly. In the meantime, our best approach to explore the association of pre-dementia NPS and dementia biomarkers is to use existing datasets to determine if there are signals, which can be explored further. Additionally, the nascent literature in this field has focused on MBI status in general and has not yet determined rates of cognitive decline for different MBI domains, which are next steps in this rapidly evolving field.
Conclusions
This study provides validation of MBI as a marker of early phase neurodegenerative disease, associated with faster disease progression, as measured by NfL. The novelty of this study lies in the examination of a rate of NfL change in relation to MBI symptomatology, adding to the evidence that NPS may be a sentinel sign of early dementia and accelerated neurodegeneration [35]. MBI is a novel approach to dementia detection using an informant rated measurement of NPS that is non-invasive, scalable, and inexpensive. Those screening positive for MBI can be investigated further clinically and considered in primary and secondary prevention clinical trials. Given that MBI is associated with faster cognitive decline and reduced time to dementia, incorporating MBI measurement into clinical trial screening protocols can serve as an efficient, inexpensive, and scalable way to identify those who are at risk for dementia and may have early dementia changes. This approach can address clinical trial issues of high costs, and prohibitive lead-time to demonstrate clinical benefit, increasing power of trials and decreasing the cost due to more efficient patient selection. Additionally, as MBI can be among the first noticeable indicators of an impending neurodegenerative disorder, our findings may suggest that the NPS clinical phenotype described by MBI constitutes a subtype at risk of accelerated disease progression, captured by NfL rate of change. The construct of MBI has immediate impact on the early identification of potential neurodegenerative disease because of ease of administration and cost-effectiveness. MBI also has immediate impact in psychiatric clinical care, identifying those at higher risk for dementia in primary and specialty care clinics due to the emphasis on natural history of psychiatric symptoms, differentiating between chronic/recurrent versus later life emergent symptomatology. These findings will enable assessment of new therapies earlier in the disease course, and determine if treatment can change the course of disease [5].
ACKNOWLEDGMENTS
Data collection and sharing for this project was funded by the Alzheimer’s Disease Neuroimaging Initiative (ADNI) (National Institutes of Health Grant U01 AG024904) and DOD ADNI (Department of Defense award number W81XWH-12-2-0012). ADNI is funded by the National Institute on Aging, the National Institute of Biomedical Imaging and Bioengineering, and through generous contributions from the following: AbbVie, Alzheimer’s Association; Alzheimer’s Drug Discovery Foundation; Araclon Biotech; BioClinica, Inc.; Biogen; Bristol-Myers Squibb Company; CereSpir, Inc.; Cogstate; Eisai Inc.; Elan Pharmaceuticals, Inc.; Eli Lilly and Company; EuroImmun; F. Hoffmann-La Roche Ltd and its affiliated company Genentech, Inc.; Fujirebio; GE Healthcare; IXICO Ltd.; Janssen Alzheimer Immunotherapy Research & Development, LLC.; Johnson & Johnson Pharmaceutical Research & Development LLC.; Lumosity; Lundbeck; Merck & Co., Inc.; Meso Scale Diagnostics, LLC.; NeuroRx Research; Neurotrack Technologies; Novartis Pharmaceuticals Corporation; Pfizer Inc.; Piramal Imaging; Servier; Takeda Pharmaceutical Company; and Transition Therapeutics. The Canadian Institutes of Health Research is providing funds to support ADNI clinical sites in Canada. Private sector contributions are facilitated by the Foundation for the National Institutes of Health (http://www.fnih.org). The grantee organization is the Northern California Institute for Research and Education, and the study is coordinated by the Alzheimer’s Therapeutic Research Institute at the University of Southern California. ADNI data are disseminated by the Laboratory for Neuro Imaging at the University of Southern California.
This research was funded by the Alzheimer’s Society of Calgary via the Hotchkiss Brain Institute, and the Alberta Innovates Summer Research Studentship award.
