Revolution of Alzheimer Precision Neurology. Passageway of Systems Biology and Neurophysiology
Abstract
The Precision Neurology development process implements systems theory with system biology and neurophysiology in a parallel, bidirectional research path: a combined hypothesis-driven investigation of systems dysfunction within distinct molecular, cellular, and large-scale neural network systems in both animal models as well as through tests for the usefulness of these candidate dynamic systems biomarkers in different diseases and subgroups at different stages of pathophysiological progression. This translational research path is paralleled by an “omics”-based, hypothesis-free, exploratory research pathway, which will collect multimodal data from progressing asymptomatic, preclinical, and clinical neurodegenerative disease (ND) populations, within the wide continuous biological and clinical spectrum of ND, applying high-throughput and high-content technologies combined with powerful computational and statistical modeling tools, aimed at identifying novel dysfunctional systems and predictive marker signatures associated with ND. The goals are to identify common biological denominators or differentiating classifiers across the continuum of ND during detectable stages of pathophysiological progression, characterize systems-based intermediate endophenotypes, validate multi-modal novel diagnostic systems biomarkers, and advance clinical intervention trial designs by utilizing systems-based intermediate endophenotypes and candidate surrogate markers. Achieving these goals is key to the ultimate development of early and effective individualized treatment of ND, such as Alzheimer’s disease. The Alzheimer Precision Medicine Initiative (APMI) and cohort program (APMI-CP), as well as the Paris based core of the Sorbonne University Clinical Research Group “Alzheimer Precision Medicine” (GRC-APM) were recently launched to facilitate the passageway from conventional clinical diagnostic and drug development toward breakthrough innovation based on the investigation of the comprehensive biological nature of aging individuals. The APMI movement is gaining momentum to systematically apply both systems neurophysiology and systems biology in exploratory translational neuroscience research on ND.
Abbreviation
18F-FDG-PET, 18F-2-fluoro-2-deoxy-D-glucose PET; Aβ42, 42-amino acid-long amyloid beta peptide; AD, Alzheimer’s disease; ADD, Alzheimer’s disease dementia; ADNI, Alzheimer’s Disease Neuroimaging Initiative; ADO, Alzheimer’s disease ontology; APMI, Alzheimer Precision Medicine Initiative; APMI-CP, Alzheimer Precision Medicine Initiative Cohort Program; APP, amyloid precursor protein; BFCS, basal forebrain cholinergic system; CSF, cerebrospinal fluid; DBS, deep brain stimulation; DLB, Dementia with Lewy bodies; DTI, diffusion tensor imaging; EEG, electroencephalography; EHRs, electronic health records; EPAD, European Prevention of Alzheimer’s Dementia consortium; EPAD LCS, EPAD Longitudinal Cohort Study; EPI, echo planar imaging; FA, fractional anisotropy; fMRI, functional magnetic resonance imaging; FTD, frontotemporal dementia; ICNs, intrinsic coherent networks; IDM, integrative disease modeling; MCI, mild cognitive impairment; MD, mean diffusivity; MEG, magnetoencephalography; MMN, mismatch negativity; MRI, magnetic resonance imaging; ND, neurodegenerative diseases; NFL, nerve fiber layer; p-tau, hyperphosphorylated tau; Nold, normal elderly subjects; PDD, dementia due to Parkinson’s; PET, Positron Emission Tomography; PM, Precision medicine; PMI, Precision Medicine Initiative; PoC, Proof-of-Concept; RGC, retinal ganglion cell; ROI, region of interest; rTMS, repetitive transcranial magnetic stimulation; SBML, Systems Biology Markup Language; SPECT, Single Photon Emission Computed Tomography; t-tau, total tau; tACS, transcranial alternating current stimulation; tDCS, transcranial direct current stimulation; WB-MRI, whole-body magnetic resonance imaging; WES, whole-exome sequencing; WGS, whole-genome sequencing; WM, white matter.
INTRODUCTION
A dementia syndrome is caused by a range of neurological disorders; Alzheimer’s disease (AD) is the most common disease-causing dementia, accounting for 50–70% of cases. Increasing age is the most important risk factor for AD and other dementias, and as life expectancy increases and demographic aging occurs in populations around the world, the number of people with dementia is expected to continue to exponentially grow. In 2015, almost 47 million people worldwide were estimated to be affected by dementia, and the numbers are expected to reach 75 million by 2030, and 131 million by 2050, with the greatest increase expected in low-income and middle-income countries [1].
On May 29, 2017, at the 70th session of the World Health Assembly in Geneva, the World Health Organization (WHO) has unanimously adopted a global plan on dementia—the Global Plan of Action on the Public Health Response to Dementia 2017–2025—that includes targets for the advancement of dementia awareness, risk reduction, diagnosis, care and treatment, support for care partners, and research (available at https://www.alz.co.uk/news/global-plan-on-dementia-adopted-by-who).
Recent years have witnessed an increasing understanding of the molecular mechanisms related to AD. The pathogenesis of this complex polygenic neurodegenerative disease (ND) involves sequentially interacting pathophysiological cascades, including both core events, i.e., accumulation of the 42-amino acid-long amyloid-β (Aβ42) peptide into amyloid plaques and self-aggregation of hyperphosphorylated tau protein to form intraneuronal neurofibrillary tangles, and downstream processes, such as generalized neuroinflammation [2, 3]. These events induce axonal degeneration [4–6] and disruption of synaptic integrity [7, 8], thus leading to synaptic dysfunction and, ultimately, deterioration of physiological neural connectivity [9].
In spite of such advancements in understanding the disease, AD is characterized by a high degree of heterogeneity in its manifestation, progression, and response to treatment, as well as susceptibility to risk factors. Phenotypic variability is currently considered one of the biggest challenges in clinical science and clinical trial design [10]. On the one hand, the same syndrome can be caused by substantially different pathophysiological mechanisms. In order to ensure more precise and definitive AD diagnosis, biomarkers are crucially needed to detect and track disease processes in the brain. On the other hand, similar pathophysiology can present itself with distinct symptomatology across patients, suggesting that additional factors can influence disease manifestation and progression. The identity and impact of such additional factors (including genetic, epigenetic, lifestyle, and phenotypic traits) deserve further investigation. Particularly, a growing body of evidence demonstrated that a factor such as an individual’s sex can modulate disease phenotype and drug response [11], thus substantially contributing to clinical heterogeneity. In AD patients, sex differences have been reported in the rate of cognitive deterioration [12, 13] and brain atrophy [14], in the absence of clear differences in amyloid or tau burden [15]. In addition, sex-genotype interaction in AD have been shown to affect both risk of onset and conversion [16] as well as response to pharmacological treatment [17, 18]. The socio-economic construct associated with the female and male position in the society (i.e., gender) can also influence disease onset and progression, as it affects education, salary, pension plans, and caregiving burden [19]. Therefore, sex and gender appear to be central drivers of phenotypic variability in AD and their role should be carefully considered when designing strategies for prevention, detection and treatment of the disease. Analysis of sex and gender effects, both alone and in combination with a variety of genetic, epigenetic, and phenotypic traits, should be the first step toward a more personalized and patient-centered approach to AD.
THE PRECISION NEUROLOGY PARADIGM IN ALZHEIMER’S DISEASE
Breakthrough conceptual shifts have recently commenced to emerge in the field of AD and other ND, highlighting the presence of risk and protection factors and the non-linear dynamic continuum of complex pathophysiologies along a wide spectrum of multi-factorial brain proteinopathies. Substantial advancements in detecting, treating, and preventing AD are expected to evolve through the generation and the systematic implementation of a strategy based on the precision medicine (PM) paradigm [20, 21], whose establishment requires the implementation of an array of integrated disciplines and technological developments such as the “omics” approaches, neuroimaging modalities, cognitive assessment tests, and clinical characteristics. These converge to several domains that need to be analyzed according to the systems theory paradigm [22]. This allows for the conceptualization of novel and original models to elucidate all systems levels, assessed by systems biology and systems neurophysiology (Fig. 1), and the different types of spatiotemporal data characterizing the genetically, biologically, pathologically, and clinically heterogeneous construct of “AD” [21]. Thus, systems biology and systems neurophysiology permit to delineate the multivariate and combinatorial profiles of genetic, biological, pathophysiological, and clinical markers reflecting the heterogeneity of this condition. Thanks to fundamental advances in research technology, we got new and better performing analysis tools to register and create comprehensive brains maps and record dynamic patterns across different systems: from molecules, neurons to brain areas. Particularly, systems neurophysiology will aim at showing how computational network models can elucidate the relationship between structure and dynamic function in brain networks, as demonstrated by recent findings in time-dependent functional connectivity measured with non-invasive neuroimaging techniques.
Fig.1
Cohorts stratified according to different neuroimaging modalities and methods are integrated in the disease modeling for classification and prediction of subsets of AD and other ND patients. The paradigm of systems neurophysiology aims at studying the fundamental principles of integrated neural systems functioning by integrating and analyzing neural information recorded in multimodal fashion through computational modeling and combining data-mining methods. This paradigm may be used to decode the information contained in experimentally-recorded neural activity using analysis methods that are able to integrate the recordings of simultaneous, single-modality brain cell activity such as fMRI or EEG to generate synergistic insight and possibly infer hidden neurophysiological variables. The ultimate goal of systems neurophysiology is to clarify how signals are represented within neocortical networks and the specific roles played by the multitude of different neuronal components. AD, Alzheimer’s disease; DTI, diffusion tensor imaging; EEG, electroencephalography; MEG, magnetoencephalography; fMRI, functional magnetic resonance imaging, sMRI, structural magnetic resonance imaging; ND, neurodegenerative diseases; PET, positron emission tomography; TMS, transcranial magnetic stimulation
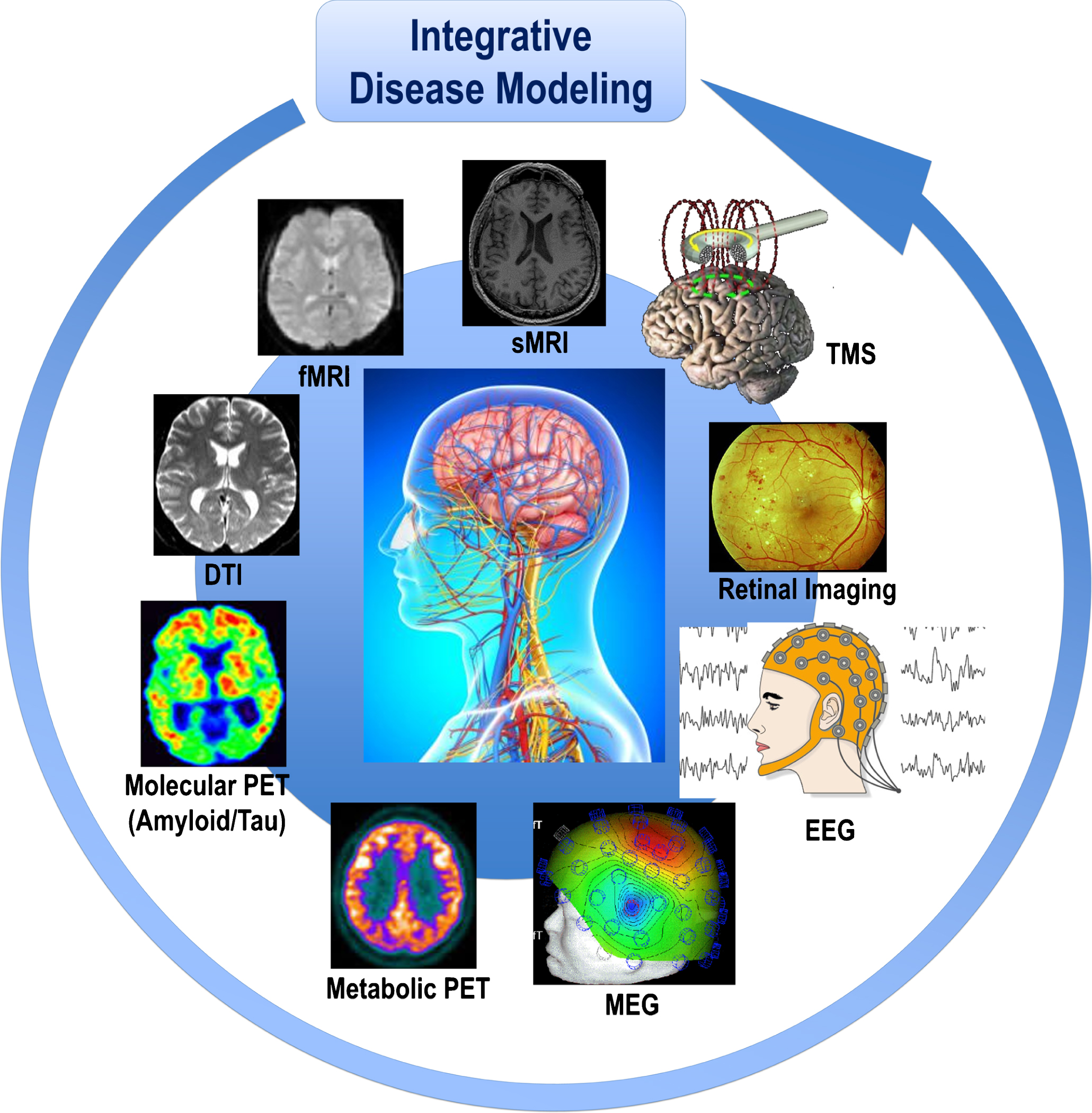
The transition to PM from the traditional model does not occur overnight. But the more we build innovative and interdisciplinary networks with partners, the faster and more effectively we can see the changes happening. To fulfill on the promise of PM, there needs to be a new ecosystem with partnerships of multiple stakeholders who collaborate to find creative and novel solutions. Such a new ecosystem, comprised of academic and community providers, industry, professional societies, government, consumers, and patient advocacy groups, could advance the following pilot initiatives on a local, national and potentially international scale.
In order to advance the development of the PM paradigm in AD, the international Alzheimer PM Initiative (APMI) and its planned Cohort Program (APMI-CP) (Fig. 2) have been recently launched by our consortium and thematically linked to the U.S. Precision Medicine Initiative (PMI) (available at https://www.whitehouse.gov/precision-medicine) and the U.S. “All of Us Research Program”, evolved from the U.S. PMI Cohort Program (available at https://www.nih.gov/research-training/allofus-research-program) (Table 1). Four pioneering translational neuroscience research programs—“MIDAS”, “PHOENIX”, “POSEIDON”, and “VISION”—have been developed and launched in an interdisciplinary local network by our group at the APMI and APMI-CP initiation site Paris, France, at the Sorbonne University (Sorbonne Université) and at the Pitié-Salpêtrière University Hospital, Institute for Memory and Alzheimer’s Disease (Institut de la Mémoire et de la Maladie d’Alzheimer, IM2A) and the Brain and Spine Institute (Institut du Cerveau et de la Moelle É pinière, ICM) in Paris to organize, combine, and integrate the components of systems biology and neurophysiology in order to facilitate the development of PM in AD, a model approach for other proteinopathies/ND of the brain. In this regard, following the APMI conceptual framework, mono-center pilot APMI subcohorts spanning from early asymptomatic preclinical populations to prodromal to dementia late stage populations, namely INSIGHT-preAD, Predict-MA PHRC, RESPIR, and SOCRATES, have been established at our central clinical recruitment site, the IM2A. These pilot APMI cohorts allow for the standardized academic university-based expert center inclusion of both cognitively intact individuals at risk for AD and patients with a full range of ND and provide an assortment of unique heterogeneous and multidimensional data. The research using these pilot AMPI cohorts is performed under the structural framework of the newly established Sorbonne University – “Clinical Research Group in Alzheimer Precision Medicine” (GRC n° 21), Sorbonne Université – “Groupe de Recherche Clinique - Alzheimer Precision Medicine”) (GRC-APM). The major objective of the Sorbonne Université GRC-APM is to accelerate the reformation of traditional Neurology, Psychiatry, and Neuroscience embracing the PM paradigm, based on complex systems theory, using systems biology and systems neurophysiology, big data science, and biomarker-guided integrative disease modeling (IDM) to improve detection, classification, and therapy development in AD and other ND.
Fig.2
Translational bench-to-bedside data flow within the conceptual framework of the Alzheimer Precision Medicine Initiative (APMI). The IDM-based “Data Sciences Lifecycle” takes advantage of both data-driven and knowledge-driven approaches so that both quantitative data (biomolecular, neuroimaging/neurophysiological, and clinical data) and qualitative data (collected from scientific literature and on-line media)—generated through the application of systems biology and systems neurophysiology paradigms—are represented in a harmonized, standardized format to be prepared for proper management within an integrative computational infrastructure. Indeed, the resulting heterogeneous, multidimensional big and deep data are harmonized, standardized, and integrated via computational and data science methods in the form of mechanistic disease models, according to the IDM conception. Disease-specific integrative computational models play a key role in the IDM paradigm and represent the foundations for “actionable” P4M measures in the area of AD and other ND. As a result, the integrative disease models are anticipated to support decision making for: 1) early diagnosis of brain disease progression with mechanistic biomarkers (predictive), 2) screening populations and stratifying individuals at high risk of developing ND based on mechanistic co-morbidities in order to reduce the likelihood of disease and disability (preventive), 3) tailoring treatment to the right patient population at the right time (personalized), and 4) optimizing “actionable” plans for the benefit of patients based on patient-oriented information gathered in EHRs and on patients’ feedback reported in social media. Internet has greatly enabled the participation of individual patients in the healthcare through sharing their experiences in various social media and other online resources (participatory). The output is anticipated to be an “actionable” model that permits the prediction of the trajectory of individual patient-centric detection or treatment within the implementation of the P4M paradigm. APMI, Alzheimer Precision Medicine Initiative; EHRs, electronic health records; IDM, integrative disease modeling; ND, neurodegenerative diseases; P4M, Predictive, Preventive, Personalized, Participatory Medicine. Modified from [21].
![Translational bench-to-bedside data flow within the conceptual framework of the Alzheimer Precision Medicine Initiative (APMI). The IDM-based “Data Sciences Lifecycle” takes advantage of both data-driven and knowledge-driven approaches so that both quantitative data (biomolecular, neuroimaging/neurophysiological, and clinical data) and qualitative data (collected from scientific literature and on-line media)—generated through the application of systems biology and systems neurophysiology paradigms—are represented in a harmonized, standardized format to be prepared for proper management within an integrative computational infrastructure. Indeed, the resulting heterogeneous, multidimensional big and deep data are harmonized, standardized, and integrated via computational and data science methods in the form of mechanistic disease models, according to the IDM conception. Disease-specific integrative computational models play a key role in the IDM paradigm and represent the foundations for “actionable” P4M measures in the area of AD and other ND. As a result, the integrative disease models are anticipated to support decision making for: 1) early diagnosis of brain disease progression with mechanistic biomarkers (predictive), 2) screening populations and stratifying individuals at high risk of developing ND based on mechanistic co-morbidities in order to reduce the likelihood of disease and disability (preventive), 3) tailoring treatment to the right patient population at the right time (personalized), and 4) optimizing “actionable” plans for the benefit of patients based on patient-oriented information gathered in EHRs and on patients’ feedback reported in social media. Internet has greatly enabled the participation of individual patients in the healthcare through sharing their experiences in various social media and other online resources (participatory). The output is anticipated to be an “actionable” model that permits the prediction of the trajectory of individual patient-centric detection or treatment within the implementation of the P4M paradigm. APMI, Alzheimer Precision Medicine Initiative; EHRs, electronic health records; IDM, integrative disease modeling; ND, neurodegenerative diseases; P4M, Predictive, Preventive, Personalized, Participatory Medicine. Modified from [21].](https://ip.ios.semcs.net:443/media/jad/2018/64-s1/jad-64-s1-jad179932/jad-64-jad179932-g002.jpg)
Table 1
The five pillars of the Alzheimer Precision Medicine Initiative (APMI). The mission of APMI is to transform Neurology and Neuroscience embracing Precision Medicine (or Precision Neurology) based on complex systems theory using integrative disease modeling (IDM) to facilitate health care solutions for brain proteinopathies, protein misfolding disorders, and neurodegenerative diseases, such as Alzheimer’s disease (AD). This is facilitated through five breakthrough theoretical scientific advances, as follows:
Concept | Comment |
(1) The emergence of the “precision medicine” paradigm | Discovery and development of treatments targeted to the needs of individuals on the basis of systems biology technology using genomic biomarker, phenotypic, or psychosocial characteristics that distinguish a given individual from others. Inherent in this definition is the goal of impacting pathophysiological progression at early disease stages and clinical outcomes at later stages and minimizing unnecessary side effects for those less likely to have a response to a particular treatment supported by pharmacogenomics. The convergence of genetics/genomics/transcriptomics, bioinformatics, neurodynamics, neuroimaging, and connectomics along with other technologies such as cell sorting, epigenetics, proteomics, lipidomics and metabolomics, is rapidly expanding the scope of precision medicine by refining the staging and classification of disease, often with important prognostic and treatment implications. Among these new technologies, genetics and next-generation DNA sequencing methods are having the greatest effect. |
(2) The emergence of the “systems biology” paradigm | Systems biology represents an integrated and deeper investigation of interacting biomolecules within cells or organisms. This approach has only recently become feasible as high-throughput technologies including cDNA microarrays, mass spectrometric analyses of proteins and lipids together with rigorous bioinformatics have evolved. High-content data point to convergent pathways among diseases, which transcend descriptive studies to reach a more integrated understanding of neurodegenerative disease pathogenesis and, in some instances, highlighting ‘druggable’ network nodes. |
(3) The emergence of the “systems neurophysiology and complex network” paradigm | This is due in large part to advances in mathematics, computer science and statistical methods applied to neuroimaging and neurophysiology; instead of thinking of the brain as a set of modules (i.e., individual brain regions) that perform specific cognitive functions, the network paradigm argues that cognitive functions are performed by dynamic interactions among different brain areas, i.e., by dynamically formed complex structural and functional networks of brain regions. |
(4) the emergence of “neural modeling” paradigm | This paradigm is required by the complex network paradigm, since, in order to deal with the large complexity of the dynamic interactions among multiple brain regions, one must employ advanced mathematical and computational methods. |
(5) The emergence of “integrative disease modeling” (IDM) paradigm | This is an evolving knowledge-based paradigm in translational research that exploits the power of advanced computational methods to collect, store, integrate, model, and interpret accumulated disease information across different biological scales, i.e., from molecules to phenotypes. IDM is a new paradigm at the core of translational research, which prepares the ground for transitioning from descriptive to mechanistic representation of disease processes. Given the tremendous potential of IDM in supporting translation of biomarker and drug research into clinically applicable diagnostic, preventive, prognostic, and therapeutic strategies, it is anticipated that computer-readable disease models will be an indispensable part of future efforts in the P4 medicine research area. |
The implementation of PM in AD is expected to result into a novel, original scientific taxonomy and a distinguished working lexicon and terminology (see Table 2) for reality-based medicine, which detects evidence from real-life scenarios.
Table 2
Evolving lexicon and terminology within the Alzheimer Precision Medicine Initiative (APMI) framework
Concept | Abbreviation | Definition |
Big Data | A repository of large amounts of data sets generated by data mining tools. Big Data includes information obtained through systems theory- and, knowledge-based approaches and clinical records. | |
Biomarkers | BMs | A defined characteristic that is measured as an indicator of normal biological processes, pathogenic process, or response to an exposure or intervention, including therapeutic interventions. Molecular, histologic, radiographic, or physiological characteristics are types of biomarkers. A biomarker is not an assessment of how an individual feels, functions, or survives. Categories of biomarkers include: susceptibility/risk biomarker, diagnostic biomarker, monitoring biomarker, prognostic biomarker, predictive biomarker, pharmacodynamics/response biomarker and safety biomarker. |
Data Science | Interdisciplinary field about processes and systems to extract knowledge from data in different forms – either structured or unstructured – which is a continuation of some of the data analysis fields including statistics, artificial intelligence, machine learning, data mining, and predictive analytics. | |
e-Health | Term indicating healthcare practice supported by electronic processes and communication. It can also include health applications and links on mobile phones, referred to as mobile health (“m-health”: smart personal mobile devices, such as phones, wearables, in-home devices and Apps, collecting health information aimed at improving patient care). | |
The term can also encompass a range of services or systems that are at the edge of medicine/healthcare and information technology, including: electronic health records (EHRs). These indicate a systematized gathering of population electronically-stored health information and clinical data in a digital format. These registries can be shared across different health care settings through network systems. | ||
European Prevention of Alzheimer’s Dementia Consortium | EPAD | Pan-European initiative whose objective is to establish a shared platform to design and conduct phase II Proof-of-Concept (PoC) clinical trials specifically aimed at developing novel treatments for the secondary prevention of AD. |
Genomic Medicine | Discipline utilizing personal genomic information (see also the definition of “Personal Genomics”) for diagnostic characterization and the development of therapeutic plans. | |
Integrative Disease Modeling | IDM | Multidisciplinary approach to standardize, manage, integrate, and interpret multiple sources of structured and unstructured quantitative and qualitative data across biological scales using computational models that assist decision making for translation of patient-specific molecular mechanisms into tailored clinical applications. |
“Omics” or “Omic” disciplines | High-throughput screening tools aimed at fully collecting, characterizing and quantifying pools of biological molecules (DNA sequences, transcripts, miRNAs, proteins/peptides, metabolites/lipids) that translate into the structure, function, and dynamics of an organism and/or whole organisms. | |
“One-size-fits-all” approach | Traditional approach used for the development of early detection, intervention, and prevention options, where biomarker candidates are being validated against the plethora of heterogeneous clinical operationalized syndromes, rather than against genetically (risk profile) and biologically (i.e., based on molecular mechanisms and cellular pathways) determined entities. | |
Ontology | Formal naming and designation of the types, properties, and interactions of the entities that really or fundamentally exist for a specific domain of discourse. | |
P4 (Predictive, Preventive, Personalized, and Participatory) Medicine | P4M | Translational medicine component of the Precision Medicine paradigm. It is a clinical practice model aimed at applying knowledge, tools, and strategies of systems medicine. It involves generation, mining, and integration of enormous amounts of data on individual patients to produce predictive and “actionable” models of wellness and disease. |
Personal Genomics | Branch of genomics that provides support in predicting the likelihood that an individual will be affected by a disease. It helps personalize drug selection and treatment delivery to get the best care, thus playing a crucial role both in predictive and personalized medicine, according to the PM paradigm. | |
Personalized Medicine | Component of the P4M aiming at tailoring treatment for individual patients in contrast with “one-size-fits-all” or traditional “magic bullet drug” approach. | |
Precision Medicine | PM | Translational science paradigm related to both health and disease. PM is a biomarker-guided medicine on systems-levels taking into account methodological advancements and discoveries of the comprehensive pathophysiological profiles of complex polygenic, multi-factorial neurodegenerative diseases (proteinopathies of the brain). It aims at optimizing the effectiveness of disease prevention and therapy, by considering (customized) an individual’s specific “biological make-up” (e.g., genetic, biochemical, phenotypic, lifestyle, and psychosocial characteristics) for targeted interventions through P4M implementation. |
Systems Biology | SB | Evolving hypothesis-free, exploratory, holistic (non-reductionistic), global, integrative, and interdisciplinary paradigm using advances in multimodal high-throughput technological platforms that enable the examination of networks of biological pathways where elevated amounts of structurally and functionally different molecules are simultaneously explored over time at a system level (i.e., at the level of cells, group of cells, tissues, organs, apparatuses, or even whole organisms). |
Systems Medicine | SM | Holistic paradigm applying systems biology-based strategies to medical research. It aims at integrating a variety of considerable biomedical data at all levels of the cellular organization (by employing global, integrative, and statistical/mathematical/computational modeling) to explicate the pathophysiological mechanisms, prognosis, diagnosis, and treatment of diseases. |
Systems Neurophysiology | SN | Paradigm aimed at studying the fundamental principles of integrated neural systems functioning by integrating and analyzing neural information recorded in multimodal fashion through computational modeling and combining data-mining methods. This paradigm may be used to decode the information contained in experimentally-recorded neural activity using analysis methods that are able to integrate the recordings of simultaneous, single-modality brain cell activity such as functional magnetic resonance imaging or electroencephalography to generate synergistic insight and possibly infer hidden neurophysiological variables. The ultimate goal of systems neurophysiology is to clarify how signals are represented within neocortical networks and the specific roles played by the multitude of different neuronal components. |
Systems Pharmacology | SP | Science of advancing knowledge about drug action at the molecular, cellular, tissue, organ, organism, and population levels” (http://www.aaps.org/Systems_Pharmacology/). |
Systems Theory | ST | Translational research theory of the Precision Medicine paradigm. It is an interdisciplinary conceptual framework allowing for the conceptualization of novel/original models to extract and explicate all systems levels and different spatiotemporal data types of complex polygenic diseases. |
Modified from [21].