Authors’ disclosures available online (https://www.j-alz.com/manuscript-disclosures/20-0011r2).
REFERENCES
[1] | McKhann GM , Knopman DS , Chertkow H , Hyman BT , Jack CR Jr , Kawas CH , Klunk WE , Koroshetz WJ , Manly JJ , Mayeux R ((2011) ) The diagnosis of dementia due to Alzheimer’s disease: recommendations from the National Institute on Aging-Alzheimer’s Association workgroups on diagnostic guidelines for Alzheimer’s disease. Alzheimers Dement 7: , 263–269. |
[2] | Ponjoan A , Garre-Olmo J , Blanch J , Fages E , Alves-Cabratosa L , Martí-Lluch R , Comas-Cufí M , Parramon D , Garcia-Gil M , Ramos R ((2019) ) Epidemiology of dementia: prevalence and incidence estimates using validated electronic health records from primary care. Clin Epidemiol 11: , 217–228. |
[3] | Reiman EM , Quiroz YT , Fleisher AS , Chen K , Velez-Pardo C , Jimenez-Del-Rio M , Fagan AM , Shah AR , Alvarez S , Arbelaez A , Giraldo M , Acosta-Baena N , Sperling RA , Dickerson B , Stern CE , Tirado V , Munoz C , Reiman RA , Huentelman MJ , Alexander GE , Langbaum JB , Kosik KS , Tariot PN , Lopera F ((2012) ) Brain imaging and fluid biomarker analysis in young adults at genetic risk for autosomal dominant Alzheimer’s disease in the presenilin 1 E280A kindred: a case-control study. Lancet Neurol 11: , 1048–1056. |
[4] | Gauthier S , Albert M , Fox N , Goedert M , Kiyipelto M , Mestre-Ferrandiz J , Middleton LT ((2016) ) Why has therapy development for dementia failed in the last two decades? Alzheimers Dement 12: , 60–64. |
[5] | Mortby ME , Black SE , Gauthier S , Miller DS , Porsteinsson A , Smith EE , Ismail Z ((2018) ) Dementia clinical trial implications of mild behavioral impairment. Int Psychogeriatr 30: , 171–175. |
[6] | Geda YE , Roberts RO , Mielke MM , Knopman DS , Christianson TJ , Pankratz VS , Boeve BF , Sochor O , Tangalos EG , Petersen RC , Rocca WA ((2014) ) Baseline neuropsychiatric symptoms and the risk of incident mild cognitive impairment: A population-based study. Am J Psychiatry 171: , 572–581. |
[7] | Rosenberg PB , Mielke MM , Appleby BS , Oh ES , Geda YE , Lyketsos CG ((2013) ) The association of neuropsychiatric symptoms in MCI with incident dementia and Alzheimer disease. Am J Geriatr Psychiatry 21: , 685–695. |
[8] | Masters MC , Morris JC , Roe CM ((2015) ) “Noncognitive” symptoms of early Alzheimer disease: a longitudinal analysis. Neurology 84: , 617–622. |
[9] | Wise EA , Rosenberg PB , Lyketsos CG , Leoutsakos JM ((2019) ) Time course of neuropsychiatric symptoms and cognitive diagnosis in National Alzheimer’s coordinating centers volunteers. Alzheimers Dement (Amst) 11: , 333–339. |
[10] | Ismail Z , Smith EE , Geda Y , Sultzer D , Brodaty H , Smith G , Agüera-Ortiz L , Sweet R , Miller D , Lyketsos CG ((2016) ) Neuropsychiatric symptoms as early manifestations of emergent dementia: provisional diagnostic criteria for mild behavioral impairment. Alzheimers Dement 12: , 195–202. |
[11] | Taragano FE , Allegri RF , Heisecke SL , Martelli MI , Feldman ML , Sánchez V , García VA , Tufro G , Castro DM , Leguizamón PP ((2018) ) Risk of conversion to dementia in a mild behavioral impairment group compared to a psychiatric group and to a mild cognitive impairment group. J Alzheimers Dis 62: , 227–238. |