An appropriately integrative understanding of AD will be propelled by advances in molecular technology and data processing that will allow generating, analyzing, interpreting, and storing huge amounts of heterogeneous and multidimensional data, termed big data. Big data in AD can be used to improve our current mechanistic understanding of the disease through the application of different computational and data science methods, under the theoretical framework of IDM [23]. Multimodal big data integration is essential to understand the link between elements from large-scale neurobiological systems such as protein interaction and genetic regulatory networks, synaptic connections and anatomical projections among brain areas. Usually, these data come from multiple levels of organizations or involve different domains of biology and data types (Fig. 3).
Fig.3
Model of non-linear dynamic temporo-spatial progression of neural network disintegration and complex brain systems failure in relation to pathophysiology of AD. Four dimensions of pathophysiological processes in AD. Dimension 1 occurs at the level of neuronal networks (coded green to red). Dimension 1 can begin extremely early in form of synaptic dysfunction and/or synaptotoxic molecular agents, thus altering the balance of the neuronal network. Dimension 2 & 3 can be regarded as the temporal and spatial spreading from almost exclusively default mode to episodic memory networks to temporal, parietal and frontal neocortical associative areas responsible for working memory, language and/or visual processes. Every one of these complex systems can experience a variable degree of decompensation (see Dimension 1), from adaptation to compensation to massive decompensation and widespread disorganization. Dimension 4 is essentially the integration of Dimensions 1 and 2 and 3 into late-stage clinically symptomatic and syndromatic cognitive and later behavioral and psychopathological dysfunction and decline. It is therefore clear how this complex, multi-scale and multilayer association of networks can be partially robust to “insults” if sufficient compensatory mechanisms are in place, but also extremely and randomly fragile if adaptation and compensation fails at any level. Sufficient decompensation in Dimension 1 will turn into a malfunction in Dimension 2 and 3 and, in turn, substantial decompensation in Dimension 2 and 3 will turn into malfunction in Dimension 4 (i.e., mild cognitive impairment, clinical dementia syndrome). AD, Alzheimer’s disease.
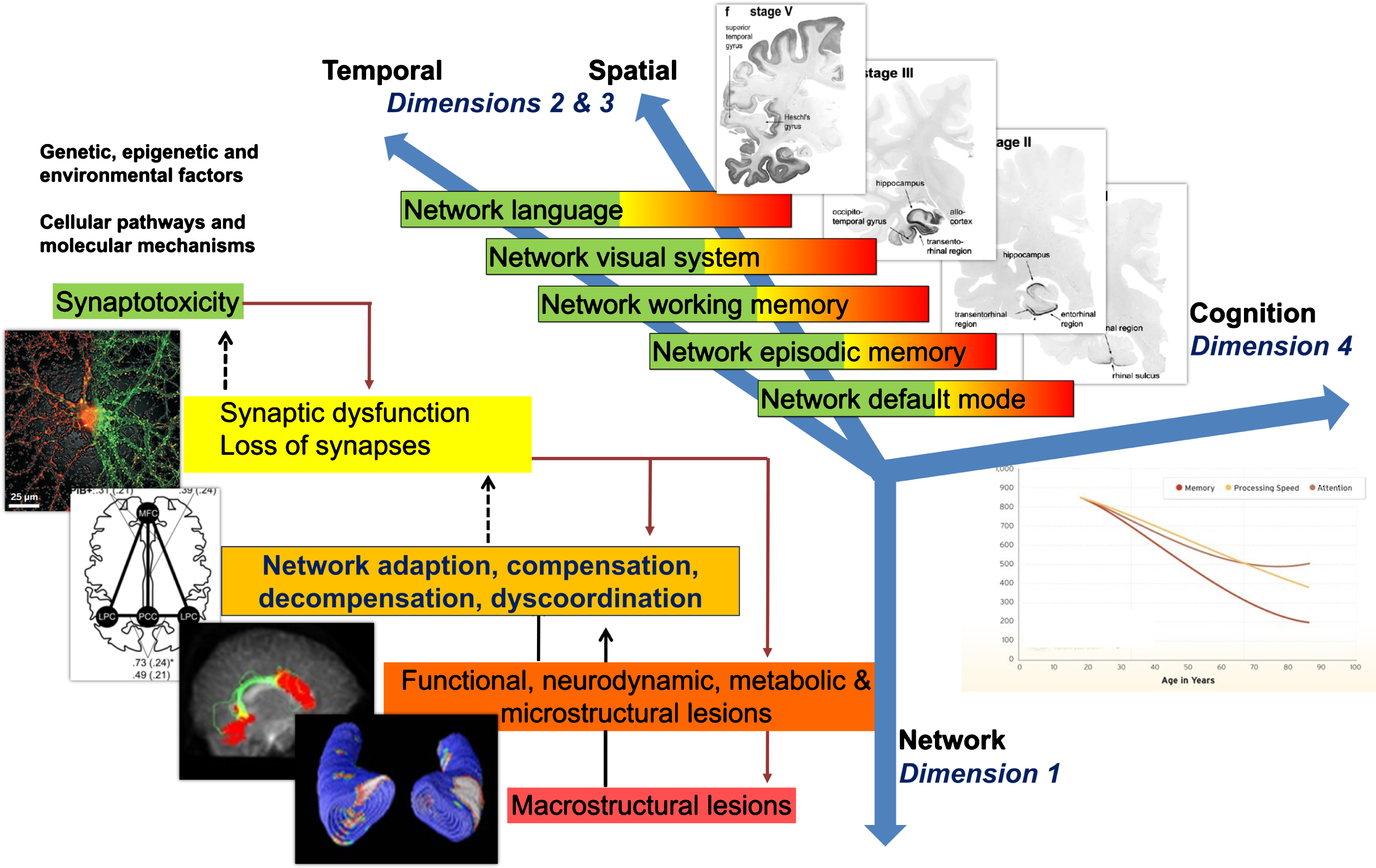
To be effective, PM needs to exploit advanced tools for collecting/managing/examining big data. Particularly, thanks to outstanding progresses in information technology, the development and implementation of electronic health records (EHRs) enable gathering/preserving longitudinal health-care records and clinical data at highly limited costs. Furthermore, the adoption of personal mobile technologies, namely phones, apps, wearables, in-home devices, as innovative ways to collect health information (mobile health or “m-health”) is becoming a common practice. These devices allow the accumulation of clinically relevant information in a more ecological/natural environment and the improvement of patient care. High-volume and dense data generated from progressively more sophisticated software applications can enrich self-reported information on both lifestyle and environment, thus providing researchers with a well-defined vision of these factors, previously difficult to obtain.
Being rooted in a multidimensional data-driven approach, PM is expected to upgrade the prevention and treatment of AD to a higher level of individualization, promoting a shift toward every single preclinical participant at risk rather than late stage patients and disease in general. This goal will be achieved mainly through the identification and validation of reliable biomarkers, which will allow better classifying patients by their probable disease risk, prognosis, and/or response to preventive measures and treatment [20, 21]. To date, PM (in general) and biomarker-guided therapeutic strategies (in particular) have witnessed their broadest applications in the field of oncology. The Food and Drug Administration (FDA) has recently approved for the first time a cancer treatment based on the presence of specific molecular aberrations rather than on the tumor’s anatomical origin. Pembrolizumab (a humanized antibody used in cancer immunotherapy) has been granted approval for adult and pediatric patients with metastatic or unresectable, microsatellite instability-high (MSI-H) or mismatch repair deficient (dMMR) solid tumors [24]. The implementation of PM in ND currently impels researchers to envision a cross-trans-fertilization from such more advanced fields of medicine. In this setting, the repurposing of some previously approved mechanistic anticancer drugs for ND may offer the potential to reduce both the cost and time to achieve licensed approval status. For instance, tyrosine kinase inhibitors like bosutinib [25] and masitinib [26] (which represent a standard approach for anticancer treatment) have shown promising clinical results in patients with amyotrophic lateral sclerosis and can also exert neuroprotective actions in other ND through the activation of autophagy. The search basin for anticancer drugs repositionable for neurodegeneration will ultimately require data-driven approaches grounded on specific biomarker data; such a strategy is aimed at identifying pathophysiological commonalities, potentially common molecular alterations between cancer and ND [26].
Apart from treatment, another important aim of PM in AD will be the preclinical detection of pathophysiology at its earliest stage and related early disease initiation and the implementation of preventive interventions at the individual level. This goal may be achieved through an integrated analysis of genetic, biomarker, imaging, and clinical characteristics that distinguish one individual from others. To achieve this goal, the availability of reliable multimodal biological indicators—biomarkers—will be required [27–34]. In this regard, several potential biological markers have been identified across the full spectrum of AD, from preclinical to prodromal to clinical stages [35–41]. This includes different categories, as follows: 1) neurogenetics/neuroepigenetics markers [42–45]; 2) neurochemistry markers [4, 46–48], including both cerebrospinal fluid (CSF) [49–55] and blood (plasma/serum) markers [56–63]; 3) markers derived from structural/functional/metabolic neuroimaging [64–68]; and 4) neurophysiology/neurodynamic markers [69]. Moreover, opinions of regulatory agencies and industry stakeholders in AD biomarker discovery area are regularly in discussion and development [70, 71]. The integration and recomposition of the experimental information obtained from biomarker studies through the systems biology and systems neurophysiology paradigms will ultimately allow to improve patient care and clinical outcomes through the PM paradigm [72] in line with the Institute of Medicine (IOM) Committee Recommendations for Advancing Appropriate Use of Biomarker Tests (companion diagnostics) for Molecularly Targeted Therapies [73].
Starting from these premises, PM can be conceptualized as a biomarker-guided medicine. According to FDA and the NIH Biomarkers, Endpoints, and other Tools (BEST) Resource, biomarker categories can be categorized as follows: 1) susceptibility/risk biomarker, 2) diagnostic biomarker, 3) monitoring biomarker, 4) prognostic biomarker, 5) predictive biomarker, 6) pharmacodynamic/response biomarker, and 7) safety biomarker [74]. Unfortunately, any attempt to provide such a clear-cut classification in the AD field remains problematic. For example, “amyloid positivity” is widely considered both a diagnostic and predictive biomarker; however, this may not be the case at an individual level [74]. To target “individual variability” will ultimately require analyzing multiple biological pathways inexpensively, quickly, and sensitively. The increasing adoption of next generation sequencing in clinical practice has been recently driven by reducing costs and high-throughput analytical methods. In this setting, unbiased whole-genome sequencing (WGS) and whole-exome sequencing (WES) represent major milestones in the area of genomic medicine since they allow the complete elucidation of the genomic determinants of a specific AD patient’s heritable make-up, and thus are among the most comprehensive tools for future clinical applications [74, 75]. Moreover, upcoming commercially available genetic tests, e.g., gene-based assays, implementing polygenic risk scoring for assessing AD onset risk, are currently in late stage clinical development. In particular, a 90% maximum prediction accuracy via polygenic risk scoring can be accomplished by predictors of genetic risk based on genomic profiles [76]. It is generally acknowledged that an individual’s health, response to environmental and lifestyle factors, susceptibility to pathophysiology/syndromes/diseases, and tolerability/response to treatments are indeed impacted to a varying degree by their own unique biological (genetic/genomic/molecular) profile. Thanks to progress in the area of personal genomics, it is possible to identify the genetic/genomic predisposition of an individual for some common diseases, carrier status for inherited diseases, and adverse reactions to common drugs. Personal genomics provides support in predicting the likelihood that an individual will be affected by a disease and may help personalize drug selection and treatment delivery to get the best possible care, thus playing a key role in predictive and personalized medicine, in the framework of the PM paradigm [77]. In this regard, the 23andMe Personal Genome Service (PGS) Test (available at https://www.23andme.com/en-gb/) uses a qualitative in vitro molecular diagnostic system used for detecting variants in genomic DNA isolated from human adults specimens (saliva) that will provide information, i.e., delivering and interpreting genetic health risk (GHR) reports, to users about their genetic risk of developing a disease to inform lifestyle choices and/or conversations with a healthcare professional. Specifically, GHR reports have already been authorized by the FDA for late-onset AD and Parkinson’s disease and the following diseases: hereditary thrombophilia, alpha-1 antitrypsin deficiency, Gaucher disease, Factor XI deficiency, celiac disease, G6PD deficiency, hereditary hemochromatosis, and early-onset primary dystonia (available at https://www.accessdata.fda.gov/cdrh_docs/pdf16/DEN160026.pdf). Based on the gene expression profiles generated by GenomeDx Biosciences Decipher Genomics Resource Information Database (Decipher GRID®), a recent analysis showed that the genomic signature PAM50, normally applied to breast cancer patients to determine their risk of reappearance, can be used in prostate cancer as well for predicting which individual may take advantage from early initiation of post-operative androgen deprivation therapy, thus delivering a potential clinical tool to customize the treatment of prostate cancer. This personalized selection of patients will ameliorate treatment outcomes and prevent many patients from unnecessary risks of toxicity [78].
Differently from the invariable genetic/genomic information, an individual’s proteomics/peptidomics and metabolomics/lipidomics profile may be modified and vary over time. Figure 4 provides an up-to-date summary of currently available “omics” technologies (genomics, transcriptomics, miRNomics, proteomics, metabolomics) and how they can be used to disentangle different systems biomarker categories [79]. At present, the majority of the documented candidate biomarkers originate from genomic and proteomic disciplines. This might be due to the higher stability of the signal and standardization achieved by using genomic and proteomic tools compared to other available “omic” methodologies. In addition, the better stability of proteins versus mRNAs might account for the greater availability and progress in discovery and validation of proteomic markers compared to, e.g., transcriptomic approaches [79]. The appropriate interpretation of the obtained high-throughput data in the context of the disease molecular pathophysiology and its specific treatment is considered the rate-limiting step in the biomarker discovery and validation process. As a result, “omics” data sets need to be rigorously identified, extracted, and interpreted in order to deliver valuable biological information [79].
Fig.4
Overview of the currently available technologies and the resulting biological marker categories used for biomarker discovery in preclinical and clinical research. CNV, copy number variations; FISH, fluorescence in situ hybridization; GCMS, gas chromatography mass spectrometry; HPLC, high-performance liquid chromatography; LCMS, liquid chromatography– mass spectrometry; NMR, nuclear magnetic resonance; PCR, polymerase chain reaction; SNPs, single nucleotide polymorphisms; SVs, structural variations. Reproduced with permission from [79].
![Overview of the currently available technologies and the resulting biological marker categories used for biomarker discovery in preclinical and clinical research. CNV, copy number variations; FISH, fluorescence in situ hybridization; GCMS, gas chromatography mass spectrometry; HPLC, high-performance liquid chromatography; LCMS, liquid chromatography– mass spectrometry; NMR, nuclear magnetic resonance; PCR, polymerase chain reaction; SNPs, single nucleotide polymorphisms; SVs, structural variations. Reproduced with permission from [79].](https://ip.ios.semcs.net:443/media/jad/2018/64-s1/jad-64-s1-jad179932/jad-64-jad179932-g004.jpg)
Within the PM framework, it has been proposed to screen and detect unsuspected age-related neurodegenerative diseases as early as possible in cognitively healthy potentially preclinical affected adults. As far as AD is concerned, it has been hypothesized that such a screening program—based on WGS combined with whole-body magnetic resonance imaging (WB-MRI), metabolomics screening, constant heart monitoring, pedigree analysis, microbiome sequencing, and standard laboratory tests—could identify people at risk of developing clinical AD decades in advance. Controversies still exist, however, regarding both the high costs inherent to this approach and the potential risks of false-positive results and overdiagnosis [80].
Very recently, a pilot study has been conducted to investigate the impact of WGS in healthy subjects examined within a primary care context. Although several potentially pathogenetic variants were identified, only a fraction of the carriers demonstrated overt clinical signs or symptoms, indicating that the expected clinical phenotype would develop later during progression of pathophysiology. Although integrating genome sequencing and other sequencing methods into the day-to-day practice will undoubtedly provide unprecedented preventive opportunities, a careful sample size determination will be necessary for achieving a sufficient statistical power to detect a clinically meaningful effect size [81].
To aid PM fully coming to life in the field of ND, the interplay of “omics”-based techniques and sequencing methods is paramount, since the availability and increasing standardization of high-throughput big data will, through adequate IDM supported by advances in data science, allow creating new biomarker-guided targeted preventive and therapeutic opportunities [20, 21]. Therefore, the use of advanced sequencing methods and of “omics”-based screening of pathophysiological disease states is anticipated to result in enhanced personalized and precise, both preventive and therapeutic, interventions by disclosing accurate patterns of pathophysiological biomarkers and molecular signatures underlying the biological mechanisms progressing non-linear dynamic in specific disease states in individual patients [82]. Extensive efforts are presently performed to explicate gene-protein links, key molecular pathways functions, protein-protein and signaling network organization, and organism-level responses via high-throughput biological data at different time points (e.g., global gene expression and comprehensive proteomic data) [83].
In this context, it is important to note that, so far, a major obstacle to our understanding and to the development of possibly novel stratification approaches for AD is, as mentioned, the fragmentation of previous research (single-center, single-method studies). Neuroscience has been highly productive, but its progress can also be somewhat unsystematic and remote to clinical practice. That said, so far conventional “big data” analytics techniques have failed to provide the qualitative change which is indispensable to provide a mechanistic (and not only statistical) understanding of AD pathophysiology, which in turn is instrumental to formulating personalized treatment strategies. A first step, as mentioned above, is the integration of complex and high-dimensional information from hundreds or thousands of patients contained in “big data” repositories. However, this alone is not sufficient; “big data” need to be turned into “smart data” by injecting not only novel methodologies but also expert knowledge and targeted clinical hypotheses. This poses a major analytics challenge, as neither single national-level studies nor single biomedical or technical disciplines can tackle the problem on their own. A number of potentially disease-modifying clinical development programs in AD have failed so far [84], and in addition we are in serious need of novel out-of-the box preclinical models that can generate actionable knowledge, either in research or, eventually, therapy. This is why, while computational and statistical modeling are increasingly invaluable in AD research, it is necessary to go beyond purely descriptive data-analysis techniques (e.g., techniques that identify associations between certain data and phenotypes). Additional efforts are needed to inject specific domain competencies which can be formalized mathematically into predictive models that can disclose how specific components of pathogenic pathways interact within complex brain networks, across molecular to cellular and systems scales. Such predictive models should, as far as possible, include realistic representations of neurobiological processes and mechanisms that allow direct comparison to experimental settings and, ultimately, pave the way to discover new strategies for targeted control and intervention. In this respect, it is also essential to form additional private-public partnerships with a strong focus on data sharing and pathway-based analysis. With this type of integrative approach, successful real-world examples of advanced simulation have already generated tangible support for clinical trials in AD.
SYSTEMS BIOLOGY OF ALZHEIMER’S DISEASE
The polygenic multifactorial nature of AD and other complex proteinopathies of the brain with progression to ND is widely recognized. Although several mechanisms have been identified that may have a role in the pathogenesis of AD and other ND, the molecular and temporal dynamics of the biological processes that lead to onset and progression of diseases such as AD remain to be well-understood on a system level. Complex chronic diseases such as AD are thought to result from an interplay between environmental, genetic, and epigenetic factors. State-of-the-art “omics” techniques such as genomics, epigenomics, transcriptomics, proteomics, and metabolomics offer remarkable promise as research tools to decipher the dynamics and biological nature of the pathogenesis ultimately leading to neurodegeneration and a spectrum of clinical neurological phenotypes for which predictive markers and selective therapeutic tools are needed. Breakthrough advances in genetic and genomic technologies are making global genome sequencing possible, affordable, and clinically practical through advanced NGS technologies. New genetic technologies, however, provide a crucial basis to the understanding of the complex pathophysiological pathways involved in proteinopathies/ND.
The concept of complex multiscale systems (consisting of macromolecules that reciprocally interact with each other in dynamic modular complexes and networks) as the underlying foundations of life has been first proposed more than 50 years ago [85]. Over the past decades, we have gained detailed insights into the structure, regulation, and function of different molecular and cellular systems, which are currently viewed as building blocks or inventories of working parts. However, the main challenge ahead is to clarify how these single agents are reciprocally associated by multiple interactions across distinct system levels and networks of structural and functional organization (e.g., DNA-protein, RNA-protein, protein-protein, protein-metabolite networks, interactomics). Major challenges exist for the development of reliable holistic models that are based on unbiased data-integration workflows and that could highlight the properties of complex biological structures, for which the whole is often greater than the sum of their parts. In this context, the main goals of systems biology in the field of ND research are as follows: 1) to characterize complex systems and/or networks in a straightforward, viable manner, by probing key layers of molecular regulation and expression on a genome-wide level and 2) to integrate different genome-wide data sets in a multidimensional manner—that is, across different layers of molecular regulation, timescales, cell types and so on—in order to generate comprehensive in silico models of ND that show the best balance between coverage and selectivity, reduce model space down to manageable numbers of highly-prioritized testable hypotheses, and are biologically precise. This will shed more light on how complex diseases may be conceptualized as a result of altered networks states [86] caused by multifactorial perturbations, which is expected to foster marker and target discovery. Under this theoretical framework, the dynamics and biology of ND processes scrutinized by systems modeling and systems biology can be more comprehensively understood. This may be achieved via a two-step approach consisting of initial animal studies followed by confirmation and validation in clinical cohort programs [87] or via an approach consisting of molecular and clinical studies in cohorts, for example the search for predictive marker signatures, followed by studies in experimental models of ND of biological and therapeutic significance associated with such marker signatures. Numerous disease conditions in humans (including proteinopathies/ND, cardiovascular disorders, malignancies, the metabolic syndrome, and diabetes) have a highly complex biological nature that cannot be entirely and adequately captured through the investigation of single linear molecular alterations. Besides being multifactorial, such diseases are primarily caused by altered essential networks required for the correct functioning of basic physiological pathways. Such disease processes are fundamentally non-linear dynamic, being the results of an evolving interplay between homeostatic defense mechanisms and impaired physiological networks through space and time [88]. Since cell survival mechanisms under the control of stress response factors may also be those that trigger cell death depending on the pathophysiological context in which they operate [89] identifying the critical phases that, at the molecular, cellular, or system levels, are associated with the dynamics of ND processes and could modify the capacity of individuals to maintain function and resist ND is essential for clinical discovery and therapeutic developments, especially in the context of the growing needs for PM.
Recent years have witnessed significant advances in our understanding of how human diseases are routed in altered molecular and cellular networks. Several genetic alterations and pathophysiological mechanisms, mainly involving the amyloid-β protein precursor (AβPP) processing and tau related networks, are considered to be significant aspects in the pathogenesis of AD [90]. Such network derangements can cause either loss or gain of specific molecular functions and an increased formation of neurotoxic molecular species (e.g., toxic amyloid or protein aggregates) that can in turn adversely affect supra-cellular levels. Another important factor that should not be overlooked in the conceptualization of complex diseases is the crucial counteracting role of homeostatic networks. In this regard, the interest into the potential protective role of resilience factors against neurodegeneration (e.g., autophagy, proteostasis, endolysosomal networks, protein folding chaperone networks, disaggregates, and other stress-protective and clearance networks) is currently gaining momentum [90].
The causative pathways that lead to the onset of AD and its clinical phenotypes at the individual level are thought to consist of genetic/epigenetic susceptibility and/or protection coupled with a continuing dynamic interplay between altered brain networks and counteracting neural mechanisms of resilience. Integrative systems biology-based approaches are crucial to disentangling this intricate interplay. First, simple model organisms mimicking the main features of AD need to be developed in order to extensively apply different “omics” techniques. This approach may offer invaluable data to shed more light on the conserved pathways that modulate the onset and progression of AD, being ultimately useful for testing potential strategies that could delay and/or modify the natural course of disease [90]. However, the regulation of gene expression and pathway activity might differ between simple model organisms and humans, which calls for integrated use of simple model organisms and higher-order models such as mouse models and human cell models, e.g., induced-pluripotent-stem-cells coaxed into neurons or neurons obtained by direct conversion of fibroblasts [91].
New evidence from preclinical models needs to be duly replicated, with a special focus on subtle initial network alterations that can be visualized by neuroimaging, which could potentially become the targets of early therapeutic interventions [92–95]. Neuroimaging and biomarker data should be fully integrated and analyzed in a longitudinal manner through computational and integrative network biology tools within a systems biology-based framework. The increasing trend toward high-throughput techniques in AD research will generate multifactorial data that will require integration in a standardized, efficient, cost-effective, and secure manner. The vast amount of data generated will cause new challenges for data science, mainly in terms of data storage, processing, and mining. As we are entering into the “era of big and deep data” in AD, computational systems biology approaches are continuously being optimized in order to support the approximate modeling of biological systems [90].
A holistic systems biology-based research strategy in AD research will likely rely on generating large and rich data sets, applying multi-layer network approaches for integration and comparative assessments of different datasets, and reckoning on the information generated for discovery of novel disease markers and targets. A translational approach from preclinical studies to bedside (complemented by reverse translational approaches) will be required to integrate and implement fundamental aspects of the systems theory and the systems biology concept into clinical practice, i.e., translational systems medicine, in the upcoming future [96–99]. Key to the success of these approaches is the use of robust data integration methods. There is a large array of methods that enable complex data sets collected in experimental models of ND or human cohorts to be analyzed and integrated on a system level [100, 101]. Methods based on graph theory (that is network approaches) such as spectral decomposition of the signal [102] weighted gene co-expression network analysis [103] and Bayesian causal inference [104] and those based on formal concept analysis [105] and tree induction [106, 107] likely hold strong promises for generating comprehensive in silico models that accurately select for biological rules, disease targets, and risk factors with potential for clinical exploitation.
APPLICATION OF SYSTEMS BIOLOGY IN AD COHORTS: THE EXAMPLE OF THE EUROPEAN PREVENTION OF ALZHEIMER’S DEMENTIA (EPAD) CONSORTIUM
Implementation of systems biology into clinical and research practice requires a number of steps. First, molecular tests and biomarkers for matching individuals/patients to clinical trials and/or targeted therapies will require continuous refinements and validation of high-throughput techniques, systems-level approaches, and computational tools. Second, all molecular tests to be used for AD, as well as all patient care-related molecular analyses, need to be performed using assays that are highly reproducible, accurate, and satisfy the FDA clinical trials guidelines, with adherence to principles of Good Clinical Practice (GCP) (available at http://www.fda.gov/regulatoryinformation/guidances/ucm122046.htm), the European Medicines Agency (EMA) (http://www.ema.europa.eu/ema/), and the European Clinical Trials Database (EudraCT) (https://eudract.ema.europa.eu/). In this scenario, the Alzheimer’s disease neuroimaging initiative (ADNI) and the Dominantly Inherited Alzheimer Network (DIAN) will provide collaborative large-scale longitudinal data on AD associated autosomal dominant mutation carriers that will be invaluable to systematize and make explicit the translation of neuroimaging and biochemical markers into clinical guidelines. Third, the era of big and deep data generation and the availability of comprehensive repositories has brought the need for collaboration, sharing, integration, normalization, and analysis of both data and metadata, with the ultimate goal to make effective translational use of this new knowledge. In this scenario, several clinical trials may benefit from the holistic approach provided by systems biology. Among them, interest in the European Prevention of Alzheimer’s Dementia (EPAD) program is gaining momentum.
The EPAD program [108] is a pan-European initiative that will establish a shared platform to design and conduct phase II Proof-of-Concept (PoC) clinical trials specifically aimed at developing new treatments for the secondary prevention of AD. To investigate different agents in the pre-AD population in the most efficient manner, a Bayesian adaptive design that learns from data accrued as the trial progresses will be used. Clearly disappointing results of recently completed phase III AD therapy trials may be explained by their exploratory (rather than confirmatory) nature, mostly caused by an incomplete exploration phase throughout phase II [109]. Hopefully, the EPAD program will be helpful to overcome previous pitfalls in the field by assuming that a correctly designed phase II trial can take several years to be completed. Other common issues that the EPAD Longitudinal Cohort Study (LCS) (available at https://clinicaltrials.gov/ct2/show/NCT02804789) will address include: 1) the high screen failure rates, 2) the unwillingness or inability to implement an adequate patient stratification, and 3) the lack of a pre-randomization run-in period. The EPAD LCS is expected to provide reliable disease models of the preclinical and prodromal periods of AD before the final implementation of a clinical trial. The EPAD LCS will be conducted in a large cohort of 5,000 subjects who had undergone a thorough assessment in terms of cognition [110, 111], neuroimaging, core CSF biomarkers (Aβ42, total tau [t-tau], and hyperphosphorylated tau [p-tau]), clinical outcomes, and genotyping. Annual assessments will be performed with the goal of identifying different disease trajectories to provide an optimal stratification for trial inclusion. Risk stratification groups with similar biological underpinnings will be helpful to identify specific classes of subjects to be included (or excluded) from the clinical trial according to the PM paradigm.
The development of an EPAD site network across the European Trial Delivery Centers will be critical to the initiative success. Site certifications, continuing training, and commitment to the EPAD program is expected to reduce study site heterogeneity and will hopefully provide highly accurate estimates of treatment effects. Each TDC will assess approximately 200 research participants, of whom 100 will be included in the clinical trial. This effort is unprecedented, as previous clinical trials involved numerous centers (up to 200), each enrolling a handful of patients. Conversely, the traditional methodology will be overturned by EPAD, inasmuch as a few centers will enroll numerous patients.
In general, the correct implementation of phase III trials preliminary requires more robust phase II outcomes. The EPAD program will improve the study methodology, ultimately favoring an optimal disease modeling and a better patient stratification before embarking on phase III confirmatory trials. The EPAD LCS was started in May 2016 at six sites, with a total of 400 participants having already been recruited. Disease modeling work is expected to be introduced as soon as an enrolment goal of 500 subjects will be achieved. It is anticipated that the EPAD PoC Study Platform trial will begin in 2018.
SYSTEMS NEUROPHYSIOLOGY OF ALZHEIMER’S DISEASE: UNDERSTANDING NEUROPHYSIOLOGY AND NEURODYNAMICS BEHIND ETIOLOGY
During the last two decades, the neuroscience field has entered a rapid phase of expansion characterized by the development of a large proportion of methodologies allowing the recording of neural data obtained from a wide range of modalities, from metabolic pathways to optical imaging to functional magnetic resonance imaging (fMRI). These data are collected through different spatiotemporal domains (Fig. 5). Most of these techniques have been so far used one at a time [112, 113]. Recently, there is an attempt toward data integration in order to create comprehensive maps and record dynamic patterns across multiple levels of organization (neurons, circuits, systems, whole brain) and involving different domains of biology and data types (such as anatomical and functional connectivity, genetic/genomic patterns [112, 114]). This effort is in line with the new paradigm of systems neurophysiology aiming at integrating “big neuroscience data” recorded in a multimodal fashion to understand the role of the complex web of interconnections among several elements of large-scale neurobiological systems [115–118]. The ultimate goal of systems neurophysiology is to clarify how signals are represented within neocortical networks and the specific roles played by the multitude of the heterogeneous neuronal components. The new interdisciplinary field of network neuroscience proposes to overcome these enduring challenges by approaching brain structures and functions via an explicitly integrative perspective [112]. Here, we will present scientific advancements related to single methodologies utilized by system neurophysiology, within wider context of the PM paradigm in AD.
Fig.5
Systems neurophysiology and network neuroscience: schematic representation of how structural levels within the nervous system integrate over multiple spatial and temporal scales. Network neuroscience encompasses the study of very different networks encountered across many spatial and temporal scales; however, the network ideas clearly extend down to the level of neuronal circuits and populations, individual neurons and synapses, as well as genetic regulatory and protein interaction networks. In network neuroscience and systems neurophysiology in general, the overall aim is to bridge information encoded in the relationships between genes and biomolecules to the information shared between neurons across to the brain level while integrating the additional information provided from the time dimension. This could eventually allow access to mechanistic understanding and models which faithfully reproduce and possibly predict both brain structure and function. Interestingly, above the single brain level, the social network level should still be considered a network neuroscience domain and, albeit with different measurement techniques, can be studied with the same paradigms with the aim to understand the larger “brain” that interacting brains give rise to (i.e., economies and cultures). Adapted from [112] and [609].
![Systems neurophysiology and network neuroscience: schematic representation of how structural levels within the nervous system integrate over multiple spatial and temporal scales. Network neuroscience encompasses the study of very different networks encountered across many spatial and temporal scales; however, the network ideas clearly extend down to the level of neuronal circuits and populations, individual neurons and synapses, as well as genetic regulatory and protein interaction networks. In network neuroscience and systems neurophysiology in general, the overall aim is to bridge information encoded in the relationships between genes and biomolecules to the information shared between neurons across to the brain level while integrating the additional information provided from the time dimension. This could eventually allow access to mechanistic understanding and models which faithfully reproduce and possibly predict both brain structure and function. Interestingly, above the single brain level, the social network level should still be considered a network neuroscience domain and, albeit with different measurement techniques, can be studied with the same paradigms with the aim to understand the larger “brain” that interacting brains give rise to (i.e., economies and cultures). Adapted from [112] and [609].](https://ip.ios.semcs.net:443/media/jad/2018/64-s1/jad-64-s1-jad179932/jad-64-jad179932-g005.jpg)
An increasingly important integrative component in this endeavor is connectomics the emerging science of brain networks, which comprises studies of both anatomical and functional brain connectivity, across modalities and methodologies. The rise of connectomics has triggered several national and international consortia devoted to mapping patterns of brain connectivity across large subject cohorts, including the Human Connectome Project funded by the U.S. National Institutes of Health [119]. These projects have pushed the boundaries of data sharing, neuroinformatics and computational analysis. Similar connectomics efforts are underway to track lifespan development [120] as well as address patient populations, including people with ND. To deal with the mounting volume of connectome data, the field is developing basic network science tools and methodology that can be applied to brain data [121]. So far, broad exploratory analysis has revealed a number of architectural principles that underpin macro- and meso-scale maps of brain connectivity, including modular organization and the existence of prominent hub regions. Much is still to be learned about the contributions of connectome architecture to human brain function and its role in pathophysiological processes. Systems neurophysiology in combination with connectomics and computational network models has great promise to illuminate the relation of structure to dynamics in brain networks as shown, for example, in recent findings on time-dependent functional connectivity as measured with non-invasive neuroimaging techniques.
CONTRIBUTION AND ROLE OF STRUCTURAL MAGNETIC RESONANCE IMAGING
Magnetic resonance imaging (MRI) is a widely, non-invasive, relatively non-expensive and versatile technology. Among MRI modalities, structural or anatomical MRI, using three-dimensional T1-weighted sequences, is the most widely used [122, 123] and validated [124, 125]. Structural MRI allows visualization and measurement of atrophy which is a macroscopic correlate of neurodegeneration, in particular of neuronal and dendritic loss. The progression of atrophy in AD approximately follows that of neurofibrillary tangles found in postmortem AD cases and described by Braak and colleagues [126] and Delacourte and colleagues [127]. Moreover, previous studies showed that structural MRI alterations correlate with tau deposition, as described by Braak stages, and CSF tau biomarkers [128]. On the contrary, not all structural MRI measures are well correlated to measures of Aβ deposition, and atrophy patterns do not follow those of amyloid deposition [129, 130]. Due to these reasons, it should be noted that brain atrophy in AD is descriptive of brain structural changes but not specific for underlying AD pathophysiology. Indeed, a given atrophy pattern can be associated with different pathophysiological processes. However, MRI atrophy measures are well correlated with cognitive and clinical functions [131, 132], and highly correlated with the concurrent rate of clinical decline [133–135]. Therefore, they constitute attractive tools to track disease progression and to monitor the effect of treatment.
Automated image analysis approaches allow measuring distributed patterns of atrophy across the whole brain, using either region-of-interest measurements, voxel-based maps of gray-matter or cortical thickness measurements [136, 137]. Machine learning algorithms applied to whole-brain atrophy maps can automatically identify patients with AD and thereby support diagnosis [138–141].
The most widely studied and accepted structural MRI marker of AD is atrophy of the medial temporal lobe [142, 143]. Assessment of medial temporal atrophy can be performed in clinical routine using visual scales [144]. However, such approach is observer-dependent and only semi-quantitative. On the other hand, fully-automated segmentation approaches provide objective, quantitative, volumetric measurement of hippocampal atrophy [145–149]. Hippocampal volumetry can discriminate AD patients from controls with high sensitivity and specificity [150]. Moreover, numerous studies have shown that patients with higher hippocampal atrophy are at higher risk of rapid cognitive decline [151–155]. However, atrophy of the hippocampus was found in other types of dementia, suggesting low specificity of this marker for the identification of AD [156, 157]. Recent developments of ultra-high field MRI (7 Tesla and higher) allow the study of anatomical alterations with an unprecedented level of detail. In particular, using 7T MRI, it is possible to distinguish between different cellular layers and anatomical subregions within the hippocampus. Its application in AD has demonstrated that hippocampal subregions and layers are differentially affected by atrophy [158, 159]. These advanced techniques have the potential to provide more sensitive measures than global hippocampal volumetry.
Another region of interest for AD is the basal forebrain cholinergic system (BFCS) since it represents the region with the majority of cholinergic nuclei efferent to the cerebral cortex [160, 161]. The measurement of BFCS nuclei has been developed and validated as a highly relevant and robust region of interest for automatic structural MRI assessment of atrophy rate of change from the preclinical to the clinical AD stages [160, 162–167]. Evidence indicates that the BFCS may even degenerate before medio-temporal lobe structures, as early as at the preclinical stage [163, 168]. In contrast to the hippocampal volume, the atrophy of BFCS was significantly correlated to in vivo brain amyloid load in AD and non-demented elderly individuals [169, 170]. Machine learning approaches based on whole brain atrophy patterns have been developed to predict the evolution of patients, in particular the progression to dementia of individuals with mild cognitive impairment (MCI) [171–173]. Nevertheless, most of these approaches have been validated on a single research dataset, most often provided by the ADNI. Therefore, their ability to generalize across datasets as well as their performance in a clinical routine context remain unclear and larger-scale validation studies are needed.
Its ability to track progression makes structural MRI also attractive to monitor the effect of treatment [29]. Of all outcome measures (including clinical, cognitive, and fluid biomarkers), structural MRI measures seem to have the highest measurement precision [135]. They are thus an attractive outcome measure for clinical trials, as well as to monitor the effect of treatment in a clinical context. It should be noted that different types of treatment seem to result in different effects on atrophy measures. In a randomized placebo-controlled trial, patients treated with donepezil, an acetylcholinesterase inhibitor, have a significantly lower rate of annual hippocampal atrophy and cortical thickness compared to those receiving placebo [174, 175]. Moreover, the treatment group demonstrated a significantly decreased annual rate of atrophy of the BFCS compared to MCI individuals that received placebo [176]. The BFCS complements hippocampal volumetry in assessing structural progression in AD and provides a promising outcome measure for clinical trials. Anti-amyloid therapies, however, seem to result in increased rate of atrophy [177]. Nevertheless, it may be hypothesized that such accelerated atrophy only occurs at the beginning of treatment, perhaps caused by a reduction in microglial activation associated with plaques, and that a reduction of atrophy may occur in the longer term. Overall, structural MRI remains an attractive tool to study the morphological effects of treatment, in particular if new molecules targeting other aspects of AD pathophysiology (e.g., anti-tau or neuroprotective treatments) become available. Furthermore, structural MRI plays an important role in monitoring safety of treatments. Indeed, microbleeds and transient cerebral edema (respectively called ARIAH and ARIAE) occur in some patients treated with active Aβ immunization [178].
In summary, structural MRI is an attractive marker for tailoring therapeutic interventions. Its most attractive features are its ability to precisely track cognitive decline, its potential for monitory the effect of treatment and to predict the evolution of patients. For prediction, the most promising avenue is that of machine learning approaches from whole-brain measurements. Such approaches require larger scale validation using multiple clinical routine cohorts. The integration of structural MRI analysis tools with other techniques such as those from functional MRI, electroencephalography (EEG), magnetoencephalography (MEG) or diffusion tensor imaging (DTI), in a multimodal fashion, will enable the investigation of temporal and topographical relationships between numerous pathological alterations and neurobiological systems related to AD. Such big data integration, will improve our understanding of the in vivo interacting pathophysiological mechanisms across brain related systems characterizing AD, as envisioned by the PM concept.
CONTRIBUTION AND ROLE OF DIFFUSION TENSOR IMAGING
Diffusion tensor imaging (DTI), which employs a Gaussian approximation to model the MR signal attenuation due to net water molecule displacements in a de facto restricted cellular environment. This technique has become the mainstream strategy for examining white matter (WM) microarchitecture, connectivity as well as integrity both in an investigative and in a clinical setting, and it has been widely employed in studies focused on AD and MCI [179–181] as well as several other pathologies [182–185]. The apparent water diffusion tensor (which is termed apparent precisely because intracellular water diffusion is not truly free) can be estimated in brain parenchyma based on relatively fast echo planar imaging (EPI) techniques [186] which only pose moderate demand in terms of in-scanner subject time. From these tensor estimates, WM tract-specific orientation information can be obtained through deterministic (based on the orientation of the main DT eigenvector) or probabilistic approaches [187]. Also, model free tractography approaches exist, a promising development of which is constrained spherical deconvolution [188–191], which has lately been extended to incorporate multi-tissue models anatomically based filtering [188, 189] (Fig. 6). Further, scalar indices derived from the diffusion tensor are rotationally invariant and are well known to be sensitive, albeit not specific, indicators of microstructural alterations. The single tensor eigenvalues as well as mean diffusivity (MD – mean of eigenvalues) and fractional anisotropy (FA – normalized variance of eigenvalues [192]) can aid in quantifying fiber integrity through region of interest (ROI), voxel- or Tract-Based Spatial Statistics based approaches [180]. A decrease in FA (possibly accompanied by an increase of MD or other directional diffusivities) is typically the hallmark of unspecific bundle degeneration, as seen in AD and MCI [193, 194]. Importantly, correlations between DTI-derived indices in WM and AD disease severity have been reported [195, 196], suggesting that DTI measures may be used as indexes of disease progression. DTI may therefore provide unique information about WM integrity [66] in AD patients and MCI subjects. Indeed, several studies have demonstrated early WM changes within the parahippocampus, hippocampus, posterior cingulum, and splenium already at the MCI stage [197–200]. However, the majority of DTI studies indicate that the uncinate fasciculus, the entire corpus callosum and the cingulum tract are most involved in pathogenesis in both MCI and AD. In a recent study on AD and MCI subjects [201] the interpretation of a selective increase in FA in the MCI group was aided by the introduction tensor mode (MO) [202], a third invariant which distinguishes the type of anisotropy (planar, e.g., in regions of crossing or kissing fibers versus linear, in regions which exhibit one predominant orientation). This, in turn, led to the detection of a relative preservation of motor-related projection fibers crossing the association fibers of the superior longitudinal fasciculus in the early-stage MCI subjects before they degenerated to AD. Also, recent DTI data seems to point toward a reconstruction of the trajectory of progressive WM degeneration in AD as it spreads with aging. In agreement with this so called retrogenesis model (cortical regions that mature earliest in infancy tend to degenerate last in AD) it has been shown that WM abnormalities in specific brain regions such as prefrontal cortex WM, inferior longitudinal fasciculus, and temporo-parietal areas [180, 197, 203, 204] appear earlier. Also, DTI has been able to offer insight into asymptomatic “preclinical” at risk stages such as subjective cognitive decline, where DTI based scalar markers of diffusion properties were significantly associated with rates of cognitive decline and hippocampus atrophy at clinical follow up, with odds ratios up to 3 [205], and DTI indexes invariants were seen to be more sensitive than CSF biomarkers in predicting cognitive decline and medial temporal atrophy in subjective cognitive decline and MCI subjects [205].
Fig.6
Sagittal slab visualization of a fiber tractogram obtained from WM fODFs estimated with SSST-CSD (left) and MSMT-CSD (right) with different fODF amplitude thresholds (top, bottom). fODF, fiber orientation distribution function; MSMT-CSD, multi-shell, multi-tissue constrained spherical deconvolution; SSST-CSD, state-of-the-art single-shell, single-tissue constrained spherical deconvolution; WM, white matter. Reproduced with permission from [188].
![Sagittal slab visualization of a fiber tractogram obtained from WM fODFs estimated with SSST-CSD (left) and MSMT-CSD (right) with different fODF amplitude thresholds (top, bottom). fODF, fiber orientation distribution function; MSMT-CSD, multi-shell, multi-tissue constrained spherical deconvolution; SSST-CSD, state-of-the-art single-shell, single-tissue constrained spherical deconvolution; WM, white matter. Reproduced with permission from [188].](https://ip.ios.semcs.net:443/media/jad/2018/64-s1/jad-64-s1-jad179932/jad-64-jad179932-g006.jpg)
Nevertheless, a recent meta-analysis indicates high variability in both the anatomy of regions studied and DTI-derived metrics [206], a partial contribution to which may be the intrinsic limits of the DTI techniques. Determining the most robust acquisition parameters and processing strategies for DTI for a multicenter setting is still an active area of research, and initial clinical and physical phantom data, i.e., scans obtained from a volunteer as well as a physical object with defined diffusion properties, suggest that the variability of DTI-based diffusion metrics across a range of MRI scanners is at least 50% higher than that of volumetric measures [207]. For prediction of conversion from MCI into AD dementia, DTI reached an accuracy of about 77% – 95% at 2 to 3 years follow up [205, 208, 209] in monocenter studies, prediction accuracy for multicenter studies still needs to be studied. Also, all diffusion weighted imaging protocols suffer from the relatively low signal-to-noise ratio inherent in the necessarily fast EPI techniques. In this respect, the increase in signal-to-noise ratio afforded by moving to ultra-high field imaging (at, e.g., 7T) is somewhat counteracted by the rapid shortening of transverse (T2) relaxation times with increasing field strength and consequent signal loss. Nevertheless, while ultra-high field diffusion weighted imaging therefore poses significant challenges, improved distortion correction techniques [210] coupled with monopolar acquisition schemes which allow a significant (about 30%) shortening of echo times, and the additional use of simultaneous multislice excitation strategies [211] may allow in vivo diffusion-weighted imaging to finally advance toward sub-millimeter imaging at ultra-high field. Accordingly, ex-vivo studies have already defined WM lesions in aging and AD at 11.4T [212], and 7T imaging has been helpful in discriminating Parkinson’s disease [213] and amyotrophic lateral sclerosis [214]. Finally, it is well known that the assumption of a Gaussian propagator (which is at the root of DTI) is insufficient in regions with more intricate fiber architecture such as mixed tissue types and/or kissing or crossing fibers [215]. To this end, more advanced protocols such as Diffusion Spectrum Imaging [216], Diffusional Kurtosis Imaging [217–221], higher order tensor models [222], compartment models [223–225], and anomalous diffusion [226, 227], which can be optimized in order to enhance their suitability in a clinical setting [228], have been already been successfully employed in augmenting information about tissue degeneration in several ND, including AD [229–232].
Another avenue for DTI-based methodology is the construction and subsequent analysis of brain-wide maps of anatomical connections that can be summarized as structural networks or graphs [115]. Basically, these efforts proceed by first dividing the brain into a set of internally coherent gray matter parcels or regions (the nodes of the network) and then estimating the strengths of anatomical projections between these nodes (the edges of the network). While the reconstruction of such maps faces significant methodological issues, the resulting structural networks have been validated against classical histological techniques in non-human species. Human structural networks capture individual differences that relate to genetics [233] and various phenotypic variables, including indices of cognitive performance [234]. They also exhibit characteristic changes across the lifespan [120], during normal aging [235], and in the course of brain disorders [236]. For example, the loss of connectivity associated with the progression of AD results a loss of links between dense clusters of functionally-related regions and hence a decreased capacity for integration [237, 238].
CONTRIBUTION AND ROLE OF FUNCTIONAL MAGNETIC RESONANCE IMAGING
Using fMRI in a PM-based paradigm to tailoring therapeutics for patient treatment would be a very innovative approach from current methods to developing therapeutics for patients. The diagnosis and classification of patients would be based on clinical criteria, where a patient would be classified according to predetermined criteria. Implementation of a PM paradigm would use fMRI as a biomarker of functional brain changes that would be part of defining the patient’s phenotype in combination with the other modalities. Thus, it would seek to integrate fMRI-based biomarkers within a systems neurophysiology context to provide an integrated picture of the patient’s status [21]. The biomarkers within a systems neurophysiology approach would inform the treatment approach that a patient would receive. Given the complexity of AD and the other ND, the fMRI-based biomarkers would be integrated within a systems biology and neurophysiology approach with the other modalities (genetic, clinical, behavioral, cognitive, etc.) where the different biomarkers would reflect disease mechanisms, pathophysiology, clinical history and permit patient stratification for treatment [20, 21].
fMRI can be used to measure the vascular response to local neuronal activation due to stimuli or a cognitive task [239]. There are two broad approaches that may be utilized with fMRI data in defining PM-based biomarkers for AD detection and diagnosis—one would examine brain activation data in response to a stimulus or cognitive paradigm whereas another approach would examine the intrinsic connectivity networks measured using resting state fMRI. The first approach would lead to biomarkers that would be associated with the cognitive paradigm or stimulus class whereas examination of the intrinsic connectivity networks would provide a search for biomarkers over all brain networks.
In terms of a PM approach with tailoring therapeutics, the use of a cognitive task or stimulus would be a form of ‘stress test’ to a specific network, for example in asymptomatic at risk stages for preclinical and clinical AD, a memory task would typically activate the hippocampus, ventral- and dorsal-prefrontal regions, posterior cingulate regions [240–249], and a working memory task would primarily activate dorsal and ventral frontal regions and inferior and superior parietal regions [250–255]. A limitation of the cognitive paradigm approach is that the patient must be able to perform the task, and variability in task performance would alter the activation pattern [256–261]. An alternative approach in AD would be to implement cognitive paradigms outside of the memory domain that individuals may still be able to perform such as visual perception, attentional tasks, or passive stimuli [262–273]. The changes found using this approach would be applicable to patients that may be clinically more advanced, but also provides an approach to measure the ‘downstream’ effects of the pattern of disease-related neuropathology. Current studies examined the differences between patients and healthy controls or among different risk groups by quantifying the average difference between the groups, where the groups are defined by clinical-descriptive phenotypes or risk groups based on genetics or family history. The proposed PM paradigm would instead examine the variability among the subjects to define phenotypes that are data-driven and may not necessarily reflect the underlying pathophysiology and clinical phenotypes. There is evidence of significant variability in brain activation from healthy status to MCI to mild AD stage, for example a using a face-name association paradigm, there was a nonlinear response in hippocampus, with higher activation in MCI subjects compared to healthy controls and AD dementia patients [242, 249, 274]. Similarly with the visual perception task the activation levels varied along the dorsal visual pathway as disease severity increased [262].
In addition to measuring brain function, one would need to integrate the above biomarkers with results from fMRI studies of the mechanisms of action of the potential therapeutics; most studies have examined cholinergic drugs over an extended treatment period in either MCI subjects or mild AD patients (see for example [273, 275–278]). Another potential approach to be used within a PM paradigm is to measure the effects of a single dose [279–282] and investigate the predictive power of the single dose over the effectiveness of the therapeutic strategy for the biomarkers-characterized patient. The single dose approach has the potential to inform the tailoring of the therapeutic intervention by providing information about potential medium to long term effects of any treatment.