[12] | Creese B , Brooker H , Ismail Z , Wesnes KA , Hampshire A , Khan Z , Megalogeni M , Corbett A , Aarsland D , Ballard C ((2019) ) Mild behavioral impairment as a marker of cognitive decline in cognitively normal older adults. Am J Geriatr Psychiatry 27: , 823–834. |
[13] | Sherman C , Liu CS , Herrmann N , Lanctot KL ((2018) ) Prevalence, neurobiology, and treatments for apathy in prodromal dementia. IntPsychogeriatr 30: , 177–184. |
[14] | Ismail Z , Gatchel J , Bateman DR , Barcelos-Ferreira R , Chantillon M , Jaeger J , Donovan NJ , Mortby ME ((2018) ) Affective and emotional dysregulation as pre-dementia risk markers: exploring the mild behavioral impairment symptoms of depression, anxiety, irritability, and euphoria. Int Psychogeriatr 30: , 185–196. |
[15] | Bateman DR , Gill S , Hu S , Foster ED , Ruthirakuhan MT , Sellek AF , Mortby ME , Matušková V , Ng KP , Tarawneh RM , Freund-Levi Y , Kumar S , Gauthier S , Rosenberg PB , Oliveira FFd , Devanand DP , Ballard C , Ismail Z ((2020) ) Agitation and impulsivity in mid and late life as possible risk markers for incident dementia. Alzheimers Dement (N Y), in press. |
[16] | Desmarais P , Lanctot KL , Masellis M , Black SE , Herrmann N ((2018) ) Social inappropriateness in neurodegenerative disorders. Int Psychogeriatr 30: , 197–207. |
[17] | Fischer CE , Aguera-Ortiz L ((2018) ) Psychosis and dementia: risk factor, prodrome, or cause? Int Psychogeriatr 30: , 209–219. |
[18] | Ismail Z , Aguera-Ortiz L , Brodaty H , Cieslak A , Cummings J , Fischer CE , Gauthier S , Geda YE , Herrmann N , Kanji J , Lanctot KL , Miller DS , Mortby ME , Onyike CU , Rosenberg PB , Smith EE , Smith GS , Sultzer DL , Lyketsos C , Istaart N-P ((2017) ) The mild behavioral impairment checklist (MBI– C): arating scale for neuropsychiatric symptoms in pre- dementia populations. J Alzheimers Dis 56: , 929–938. |
[19] | Mattsson N , Cullen NC , Andreasson U , Zetterberg H , Blennow K ((2019) ) Association between longitudinal plasma neurofilament light and neurodegeneration in patients with alzheimer disease. JAMA Neurol 76: , 791–799. |
[20] | Weston PSJ , Poole T , Ryan NS , Nair A , Liang Y , Macpherson K , Druyeh R , Malone IB , Ahsan RL , Pemberton H , Klimova J , Mead S , Blennow K , Rossor MN , Schott JM , Zetterberg H , Fox NC ((2017) ) Serum neurofilament light in familial Alzheimer disease: a marker of early neurodegeneration. Neurology 89: , 2167–2175. |
[21] | Gaiottino J , Norgren N , Dobson R , Topping J , Nissim A , Malaspina A , Bestwick JP , Monsch AU , Regeniter A , Lindberg RL , Kappos L , Leppert D , Petzold A , Giovannoni G , Kuhle J ((2013) ) Increased neurofilament light chain blood levels in neurodegenerative neurological diseases. PLoS One 8: , e75091. |
[22] | Mattsson N , Andreasson U , Zetterberg H , Blennow K , Alzheimer’s Disease Neuroimaging Initiative ((2017) ) Association of plasma neurofilament light with neurodegeneration in patients with Alzheimer disease. JAMA Neurol 74: , 557–566. |