The various fMRI-based paradigms described above would provide information about a specific brain network or set of brain regions and any data-driven approach would be limited to data from the brain network or regions activated during the task. An alternative approach utilizing fMRI would be to use whole-brain resting state fMRI to measure so-called resting-state networks or intrinsic connectivity networks (ICNs) [283–286]. These ICNs have been shown to be highly reproducible across individuals [287], exhibit characteristic dynamic fluctuations [288] as well as patterns of change across development, life span and in the course of brain disorders [236]. The topography of ICNs resembles other networks, such as those engaged during human behavior and cognition (for example, see [289–293]), derived from gene co-expression [294, 295], disease phenotypes and disease progression (for example [246, 296–303]), as well as brain activation level and cognitive performance (for example [293, 304–306]). The structure of ICN networks can be probed with a variety of network tools to reveal individual differences in their internal coherence and their mutual interactions. In combination with these advanced analytics, ICNs can potentially provide a rich set of biomarkers of brain function, including insights into which ICNs are specifically disturbed as a result of pathophysiology, and thus yield a more integrated perspective on system-wide changes within a patient. The tailoring of therapeutics could benefit from associations between biomarkers and the presence of the disease pathophysiology. Given the variability that is present in AD patients and MCI subjects, the ICN-based biomarkers and their relation to genetic profiles [68] may be able to provide an improved systems biology characterization of brain function. The use of ICNs for tailoring therapeutics still needs considerable development work, and there is currently only limited work on the effects of an AD-related drug on ICNs [307]. It should be noted that while the task-free design of resting fMRI lends itself to application in clinical cohorts, the sensitivity to motion artifacts and ongoing temporal fluctuations in the network structure of ICNs entail greater reproducibility as scan lengths are increased (for example, see [308]).
The potential of fMRI to assist in the PM-oriented targeting of therapeutics for AD patients is strong but also will require very significant development work. The integration of fMRI with the other domains such as genetics, cognition, clinical measures has so far mostly been attempted within a group analysis context, and a PM paradigm would need development of new statistical models to define potential therapeutic strategy on a single individuals basis [309].
CONTRIBUTION AND ROLE OF ELECTROENCEPHALOGRAPHY
Candidate topographic neurophysiological (neurodynamic) biomarkers of AD can be derived from resting state eyes-closed electroencephalographic (rsEEG) rhythms recorded in subjects relaxed in quiet wakefulness (eyes closed, no sleep) with their mind freely wandering [310]. These rsEEG markers are non-invasive, cost-effective, available worldwide, and repeatable even in severe dementia. They may probe the neurophysiological “reserve” in AD patients, as one of the dimensions of the brain reserve [311]. This neurophysiological “reserve” may reflect residual mechanisms for 1) “synchronization” of neural activity in a given cortical region and 2) the coupling of activity between nodes of a given brain neural networks as a sign of functional cortical “connectivity” [310, 312].
RsEEG markers in AD at the group level reflect the neurophysiological reserve of the disease over time and after cholinergic therapy
Previous rsEEG studies using “synchronization” markers showed that compared with groups of normal elderly (Nold) subjects, AD groups with dementia (ADD) exhibited lower power density in posterior cortical alpha (8–12 Hz) and beta (13–30 Hz) rhythms [313–319]. There was also higher power density in widespread delta (<4 Hz) and theta (4–7 Hz) rhythms [320–325]. Finally, ADD, dementia due to Parkinson’s (PDD), and dementia with Lewy bodies (DLB) groups were characterized by abnormally lower posterior alpha source activities [326]. The effect was dramatic in the ADD, marked in the DLB, and moderate in the PDD [326]. There were also abnormally higher occipital delta source activities with dramatic effects in the PDD group, marked in the DLB group, and moderate in the ADD group [326].
Concerning “connectivity” markers, ADD groups were characterized by abnormally lower spectral coherence in alpha and beta (13–20 Hz) rhythms between posterior electrode pairs [316, 327–339]. These effects were observed in temporo-parieto-occipital electrode pairs in some studies [316, 327, 333, 337] and in frontocentral electrode pairs in others [329, 332, 340]. Other studies reported either a global decrease [327, 334] or increase [337, 341] of delta and theta coherences between electrode pairs in ADD groups. Another investigation pointed to a complex topographical pattern of coherence increase and a decrease in those groups [342]. Alternative techniques of “connectivity” unveiled a decrement of synchronization likelihood between electrode pairs in frontoparietal alpha rhythms in ADD and its prodromal stage of amnesic MCI [319, 343]. Finally, there were reduced cortical connectivity and “small-worldness” in ADD groups as revealed by graph theory indexes [344–347].
RsEEG rhythms deteriorate across time (e.g., about 12–24 months) in groups of aMCI subjects and ADD patients (see for a review [348]): 1) increased delta-theta and increased alpha-beta power density at parieto-occipital electrodes [349]; 2) increased theta power density, decreased beta power density, and decreased mean frequency at the temporal and temporo-occipital electrodes [316, 350, 351]; 3) increased delta and increased alpha 1 in parieto-occipital sources [352, 353]; and 4) reduced cortical connectivity as revealed by graph theory indexes [347].
In groups of ADD patients, acetylcholinesterase inhibitor drugs (i.e., enhancing the cholinergic tone) showed beneficial or protective effects in delta [320, 354–356], theta [321, 356, 357], and alpha rhythms [355, 358]. When observed at short-term, these effects predicted longer-term therapy efficacy [357, 359, 360] (for a review, see [352]). However, some contradictory findings suggest future more controlled cross-validation studies [361, 362].
Abnormal posterior cortical delta rhythms in ADD patients might reflect an upregulation of their generation mechanisms in quiet wakefulness, possibly due to cortical blood hypoperfusion and synaptic dysfunction in the same regions [363–366] and atrophy in the posterior cortex [312, 352, 367–369]. Furthermore, reduced posterior cortical alpha rhythms in ADD subjects might be due to an unselective tonic cortical excitation in populations of cortical pyramidal, thalamo-cortical, and reticular thalamic neurons generating those rhythms [370–372]. Such cortical over excitation might induce a background noise in the neural information processing interfering with vigilance and cognition [310].
RsEEG markers in AD at the individual level: Classification accuracy and predictions
RsEEG markers allowed the discrimination of ADD patients from Nold individuals and others with neurodegenerative dementing disorders such as PDD and DLB persons. Global delta and alpha coherences between electrode pairs successfully classified ADD compared with DLB people with 0.75–0.80 (e.g., 1 = 100%; [373]). Furthermore, twenty discriminant scalp rsEEG power density and coherence variables showed a classification accuracy of 0.90 in the discrimination of ADD versus Nold and ADD versus PDD subjects [374]. Another study in small populations of ADD, PDD/DLB, and frontotemporal dementia (FTD) patients reached a classification accuracy of 1.0 using 25 discriminant scalp rsEEG power density and functional cortical connectivity (i.e., Granger causality) variables [375]. In another study, combining quantitative rsEEG variables (including those of functional cortical connectivity) with neuropsychological, clinical, neuroimaging, cerebrospinal fluid, and visual EEG data reached “only” a classification accuracy of 0.87 in the discrimination between ADD, PDD, and DLB persons [376]. Concerning cortical source space, resting state delta and alpha sources classified Nold subjects versus ADD/DLB/PDD patients and ADD versus PDD patients with 0.85–0.90 [326]. Milder classification effects were observed in PDD and ADD individuals with MCI [377]. RsEEG markers predicted cognitive decline in aMCI individuals at about 6–24 months (see [348] for a review). The main effects are summarized as follows: 1) combined alpha-theta power density and mean frequency from left temporal-occipital regions [316]; 2) anterior localization of alpha sources [315]; 3) high temporal delta sources [378]; 4) high theta power density [379]; and 5) low posterior alpha power density [380].
Concluding remarks on EEG implementation
Overall, it is suggested that resting state cortical delta and alpha rhythms might unveil more compromised neurophysiological reserve in AD, at the group and the individual level. These rsEEG markers predicted and tracked the AD progression as neurophysiological endpoints for therapeutic interventions. Future multi-centric longitudinal studies should provide a large open access database for a systematic comparison of rsEEG markers of “synchronization” and “connectivity” markers for a better definition of “neurophysiological reserve” for clinical applications and research.
CONTRIBUTION AND ROLE OF MAGNETOENCEPHALOGRAPHY
Magnetoencephalography (MEG) allows recording the magnetic signals of the order of 10–12 Teslas, which are produced at the scalp surface by the activity of neuronal assemblies. It may provide information complementary to EEG for uncovering new neurodynamic biomarkers of AD, particularly in its very early asymptomatic at risk and preclinical stages, therefore before the prodromal and clinical stages.
MEG can be used to investigate cognitive functions in a way very similar to EEG. With this approach, impaired brain functional activities were characterized in AD and MCI stages during memory tasks for instance. Walla and colleagues [381] used a recognition memory task in which they manipulated the depth of encoding of verbal information. They showed alteration of temporo-parietal event-related responses to old—previously encoded—versus new items in AD patients relative to controls, after deep encoding. The mismatch negativity (MMN) was also shown to be a potential AD marker. The mismatch negativity is a well-known component of the event-related potential response, which is associated with the detection of deviant stimuli in a stream of standard, repeated stimuli—classically in the auditory modality, hence allowing the assessment of the quality of sensory processing, memory, and predictive coding [382, 383]. Its magnetic counterpart, the MMNm, was shown to be delayed in latency in AD compared to healthy elderly controls [384] (see also [385]). Most interestingly, using memory tasks in pre-clinical stages of AD, e.g., in APOE ɛ4 carriers, some studies pointed to the capacity of MEG for revealing neurophysiological markers of subjects’ decline, potentially predictive of pathology emergence [386, 387]. In sum, MEG can be used in the same way as EEG to investigate cognitive functions during various task performance; both these methods provide highly convergent and temporally detailed data on information processing and cognitive functions in normal and pathological aging.
However, the most unique potential of MEG for uncovering pathophysiological mechanisms and providing new neurodynamic biomarkers in the field of AD may lie in the study of functional brain networks, particularly of resting state networks (for review, [388]). As mentioned above, fMRI studies have shown that, in the absence of task demand, the resting brain exhibits spontaneous and highly structured, often oscillatory, fluctuations in activity [389]. MEG and EEG provide a richer view of these networks in the time and frequency domains [390–395]. Resting state networks are usually studied using time-frequency decomposition of MEG (or EEG) signals. This allows identifying a rich set of resting state networks in distinct frequency bands (e.g., [390, 392, 393, 396]). It was shown that AD patients show altered resting state network activity. This was revealed at the level of oscillatory activity characteristics, pointing to an overall slowing of brain rhythms with particular abnormalities in the delta (<4 Hz) and beta (∼20 Hz) frequency ranges [397–402]. Moreover, alteration of resting state networks, correlated with memory impairment, was recently shown using a graph-theoretical approach applied to neuromagnetic data [403]. Important questions are: When do these changes emerge in the course of the disease and which changes are predictive of or specific for the development of molecular and clinical AD? There is particular potential in EEG and MEG methods to provide such a surrogate biomarker for clinical outcome. Moreover, there is evidence that some MEG markers of functional brain networks may be predictive of the conversion from MCI to AD dementia [397, 400, 404].
On a practical note, it is important to underline that resting state studies have the advantage to be particularly adapted for elderly patients, because they require no cognitive effort and require relatively modest data acquisition time. It is worth mentioning that MEG, in comparison to most EEG systems, requires only a short time of subject’s preparation for recording. The whole-head MEG systems that are available at present comprise about 300 sensors that are fixed in a rigid helmet. After head shape numeration and the installation of a few reference sensors, individuals are comfortably seated with their head placed in the helmet. The installation time takes as little as 20 minutes. Moreover, the total “innocuity” of MEG allows close follow-up and detailed longitudinal assessment of disease progression.
The recent development and promising results of neuromagnetic imaging methods has led to the Magnetoencephalography International Consortium of Alzheimer’s Disease (MAGIC-AD) initiative. This initiative aims at advancing the use of MEG for AD and pre-AD research, combining data from resting state and simple memory and MMN tasks, in a multi-centric study [405]. While still in its burgeoning with regard to clinical applications, MEG has the potential to provide new tools for patient stratification, in order to better target patient population for clinical trials, and for treatment evaluation [406, 407], and to shed new light on the neurodynamic pathophysiological mechanisms of AD. It allows to foresee the identification of individualized signatures of disease progression in the form of temporal profiles of early adaptive, compensatory, and decompensatory brain network changes. Moreover, it is clear that the full power of MEG will come from its combination with other methods to allow multimodal assessment of individuals and IDM of multi-modal big data. For example, the combination of genetic data, such as the APOE polymorphism characterization with MEG resting state analysis has revealed promising in identifying MCI subjects at high risk of conversion to AD dementia as well as asymptomatic subjects at high risk of developing significant cognitive deterioration [408]. Multifactorial characterization of MCI subjects, including neuropsychological assessment, structural and functional brain measures, APOE genotyping, demonstrated very high sensitivity and specificity for predicting conversion to AD [409].
In conclusion, the advances in the characterization of the dynamics of functional brain networks based on MEG stands the chance to provide new insights into the pathophysiological mechanisms of AD. In doing so, it shall constitute a powerful tool to bridge the gap between what is known from the cellular and molecular pathways of the disease—its start and its progression—and the cognitive dysfunctions constituting its clinical and behavioral hallmark. This is likely to be key for developing new biomarker-guided targeted treatments and PM, based on the characterization of the individual genetic patterns and pathophysiological pathways towards neurodegeneration and dementia.
CONTRIBUTION AND ROLE OF NEUROMODULATION
Neuromodulation refers to forms of more or less invasive targeted and reversible electrical stimulation of discrete brain regions; it usually assists, but not replaces, traditional pharmacological treatments, with the aim to induce long-lasting changes of firing neural properties, both in the target region and connected networks, thereby modifying behavior or diseases’ symptoms. Therefore, neuromodulation fits well with the broad paradigm of PM that is the customization of healthcare tailored on the individual patients’ demands and disease’s pathophysiology.
Invasive neuromodulation in AD
Neuromodulation through deep brain stimulation (DBS) is an emerging opportunity in AD, being already an established therapy for advanced neurological and psychiatric diseases [410]. Several subcortical and cortical targets of stimulation have experimentally shown improvements in learning and memory, reinforcement of synaptic strength and restoring of physiological patterns of oscillatory brain activity, especially in the theta band, a rhythm that is functional to memorization [411]. DBS of the entorhinal cortex [412] enhanced memory of spatial information when applied during learning. DBS of the nucleus basalis of Meynert was studied in six patients with mild to moderate AD in a 12-month pilot study [413]. DBS was well tolerated and 4 of 6 patients were considered stable or improved at 12 months based on cognitive scores. The fornix, a deep WM tract interconnecting hippocampus with mammillary bodies, and a central node of the Papez circuitry which is integral to memory function [411], has been the most investigated, human DBS target for AD [414–417].
A 12-month follow-up of the first implanted 6 patients in the bilateral fornix showed a possible slowing of cognitive decline in some of them, accompanied by increase of metabolism in memory-related neural network structures [418], and by a reversal of the usual hippocampal atrophy found in AD [416]. These promising results prompted the first multicenter, 12-month, double-blind, randomized, controlled study of bilateral DBS of bilateral fornix in 42 patients with mild probable AD [419, 420]. The study showed no differences between those patients who received stimulation compared to controls who were not stimulated in cognitive measures. However, patients who received stimulation showed an increase in glucose metabolism in pre-selected brain regions at 6 and 12 months whereas those who were not stimulated showed decreased metabolism as expected. In a post-hoc regression analysis age was associated with outcome. Patients with late onset disease (≥65 years old) receiving stimulation showed a slowing of decline in cognitive measures when compared to those not stimulated. Improvement in glucose metabolism in this subgroup was greater in magnitude compared to the group as a whole. Stimulation of the fornix appeared to be safe. The overall perioperative adverse effects of the procedure, despite the cortical atrophy and the trans-ventricular trajectories of the electrodes towards the deep target, were comparable in DBS in other ND and there was no evidence of mortality or neurological morbidity at three months from the implant [419].
Non-invasive neuromodulation in AD
A different, non-invasive yet still experimental in AD, research approach for neuromodulation is the targeting of neocortical regions relevant to AD pathophysiology, through the scalp by applying repetitive transcranial magnetic stimulation (rTMS) or weak currents via transcranial direct current stimulation (tDCS), in repeated daily sessions of stimulation [421]. Mechanisms of action are different, as rTMS makes cortical neurons to fire trans-synaptically [422], while tDCS shifts the level of their firing probability in a polarity-dependent manner [423]. Both stimulation techniques induce controllable excitatory or inhibitory after effects: high-frequency rTMS and anodal tDCS generally increase cortical excitability, while low-frequency rTMS and cathodal tDCS do the opposite [424, 425]; these effects are either local or involve the cortico-subcortical network to which the targeted region belongs [426]. In case of AD, the mere “stimulation” of a cortical target, even if prolonged for several daily sessions, does not help so much in preventing the decline of memory and other cognitive functions [421]. However, there are few controlled studies for rTMS in AD and even less for tDCS, for a total of a few dozens of patients treated so far [421]. What is emerging as a possible role for non-invasive neuromodulation is the coupling of stimulation with cognitive therapy, with the aim to promote plastic associative learning mechanisms to synergically improve the effects of cognitive rehabilitation only [427–429]. This approach, while still in need of quantitative characterization [430–432] seems promising only in mild AD, when the severity of neurodegeneration makes still available a residual neural substrate to possibly intervene on [433].
From the bench to the patient: A future way of non-invasive neuromodulation?
Physiological cerebral activity is composed of oscillatory activity across a wide range of frequencies, ranging from 0.05 up to 500–600 Hz: oscillations in the 30–80 Hz range are known as “gamma” activity. A relative attenuation of gamma activity is a consistent finding in patients with AD [315]. Moreover, dysregulation of hippocampal theta/gamma coupling may precede amyloid deposit activity in animal models of AD [434]. A seminal recent study in pre-symptomatic and amyloid pre-depositing AD mice, showed that exogenously-induced flickering lights oscillating at 40 Hz reduce Aβ concentrations and amyloid plaques, as well as tau concentrations, in a mouse model of AD [435], preventing subsequent neurodegeneration and behavioral deficits, thus suggesting that gamma induction may represent a novel therapeutic approach for AD. This opens translational perspectives, as the possibility of modulating gamma activity in humans, potentially leading to the same beneficial effects observed in mouse models. The possibility of modulating brain oscillatory patterns in AD patients has been recently shown, with EEG changes in brain connectivity in the gamma band following the administration of antiepileptic drugs [436].
A viable way to interact with brain oscillations is transcranial alternating current stimulation (tACS), where low intensity (max 2 mA) alternating sinusoidal currents are applied via scalp electrodes. Due to the safety [437] and controllability (in terms of stimulation frequency and the possibility to target almost any cortical region) of the procedure, tACS has gained consensus as one of the most promising techniques to modulate brain oscillations in the healthy and pathological brains. Empirical evidence using neurophysiological markers, demonstrate that tACS modulates brain oscillatory activity via network resonance, suggesting that a weak stimulation at a resonant frequency could cause large-scale modulation of network activity and amplify endogenous network oscillations in a frequency-specific manner [438–441]. The application of tACS in the gamma band (specifically 40 Hz) has been shown effective in transiently modulating various abilities in humans, including those related to higher-order cognition [442, 443] and sensorimotor performance [444]. The repeated administration of tACS in AD patients, if individually tailored on cortical regions with higher concentration of Aβ, might constitute a timely, disease-transforming, personalized therapeutic application worth to be tested in patient populations.
CONTRIBUTION AND ROLE OF POSITRON EMISSION TOMOGRAPHY
Positron emission tomography (PET) has the potential to make a major contribution to selection for treatment in AD. This is of particular interest at very early asymptomatic stages of the disease, when clinical symptoms are still absent. In addition, it may also turn out as important at later stages as it is increasingly being recognized that several distinct pathophysiological processes can contribute to the development and manifestation of first symptoms and dementia. They vary considerably among patients, and one would therefore want to target the leading cause in individual patients.
At preclinical or prodromal disease stages identification of fibrillary amyloid deposits by PET currently is of obvious importance as an approved imaging biomarker for clinical trials. Use of a conservative cut-point has been suggested to minimize inclusion of elderly subjects with beginning amyloid deposition but without subsequent worsening [445]. Depending on a positive outcome of trials, amyloid PET might become a theragnostic procedure to select patients for anti-amyloid treatment.
In individuals with manifest dementia, differential diagnosis between AD and other diseases, such as FTD and vascular dementia, is important for selecting symptomatic treatment. 18F-2-fluoro-2-deoxy-D-glucose PET (18F-FDG-PET) has repeatedly been demonstrated to provide reliable differentiation between AD and FTD [446]. Beyond its relevance in the differential diagnosis, 18F-FDG-PET is a topographic marker of AD that can be used to measure disease progression and help identifying clinical subtypes [447]. Thus, it has a mediational effect between the neuropathological hallmarks of the disease (neurofibrillary tangles and Aβ) and the cognitive symptoms [448]. It has also been used successfully to study mechanisms underlying cognitive reserve, which delays the onset of dementia [449]. Identification of in vivo AD pathology has also proven to be relevant in disease identification. Indeed, some AD clinical phenotypes can be underlain by several neurodegenerative disorders (e.g., primary progressive aphasia, corticobasal syndrome), including the classical amnestic AD [450]. In such cases amyloid PET can identify fibrillary amyloid as an indicator of AD. Fibrillary amyloid can also coexist with other pathologies, which is frequently the case in patients with DLB and vascular dementia (which might be termed mixed dementia), but is also possible with FTD and may possibly contribute to more rapid progression [451, 452]. Thus, if anti-amyloid therapy did eventually show clinical benefit in AD patients, patients with non-AD dementia and positive amyloid PET might also benefit.
Among the large variety of possible pathophysiological contributors to AD, many are accessible by specific PET tracers. The most prominent are fibrillary tau deposits. The current generation of PET tau tracers has been demonstrated to reflect the pathological staging of tau deposits in AD, but there is also evidence of some off-target binding that complicates the interpretation of scans. Next generation tracers are being developed to overcome these limitations [453].
Neuroinflammation is another major factor which has been shown to accelerate disease progression. It is associated with activation of microglia, which can be imaged by PET using the translocator protein (TSPO) tracers. 11C-(R)-PK11195 has been the first of those, and in spite of some limitations due to a relatively high level of non-specific binding is still widely used. A large number of second generation tracers with higher specificity has been developed but their binding is subject to a genetic polymorphisms that blurs the advantage of these tracers [454]. Nonetheless, beyond these limitations, the development of these tracers could provide relevant biomarkers and offer new insights in the variability of evolution of AD [455]. There are also tracers for imaging of astrogliosis, and markers for cytokines and inflammatory endothelial changes are being developed. Further translational research will investigate the molecular characteristics and the effects of targeted interventions on microglial and astrocytic activation. Deficits in cholinergic transmission play a major role for deficits in memory and attention in patients with dementia. Tracers have been developed for nicotinic and muscarinic receptors, for vesicular transporters and acetylcholinesterase. Clinical studies have provided preliminary evidence that such tracers could be used to identify responders to acetylcholinesterase inhibitor therapy, and further research into this issue is required [456].
There are well established single photon emission computed tomography (SPECT) and PET tracers for identification in dopaminergic transmission, which is most severely affected in DLB. This is providing a useful diagnostic tool for differentiation between AD and DLB, while research is ongoing to identify the cognitive deficits associated with that deficit and potential targeted therapeutic interventions [457].
There is also current research into PET imaging of glucose energy metabolism, mitochondrial damage, glutamatergic and GABAergic dysfunction, blood-brain barrier damage and defects in transcriptional regulation and protein synthesis. They may play an important role in AD pathophysiology and offer windows for targeted intervention.
In conclusion, there is a huge potential of PET to contribute development of the PM paradigm in AD. Currently, amyloid imaging has been progressed most as a biomarker in clinical trials towards that goal. 18F-FDG-PET and tau-PET imaging are also involved in multiple trials, while a large variety of other tracer for specific targets in AD pathophysiology are still at earlier stages of translational research.
CONTRIBUTION AND ROLE OF RETINAL IMAGING
Over the past three decades, growing evidence indicates that AD is not confined to the brain but also affects the eye. Patients with AD and subjects with MCI experience a wide spectrum of visual deficits [458–464], sleep disturbances [465–471], and ocular abnormalities [466, 472–489]. Historically, these visual and circadian rhythm disturbances were attributed to pathology in the brain yet are now being revisited and explored as a potential direct outcome of ocular pathologies. Among ocular tissues, studies have shown that the retina is massively impacted by AD [466, 472, 474–479, 482, 484, 486, 487, 490–507]. The retina of MCI subjects and AD patients displays a host of abnormalities including nerve fiber layer (NFL) thinning, optic nerve and retinal ganglion cell (RGC) degeneration, macular volume changes, retinal angiopathy involving reduced blood flow and vascular structural alterations, astrogliosis, and abnormal electroretinogram patterns [472]. Given these findings, it is no surprise that attention has begun shifting towards the neuro-retina as a site of AD manifestation.
As a CNS tissue derived from the embryonic diencephalon, the retina shares many structural and functional features with the brain [508], including the presence of neurons, astroglia, microglia, pericytes, microvasculature with similar morphological and physiological properties, and a blood barrier [509–511]. Axons of the optic nerve directly connect the retina and brain, facilitating vesicular transportation of AβPP synthesized in RGCs [512]. Further, retinal neurons and glia secrete proteins associated with the amyloid cascade including γ-secretase, BACE1, Apolipoprotein E, and clusterin [511, 513, 514]. However, the skull-encased brain is shielded by bone, whereas the retina is accessible for direct, non-invasive high-resolution imaging.
The converging evidence denoting retinal abnormalities related to nerve degeneration and vascular changes, common to various neurological and ocular diseases, have long been described in MCI subjects and AD patients. Yet, the AD-specific pathophysiological hallmark, Aβ plaques, was only recently identified in postmortem retinas of AD patients and early-stage cases [490]. Subsequent studies corroborated these findings of retinal Aβ deposits and further indicated the presence of p-tau in retinas of AD patients [466, 485, 489, 515, 516]. These studies provided evidence for elevated retinal Aβ40 and Aβ42 peptides using biochemical assays on whole retinal extracts and revealed diverse retinal Aβ plaque morphology in flatmounts, often associated with blood vessels or co-localized with sites of cell degeneration (Fig. 7A-H) [466, 485, 489, 490, 515, 516]. Recent data showed that retinal Aβ deposits were found in clusters and frequently mapped to peripheral regions in the superior quadrant in AD patients (Fig. 7C, F). The load of Aβ42-containg retinal plaques in the superior quadrant was substantially elevated by 4.7-fold in patients compared to age- and gender-matched controls (Fig. 7C, D) [485]. While two groups were unable to detect Aβ or p-tau in the human AD retina [489, 517], they relied on analysis of cross sections prepared from narrow strips spanning horizontally from nasal to temporal quadrants, regions scarce in Aβ pathology. In contrast, a recent study provided in-depth characterization of retinal Aβ deposits in larger cohorts of definite AD patients via scans of large retinal areas in flatmounts and in cross sections derived from geometrical regions abundant with Aβ pathology [485]. The discovery of classical, dense-core (compact), and neuritic-like plaques in these patients, albeit smaller in average size compared to plaques in the brain, along with neurofibrillary tangles, Aβ42 fibrils, protofibrils, and structures resembling oligomers, suggests that the specific signs of AD are shared between the retina and the brain (Fig. 7G). A correlation analysis in a subset of patients has validated positive relationships between retinal and respective cerebral Aβ plaque burden, with a tighter association to plaques in the primary visual cortex (Fig. 7H) [485]. Notably, retinal regions in AD patients where abundant Aβ pathology was detected—the periphery of the superior quadrant and the innermost retinal layers—also showed a significant decrease in retinal neuronal cells (Fig. 7E, F), in agreement with previous studies showing a marked RGC loss and NFL thinning in the superior quadrants [466, 476, 484, 491, 498, 502, 518, 519]. A recent clinical study identified circadian abnormalities in AD patients along with a significant loss of melanopsin RGCs (mRGCs), photoreceptors known to drive circadian photoentrainment [520], and discovered Aβ accumulation within and around these degenerating cells. The loss of mRGCs may therefore result from their increased susceptibility to toxic Aβ forms and offers a plausible retina-based explanation for sleep disturbances in AD [466].
Fig.7
Retinal amyloid imaging: from histological examination to clinical trials. A) Spectral analysis of Aβ plaque in AD human flatmount retina via specific curcumin labeling. Representative image and spectra curves of retinal Aβ plaque double-labeled with curcumin [region of interest (ROI) 1; orange line] and anti-Aβ40 antibody-Cy5 conjugate (ROI2; purple line) and corresponding background areas (ROI3 and ROI4; dashed lines) at excitation wavelengths of 550 nm (for curcumin spectra) and 640 nm (for Ab-Cy5 conjugate). Sudan black B (SBB) was applied to quench autofluorescence. Peak emission wavelengths captured for the same individual Aβ plaque (605 nm for curcumin when bound to Aβ plaque and 675 nm for anti-Aβ Ab conjugated Cy5) are distinct, indicating specific fluorescent signals for each fluorochrome and signifying the detection of Aβ plaque by curcumin. B) Representative z-axis projection images of flatmount retinas from AD patients. Retinal Aβ plaques (yellow spots) co-labeled with curcumin (green) and anti-Aβ40 monoclonal antibody (11A50-B10; red) are detected. Analysis included definite AD (n = 8), probable/possible AD (n = 5), and age-matched controls (n = 5). High-magnification image (right) showing an extracellular Aβ plaque. Images A-B are adopted from [490]. C) Representative microscopic images from flatmount retinas of a healthy control individual (CTRL; 71 years) and a definite AD patient (74 years) stained with anti-Aβ42 C-terminal-specific antibody (12F4) and visualized with peroxidase-based labeling. High-magnification image showing different Aβ42 plaques including classical morphology. Analysis included definite AD patients (n = 5) and matched controls (n = 5). Images reproduced from [466, 472]. D) Quantitative analysis of retinal Aβ42-containing plaques (12F4-immunoreactive area) in the superior quadrant shows a significant increase in AD patients versus matched controls. E) Quantitative Nissl+ neuronal area in retinal cross sections indicated a significant reduction in AD patients compared to CTRLs, which is associated with retinal neuronal loss. D, E) Data reprinted from [485] (n = 23 AD patients and n = 14 controls). F) Retinal flatmount illustration demonstrating the geometric distribution of pathology in AD retina by quadrant, with more consistent findings of nerve fiber layer thinning, neuronal degeneration and retinal Aβ deposits mapped to peripheral regions of the superior quadrant. Adopted from [472]. G) Representative images of a frontal cortex section and a flatmount retina from AD patients stained with 12F4 monoclonal antibody (brown) showing different Aβ42 plaque morphology including classical plaques (inserts). Clusters of Aβ42-containing plaques are often associated with blood vessels (bv; right image). H) Correlation analyses using Pearson’s coefficient (r) test between retinal 12F4+-plaque burden in the superior-temporal (ST) quadrant and cerebral plaque burden (Thioflavin-S staining) in a total of seven brain regions (Brain; black) and in the primary visual cortex alone (PV Ctx.; green) in a subset of AD patients and matched CTRLs. I, J) Illustration displaying non-invasive retinal amyloid imaging using Longvida® curcumin and a modified scanning laser ophthalmoscope in human trials. K-M) In vivo retinal imaging in AD patients and age-matched controls. K, L) Increased curcumin fluorescent signal (red dots) in superior hemisphere in AD patient versus CTRL. Color-coded spot overlay images: red spots are above threshold and considered curcumin-positive amyloid deposits; green spots exceed 1:1 reference but not threshold; blue spots fall below reference. Heat map images with red spot centroids (lower panel) showing regions of interest with more amyloid plaques in the retina. L) Automated calculation of retinal amyloid index (RAI). Blue line is 1:1 reference; green line represents the threshold level, determined at 500 counts and above; red spots are above the threshold. The same automated image processing and analysis was applied on all human subjects (n = 16). M) RAI scores showing significant increase in AD patients compared to age-matched CTRLs. G-M) Republished with permission of American Society for Clinical Investigation from [485]; permission conveyed through Copyright Clearance Center, Inc. Group means and SEMs are shown. **p <0.01, unpaired two-tailed Student’s t-test.
![Retinal amyloid imaging: from histological examination to clinical trials. A) Spectral analysis of Aβ plaque in AD human flatmount retina via specific curcumin labeling. Representative image and spectra curves of retinal Aβ plaque double-labeled with curcumin [region of interest (ROI) 1; orange line] and anti-Aβ40 antibody-Cy5 conjugate (ROI2; purple line) and corresponding background areas (ROI3 and ROI4; dashed lines) at excitation wavelengths of 550 nm (for curcumin spectra) and 640 nm (for Ab-Cy5 conjugate). Sudan black B (SBB) was applied to quench autofluorescence. Peak emission wavelengths captured for the same individual Aβ plaque (605 nm for curcumin when bound to Aβ plaque and 675 nm for anti-Aβ Ab conjugated Cy5) are distinct, indicating specific fluorescent signals for each fluorochrome and signifying the detection of Aβ plaque by curcumin. B) Representative z-axis projection images of flatmount retinas from AD patients. Retinal Aβ plaques (yellow spots) co-labeled with curcumin (green) and anti-Aβ40 monoclonal antibody (11A50-B10; red) are detected. Analysis included definite AD (n = 8), probable/possible AD (n = 5), and age-matched controls (n = 5). High-magnification image (right) showing an extracellular Aβ plaque. Images A-B are adopted from [490]. C) Representative microscopic images from flatmount retinas of a healthy control individual (CTRL; 71 years) and a definite AD patient (74 years) stained with anti-Aβ42 C-terminal-specific antibody (12F4) and visualized with peroxidase-based labeling. High-magnification image showing different Aβ42 plaques including classical morphology. Analysis included definite AD patients (n = 5) and matched controls (n = 5). Images reproduced from [466, 472]. D) Quantitative analysis of retinal Aβ42-containing plaques (12F4-immunoreactive area) in the superior quadrant shows a significant increase in AD patients versus matched controls. E) Quantitative Nissl+ neuronal area in retinal cross sections indicated a significant reduction in AD patients compared to CTRLs, which is associated with retinal neuronal loss. D, E) Data reprinted from [485] (n = 23 AD patients and n = 14 controls). F) Retinal flatmount illustration demonstrating the geometric distribution of pathology in AD retina by quadrant, with more consistent findings of nerve fiber layer thinning, neuronal degeneration and retinal Aβ deposits mapped to peripheral regions of the superior quadrant. Adopted from [472]. G) Representative images of a frontal cortex section and a flatmount retina from AD patients stained with 12F4 monoclonal antibody (brown) showing different Aβ42 plaque morphology including classical plaques (inserts). Clusters of Aβ42-containing plaques are often associated with blood vessels (bv; right image). H) Correlation analyses using Pearson’s coefficient (r) test between retinal 12F4+-plaque burden in the superior-temporal (ST) quadrant and cerebral plaque burden (Thioflavin-S staining) in a total of seven brain regions (Brain; black) and in the primary visual cortex alone (PV Ctx.; green) in a subset of AD patients and matched CTRLs. I, J) Illustration displaying non-invasive retinal amyloid imaging using Longvida® curcumin and a modified scanning laser ophthalmoscope in human trials. K-M) In vivo retinal imaging in AD patients and age-matched controls. K, L) Increased curcumin fluorescent signal (red dots) in superior hemisphere in AD patient versus CTRL. Color-coded spot overlay images: red spots are above threshold and considered curcumin-positive amyloid deposits; green spots exceed 1:1 reference but not threshold; blue spots fall below reference. Heat map images with red spot centroids (lower panel) showing regions of interest with more amyloid plaques in the retina. L) Automated calculation of retinal amyloid index (RAI). Blue line is 1:1 reference; green line represents the threshold level, determined at 500 counts and above; red spots are above the threshold. The same automated image processing and analysis was applied on all human subjects (n = 16). M) RAI scores showing significant increase in AD patients compared to age-matched CTRLs. G-M) Republished with permission of American Society for Clinical Investigation from [485]; permission conveyed through Copyright Clearance Center, Inc. Group means and SEMs are shown. **p <0.01, unpaired two-tailed Student’s t-test.](https://ip.ios.semcs.net:443/media/jad/2018/64-s1/jad-64-s1-jad179932/jad-64-jad179932-g007.jpg)
In line with the above findings, numerous studies examining the retina of transgenic and sporadic animal models of AD have reported Aβ deposits, vascular Aβ, p-tau, and paired helical filament-tau (PHF-tau), often in association with RGC degeneration, local inflammation (i.e., microglial activation), and impairments in retinal structure and function [472, 485, 490, 515, 516, 520–537]. These investigations, which included a variety of transgenic rat and mouse models (ADtg) as well as the sporadic rodent model of AD, O. degus, demonstrated abundant Aβ deposits, mainly in the GCL and NFL [490, 516, 521, 525, 528, 530, 533]. Furthermore, several publications have described positive responses to therapies in reducing retinal Aβ plaque burden in ADtg mice, often reflecting the reactions observed in the respective brains [490, 524, 527, 528, 532, 536].
To visualize retinal Aβ pathology in live subjects, a non-invasive retinal amyloid imaging approach was initially developed in ADtg mice, utilizing curcumin as a fluorescent probe [490, 527]. Curcumin is a natural and safe fluorochrome that crosses the blood-brain and -retinal barriers and binds to Aβ fibrils and oligomers with high affinity [490, 527, 538–551], with the ability for ex vivo and in vivo visualization when specifically bound to retinal Aβ plaques (Fig. 7A, B) [485, 490, 527]. This approach enabled non-invasive detection and monitoring of desecrate retinal Aβ deposits in live animal models of AD [490], including the capability to track the dynamic appearance and clearance of individual plaques and their substantial reduction after glatiramer acetate immunotherapy [527, 552, 553].
In a proof-of-concept clinical trial, the safety and feasibility to non-invasively detect and quantify retinal amyloid deposits in live human patients was demonstrated using a modified scanning laser ophthalmoscope and a proprietary oral curcumin formulation (Longvida®) with increased bioavailability (Fig. 7I-M) [485]. Corresponding to the pattern reported in histological examinations, retinal amyloid deposits in living AD patients were frequently concentrated in the mid- and far-periphery of the superior hemisphere (Fig. 7K). A significant 2.1-fold increase in retinal amyloid index, a quantitative measure developed to assess numerical value of amyloid burden in the retina of living patients, was revealed in AD patients versus matched controls (Fig. 7L, M) [485]. Recent studies applying non-invasive retinal imaging in live AD patients, which detected NFL thinning [466, 477], increased inclusion bodies [554, 555], reduced blood flow, microvasculature alterations, and oxygen saturation in arterioles and venules [479, 556, 557], and importantly, hallmark Aβ deposits [485], are encouraging first steps toward the development of practical tools for predicting disease risk and progression. Since the retina in other ND such as multiple sclerosis, ischemic stroke, and Parkinson’s disease also exhibits pathophysiological processes similar to those detected in the brain [501, 558–561], retinal imaging may also facilitate differential diagnosis for different proteinopathies, neurodegenerative and neurological diseases.
As research exploring AD in the brain, the possibility that the easily accessible retina may faithfully reflect AD neuropathology warrants further investigation. The preliminary evidence of retinal Aβ accumulation in early-stage cases together with the indication of amyloid-related neurodegeneration in the AD retina [466, 485, 490] suggests that AD is both a cerebral and an ocular disease, and may support retinal imaging as a screening tool even during the asymptomatic at risk stage. Future studies are needed to assess the nature of the relationship between cerebral and retinal amyloid burden in larger cohorts and in specific anatomical regions, and perhaps also to determine the potential link among cerebral amyloid angiopathy and retinal vascular amyloid. Given that retinal amyloid pathology could foretell brain disease and cognitive decline, it may prove essential for early detection of AD, predicting disease progression, and monitoring response to therapy.
In addition, non-invasive functional tests of pupil reactivity to light may complement the characterization of retinal abnormalities with imaging techniques [562]. Indeed, pupil responses to light stimulations are abnormal in AD patients [563], who show hypersensitive pupil-dilation to tropicamide, an acetylcholine receptor antagonist, as well as a diminished pupil light reflex [564, 565]. Although the retinal abnormalities mentioned above could account for these pupillary effects, the Edinger-Westphal nucleus, a major relay involved in pupil control where early signs of AD (cell loss and amyloid plaques) have also been observed, could also contribute to pupillary abnormalities. Conducting focal tests in different regions of the visual field to probe the pupil response can help identifying the functional consequences of the retinal amyloid imaging results. If the results of retinal imaging and functional tests were strongly correlated, pupil reactivity could be used as a proxy for AD severity, with the advantage that functional tests of pupil reactivity are easy, cheap and fast to perform, do not require a strong involvement of the patients, and can routinely be conducted to detect and track the evolution of AD, as well as the response to therapy.
In this regard, the “VISION” pilot translational neuroscience research program, belonging to the previously mentioned Sorbonne Université GRC-APM (GRC n° 21), has been developed and launched in an early asymptomatic preclinical population to assess retinal amyloid imaging for 1) screening of amyloid and tracking its progression as well as 2) predicting pathophysiological disease progression, cognitive decline, and conversion to prodromal AD. The non-invasive nature, easy accessibility and generalizability are appealing features regarding a potential context of use.
SPATIOTEMPORAL MODELING OF MULTIMODAL LONGITUDINAL DATA ANALYSIS
Nowadays, deepening our understanding of AD pathophysiology is made possible by the following biomarkers that can be derived in vivo from the subject: “fluid” from blood (e.g., genetic risk factors) and CSF (e.g., abnormal Aβ42 and p-tau dosing); “structural” (e.g., brain atrophy as a sign of neurodegeneration) and “functional” (e.g., brain disconnection syndrome) from MRI, “molecular” (e.g., brain hypometabolism and deposition of Aβ42 and p-tau) from PET, and “neurophysiological” (e.g., abnormal cortical neural synchronization and coupling). Furthermore, fine neuropsychological and clinical scales allow a detailed measurement of cognitive impairment, self-care, independence in living in a community, and mental disorders (e.g., anxiety, mood, psychosis, and behavior). All these measurements allow a personalized evaluation of cerebral residual capacity and function over time by the repetition of the recording sessions.
Keeping in mind this premise, a major issue is the identification of the best statistical and mathematical procedures, from computational neurosciences, weighting the information value of the above biomarkers and clinical indices for early diagnosis (even in preclinical or prodromal stages preceding dementia), monitoring, therapy response, and prediction of the disease evolution.
To this aim, digital brain models have been developed in recent years, as a way to synthetize a 3D geometrical model summarizing the anatomical invariants in a group of subjects [566–569]. This model has been extended recently to functional data [570, 571]. The main interest of such models is that they do not only illustrate the effects of the AD on brain structure and function at the group level but also include information about individual variability allowing the computation of the difference between a given patient and the reference groups of healthy subjects and patients with other dementing disorders to provide diagnostic information as sensitivity (detection of AD patients), specificity (detection of healthy subjects or patients with other diseases), and global classification accuracy.
These diagnostic models are based on the Bayesian inference of non-linear mixed-effects models, which complement the usual linear mixed-effects models typically used in biostatistics [569, 572]. This combination of statistical and geometric approaches accounts for the inherent structure in the data such as the specific organization of the brain anatomy as prior knowledge. It allows the rendering of the inter-individual variability as a realistic and interpretable change of the 3D model. Individual characteristics are summarized by a multivariate descriptor, which may be used in turn to explore the distribution of the individuals in different clusters, to correlate it with external factors, or to use as input in machine learning algorithms to make individual predictions [568].
Ideally, such a static model should be adapted to account for the disease progression over time and provide prognosis of clinical evolution in individual AD patients. Digital models of brain ageing are constructed as dynamical models showing the complex spatiotemporal patterns of changes in the above biomarkers while the disease progresses. Inter-individual variability is expressed in terms of changes in individual spatiotemporal trajectories. The construction of such models of disease progression results from several key components [570, 571, 573–576]: 1) artificial intelligence approaches that are used to combine several short-term data sequences in longitudinal data sets to synthetize a long-term scenario of disease progression; 2) different data modalities that are integrated in the model by converting them into a common abstract mathematical space (called a Riemannian manifold) where statistical distributions of spatiotemporal trajectories may be rigorously defined; 3) variability in trajectories accounting for the direction of the trajectories and the dynamics at which these trajectories are followed.
Each individual disease trajectory is now positioned in a spatiotemporal coordinate system, where a multivariate descriptor encodes the variability in the direction of the trajectory, and dynamical parameters encode for the variability in age at disease onset and pace of disease progression. Given the observation of a new subject at one or few time-points, one may personalize the scenario of disease progression by adjusting model parameters, thus transferring the knowledge gained from the automatic analysis of a longitudinal data set to this new individual. This personalized model may be utilized then to predict the future state of the subject, for instance the time to the onset of a specific symptom. We have employed such an approach to predict the time-to-diagnosis in mild cognitive impaired subjects using a model of cognitive decline from neuropsychological assessments [577], and to predict the future map of cortical thickness for the same subjects using structural imaging [571]. This approach opens up the way to build efficient decision support systems for monitoring disease progression and selecting patients in clinical trials with a specific biomarker-based diagnosis of AD, at a specific disease stage (e.g., preclinical, prodromal, or manifest dementia) and with an expected pattern of progression.
In addition, such a personalized scenario may offer a new way to assess treatment efficacy by evaluating to which extend it changes the disease trajectory, that is the complex non-linear spatiotemporal patterns of changes. This approach evolves the standard procedure based on annual percentage rate of an outcome measure since: 1) it does not assume a linear variation of the outcome at all disease stage but account for the non-linear dynamics of changes across disease stages, and 2) it makes use of a multivariate descriptor of disease trajectory and not only a univariate outcome measure.
THE EMERGING FIELD OF SYSTEMS PHARMACOLOGY IN ALZHEIMER’S DISEASE
The consequences of the highly complexity of AD pathophysiology can be clearly observed in the results of drug development pipeline for the disease: out of 413 clinical trials conducted during the 2002 to 2012 period, 99.6% failed [578]. Moreover, a review of AD drug development pipeline in 2016 showed that although the pipeline has increased in size, it is significantly smaller compared to the cancer field, and that the most common target (76%) is still amyloid, reflecting the urgent need for deeper understanding the pathophysiology of the disease [579]. In fact, disappointing results of anti-amyloid drug candidates can be attributed to three major factors relating to drug discovery and development, namely 1) inter-species mechanistic differences between animal models and human, 2) complex biology of Aβ in relation to disease staging, and 3) ignorance of non-amyloid pathways. Thus, it is imperative to delineate the complexity of AD pathophysiology using systems biology-based approaches, which take advantage of computational analysis and modeling of both quantitative (e.g., “omics”-based) and qualitative (e.g., literature-based) data. The goal of systems biology methods is to aid researchers develop hypotheses regarding the disease system and gain better mechanistic insights into the pathophysiology and progression of disease across multiple biological scales and time. Mechanistic systems models are either mathematical representations of pathophysiologic processes or computable cellular networks but the latter has gained more attention for analysis of drug action [580]. Since these models use networks instead of single transduction pathways, complex patterns of drug action within the target biological context can be studied in more details, a field that has emerged as systems pharmacology.
According to the American Association of Pharmaceutical Scientists (AAPS), systems pharmacology is “the science of advancing knowledge about drug action at the molecular, cellular, tissue, organ, organism, and population levels” (available at http://www.aaps.org/Systems_Pharmacology/). To obtain full understanding of drug action at the systems level, we need to combine disease mechanism, pharmacodynamics, and pharmacokinetic data into a single model. However, incorporation of quantitative parameters and measurements increases the model complexity so that special mathematical techniques are required to reduce the number of parameters without affecting the behavior of the system; thus, disease mechanistic models are considered as the first substrate for building full-fledged systems pharmacology models [581]. Disease mechanistic models are molecular and cellular networks that aim to elucidate the impact of therapeutics or new drug candidates on impaired biological functions under disease conditions. The key to usefulness of disease models is context-sensitivity, meaning that disease network models should represent the real-world context in terms of cell and tissue type (spatial dimension), disease sub-type (functional dimension), and progression stages (temporal dimension). It is only in the right context that correct inferences, interpretations, and predictions can be made out of the model. The focus of earlier models was to relate drugs to proteins in the form of drug-target networks where protein-protein interaction networks were used as the fundamental model for interpretation of drug mode-of-action [582]. Interestingly, these models also revealed an important aspect of systems pharmacology paradigm, which was conceptualized and coined as “polypharmacology” [583]. This concept changed the single-target approach to designing new drugs in the discovery phase because topological analysis of drug targets in network models demonstrated that a compound binds to multiple targets. As a consequence, a drug hits additional targets, known as off-targets, which leads to side effects. Campillos and colleagues (2008) used drug-drug and drug-target networks enriched with side-effect phenotype information for all approved drugs across many disease indications and based on side-effect similarities predicted and experimentally validated novel drug-target relations [584]. This approach enables researchers to predict off-targets and thereby probable side effects for candidate drugs in preclinical settings. The so-called structural systems pharmacology aims at modeling energetic and dynamic modifications of genomic macromolecules including proteins, DNA, and RNA by drug candidates [585]. This strategy has been implemented by Nikolic and colleagues (2016) to predict both primary target and off-target profiles of several anti-neurodegenerative compounds based on their chemical structures [586]. Their analysis resulted in identification of novel compounds that hit multiple targets and inhibited acetylcholinesterase, butyrylcholinesterase, monoamine oxidases A and B in the context of AD pathophysiology. Moreover, knowing which drug properties distinguishes CNS drugs from others can help drug designers select those properties in the new drug candidates that confer the least side effects and the best efficacy. To this end, Shahid and colleagues (2013) developed a computational method that identified and classified neurodegenerative drugs from non-neurodegenerative drugs with 80% accuracy [587]. DrugGenEx-Net is a computational platform that predicts disease-specific drug polypharmacology based on multi-tiered network analysis of drug-target, disease-target, pathway-target and target-target interactions [588]; the model revealed that Sunitinib, an approved drug for renal cell carcinoma, hits multiple targets associated with AD pathways and thus can be considered for repurposing.
With advancements in systems biology modeling languages, such as Systems Biology Markup Language (SBML) and Open Biological Expression Language (OpenBEL), drug-mode-of-action can now be investigated in a context-sensitive, rich environment that goes beyond simple representation of protein-protein interactions by including various types of biological entities covering genotype to phenotype scales. For instance, Fujita and colleagues (2014) developed a comprehensive molecular interaction map of Parkinson’s disease that included major signaling pathways in Parkinson’s disease, modeled and presented in SBML format; however, they did not include drug information in their model [589]. AlzPathway is the result of an early initiative that attempted to systematically collect AD-related signaling pathways from literature and bring them together within the first map of cellular AD signaling pathways, represented in SBML [590]. Recently, Iyappan and colleagues (2016) identified all signaling pathways reportedly involved in the human ND, mapped them back onto their corresponding anatomic sites on the human brain, and used these pathways for explaining the mode-of-action of the AD approved drug, Rasagiline [591].
In the past years, with the availability of increasing amount of data and knowledge on the one hand, and emergence of new computational biology methods on the other, the IDM framework has increasingly drawn more attention by academic and pharmaceutical research groups. The models generated by this approach combine data-driven and knowledge-driven models into a single integrative model and represent signaling pathways with cause and effect relations [23]. However, a major challenge for this approach is integration of heterogeneous datasets and information that come from various data sources. For instance, the ADNI provides big neuroimaging data along with genetic and biomarker data from AD and MCI subjects [592]. If integrated into predictive models, ADNI data will have maximal impact on the AD drug research. But, the first step toward IDM is standardization and harmonization of different datasets so that they are semantically compatible. Ontologies are semantic frameworks that provide a reference for standardization and harmonization of diverse datasets. For instance, AD ontology (ADO) has been developed to provide such a reference for AD knowledge domain [593]. ADO was used by Kodamullil and colleagues (2015) to represent scientific findings in a computable, cause-and-effect model of AD pathology, which was designed and coded in Open Biological Expression Language (available at http://openbel.org/) [594]. This model contains causal and correlative relationships between biomolecules, pathways, and clinical readouts and was used for model-guided interpretation of genetic variation data for a comorbidity analysis between AD and type 2 diabetes mellitus. Similarly, drug-target interactions and drug mode-of-action can be investigated and predicted using these models. Indeed, integrative models that encompass data from genome to phenome across biological scales from cells to clinical outcomes, enable us to predict the mode-of-action of candidate drugs within the right pathophysiological context and in a multidimensional space of human biology. Perhaps one of the most fundamental works in this area is the study by Emon and colleagues (2017) who systematically analyzed the brain chemical space and identified drug candidates for repositioning in AD [595]. They first generated a large model in BEL containing genes, proteins, drugs and chemicals, biological processes, and disease concepts in the context of neurodegeneration. Then, by mechanistic analysis of this model, they not only suggested Donepezil as repurposing candidate for amyotrophic lateral sclerosis, but also found a mechanism of action by which Riluzole, a drug used in amyotrophic lateral sclerosis, could be predicted to interfere with several pathophysiological pathways in AD. Moreover, the mode-of-action analysis of other drugs in the context of AD using this model predicted that Cyclosporine, a drug used for treatment of rheumatoid arthritis, which shares common targets with 5 approved drugs for AD, can exert neuroprotective effects. Several lines of evidence that experimentally proved its anti-AD effects supported this prediction.