[23] | Lewczuk P , Ermann N , Andreasson U , Schultheis C , Podhorna J , Spitzer P , Maler JM , Kornhuber J , Blennow K , Zetterberg H ((2018) ) Plasma neurofilament light as a potential biomarker of neurodegeneration in Alzheimer’s disease. Alzheimers Res Ther 10: , 71. |
[24] | Hampel H , O’Bryant SE , Molinuevo JL , Zetterberg H , Masters CL , Lista S , Kiddle SJ , Batrla R , Blennow K ((2018) ) Blood-based biomarkers for Alzheimer disease: mapping the road to the clinic. Nat Rev Neurol 14: , 639–652. |
[25] | Benedet AL , Ashton NJ , Pascoal TA , Leuzy A , Mathotaarachchi S , Kang MS , Therriault J , Savard M , Chamoun M , Scholl M , Zimmer ER , Gauthier S , Labbe A , Zetterberg H , Blennow K , Neto PR ((2019) ) Plasma neurofilament light associates with Alzheimer’s disease metabolic decline in amyloid-positive individuals. Alzheimers Dement (Amst) 11: , 679–689. |
[26] | Rohrer JD , Woollacott IO , Dick KM , Brotherhood E , Gordon E , Fellows A , Toombs J , Druyeh R , Cardoso MJ , Ourselin S ((2016) ) Serum neurofilament light chain protein is a measure of disease intensity in frontotemporal dementia. Neurology 87: , 1329–1336. |
[27] | Sheikh F , Ismail Z , Mortby ME , Barber P , Cieslak A , Fischer K , Granger R , Hogan DB , Mackie A , Maxwell CJ , Menon B , Mueller P , Patry D , Pearson D , Quickfall J , Sajobi T , Tse E , Wang M , Smith EE , PROMPT registry investigators ((2018) ) Prevalence of mild behavioral impairment in mild cognitive impairment and subjective cognitive decline, and its association with caregiver burden. Int Psychogeriatr 30: , 233–244. |
[28] | Cano J , Chan V , Kan CN , Chen C , Hilal S , Venketasubramanian N , Xu X ((2018) ) Mild behavioral impairment: prevalence in clinical setting and cognitive correlates. Alzheimers Dement 14: , 639–640. |
[29] | Yoon E , Ismail Z , Hanganu A , Kibreab M , Hammer T , Cheetham J , Kathol I , Sarna JR , Martino D , Furtado S , Monchi O ((2019) ) Mild behavioral impairment is linked to worse cognition and brain atrophy in Parkinson’s disease. Neurology 93: , e766–e777. |
[30] | Matsuoka T , Ismail Z , Narumoto J ((2019) ) Prevalence of mild behavioral impairment and risk of dementia in a psychiatric outpatient clinic. J Alzheimers Dis 70: , 505–513. |
[31] | Ismail Z , Hu S , Gill S , Forkert ND , Smith EE ((2019) ) Subjective cognitive decline (SCD) and mild behavioral impairment (MBI) together predict mild cognitive impairment at 3 years better than either syndrome alone. Alzheimers Dement 15 (7 Suppl): , P1535–P1536. |
[32] | Andrews SJ , Ismail Z , Anstey KJ , Mortby M ((2018) ) Association of Alzheimer’s genetic loci with mild behavioral impairment. Am J Med Genet B Neuropsychiatr Genet 177: , 727–735. |
[33] | Gill S , Wang M , Forkert ND , MacMaster FP , Smith EE , Ismail Z ((2019) ) Diffusion tensor imaging in pre-dementia risk states: white matter atrophy findings in mild behavioral impairment. Neurology 92: , P5.1–025. |
[34] | Jack CR Jr , Bennett DA , Blennow K , Carrillo MC , Dunn B , Haeberlein SB , Holtzman DM , Jagust W , Jessen F , Karlawish J ((2018) ) NIA-AA research framework: toward a biological definition of Alzheimer’s disease. Alzheimers Dement 14: , 535–562. |