Currently, several initiatives have undertaken the effort to facilitate systems pharmacology studies in the field of ND in general and AD in particular. The AETIONOMY project, funded by the Innovative Medicine Initiative (see http://www.imi.europa.eu/), has already set up a specialized knowledgebase for ND with focus on AD and Parkinson’s diseases, and takes an integrative modeling approach to computationally predict and clinically validate mechanistic signatures that stratify AD and Parkinson’s patients (see http://www.aetionomy.eu/). The mission of this project is to lay foundation for development of new drugs targeting patient subgroups and thus promoting personalized medicine. The Brain Health Modeling Initiative (BHMI) is another project that takes advantage of integrative mechanism-based computational models and simulations using big data with the aim of matching right targets and biomarkers for optimal drug design in AD [596]. The European commission-funded project SysPharmAD proposes a systems pharmacology approach to the discovery of novel therapeutics in AD using an integrative network model that combines “omics” data with stage-specific clinical data. The aim of this project is to design and validate a systems pharmacology strategy based on AD staging that helps researchers identify synergistic multi-targeting compounds modifying the disease path (available at http://cordis.europa.eu/project/rcn/185567_en.html).
CONCLUSIONS
The multidimensional nature of all ND, AD included, is well established to-date, along with the fact that their onset and progression arise from dysregulation processes which evolve at both intracellular and extracellular levels. At the cellular level, ND are characterized by dystrophic neuronal structural changes leading to loss of function and, eventually, cell death. These phenomena spread in a “cell-to-cell” fashion in which intraneuronal protein misfolding affects structural plasticity in a nearby neuron by self-propagation of pathogenic protein aggregates. This, in turn, leads to decreased dendritic spines and synaptic sites density, and, eventually, loss of brain connections.
At the subcellular and molecular level, the core pathophysiological phenomenon is represented by failure of proteostasis cellular pathways [597, 598], from protein misfolding and aggregation to decreased clearance, mitochondrial dysfunction, loss of cell homeostasis, and, consequently, enhanced cell signaling pathways related to apoptosis. Therefore, ND are initially characterized by several alterations of subcellular frameworks, mostly concerning proteostasis, on which both the anatomy and physiology of neurons and glial cells are founded.
The genome, through mutual interactions with endogenous and exogenous factors, leads to a wide spectrum of variations at the level of proteome and metabolome that, incontrovertibly, account for both intracellular and extracellular integrity. As a result, the systems biology and systems neurophysiology paradigms can provide a conceptual model where structural and functional networks are dynamically interconnected across different dimensional levels into accounting a multiscale dynamical system which has already been seen to manifest also into peripheral branches like the autonomic nervous system in health and disease [599, 600].
At present, there is an urgent need to identify a large array of reliable biomarkers to in vivo identify the above mentioned interacting multidimensional levels which characterize ND. Such biomarkers need to be able to chart the spatio-temporal trajectories of complex brain pathophysiological mechanisms, at the same time taking into account interindividual variables. Complex, time varying higher order statistics as well as structural model should also be considered within the systems neurophysiology modeling approach [601–604]. Pathophysiological biomarkers are required to track the pathophysiological mechanisms underlying ND (Fig. 8). For instance, cerebral amyloid-PET is commonly considered as a molecular proxy of the Aβ metabolism impairment rather than a conventional biomarker of neocortical deposition of neuritis plaques. In this context, biomarkers are the appropriate tools for developing receptor-tailored drugs, as already demonstrated and currently practiced in the field of oncology. Both structural and functional brain markers are expected to elucidate the link between clinical phenotypes and molecular pathophysiological mechanisms.
Fig.8
Evolving spectrum of biomarkers and modalities. A) The ideal biomarker should be minimally-invasive, unexpansive, practical, rapid and reliable with low level of expertise required. Therefore, in the clinical-setting, biomarkers should be assessed in a multi-stage diagnostic workout carried-out along four steps (blood biomarkers, structural MRI, lumbar puncture, PET scans) according to the overall balance among the following factors: cost-effectiveness, time-effectiveness, invasiveness and accessibility. B) Biomarkers represent one strategy to tailor therapy. The idealistic markers for ND would enable their implementation in screening, diagnosis, progression of the disease, and monitoring of the response to therapy. Therefore, in clinical trials, biomarkers can be used for several purposes: 1) to identify people eligible for the trial, i.e., those considered at high risk for ND (screening biomarkers); 2) to guide clinical diagnosis (diagnostic markers); 3) to optimize treatment decisions, providing information on the likelihood of response to a given drug (predictive biomarkers); 4) to detect and quantify the response rate to treatment (response markers). MRI, magnetic resonance imaging; PET, positron emission tomography; ND, neurodegenerative diseases.
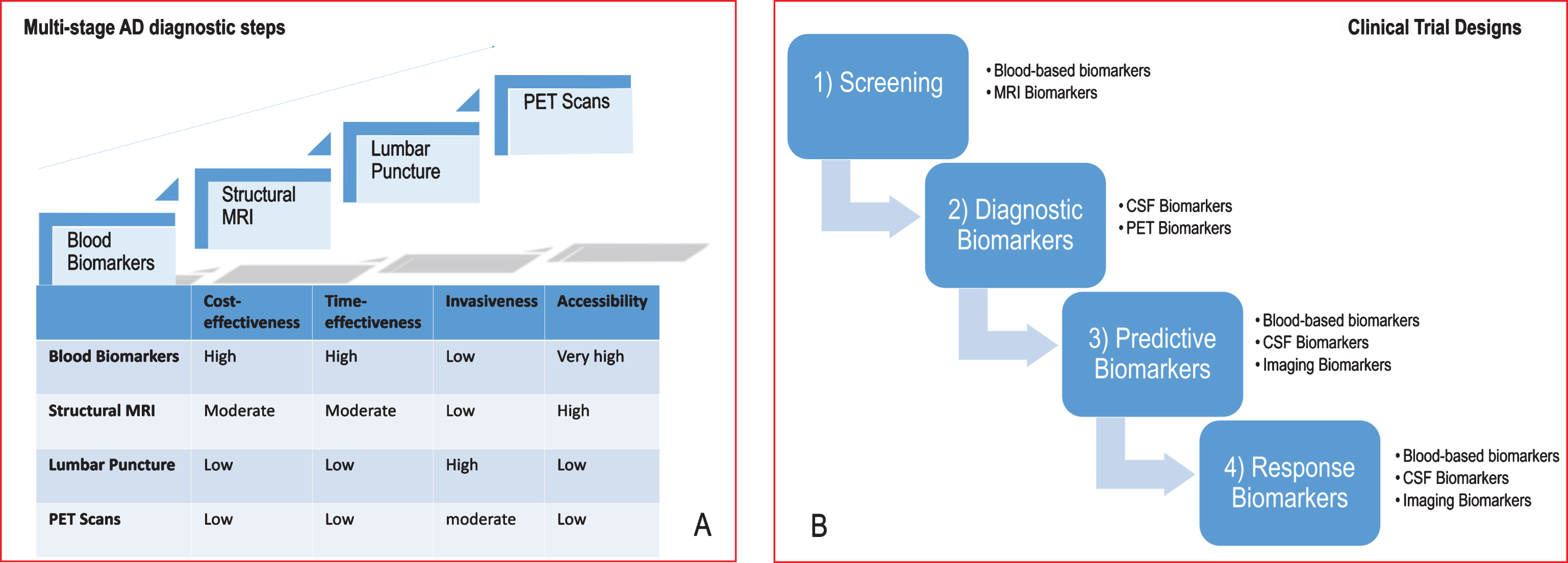
Notably, cerebral 18F-FDG-PET is commonly used as prognostic indicator in several clinical trials on AD and other ND. Indeed, the early recovery of specific brain functions or networks is crucial to identify downstream effects of disease therapies, even before measuring the clinical benefit. As another example, in the context of identifying brain biomarkers from non-invasive imaging within a more individually tailored, PM-based approach, recent developments have pointed out the concept and added value of “dense sampling of individual brains” [605–607]. This interesting development is based on the realization that, while a large body of research is accustomed to averaging neuroimaging data across individuals and, hence, implicitly assuming a high degree of functional homology, by definition there must be a finer scale at which this homology breaks down, possibly the scale which encodes the individual idiosyncrasies at the base of a unique individual’s disease trajectory and/or therapy response. By sampling relatively few brains for several hours, the authors demonstrate how individual differences in well-known networks, e.g., the default mode and the salience network, are clearly visible. Therefore, it is possible that future developments in neuroimaging will shift more toward longer (several hours/days) sampling of individual brains/patients, thus providing more solid bases for the implementation of the “precision neuroscience” paradigm that will likely be needed to understand ND.
Interestingly, functional and topographic biomarkers could also be employed in identifying the adequate target. In particular, they could be valuable in detecting specific brain areas for potential trials of targeted neuromodulation, thus providing comprehensive information on regional atrophy, impaired connectivity, metabolic alterations, and regional decrease of cerebral blood flow. Finally, both clinical examination and full psychometric evaluation still remain the first-line approach in identifying pathological phenotypes supporting the whole diagnostic workout. For instance, to date, the identification of hippocampal-like amnestic impairment supports the clinical diagnosis of AD, thus justifying an anticholinesterase inhibitor-based treatment. Notably, in the context of a systems biology- and systems neurophysiology-based interpretation of ND phenotype, clinical markers should be considered the highest level “descriptors” of the disease and represent the ultimate measures to identify effective therapies.
In summary, the future implementation of the systems biology and systems neurophysiology paradigms, based on the integrated analysis of big and deep heterogeneous data sources, will be crucial to reach a deeper understanding of the pathophysiology of AD and other ND. The main challenges ahead will certainly lie in the development of analytical applications capable of processing massive quantities of stored laboratory and clinical data. Against this backdrop, the big data approach should be leveraged to maximize the information that can be extracted from preclinical and clinical records, ultimately augmenting our knowledge regarding the molecular, cellular, and systems processes underlying AD development. As we unravel the dynamic and longitudinal changes of the biomarker landscape in AD, we will make a further step toward a holistic understanding of the natural course of the disease. Integrating different sources of information will enable researchers to obtain a new integrated picture of the pathophysiological process of the disease that will span from molecular alterations to cognitive manifestations. In this scenario, the Big Data Research and Development Initiative (available at https://obamawhitehouse.archives.gov/blog/2012/03/29/big-data-big-deal), promoted by the previous Obama Administration under the “Big Data is a Big Deal” motto, is expected to accelerate progress toward a new era of PM in AD. This ultimate mission will be accomplished by assembling, linking, and harmonizing big data to facilitate high-impact, multidisciplinary, and collaborative research efforts. After a decade of failed clinical trials in AD, the adoption of “big data science” within an IDM theoretical framework by the international APMI allowed us to enter into a transformative research scenario. It is currently expected that PM will underpin most, if not all, of the prevention and treatment advances yet to come. Significant breakthroughs in our understanding of the early phases of AD and other ND and the rapid advent of new laboratory technologies are providing unprecedented opportunities to make a major impact on the natural history of AD at the earliest preclinical asymptomatic stage [608]. We are currently standing at the edge of a new frontier that will thoroughly explore the molecular and cellular events that drive the development of the disease before cognitive symptoms are evident. New preventive approaches and therapies developed through PM may improve compliance and increased level of trust and confidence among all stakeholders and reduce the number of failures. In this context, we are expected to move swiftly from the traditional “one-size-fits-all – magic bullet therapies” scenario to a personalized PM-based approach. The unprecedented effort promoted by the APMI is ultimately tailored to implement a paradigm shift in AD research which will be backboned by large, international, and interdisciplinary collaborative academic, private and industry networks. The field of PM does not lack for enthusiastic, dedicated pioneers who are moving forward expeditiously to clinical adoption. As the evidence base supported by the APMI expands, much more can and should be done to accelerate the process for the benefit of individual patients, the healthcare system, and society overall.
Contributors to the Alzheimer Precision Medicine Initiative – Working Group (APMI-WG):
Principal Investigator and Speaker: Harald Hampel; Aguilar LF (Montréal), Babiloni C (Rome), Baldacci F (Pisa), Benda N (Bonn), Black KL (Los Angeles), Bokde ALW (Dublin), Bonuccelli U (Pisa), Broich K (Bonn), Bun RS (Paris), Cacciola F (Siena), Castrillo J† (Derio), Cavedo E (Paris), Ceravolo R (Pisa), Chiesa PA (Par-is), Colliot O (Paris), Coman CM (Paris), Corvol JC (Paris), Cuello AC (Montréal), Cummings JL (Las Vegas), Dubois B (Paris), Duggento A (Rome), Durrleman S (Paris), Escott-Price V (Cardiff), Federoff H (Irvine), Ferretti MT (Zürich), Fiandaca M (Irvine), Frank RA (Malvern), Garaci F (Rome), Genthon R (Paris), George N (Paris), Giorgi FS (Pisa), Graziani M (Roma), Haberkamp M (Bonn), Habert MO (Paris), Hampel H (Paris), Herholz K (Manches-ter), Karran E (Cambridge), Kim SH (Seoul), Koronyo Y (Los Angeles), Koronyo-Hamaoui M (Los Angeles), Lamari F (Paris), Langevin T (Minneapolis-Saint Paul), Lehéricy S (Paris), Lista S (Paris), Lorenceau J (Paris), Mapstone M (Irvine), Neri C (Paris), Nisticò R (Rome), Nyasse-Messene F (Paris), O’Bryant SE (Fort Worth), Perry G (San Antonio), Ritchie C (Ed-inburgh), Rojkova K (Paris), Rossi S (Siena), Santarnecchi E (Siena), Schneider LS (Los Angeles), Sporns O (Bloomington), Toschi N (Rome), Verdooner SR (Sacramento), Vergallo A (Paris), Villain N (Paris), Welikovitch L (Montréal), Woodcock J (Silver Spring), Younesi E (Esch-sur-Alzette).
ACKNOWLEDGMENTS
Dr. Harald Hampel is supported by the AXA Research Fund, the “Fondation partenariale Sorbonne Université” and the “Fondation pour la Recherche sur Alzheimer”, Paris, France. Ce travail a bénéficié d’une aide de l’Etat “Investissements d’avenir” ANR-10-IAIHU-06. The research leading to these results has received funding from the program “Investissements d’avenir” ANR-10-IAIHU-06 (Agence Nationale de la Recherche-10-IA Agence Institut Hospitalo-Universitaire-6).
Dr. Arun L.W. Bokde received funding from the Meath Foundation, Ireland.
Dr. Olivier Colliot received funding from the program “Investissements d’avenir” ANR-10-IAIHU-06 (Agence Nationale de la Recherche-10-IA Agence Institut Hospitalo-Universitaire-6), from the European Union H2020 Program (project EuroPOND, grant number No 666992), and from the joint NSF/NIH/ANR program “Collaborative Research in Computational Neuroscience” (project HIPLAY7, grant number ANR-16-NEUC-0001-01). He supported by a “Contrat d’Interface Local” from Assistance Publique-Hôpitaux de Paris (AP-HP).
Dr. Stanley Durrleman is funded by the European Research Council (ERC) under grant agreement No 678304, the European Union’s Horizon 2020 Research and Innovation Programme under grant agreement No 666992, and the program “Investissements d’avenir” ANR-10-IAIHU-06.
Dr. Maria-Teresa Ferretti is the President of Women’s Brain Project and is supported by a research fellowship by the Synapsis Foundation - Alzheimer Research Switzerland (ARS).
Dr. Nathalie George is supported by the French program “Investissements d’avenir” under Grant numbers ANR-10-IAIHU-06 (IHU-A-ICM) and ANR-11-INBS-0006 (France Life Imaging).
Dr. Maya Koronyo-Hamaoui is supported by NIH/NIA AG044897 and AG056478 Awards and by The Saban and The Marciano Private Foundations.
Dr. Karl Herholz is supported by research grants from GE Healthcare (GEHC) and GlaxoSmithKline (GSK).
Dr. Olaf Sporns acknowledges support from the U.S. National Institutes of Health (R01-AT009036).
Dr. Andrea Vergallo is supported by Rotary Club Livorno “Mascagni”/The Rotary Foundation (Global Grant No GG1758249).
Authors’ disclosures available online (https://www.j-alz.com/manuscript-disclosures/17-9932).
REFERENCES
[1] | Prince M , Wimo A , Guerchet M , Ali G , Wu Y , Prina M ((2015) ) World Alzheimer Report 2015. The global impact of dementia. An analysis of prevalence, incidence, cost & trends. Alzheimer’s Disease International, London. Available at: https://www.alz.co.uk/research/WorldAlzheimerReport2015.pdf |
[2] | Baldacci F , Lista S , Cavedo E , Bonuccelli U , Hampel H ((2017) ) Diagnostic function of the neuroinflammatory biomarker YKL-40 in Alzheimer’s disease and other neurodegenerative diseases. Expert Rev Proteomics 14: , 285–299. |
[3] | Baldacci F , Toschi N , Lista S , Zetterberg H , Blennow K , Kilimann I , Teipel S , Cavedo E , dos Santos AM , Epelbaum S , Lamari F , Dubois B , Floris R , Garaci F , Bonuccelli U , Hampel H ((2017) ) Two-level diagnostic classification using cerebrospinal fluid YKL-40 in Alzheimer’s disease. Alzheimers Dement 13: , 993–1003. |
[4] | Olsson B , Lautner R , Andreasson U , Öhrfelt A , Portelius E , Bjerke M , Hölttä M , Rosén C , Olsson C , Strobel G , Wu E , Dakin K , Petzold M , Blennow K , Zetterberg H ((2016) ) CSF and blood biomarkers for the diagnosis of Alzheimer’s disease: A systematic review and meta-analysis. Lancet Neurol 15: , 673–684. |
[5] | Zetterberg H , Skillbäck T , Mattsson N , Trojanowski JQ , Portelius E , Shaw LM , Weiner MW , Blennow K ((2016) ) Association of cerebrospinal fluid neurofilament light concentration with Alzheimer disease progression. JAMA Neurol 73: , 60. |
[6] | Lista S , Toschi N , Baldacci F , Zetterberg H , Blennow K , Kilimann I , Teipel SJ , Cavedo E , dos Santos AM , Epelbaum S , Lamari F , Dubois B , Floris R , Garaci F , Hampel H ((2017) ) Diagnostic accuracy of CSF neurofilament light chain protein in the biomarker-guided classification system for Alzheimer’s disease. Neurochem Int 108: , 355–360. |
[7] | Lista S , Hampel H ((2016) ) Synaptic degeneration and neurogranin in the pathophysiology of Alzheimer’s disease. Expert Rev Neurother 17: , 47–57. |
[8] | Lista S , Toschi N , Baldacci F , Zetterberg H , Blennow K , Kilimann I , Teipel SJ , Cavedo E , dos Santos AM , Epelbaum S , Lamari F , Dubois B , Nisticò R , Floris R , Garaci F , Hampel H ((2017) ) Cerebrospinal fluid neurogranin as a biomarker of neurodegenerative diseases: A cross-sectional study. J Alzheimers Dis 59: , 1327–1334. |
[9] | Spires-Jones Tara L , Hyman Bradley T ((2014) ) The intersection of amyloid beta and tau at synapses in Alzheimer’s disease. Neuron 82: , 756–771. |
[10] | Husain M ((2017) ) Alzheimer’s disease: Time to focus on the brain, not just molecules. Brain 140: , 251–253. |
[11] | Legato MJ , Johnson PA , Manson JE ((2016) ) Consideration of sex differences in medicine to improve health care and patient outcomes. JAMA 316: , 1865. |
[12] | Irvine K , Laws KR , Gale TM , Kondel TK ((2012) ) Greater cognitive deterioration in women than men with Alzheimer’s disease: A meta analysis. J Clin Exp Neuropsychol 34: , 989–998. |
[13] | Lin KA , Choudhury KR , Rathakrishnan BG , Marks DM , Petrella JR , Doraiswamy PM , Alzheimer’s Disease Neuroimaging Initiative ((2015) ) Marked gender differences in progression of mild cognitive impairment over 8 years. Alzheimers Dement (N Y) 1: , 103–110. |
[14] | Hua X , Hibar DP , Lee S , Toga AW , Jack CR , Weiner MW , Thompson PM ((2010) ) Sex and age differences in atrophic rates: An ADNI study with n=1368 MRI scans. Neurobiol Aging 31: , 1463–1480. |
[15] | Mattsson N , Lönneborg A , Boccardi M , Blennow K , Hansson O ((2017) ) Clinical validity of cerebrospinal fluid Aβ42, tau, and phospho-tau as biomarkers for Alzheimer’s disease in the context of a structured 5-phase development framework. Neurobiol Aging 52: , 196–213. |
[16] | Altmann A , Tian L , Henderson VW , Greicius MD ((2014) ) Sex modifies theAPOE-related risk of developing Alzheimer disease. Ann Neurol 75: , 563–573. |
[17] | Depypere H , Vierin A , Weyers S , Sieben A ((2016) ) Alzheimer’s disease, apolipoprotein E and hormone replacement therapy. Maturitas 94: , 98–105. |
[18] | Scacchi R , Gambina G , Broggio E , Corbo RM ((2013) ) Sex and ESR1 genotype may influence the response to treatment with donepezil and rivastigmine in patients with Alzheimer’s disease. Int J Geriatr Psychiatry 29: , 610–615. |
[19] | ((2014) ) 2014 Alzheimer’s disease facts and figures. Alzheimers Dement 10: , e47–e92. |
[20] | Hampel H , O’Bryant SE , Castrillo JI , Ritchie C , Rojkova K , Broich K , Benda N , Nistico R , Frank RA , Dubois B , Escott-Price V , Lista S ((2016) ) PRECISION MEDICINE - The Golden Gate for detection, treatment and prevention of Alzheimer’s disease. J Prev Alzheimers Dis 3: , 243–259. |
[21] | Hampel H , O’Bryant SE , Durrleman S , Younesi E , Rojkova K , Escott-Price V , Corvol JC , Broich K , Dubois B , Lista S ((2017) ) A Precision Medicine Initiative for Alzheimer’s disease: The road ahead to biomarker-guided integrative disease modeling. Climacteric 20: , 107–118. |
[22] | Lista S , Khachaturian ZS , Rujescu D , Garaci F , Dubois B , Hampel H ((2016) ) Application of systems theory in longitudinal studies on the origin and progression of Alzheimer’s disease. Methods Mol Biol 1303: , 49–67. |
[23] | Younesi E , Hofmann-Apitius M ((2013) ) From integrative disease modeling to predictive, preventive, personalized and participatory (P4) medicine. EPMA J 4: , 23. |
[24] | FDA approves first cancer treatment for any solid tumor with aspecific genetic feature.,https://www.fda.gov/newsevents/newsroom/pressannouncements/ucm560167.htm. 2017. |
[25] | Imamura K , Izumi Y , Watanabe A , Tsukita K , Woltjen K , Yamamoto T , Hotta A , Kondo T , Kitaoka S , Ohta A , Tanaka A , Watanabe D , Morita M , Takuma H , Tamaoka A , Kunath T , Wray S , Furuya H , Era T , Makioka K , Okamoto K , Fujisawa T , Nishitoh H , Homma K , Ichijo H , Julien J-P , Obata N , Hosokawa M , Akiyama H , Kaneko S , Ayaki T , Ito H , Kaji R , Takahashi R , Yamanaka S , Inoue H ((2017) ) The Src/c-Abl pathway is a potential therapeutic target in amyotrophic lateral sclerosis. , eaaf. Sci Transl Med 9: , 3962. |
[26] | Certain Cancer Drugs Held Out as Prospects for ALS Therapy, http://www.alzforum.org/news/research-news/certain-cancer-drugs-held-out-prospects-als-therapy. 2017. |
[27] | Hampel H , Lista S , Teipel SJ , Garaci F , Nisticò R , Blennow K , Zetterberg H , Bertram L , Duyckaerts C , Bakardjian H , Drzezga A , Colliot O , Epelbaum S , Broich K , Lehéricy S , Brice A , Khachaturian ZS , Aisen PS , Dubois B ((2014) ) Perspective on future role of biological markers in clinical therapy trials of Alzheimer’s disease: A long-range point of view beyond 2020. Biochem Pharmacol 88: , 426–449. |
[28] | Lista S , Garaci FG , Ewers M , Teipel S , Zetterberg H , Blennow K , Hampel H ((2014) ) CSF Aβ1-42 combined with neuroimaging biomarkers in the early detection, diagnosis and prediction of Alzheimer’s disease. Alzheimers Dement 10: , 381–392. |
[29] | Cavedo E , Lista S , Khachaturian Z , Aisen P , Amouyel P , Herholz K , Jack CRJr , Sperling R , Cummings J , Blennow K , O’Bryant S , Frisoni GB , Khachaturian A , Kivipelto M , Klunk W , Broich K , Andrieu S , de Schotten MT , Mangin JF , Lammertsma AA , Johnson K , Teipel S , Drzezga A , Bokde A , Colliot O , Bakardjian H , Zetterberg H , Dubois B , Vellas B , Schneider LS , Hampel H ((2014) ) The road ahead to cure Alzheimer’s disease: Development of biological markers and neuroimaging methods for prevention trials across all stages and target populations. J Prev Alzheimers Dis 1: , 181–202. |
[30] | Hampel H , Lista S ((2013) ) Use of biomarkers and imaging to assess pathophysiology, mechanisms of action and target engagement. J Nutr Health Aging 17: , 54–63. |