[35] | Lussier FZ , Pascoal TA , Chamoun M , Therriault J , Tissot C , Savard M , Kang MS , Mathotaarachchi S , Benedet AL , Parsons M , Qureshi MNI , Thomas ÉM , Shin M , Dion L-A , Massarweh G , Soucy J-P , Tsai I-H , Vitali P , Ismail Z , Rosa-Neto P , Gauthier S ((2020) ) Mild behavioral impairment is associated with β-amyloid but not tau or neurodegeneration in cognitively intact elderly individuals. Alzheimers Dement 16: , 192–199. |
[36] | Mielke MM , Syrjanen JA , Blennow K , Zetterberg H , Vemuri P , Skoog I , Machulda MM , Kremers WK , Knopman DS , Jack CJr. , Petersen RC , Kern S ((2019) ) Plasma and CSF neurofilament light: relation to longitudinal neuroimaging and cognitive measures. Neurology 93: , e252–e260. |
[37] | Al Shweiki MR , Steinacker P , Oeckl P , Hengerer B , Danek A , Fassbender K , Diehl-Schmid J , Jahn H , Anderl-Straub S , Ludolph AC ((2019) ) Neurofilament light chain as a blood biomarker to differentiate psychiatric disorders from behavioural variant frontotemporal dementia. J Psychiatr Res 113: , 137–140. |
[38] | Katisko K , Cajanus A , Jääskeläinen O , Kontkanen A , Hartikainen P , Korhonen VE , Helisalmi S , Haapasalo A , Koivumaa-Honkanen H , Herukka S-K ((2020) ) Serum neurofilament light chain is a discriminative biomarker between frontotemporal lobar degeneration and primary psychiatric disorders. J Neurol 267: , 162–167. |
[39] | Bacioglu M , Maia LF , Preische O , Schelle J , Apel A , Kaeser SA , Schweighauser M , Eninger T , Lambert M , Pilotto A , Shimshek DR , Neumann U , Kahle PJ , Staufenbiel M , Neumann M , Maetzler W , Kuhle J , Jucker M ((2016) ) Neurofilament light chain in blood and CSF as marker of disease progression in mouse models and in neurodegenerative diseases. Neuron 91: , 494–496. |
[40] | Quiroz YT , Zetterberg H , Reiman EM , Chen Y , Garcia G , Villegas A , Sepulveda D , Villada M , Arboleda-Velasquez JF , Giraldo M ((2019) ) Plasma neurofilament light measurements in more than 2,100 presenilin-1 E280 mutation carriers and non-carriers from the world’s largest autosomal dominant Alzheimer’sdisease kindred. Alzheimers Dement 15: , 1627–1628. |
[41] | Hanseeuw BJ , Jonas V , Jackson J , Betensky RA , Rentz DM , Johnson KA , Sperling RA , Donovan NJ ((2018) ) Association of anxiety with subcortical amyloidosis in cognitively normal older adults. Mol Psychiatry 16: , doi: 10.1038/s41380-018-0214-2. |
[42] | Šimić G , Leko MB , Wray S , Harrington CR , Delalle I , Jovanov-Milošević N , Bažadona D , Buée L , de Silva R , Di Giovanni G ((2017) ) Monoaminergic neuropathology in Alzheimer’s disease. Prog Neurobiol 151: , 101–138. |
[43] | Gatchel JR , Rabin JS , Buckley RF , Locascio JJ , Quiroz YT , Yang H-S , Vannini P , Amariglio RE , Rentz DM , Properzi M ((2019) ) Longitudinal association of depression symptoms with cognition and cortical amyloid among community-dwelling older adults. JAMA Netw Open 2: , e198964–e198964. |
[44] | Mortby ME , Ismail Z , Anstey KJ ((2018) ) Prevalence estimates of mild behavioral impairment in a population-based sample of pre-dementia states and cognitively healthy older adults. IntPsychogeriatr 30: , 221–232. |
[45] | Mallo SC , Ismail Z , Pereiro AX , Facal D , Lojo-Seoane C , Campos-Magdaleno M , Juncos-Rabadán O ((2019) ) Assessing mild behavioral impairment with the mild behavioral impairment checklist in people with subjective cognitive decline. IntPsychogeriatr 31: , 231–239. |
[46] | Mallo SC , Ismail Z , Pereiro AX , Facal D , Lojo-Seoane C , Campos-Magdaleno M , Juncos-Rabadán O ((2018) ) Assessing mild behavioral impairment with the mild behavioral impairment-checklist in people with mild cognitive impairment. J AlzheimersDis 66: , 83–95. |
[47] | Gill S , Mouches P , Hu S , Rajashekar D , MacMaster FP , Smith EE , Forkert ND , Ismail Z ; Alzheimer’s Disease Neuroimaging Initiative ((2020) ) Using machine learning to predict dementia from neuropsychiatric symptom and neuroimaging data. J Alzheimers Dis 75: , 277–288. |
[48] | Lussier F , Pascoal T , Therriault J , Chamoun M , Tissot C , Savard M , Mathotaarachchi S , Ismail Z , Rosa-Neto P , Gauthier S ((2019) ) Mild behavioral impairment is associated with beta-amyloid and tau across the Alzheimer’s disease spectrum. . J Cereb Blood Flow Metab 39,: , 158–159. |
[49] | Hu S , Patten SB , Fick G , Smith EE , Ismail Z ((2019) ) Validation of the mild behavioral impairment checklist (MBI– C) in a clinic based sample. Alzheimers Dement 15: , 365. |
[50] | Creese B , Griffiths A , Brooker H , Corbett A , Aarsland D , Ballard C , Ismail Z ((2019) ) Profile of mild behavioral impairment and factor structure of the mild behavioral impairment checklist in cognitively normal older adults. Int Psychogeriatr 17: , 1–13. |
[51] | Chertkow H , Borrie M , Whitehead V , Black SE , Feldman HH , Gauthier S , Hogan DB , Masellis M , McGilton K , Rockwood K ((2019) ) The comprehensive assessment of neurodegeneration and dementia: Canadian cohort study. Can J Neurol Sci 46: , 499–511. |
[52] | Jutten RJ , Harrison J , de Jong FJ , Aleman A , Ritchie CW , Scheltens P , Sikkes SA ((2017) ) A composite measure of cognitive and functional progression in Alzheimer’s disease: design of the capturing changes in cognition study. Alzheimers Dement (N Y) 3: , 130–138. |
[53] | Nosheny RL , Camacho MR , Insel PS , Flenniken D , Fockler J , Truran D , Finley S , Ulbricht A , Maruff P , Yaffe K ((2018) ) Online study partner-reported cognitive decline in the brain health registry. Alzheimers Dement (N Y) 4: , 565–574. |
[54] | Zeghari R , Manera V , Fabre R , Guerchouche R , König A , Phan Tran MK , Robert P ((2020) ) The “Interest Game”: A ludic application to improve apathy assessment in patients with neurocognitive disorders. J Alzheimers Dis 74: , 669–677. |
[55] | Sheardova K , Vyhnalek M , Nedelska Z , Laczo J , Andel R , Marciniak R , Cerman J , Lerch O , Hort J ((2019) ) Czech Brain Aging Study (CBAS): prospective multicentre cohort study on risk and protective factors for dementia in the Czech Republic. BMJ Open 9: , e030379. |
[56] | Leuzy A , Smith R , Ossenkoppele R , Santillo A , Borroni E , Klein G , Olsson T , Jogi J , Palmqvist S , Mattsson N , Strandberg O , Stomrud E , Hansson O ((2020) ) Diagnostic performance of [18F]RO948 tau positron emission tomography in the differentiation of AD from other neurodegenerative disorders. JAMA Neurol 11: , e200989. |