[31] | Lista S , Garaci F , Toschi N , Hampel H ((2013) ) Biochemical and imaging markers for the diagnosis of Alzheimer’s disease: An overview. Eur J Neurodegener Dis 2: , 143–158. |
[32] | Teipel SJ , Sabri O , Grothe M , Barthel H , Prvulovic D , Buerger K , Bokde ALW , Ewers M , Hoffmann W , Hampel H ((2013) ) Perspectives for multimodal neurochemical and imaging biomarkers in Alzheimer’s disease. J Alzheimers Dis 33: , S329–S347. |
[33] | Hampel H , Lista S , Khachaturian ZS ((2012) ) Development of biomarkers to chart all Alzheimer’s disease stages: The royal road to cutting the therapeutic Gordian Knot. Alzheimers Dement 8: , 312–336. |
[34] | Lista S , Emanuele E ((2011) ) Role of amyloid β1–42 and neuroimaging biomarkers in Alzheimer’s disease. Biomark Med 5: , 411–413. |
[35] | Hampel H , Lista S ((2016) ) Dementia: The rising global tide of cognitive impairment. Nat Rev Neurol 12: , 131–132. |
[36] | Toledo JB , Zetterberg H , van Harten AC , Glodzik L , Martinez-Lage P , Bocchio-Chiavetto L , Rami L , Hansson O , Sperling R , Engelborghs S , Osorio RS , Vanderstichele H , Vandijck M , Hampel H , Teipl S , Moghekar A , Albert M , Hu WT , Monge Argilés JA , Gorostidi A , Teunissen CE , De Deyn PP , Hyman BT , Molinuevo JL , Frisoni GB , Linazasoro G , de Leon MJ , van der Flier WM , Scheltens P , Blennow K , Shaw LM , Trojanowski JQ ((2015) ) Alzheimer’s disease cerebrospinal fluid biomarker in cognitively normal subjects. Brain 138: , 2701–2715. |
[37] | van Harten AC , Visser PJ , Pijnenburg YAL , Teunissen CE , Blankenstein MA , Scheltens P , van der Flier WM ((2013) ) Cerebrospinal fluid Aβ42 is the best predictor of clinical progression in patients with subjective complaints. Alzheimers Dement 9: , 481–487. |
[38] | Lista S , Molinuevo JL , Cavedo E , Rami L , Amouyel P , Teipel SJ , Garaci F , Toschi N , Habert M-O , Blennow K , Zetterberg H , O’Bryant SE , Johnson L , Galluzzi S , Bokde ALW , Broich K , Herholz K , Bakardjian H , Dubois B , Jessen F , Carrillo MC , Aisen PS , Hampel H ((2015) ) Evolving evidence for the value of neuroimaging methods and biological markers in subjects categorized with subjective cognitive decline. J Alzheimers Dis 48: , S171–S191. |
[39] | Wolfsgruber S , Polcher A , Koppara A , Kleineidam L , Frölich L , Peters O , Hüll M , Rüther E , Wiltfang J , Maier W , Kornhuber J , Lewczuk P , Jessen F , Wagner M ((2017) ) Cerebrospinal fluid biomarkers and clinical progression in patients with subjective cognitive decline and mild cognitive impairment. J Alzheimers Dis 58: , 939–950. |
[40] | Herukka S-K , Simonsen AH , Andreasen N , Baldeiras I , Bjerke M , Blennow K , Engelborghs S , Frisoni GB , Gabryelewicz T , Galluzzi S , Handels R , Kramberger MG , Kulczyńska A , Molinuevo JL , Mroczko B , Nordberg A , Oliveira CR , Otto M , Rinne JO , Rot U , Saka E , Soininen H , Struyfs H , Suardi S , Visser PJ , Winblad B , Zetterberg H , Waldemar G ((2017) ) Recommendations for cerebrospinal fluid Alzheimer’s disease biomarkers in the diagnostic evaluation of mild cognitive impairment. Alzheimers Dement 13: , 285–295. |
[41] | Simonsen AH , Herukka S-K , Andreasen N , Baldeiras I , Bjerke M , Blennow K , Engelborghs S , Frisoni GB , Gabryelewicz T , Galluzzi S , Handels R , Kramberger MG , Kulczyńska A , Molinuevo JL , Mroczko B , Nordberg A , Oliveira CR , Otto M , Rinne JO , Rot U , Saka E , Soininen H , Struyfs H , Suardi S , Visser PJ , Winblad B , Zetterberg H , Waldemar G ((2017) ) Recommendations for CSF AD biomarkers in the diagnostic evaluation of dementia. Alzheimers Dement 13: , 274–284. |
[42] | Lista S , Garaci F , Toschi N , Hampel H ((2013) ) Imaging epigenetics in Alzheimer’s disease. Curr Pharm Des 19: , 6393–6415. |
[43] | Hampel H , Lista S ((2012) ) Alzheimer disease: From inherited to sporadic AD—crossing the biomarker bridge. Nat Rev Neurol 8: , 598–600. |
[44] | Faltraco F , Lista S , Garaci FG , Hampel H ((2012) ) Epigenetic mechanisms in Alzheimer’s disease: State-of-the-art. Eur J Neurodegener Dis 1: , 1–19. |
[45] | Zetzsche T , Rujescu D , Hardy J , Hampel H ((2010) ) Advances and perspectives from genetic research: Development of biological markers in Alzheimer’s disease. Expert Rev Mol Diagn 10: , 667–690. |
[46] | Lista S , O’Bryant SE , Blennow K , Dubois B , Hugon J , Zetterberg H , Hampel H ((2015) ) Biomarkers in sporadic and familial Alzheimer’s disease. J Alzheimers Dis 47: , 291–317. |
[47] | Rosén C , Hansson O , Blennow K , Zetterberg H ((2013) ) Fluid biomarkers in Alzheimer’s disease – current concepts. Mol Neurodegener 8: , 20. |
[48] | Blennow K , Zetterberg H , Fagan AM ((2012) ) Fluid biomarkers in Alzheimer disease. Cold Spring Harb Perspect Med 2: , a006221–a006221. |
[49] | Blennow K , Dubois B , Fagan AM , Lewczuk P , de Leon MJ , Hampel H ((2015) ) Clinical utility of cerebrospinal fluid biomarkers in the diagnosis of early Alzheimer’s disease. Alzheimers Dement 11: , 58–69. |
[50] | Zetterberg H , Lautner R , Skillbäck T , Rosén C , Shahim P , Mattsson N , Blennow K ((2014) ) CSF in Alzheimer’s disease. Adv Clin Chem 65: , 143–172. |
[51] | Ghidoni R , Benussi L , Paterlini A , Albertini V , Binetti G , Emanuele E ((2011) ) Cerebrospinal fluid biomarkers for Alzheimer’s disease: The present and the future. Neurodegener Dis 8: , 413–420. |
[52] | Blennow K , Hampel H , Weiner M , Zetterberg H ((2010) ) Cerebrospinal fluid and plasma biomarkers in Alzheimer disease. Nat Rev Neurol 6: , 131–144. |
[53] | Hampel H , Frank R , Broich K , Teipel SJ , Katz RG , Hardy J , Herholz K , Bokde ALW , Jessen F , Hoessler YC , Sanhai WR , Zetterberg H , Woodcock J , Blennow K ((2010) ) Biomarkers for Alzheimer’s disease: Academic industry and regulatory perspectives. Nat Rev Drug Discov 9: , 560–574. |
[54] | Hampel H , Shen Y , Walsh DM , Aisen P , Shaw LM , Zetterberg H , Trojanowski JQ , Blennow K ((2010) ) Biological markers of amyloid β-related mechanisms in Alzheimer’s disease. Exp Neurol 223: , 334–346. |
[55] | Hampel H , Blennow K , Shaw LM , Hoessler YC , Zetterberg H , Trojanowski JQ ((2010) ) Total and phosphorylated tau protein as biological markers of Alzheimer’s disease. Exp Gerontol 45: , 30–40. |
[56] | O’Bryant SE , Mielke MM , Rissman RA , Lista S , Vanderstichele H , Zetterberg H , Lewczuk P , Posner H , Hall J , Johnson L , Fong Y-L , Luthman J , Jeromin A , Batrla-Utermann R , Villarreal A , Britton G , Snyder PJ , Henriksen K , Grammas P , Gupta V , Martins R , Hampel H ((2017) ) Blood-based biomarkers in Alzheimer disease: Current state of the science and a novel collaborative paradigm for advancing from discovery to clinic. Alzheimers Dement 13: , 45–58. |
[57] | O’Bryant SE , Lista S , Rissman RA , Edwards M , Zhang F , Hall J , Zetterberg H , Lovestone S , Gupta V , Graff-Radford N , Martins R , Jeromin A , Waring S , Oh E , Kling M , Baker LD , Hampel H ((2016) ) Comparing biological markers of Alzheimer’s disease across blood fraction and platforms: Comparing apples to oranges. Alzheimers Dement (Amst) 3: , 27–34. |
[58] | O’Bryant SE , Gupta V , Henriksen K , Edwards M , Jeromin A , Lista S , Bazenet C , Soares H , Lovestone S , Hampel H , Montine T , Blennow K , Foroud T , Carrillo M , Graff-Radford N , Laske C , Breteler M , Shaw L , Trojanowski JQ , Schupf N , Rissman RA , Fagan AM , Oberoi P , Umek R , Weiner MW , Grammas P , Posner H , Martins R ((2015) ) Guidelines for the standardization of preanalytic variables for blood-based biomarker studies in Alzheimer’s disease research. Alzheimers Dement 11: , 549–560. |
[59] | Henriksen K , O’Bryant SE , Hampel H , Trojanowski JQ , Montine TJ , Jeromin A , Blennow K , Lönneborg A , Wyss-Coray T , Soares H , Bazenet C , Sjögren M , Hu W , Lovestone S , Karsdal MA , Weiner MW ((2014) ) The future of blood-based biomarkers for Alzheimer’s disease. Alzheimers Dement 10: , 115–131. |
[60] | Snyder HM , Carrillo MC , Grodstein F , Henriksen K , Jeromin A , Lovestone S , Mielke MM , O’Bryant S , Sarasa M , Sjögren M , Soares H , Teeling J , Trushina E , Ward M , West T , Bain LJ , Shineman DW , Weiner M , Fillit HM ((2014) ) Developing novel blood-based biomarkers for Alzheimer’s disease. Alzheimers Dement 10: , 109–114. |
[61] | Gupta V , Sundaram R , Martins RN ((2013) ) Multiplex biomarkers in blood. Alzheimers Res Ther 5: , 31. |
[62] | Lista S , Faltraco F , Prvulovic D , Hampel H ((2013) ) Blood and plasma-based proteomic biomarker research in Alzheimer’s disease. Prog Neurobiol 101-102: , 1–17. |
[63] | Lista S , Faltraco F , Hampel H ((2013) ) Biological and methodical challenges of blood-based proteomics in the field of neurological research. Prog Neurobiol 101-102: , 18–34. |
[64] | Teipel SJ , Grothe M , Lista S , Toschi N , Garaci FG , Hampel H ((2013) ) Relevance of magnetic resonance imaging for early detection and diagnosis of Alzheimer disease. Med Clin North Am 97: , 399–424. |
[65] | Ewers M , Sperling RA , Klunk WE , Weiner MW , Hampel H ((2011) ) Neuroimaging markers for the prediction and early diagnosis of Alzheimer’s disease dementia. Trends Neurosci 34: , 430–442. |
[66] | Ewers M , Frisoni GB , Teipel SJ , Grinberg LT , Amaro E Jr , Heinsen H , Thompson PM , Hampel H ((2011) ) Staging Alzheimer’s disease progression with multimodality neuroimaging. Prog Neurobiol 95: , 535–546. |
[67] | Hampel H , Prvulovic D , Teipel SJ , Bokde ALW ((2011) ) Recent developments of functional magnetic resonance imaging research for drug development in Alzheimer’s disease. Prog Neurobiol 95: , 570–578. |
[68] | Chiesa PA , Cavedo E , Lista S , Thompson PM , Hampel H , Initiative APM ((2017) ) Revolution of resting-state functional neuroimaging genetics in Alzheimer’s disease. Trends Neurosci 40: , 469–480. |
[69] | Engels MMA , van der Flier WM , Stam CJ , Hillebrand A , Scheltens P , van Straaten ECW ((2017) ) Alzheimer’s disease: The state of the art in resting-state magnetoencephalography. Clin Neurophysiol 128: , 1426–1437. |
[70] | Broich K , Weiergräber M , Hampel H ((2011) ) Biomarkers in clinical trials for neurodegenerative diseases: Regulatory perspectives and requirements. Prog Neurobiol 95: , 498–500. |
[71] | Nisticò G , Broich K , Hampel H ((2013) ) Need for new guidelines for Alzheimer’s disease clinical trials. Eur J Neurodegener Dis 2: , 181–186. |
[72] | Jørgensen JT ((2015) ) Companion diagnostics: The key to personalized medicine. Expert Rev Mol Diagn 15: , 153–156. |
[73] | Lyman GH , Moses HL ((2016) ) Biomarker tests for molecularly targeted therapies —the key to unlocking precision medicine. N Engl J Med 375: , 4–6. |
[74] | Daly AK ((2010) ) Pharmacogenetics and human genetic polymorphisms. Biochem J 429: , 435–449. |
[75] | Guerreiro R , Wojtas A , Bras J , Carrasquillo M , Rogaeva E , Majounie E , Cruchaga C , Sassi C , Kauwe JSK , Younkin S , Hazrati L , Collinge J , Pocock J , Lashley T , Williams J , Lambert J-C , Amouyel P , Goate A , Rademakers R , Morgan K , Powell J , St. George-Hyslop P , Singleton A , Hardy J ((2013) ) TREM2 variants in Alzheimer’s disease. N Engl J Med 368: , 117–127. |
[76] | Escott-Price V , Shoai M , Pither R , Williams J , Hardy J ((2017) ) Polygenic score prediction captures nearly all common genetic risk for Alzheimer’s disease. Neurobiol Aging 49: , 214.e217–214.e211. |
[77] | Rehm HL ((2017) ) Evolving health care through personal genomics. Nat Rev Genet 18: , 259–267. |
[78] | Zhao SG , Chang SL , Erho N , Yu M , Lehrer J , Alshalalfa M , Speers C , Cooperberg MR , Kim W , Ryan CJ , Den RB , Freedland SJ , Posadas E , Sandler H , Klein EA , Black P , Seiler R , Tomlins SA , Chinnaiyan AM , Jenkins RB , Davicioni E , Ross AE , Schaeffer EM , Nguyen PL , Carroll PR , Karnes RJ , Spratt DE , Feng FY ((2017) ) Associations of luminal and basal subtyping of prostate cancer with prognosis and response to androgen deprivation therapy. JAMA Oncol 3: , 1663–1672. |
[79] | Deyati A , Younesi E , Hofmann-Apitius M , Novac N ((2013) ) Challenges and opportunities for oncology biomarker discovery. Drug Discovery Today 18: , 614–624. |
[80] | Cross R ((2017) ) This $25,000 physical has found some ‘serious’health problems. Others say it has serious problems. Science,http://www.sciencemag.org/news/2017/05/25000-physical-has-found-some-serious-health-problems-others-say-it-has-serious |
[81] | Vassy JL , Christensen KD , Schonman EF , Blout CL , Robinson JO , Krier JB , Diamond PM , Lebo M , Machini K , Azzariti DR , Dukhovny D , Bates DW , MacRae CA , Murray MF , Rehm HL , McGuire AL , Green RC ((2017) ) The impact of whole-genome sequencing on the primary care and outcomes of healthy adult patients. Ann Intern Med 167: , 159. |
[82] | Weston AD , Hood L ((2004) ) Systems biology, proteomics, and the future of health care: Toward predictive, preventative, and personalized medicine. J Proteome Res 3: , 179–196. |
[83] | FDA-NIH Biomarker Working Group ((2016) ) BEST (Biomarkers, EndpointS, and other Tools) Resource. Available at: https://www.ncbi.nlm.nih.gov/books/NBK326791/, Silver Spring (MD); Food and Drug Administration (US); National Institutes of Health (US), Bethesda (MD). |
[84] | Haas M , Stephenson D , Romero K , Gordon MF , Zach N , Geerts H , Brain Health Modeling Initiative (BHMI) ((2016) ) Big data to smart data in Alzheimer’s disease: Real-world examples of advanced modeling and simulation. Alzheimers Dement 12: , 1022–1030. |
[85] | Vidal M ((2009) ) A unifying view of 21st century systems biology. FEBS Lett 583: , 3891–3894. |
[86] | Friend SH ((2010) ) The need for precompetitive integrative bionetwork disease model building. Clin Pharmacol Ther 87: , 536–539. |
[87] | Kitano H ((2002) ) Systems biology: A brief overview. Science 295: , 1662–1664. |
[88] | Vidal M , Cusick ME , Barabasi AL ((2011) ) Interactome networks and human disease. Cell 144: , 986–998. |
[89] | Martins R , Lithgow GJ , Link W ((2016) ) Long live FOXO: Unraveling the role of FOXO proteins in aging and longevity. Aging Cell 15: , 196–207. |
[90] | Castrillo JI , Oliver SG ((2016) ) Alzheimer’s as a systems-level disease involving the interplay of multiple cellular networks. Methods Mol Biol 1303: , 3–48. |
[91] | Jiang H , Xu Z , Zhong P , Ren Y , Liang G , Schilling HA , Hu Z , Zhang Y , Wang X , Chen S ((2015) ) Cell cycle and p53 gate the direct conversion of human fibroblasts to dopaminergic neurons. Nat Commun 6: , 10100. |
[92] | Masdeu JC , Kreisl WC , Berman KF ((2012) ) The neurobiology of Alzheimer disease defined by neuroimaging. Curr Opin Neurol 25: , 410–420. |
[93] | Sepulcre J , Sabuncu MR , Becker A , Sperling R , Johnson KA ((2013) ) In vivo characterization of the early states of the amyloid-beta network. Brain 136: , 2239–2252. |
[94] | Leuzy A , Zimmer ER , Heurling K , Rosa-Neto P , Gauthier S ((2014) ) Use of amyloid PET across the spectrum of Alzheimer’s disease: Clinical utility and associated ethical issues. Amyloid 21: , 143–148. |
[95] | Zimmer ER , Leuzy A , Bhat V , Gauthier S , Rosa-Neto P ((2014) ) In vivo tracking of tau pathology using positron emission tomography (PET) molecular imaging in small animals. Transl Neurodegener 3: , 6. |
[96] | Hood L , Flores M ((2012) ) A personal view on systems medicine and the emergence of proactive P4 medicine: Predictive, preventive, personalized and participatory. N Biotechnol 29: , 613–624. |
[97] | Lausted C , Lee I , Zhou Y , Qin S , Sung J , Price ND , Hood L , Wang K ((2014) ) Systems approach to neurodegenerative disease biomarker discovery. Annu Rev Pharmacol Toxicol 54: , 457–481. |
[98] | Hood L , Tian Q ((2012) ) Systems approaches to biology and disease enable translational systems medicine. Genomics Proteomics Bioinformatics 10: , 181–185. |
[99] | Hood L , Auffray C ((2013) ) Participatory medicine: A driving force for revolutionizing healthcare. Genome Med 5: , 110. |
[100] | Yan J , Risacher SL , Shen L , Saykin AJ ((2017) ) Network approaches to systems biology analysis of complex disease: Integrative methods for multi-omics data. Brief Bioinform. doi: 10.1093/bib/bbx066 |
[101] | Gligorijević V , Pržulj N ((2015) ) Methods for biological data integration: Perspectives and challenges. J R Soc Interface 12: , 20150571. |
[102] | Tourette C , Farina F , Vazquez-Manrique RP , Orfila A-M , Voisin J , Hernandez S , Offner N , Parker JA , Menet S , Kim J ((2014) ) The Wnt receptor Ryk reduces neuronal and cell survival capacity by repressing FOXO activity during the early phases of mutant huntingtin pathogenicity. PLoS Biol 12: , e1001895. |
[103] | Miller JA , Guillozet-Bongaarts A , Gibbons LE , Postupna N , Renz A , Beller AE , Sunkin SM , Ng L , Rose SE , Smith KA ((2017) ) Neuropathological and transcriptomic characteristics of the aged brain. eLife 6: , e31126. |
[104] | Bielza C , Larrañaga P ((2014) ) Bayesian networks in neuroscience: A survey. Front Comput Neurosci 8: , 131. |
[105] | Hristoskova A , Boeva V , Tsiporkova E ((2014) ) A formal concept analysis approach to consensus clustering of multi-experiment expression data. BMC Bioinformatics 15: , 151. |
[106] | Farhan S , Fahiem MA , Tauseef H ((2014) ) An ensemble-of-classifiers based approach for early diagnosis of Alzheimer’s disease: Classification using structural features of brain images. Comput Math Methods Med 2014: , 862307. |
[107] | Zhang Q , Abel H , Wells A , Lenzini P , Gomez F , Province MA , Templeton AA , Weinstock GM , Salzman NH , Borecki IB ((2015) ) Selection of models for the analysis of risk-factor trees: Leveraging biological knowledge to mine large sets of risk factors with application to microbiome data. Bioinformatics 31: , 1607–1613. |
[108] | Ritchie CW , Molinuevo JL , Truyen L , Satlin A , Van der Geyten S , Lovestone S ((2016) ) Development of interventions for the secondary prevention of Alzheimer’s dementia: The European Prevention of Alzheimer’s Dementia (EPAD) project. Lancet Psychiatry 3: , 179–186. |
[109] | Schneider LS , Mangialasche F , Andreasen N , Feldman H , Giacobini E , Jones R , Mantua V , Mecocci P , Pani L , Winblad B , Kivipelto M ((2014) ) Clinical trials and late-stage drug development for Alzheimer’s disease: An appraisal from 1984 to 2014. J Intern Med 275: , 251–283. |
[110] | Ritchie K , Ropacki M , Albala B , Harrison J , Kaye J , Kramer J , Randolph C , Ritchie CW ((2017) ) Recommended cognitive outcomes in preclinical Alzheimer’s disease: Consensus statement from the European Prevention of Alzheimer’s Dementia project. Alzheimers Dement 13: , 186–195. |
[111] | Mortamais M , Ash JA , Harrison J , Kaye J , Kramer J , Randolph C , Pose C , Albala B , Ropacki M , Ritchie CW , Ritchie K ((2017) ) Detecting cognitive changes in preclinical Alzheimer’s disease: A review of its feasibility. Alzheimers Dement 13: , 468–492. |
[112] | Bassett DS , Sporns O ((2017) ) Network neuroscience. Nat Neurosci 20: , 353–364. |
[113] | Sejnowski TJ , Churchland PS , Movshon JA ((2014) ) Putting big data to good use in neuroscience. Nat Neurosci 17: , 1440–1441. |
[114] | Teipel S , Drzezga A , Grothe MJ , Barthel H , Chételat G , Schuff N , Skudlarski P , Cavedo E , Frisoni GB , Hoffmann W ((2015) ) Multimodal imaging in Alzheimer’s disease: Validity and usefulness for early detection. Lancet Neurol 14: , 1037–1053. |
[115] | Bullmore E , Sporns O ((2009) ) Complex brain networks: Graph theoretical analysis of structural and functional systems. Nat Rev Neurosci 10: , 186–198. |
[116] | Sporns O ((2014) ) Contributions and challenges for network models in cognitive neuroscience. Nat Neurosci 17: , 652–660. |
[117] | Medaglia JD , Lynall M-E , Bassett DS ((2015) ) Cognitive network neuroscience. J Cogn Neurosci 27: , 1471–1491. |
[118] | Sporns O ((2010) ). Networks of the Brain, MIT Press. |
[119] | Van Essen DC , Smith SM , Barch DM , Behrens TE , Yacoub E , Ugurbil K , WU-Minn HCP Consortium ((2013) ) The WU-Minn human connectome project: An overview. Neuroimage 80: , 62–79. |
[120] | Zuo X-N , He Y , Betzel RF , Colcombe S , Sporns O , Milham MP ((2017) ) Human connectomics across the life span. Trends Cogn Sci 21: , 32–45. |
[121] | Fornito A , Zalesky A , Bullmore E ((2016) ) Fundamentals of brain network analysis, Academic Press. |
[122] | Pini L , Pievani M , Bocchetta M , Altomare D , Bosco P , Cavedo E , Galluzzi S , Marizzoni M , Frisoni GB ((2016) ) Brain atrophy in Alzheimer’s disease and aging. Ageing Res Rev 30: , 25–48. |
[123] | Cavedo E , Frisoni G ((2011) ) The dynamic marker hypothesis of Alzheimer’s disease and its implications for clinical imaging. Q J Nucl Med Mol Imaging 55: , 237–249. |
[124] | Cavedo E , Redolfi A , Angeloni F , Babiloni C , Lizio R , Chiapparini L , Bruzzone MG , Aquino D , Sabatini U , Alesiani M ((2014) ) The Italian Alzheimer’s Disease Neuroimaging Initiative (I-ADNI): Validation of structural MR imaging. J Alzheimers Dis 40: , 941–952. |
[125] | Jack CR , Bernstein MA , Borowski BJ , Gunter JL , Fox NC , Thompson PM , Schuff N , Krueger G , Killiany RJ , DeCarli CS ((2010) ) Update on the magnetic resonance imaging core of the Alzheimer’s disease neuroimaging initiative. Alzheimers Dement 6: , 212–220. |
[126] | Braak H , Braak E ((1995) ) Staging of Alzheimer’s disease-related neurofibrillary changes. Neurobiol Aging 16: , 271–278. |
[127] | Delacourte A , David JP , Sergeant N , Buee L , Wattez A , Vermersch P , Ghozali F , Fallet-Bianco C , Pasquier F , Lebert F , Petit H , Di Menza C ((1999) ) The biochemical pathway of neurofibrillary degeneration in aging and Alzheimer’s disease. Neurology 52: , 1158–1158. |
[128] | de Souza LC , Chupin M , Lamari F , Jardel C , Leclercq D , Colliot O , Lehéricy S , Dubois B , Sarazin M ((2012) ) CSF tau markers are correlated with hippocampal volume in Alzheimer’s disease. Neurobiol Aging 33: , 1253–1257. |
[129] | Jack CR , Lowe VJ , Senjem ML , Weigand SD , Kemp BJ , Shiung MM , Knopman DS , Boeve BF , Klunk WE , Mathis CA , Petersen RC ((2008) ) 11C PiB and structural MRI provide complementary information in imaging of Alzheimer’s disease and amnestic mild cognitive impairment. Brain 131: , 665–680. |
[130] | Josephs KA , Whitwell JL , Ahmed Z , Shiung MM , Weigand SD , Knopman DS , Boeve BF , Parisi JE , Petersen RC , Dickson DW , Jack CR ((2008) ) β-amyloid burden is not associated with rates of brain atrophy. Ann Neurol 63: , 204–212. |
[131] | Ferrarini L , Palm WM , Olofsen H , van der Landen R , Jan Blauw G , Westendorp RGJ , Bollen ELEM , Middelkoop HAM , Reiber JHC , van Buchem MA , Admiraal-Behloul F ((2008) ) MMSE scores correlate with local ventricular enlargement in the spectrum from cognitively normal to Alzheimer disease. Neuroimage 39: , 1832–1838. |
[132] | Sarazin M , Chauviré V , Gerardin E , Colliot O , Kinkingnéhun S , de Souza LC , Hugonot-Diener L , Garnero L , Lehéricy S , Chupin M , Dubois B ((2010) ) The amnestic syndrome of hippocampal type in Alzheimer’s disease: An MRI study. J Alzheimers Dis 22: , 285–294. |
[133] | Fox NC , Cousens S , Scahill R , Harvey RJ , Rossor MN ((2000) ) Using serial registered brain magnetic resonance imaging to measure disease progression in Alzheimer disease. Arch Neurol 57: , 339. |
[134] | Fox NC , Scahill RI , Crum WR , Rossor MN ((1999) ) Correlation between rates of brain atrophy and cognitive decline in AD. Neurology 52: , 1687–1687. |
[135] | Jack CR , Petersen RC , Grundman M , Jin S , Gamst A , Ward CP , Sencakova D , Doody RS , Thal LJ ((2008) ) Longitudinal MRI findings from the vitamin E and donepezil treatment study for MCI. Neurobiol Aging 29: , 1285–1295. |
[136] | Baron JC , Chételat G , Desgranges B , Perchey G , Landeau B , de la Sayette V , Eustache F ((2001) ) In vivo mapping of gray matter loss with voxel-based morphometry in mild Alzheimer’s disease. Neuroimage 14: , 298–309. |
[137] | Singh V , Chertkow H , Lerch JP , Evans AC , Dorr AE , Kabani NJ ((2006) ) Spatial patterns of cortical thinning in mild cognitive impairment and Alzheimer’s disease. Brain 129: , 2885–2893. |
[138] | Cuingnet R , Glaunes JA , Chupin M , Benali H , Colliot O ((2013) ) Spatial and anatomical regularization of SVM: A general framework for neuroimaging data. IEEE Trans Pattern Anal Mach Intell 35: , 682–696. |
[139] | Davatzikos C , Fan Y , Wu X , Shen D , Resnick SM ((2008) ) Detection of prodromal Alzheimer’s disease via pattern classification of magnetic resonance imaging. Neurobiol Aging 29: , 514–523. |
[140] | Kloppel S , Stonnington CM , Chu C , Draganski B , Scahill RI , Rohrer JD , Fox NC , Jack CR , Ashburner J , Frackowiak RSJ ((2008) ) Automatic classification of MR scans in Alzheimer’s disease. Brain 131: , 681–689. |
[141] | Plant C , Teipel SJ , Oswald A , Böhm C , Meindl T , Mourao-Miranda J , Bokde AW , Hampel H , Ewers M ((2010) ) Automated detection of brain atrophy patterns based on MRI for the prediction of Alzheimer’s disease. Neuroimage 50: , 162–174. |
[142] | Cavedo E , Boccardi M , Ganzola R , Canu E , Beltramello A , Caltagirone C , Thompson P , Frisoni G ((2011) ) Local amygdala structural differences with 3T MRI in patients with Alzheimer disease. Neurology 76: , 727–733. |
[143] | Frisoni GB , Jack CR , Bocchetta M , Bauer C , Frederiksen KS , Liu Y , Preboske G , Swihart T , Blair M , Cavedo E ((2015) ) The EADC-ADNI Harmonized Protocol for manual hippocampal segmentation on magnetic resonance: Evidence of validity. Alzheimers Dement 11: , 111–125. |
[144] | Scheltens P , Launer LJ , Barkhof F , Weinstein HC , Gool WA ((1995) ) Visual assessment of medial temporal lobe atrophy on magnetic resonance imaging: Interobserver reliability. J Neurol 242: , 557–560. |
[145] | Cavedo E , Suppa P , Lange C , Opfer R , Lista S , Galluzzi S , Schwarz AJ , Spies L , Buchert R , Hampel H ((2017) ) Fully automatic MRI-based hippocampus volumetry using FSL-FIRST: Intra-scanner test-retest stability, inter-field strength variability, and performance as enrichment biomarker for clinical trials using prodromal target populations at risk for Alzheimer’s disease. J Alzheimers Dis 60: , 151–164. |
[146] | Ritter K , Lange C , Weygandt M , Mäurer A , Roberts A , Estrella M , Suppa P , Spies L , Prasad V , Steffen I ((2016) ) Combination of structural MRI and FDG-PET of the brain improves diagnostic accuracy in newly manifested cognitive impairment in geriatric inpatients. J Alzheimers Dis 54: , 1319–1331. |
[147] | Suppa P , Hampel H , Kepp T , Lange C , Spies L , Fiebach JB , Dubois B , Buchert R ((2016) ) Performance of hippocampus volumetry with FSL-first for prediction of Alzheimer’s disease dementia in at risk subjects with amnestic mild cognitive impairment. J Alzheimers Dis 51: , 867–873. |
[148] | Suppa P , Anker U , Spies L , Bopp I , Rüegger-Frey B , Klaghofer R , Gocke C , Hampel H , Beck S , Buchert R ((2015) ) Fully automated atlas-based hippocampal volumetry for detection of Alzheimer’s disease in a memory clinic setting. J Alzheimers Dis 44: , 183–193. |
[149] | Boutet C , Chupin M , Colliot O , Sarazin M , Mutlu G , Drier A , Pellot A , Dormont D , Lehéricy S ((2012) ) Is radiological evaluation as good as computer-based volumetry to assess hippocampal atrophy in Alzheimer’s disease? Neuroradiology 54: , 1321–1330. |
[150] | Colliot O , Chételat G , Chupin M , Desgranges B , Magnin B , Benali H , Dubois B , Garnero L , Eustache F , Lehéricy S ((2008) ) Discrimination between Alzheimer disease, mild cognitive impairment, and normal aging by using automated segmentation of the hippocampus. Radiology 248: , 194–201. |
[151] | Bakkour A , Morris JC , Dickerson BC ((2008) ) The cortical signature of prodromal AD: Regional thinning predicts mild AD dementia. Neurology 72: , 1048–1055. |
[152] | Desikan RS , Cabral HJ , Hess CP , Dillon WP , Glastonbury CM , Weiner MW , Schmansky NJ , Greve DN , Salat DH , Buckner RL , Fischl B ((2009) ) Automated MRI measures identify individuals with mild cognitive impairment and Alzheimer’s disease. Brain 132: , 2048–2057. |
[153] | Jack CR , Petersen RC , Xu YC , O’Brien PC , Smith GE , Ivnik RJ , Boeve BF , Waring SC , Tangalos EG , Kokmen E ((1999) ) Prediction of AD with MRI-based hippocampal volume in mild cognitive impairment. Neurology 52: , 1397–1397. |
[154] | Landau SM , Harvey D , Madison CM , Reiman EM , Foster NL , Aisen PS , Petersen RC , Shaw LM , Trojanowski JQ , Jack CR , Weiner MW , Jagust WJ ((2010) ) Comparing predictors of conversion and decline in mild cognitive impairment. Neurology 75: , 230–238. |
[155] | Visser P , Verhey F , Hofman P , Scheltens P , Jolles J ((2002) ) Medial temporal lobe atrophy predicts Alzheimer’s disease in patients with minor cognitive impairment. J Neurol Neurosurg Psychiatry 72: , 491–497. |
[156] | de Souza L , Chupin M , Bertoux M , Lehéricy S , Dubois B , Lamari F , Le Ber I , Bottlaender M , Colliot O , Sarazin M ((2013) ) Is hippocampal volume a good marker to differentiate Alzheimer’s disease from frontotemporal dementia?. Alzheimers Dement 9: , P380–P381. |
[157] | Hashimoto M , Kitagaki H , Imamura T , Hirono N , Shimomura T , Kazui H , Tanimukai S , Hanihara T , Mori E ((1998) ) Medial temporal and whole-brain atrophy in dementia with Lewy bodies: A volumetric MRI study. Neurology 51: , 357–362. |
[158] | Boutet C , Chupin M , Lehéricy S , Marrakchi-Kacem L , Epelbaum S , Poupon C , Wiggins C , Vignaud A , Hasboun D , Defontaines B , Hanon O , Dubois B , Sarazin M , Hertz-Pannier L , Colliot O ((2014) ) Detection of volume loss in hippocampal layers in Alzheimer’s disease using 7 T MRI: A feasibility study. Neuroimage Clin 5: , 341–348. |
[159] | Wisse LEM , Biessels GJ , Heringa SM , Kuijf HJ , Koek DL , Luijten PR , Geerlings MI ((2014) ) Hippocampal subfield volumes at 7T in early Alzheimer’s disease and normal aging. Neurobiol Aging 35: , 2039–2045. |
[160] | Kilimann I , Grothe M , Heinsen H , Alho EJL , Grinberg L , Amaro E Jr , Dos Santos GAB , Da Silva RE , Mitchell AJ , Frisoni GB ((2014) ) Subregional basal forebrain atrophy in Alzheimer’s disease: A multicenter study. J Alzheimers Dis 40: , 687–700. |
[161] | Pepeu G , Grazia Giovannini M ((2017) ) The fate of the brain cholinergic neurons in neurodegenerative diseases. Brain Res 1670: , 173–184. |
[162] | Brüggen K , Dyrba M , Barkhof F , Hausner L , Filippi M , Nestor PJ , Hauenstein K , Klöppel S , Grothe MJ , Kasper E ((2015) ) Basal forebrain and hippocampus as predictors of conversion to Alzheimer’s disease in patients with mild cognitive impairment– A multicenter DTI and volumetry study. J Alzheimers Dis 48: , 197–204. |
[163] | Grothe M , Heinsen H , Teipel SJ ((2012) ) Atrophy of the cholinergic basal forebrain over the adult age range and in early stages of Alzheimer’s disease. Biol Psychiatry 71: , 805–813. |
[164] | Zhang H , Trollor JN , Wen W , Zhu W , Crawford JD , Kochan NA , Slavin MJ , Brodaty H , Reppermund S , Kang K ((2011) ) Grey matter atrophy of basal forebrain and hippocampus in mild cognitive impairment. J Neurol Neurosurg Psychiatry 82: , 487–493. |
[165] | Grothe MJ , Heinsen H , Amaro E Jr , Grinberg LT , Teipel SJ ((2015) ) Cognitive correlates of basal forebrain atrophy and associated cortical hypometabolism in mild cognitive impairment. Cereb Cortex 26: , 2411–2426. |
[166] | Teipel SJ , Meindl T , Grinberg L , Grothe M , Cantero JL , Reiser MF , Möller HJ , Heinsen H , Hampel H ((2011) ) The cholinergic system in mild cognitive impairment and Alzheimer’s disease: An in vivo MRI and DTI study. Hum Brain Mapp 32: , 1349–1362. |
[167] | Teipel SJ , Flatz WH , Heinsen H , Bokde AL , Schoenberg SO , Stöckel S , Dietrich O , Reiser MF , Möller H-J , Hampel H ((2005) ) Measurement of basal forebrain atrophy in Alzheimer’s disease using MRI. Brain 128: , 2626–2644. |
[168] | Schmitz TW , Nathan Spreng R , Alzheimer’s Disease Neuroimaging Initiative ((2016) ) Basal forebrain degeneration precedes and predicts the cortical spread of Alzheimer’s pathology. Nat Commun 7: , 13249. |
[169] | Grothe MJ , Ewers M , Krause B , Heinsen H , Teipel SJ , Alzheimer’s Disease Neuroimaging Initiative ((2014) ) Basal forebrain atrophy and cortical amyloid deposition in nondemented elderly subjects. Alzheimers Dement 10: , S344–S353. |
[170] | Kerbler GM , Fripp J , Rowe CC , Villemagne VL , Salvado O , Rose S , Coulson EJ , Alzheimer’s Disease Neuroimaging Initiative ((2015) ) Basal forebrain atrophy correlates with amyloid β burden in Alzheimer’s disease. Neuroimage Clin 7: , 105–113. |
[171] | Cuingnet R , Gerardin E , Tessieras J , Auzias G , Lehéricy S , Habert M-O , Chupin M , Benali H , Colliot O ((2011) ) Automatic classification of patients with Alzheimer’s disease from structural MRI: A comparison of ten methods using the ADNI database. Neuroimage 56: , 766–781. |
[172] | Davatzikos C , Bhatt P , Shaw LM , Batmanghelich KN , Trojanowski JQ ((2011) ) Prediction of MCI to AD conversion, via MRI, CSF biomarkers, and pattern classification. Neurobiol Aging 32: , 2322.e2319–2322.e2327. |
[173] | Sun Z , van de Giessen M , Lelieveldt BPF , Staring M ((2017) ) Detection of conversion from mild cognitive impairment to Alzheimer’s disease using longitudinal brain MRI. Front Neuroinform 11: , 16. |
[174] | Cavedo E , Dubois B , Colliot O , Lista S , Croisile B , Tisserand GL , Touchon J , Bonafe A , Ousset PJ , Rouaud O , Ricolfi F , Vighetto A , Pasquier F , Galluzzi S , Delmaire C , Ceccaldi M , Girard N , Lehericy S , Duveau F , Chupin M , Sarazin M , Dormont D , Hampel H ((2016) ) Reduced regional cortical thickness rate of change in donepezil-treated subjects with suspected prodromal Alzheimer’s disease. J Clin Psychiatry 77: , e1631–e1638. |
[175] | Dubois B , Chupin M , Hampel H , Lista S , Cavedo E , Croisile B , Tisserand GL , Touchon J , Bonafe A , Ousset PJ ((2015) ) Donepezil decreases annual rate of hippocampal atrophy in suspected prodromal Alzheimer’s disease. Alzheimers Dement 11: , 1041–1049. |
[176] | Cavedo E , Grothe MJ , Colliot O , Lista S , Chupin M , Dormont D , Houot M , Lehéricy S , Teipel S , Dubois B ((2017) ) Reduced basal forebrain atrophy progression in a randomized Donepezil trial in prodromal Alzheimer’s disease. Sci Rep 7: , 11706. |
[177] | Fox NC , Black RS , Gilman S , Rossor MN , Griffith SG , Jenkins L , Koller M ((2005) ) Effects of A immunization (AN1792) on MRI measures of cerebral volume in Alzheimer disease. Neurology 64: , 1563–1572. |
[178] | Sperling R , Salloway S , Brooks DJ , Tampieri D , Barakos J , Fox NC , Raskind M , Sabbagh M , Honig LS , Porsteinsson AP , Lieberburg I , Arrighi HM , Morris KA , Lu Y , Liu E , Gregg KM , Brashear HR , Kinney GG , Black R , Grundman M ((2012) ) Amyloid-related imaging abnormalities in patients with Alzheimer’s disease treated with bapineuzumab: A retrospective analysis. Lancet Neurol 11: , 241–249. |
[179] | Bozzali M , Cherubini A ((2007) ) Diffusion tensor MRI to investigate dementias: A brief review. Magn Reson Imaging 25: , 969–977. |
[180] | Chua TC , Wen W , Slavin MJ , Sachdev PS ((2008) ) Diffusion tensor imaging in mild cognitive impairment and Alzheimer’s disease: A review. Curr Opin Neurol 21: , 83–92. |
[181] | Hess CP ((2009) ) Update on diffusion tensor imaging in Alzheimer’s disease. Magn Reson Imaging Clin N Am 17: , 215–224. |
[182] | Nucifora PG , Verma R , Lee SK , Melhem ER ((2007) ) Diffusion-tensor MR imaging and tractography: Exploring brain microstructure and connectivity. Radiology 245: , 367–384. |
[183] | Garaci FG , Bolacchi F , Cerulli A , Melis M , Spano A , Cedrone C , Floris R , Simonetti G , Nucci C ((2009) ) Optic nerve and optic radiation neurodegeneration in patients with glaucoma: In vivo analysis with 3-T diffusion-tensor MR imaging. Radiology 252: , 496–501. |
[184] | Pardini M , Elia M , Garaci FG , Guida S , Coniglione F , Krueger F , Benassi F , Emberti Gialloreti L ((2012) ) Long-term cognitive and behavioral therapies, combined with augmentative communication, are related to uncinate fasciculus integrity in autism. J Autism Dev Disord 42: , 585–592. |
[185] | Pardini M , Garaci FG , Bonzano L , Roccatagliata L , Palmieri MG , Pompili E , Coniglione F , Krueger F , Ludovici A , Floris R , Benassi F , Emberti Gialloreti L ((2009) ) White matter reduced streamline coherence in young men with autism and mental retardation. Eur J Neurol 16: , 1185–1190. |
[186] | Jones DK , Leemans A ((2011) ) Diffusion tensor imaging. Methods Mol Biol 711: , 127–144. |
[187] | Jones DK ((2008) ) Studying connections in the living human brain with diffusion MRI. Cortex 44: , 936–952. |
[188] | Jeurissen B , Tournier JD , Dhollander T , Connelly A , Sijbers J ((2014) ) Multi-tissue constrained spherical deconvolution for improved analysis of multi-shell diffusion MRI data. Neuroimage 103: , 411–426. |
[189] | Smith RE , Tournier JD , Calamante F , Connelly A ((2013) ) SIFT: Spherical-deconvolution informed filtering of tractograms. Neuroimage 67: , 298–312. |
[190] | Jeurissen B , Leemans A , Jones DK , Tournier JD , Sijbers J ((2011) ) Probabilistic fiber tracking using the residual bootstrap with constrained spherical deconvolution. Hum Brain Mapp 32: , 461–479. |
[191] | Tournier JD , Yeh CH , Calamante F , Cho KH , Connelly A , Lin CP ((2008) ) Resolving crossing fibres using constrained spherical deconvolution: Validation using diffusion-weighted imaging phantom data. Neuroimage 42: , 617–625. |
[192] | Giannelli M , Belmonte G , Toschi N , Pesaresi I , Ghedin P , Traino AC , Bartolozzi C , Cosottini M ((2011) ) Technical note: DTI measurements of fractional anisotropy and mean diffusivity at 1.5 T: Comparison of two radiofrequency head coils with different functional designs and sensitivities. Med Phys 38: , 3205–3211. |
[193] | Medina D , DeToledo-Morrell L , Urresta F , Gabrieli JD , Moseley M , Fleischman D , Bennett DA , Leurgans S , Turner DA , Stebbins GT ((2006) ) White matter changes in mild cognitive impairment and AD: A diffusion tensor imaging study. Neurobiol Aging 27: , 663–672. |
[194] | Liu Y , Spulber G , Lehtimaki KK , Kononen M , Hallikainen I , Grohn H , Kivipelto M , Hallikainen M , Vanninen R , Soininen H ((2011) ) Diffusion tensor imaging and tract-based spatial statistics in Alzheimer’s disease and mild cognitive impairment. Neurobiol Aging 32: , 1558–1571. |
[195] | Fjell AM , Amlien IK , Westlye LT , Walhovd KB ((2009) ) Mini-mental state examination is sensitive to brain atrophy in Alzheimer’s disease. Dement Geriatr Cogn Disord 28: , 252–258. |
[196] | Heo JH , Lee ST , Kon C , Park HJ , Shim JY , Kim M ((2009) ) White matter hyperintensities and cognitive dysfunction in Alzheimer disease. J Geriatr Psychiatry Neurol 22: , 207–212. |
[197] | Chua TC , Wen W , Chen X , Kochan N , Slavin MJ , Trollor JN , Brodaty H , Sachdev PS ((2009) ) Diffusion tensor imaging of the posterior cingulate is a useful biomarker of mild cognitive impairment. Am J Geriatr Psychiatry 17: , 602–613. |
[198] | Takahashi S , Yonezawa H , Takahashi J , Kudo M , Inoue T , Tohgi H ((2002) ) Selective reduction of diffusion anisotropy in white matter of Alzheimer disease brains measured by 3.0 Tesla magnetic resonance imaging. Neurosci Lett 332: , 45–48. |
[199] | Zhang Y , Schuff N , Jahng GH , Bayne W , Mori S , Schad L , Mueller S , Du AT , Kramer JH , Yaffe K , Chui H , Jagust WJ , Miller BL , Weiner MW ((2007) ) Diffusion tensor imaging of cingulum fibers in mild cognitive impairment and Alzheimer disease. Neurology 68: , 13–19. |
[200] | Zhuang L , Wen W , Zhu W , Trollor J , Kochan N , Crawford J , Reppermund S , Brodaty H , Sachdev P ((2010) ) White matter integrity in mild cognitive impairment: A tract-based spatial statistics study. Neuroimage 53: , 16–25. |
[201] | Douaud G , Jbabdi S , Behrens TE , Menke RA , Gass A , Monsch AU , Rao A , Whitcher B , Kindlmann G , Matthews PM , Smith S ((2011) ) DTI measures in crossing-fibre areas: Increased diffusion anisotropy reveals early white matter alteration in MCI and mild Alzheimer’s disease. Neuroimage 55: , 880–890. |
[202] | Ennis DB , Kindlmann G ((2006) ) Orthogonal tensor invariants and the analysis of diffusion tensor magnetic resonance images. Magn Reson Med 55: , 136–146. |
[203] | Stricker NH , Schweinsburg BC , Delano-Wood L , Wierenga CE , Bangen KJ , Haaland KY , Frank LR , Salmon DP , Bondi MW ((2009) ) Decreased white matter integrity in late-myelinating fiber pathways in Alzheimer’s disease supports retrogenesis. Neuroimage 45: , 10–16. |
[204] | Teipel SJ , Stahl R , Dietrich O , Schoenberg SO , Perneczky R , Bokde AL , Reiser MF , Moller HJ , Hampel H ((2007) ) Multivariate network analysis of fiber tract integrity in Alzheimer’s disease. Neuroimage 34: , 985–995. |
[205] | Selnes P , Aarsland D , Bjornerud A , Gjerstad L , Wallin A , Hessen E , Reinvang I , Grambaite R , Auning E , Kjaervik VK , Due-Tonnessen P , Stenset V , Fladby T ((2013) ) Diffusion tensor imaging surpasses cerebrospinal fluid as predictor of cognitive decline and medial temporal lobe atrophy in subjective cognitive impairment and mild cognitive impairment. J Alzheimers Dis 33: , 723–736. |
[206] | Sexton CE , Kalu UG , Filippini N , Mackay CE , Ebmeier KP ((2011) ) A meta-analysis of diffusion tensor imaging in mild cognitive impairment and Alzheimer’s disease. Neurobiol Aging 32: 2322, e2325–2318. |
[207] | Teipel SJ , Reuter S , Stieltjes B , Acosta-Cabronero J , Ernemann U , Fellgiebel A , Filippi M , Frisoni G , Hentschel F , Jessen F , Kloppel S , Meindl T , Pouwels PJ , Hauenstein KH , Hampel H ((2011) ) Multicenter stability of diffusion tensor imaging measures: A European clinical and physical phantom study. Psychiatry Res 194: , 363–371. |
[208] | Mielke MM , Okonkwo OC , Oishi K , Mori S , Tighe S , Miller MI , Ceritoglu C , Brown T , Albert M , Lyketsos CG ((2012) ) Fornix integrity and hippocampal volume predict memory decline and progression to Alzheimer’s disease. Alzheimers Dement 8: , 105–113. |
[209] | Douaud G , Menke RA , Gass A , Monsch AU , Rao A , Whitcher B , Zamboni G , Matthews PM , Sollberger M , Smith S ((2013) ) Brain microstructure reveals early abnormalities more than two years prior to clinical progression from mild cognitive impairment to Alzheimer’s disease. J Neurosci 33: , 2147–2155. |
[210] | Andersson JL , Skare S , Ashburner J ((2003) ) How to correct susceptibility distortions in spin-echo echo-planar images: Application to diffusion tensor imaging. Neuroimage 20: , 870–888. |
[211] | Eichner C , Setsompop K , Koopmans PJ , Lutzkendorf R , Norris DG , Turner R , Wald LL , Heidemann RM ((2014) ) Slice accelerated diffusion-weighted imaging at ultra-high field strength. Magn Reson Med 71: , 1518–1525. |
[212] | Back SA , Kroenke CD , Sherman LS , Lawrence G , Gong X , Taber EN , Sonnen JA , Larson EB , Montine TJ ((2011) ) White matter lesions defined by diffusion tensor imaging in older adults. Ann Neurol 70: , 465–476. |
[213] | Cosottini M , Frosini D , Pesaresi I , Costagli M , Biagi L , Ceravolo R , Bonuccelli U , Tosetti M ((2014) ) MR imaging of the substantia nigra at 7 T enables diagnosis of Parkinson disease. Radiology 271: , 831–838. |
[214] | Verstraete E , Polders DL , Mandl RC , Van Den Heuvel MP , Veldink JH , Luijten P , Van Den Berg LH , Hoogduin J ((2014) ) Multimodal tract-based analysis in ALS patients at 7T: A specific white matter profile? Amyotroph Lateral Scler Frontotemporal Degener 15: , 84–92. |
[215] | Alexander AL , Hasan KM , Lazar M , Tsuruda JS , Parker DL ((2001) ) Analysis of partial volume effects in diffusion-tensor MRI. Magn Reson Med 45: , 770–780. |
[216] | Wedeen VJ , Wang RP , Schmahmann JD , Benner T , Tseng WY , Dai G , Pandya DN , Hagmann P , D’Arceuil H , de Crespigny AJ ((2008) ) Diffusion spectrum magnetic resonance imaging (DSI) tractography of crossing fibers. Neuroimage 41: , 1267–1277. |
[217] | Fieremans E , Jensen JH , Helpern JA ((2011) ) White matter characterization with diffusional kurtosis imaging. Neuroimage 58: , 177–188. |
[218] | Hui ES , Cheung MM , Qi L , Wu EX ((2008) ) Advanced MR diffusion characterization of neural tissue using directional diffusion kurtosis analysis. Conf Proc IEEE Eng Med Biol Soc 2008: , 3941–3944. |
[219] | Jensen JH , Helpern JA ((2010) ) Progress in diffusion-weighted imaging: Concepts, techniques and applications to the central nervous system. NMR Biomed 23: , 659–660. |
[220] | Lanzafame S , Giannelli M , Garaci F , Floris R , Duggento A , Guerrisi M , Toschi N ((2016) ) Differences in Gaussian diffusion tensor imaging and non-Gaussian diffusion kurtosis imaging model-based estimates of diffusion tensor invariants in the human brain. Med Phys 43: , 2464. |
[221] | Giannelli M , Toschi N ((2016) ) On the use of trace-weighted images in body diffusional kurtosis imaging. Magn Reson Imaging 34: , 502–507. |
[222] | Alexander DC ((2005) ) Multiple-fiber reconstruction algorithms for diffusion MRI. Ann N Y Acad Sci 1064: , 113–133. |
[223] | Assaf Y , Blumenfeld-Katzir T , Yovel Y , Basser PJ ((2008) ) AxCaliber: A method for measuring axon diameter distribution from diffusion MRI. Magn Reson Med 59: , 1347–1354. |
[224] | Assaf Y , Basser PJ ((2005) ) Composite hindered and restricted model of diffusion (CHARMED) MR imaging of the human brain. Neuroimage 27: , 48–58. |
[225] | Santis SD , Granberg T , Ouellette R , Treaba CA , Fan Q , Herranz E , Mainero C , Toschi N ((2017) ) Early axonal damage in normal appearing white matter in multiple sclerosis: Novel insights from multi-shell diffusion MRI. 2017 39th Annual International Conference of the IEEE Engineering in Medicine and Biology Society (EMBC), pp. 3024–3027. |
[226] | Alexander DC , Hubbard PL , Hall MG , Moore EA , Ptito M , Parker GJ , Dyrby TB ((2010) ) Orientationally invariant indices of axon diameter and density from diffusion MRI. Neuroimage 52: , 1374–1389. |
[227] | De Santis S , Gabrielli A , Bozzali M , Maraviglia B , Macaluso E , Capuani S ((2011) ) Anisotropic anomalous diffusion assessed in the human brain by scalar invariant indices. Magn Reson Med 65: , 1043–1052. |
[228] | De Santis S , Gabrielli A , Palombo M , Maraviglia B , Capuani S ((2011) ) Non-Gaussian diffusion imaging: A brief practical review. Magn Reson Imaging 29: , 1410–1416. |
[229] | Mintun MA , Larossa GN , Sheline YI , Dence CS , Lee SY , Mach RH , Klunk WE , Mathis CA , DeKosky ST , Morris JC ((2006) ) [11C]PIB in a nondemented population: Potential antecedent marker of Alzheimer disease. Neurology 67: , 446–452. |
[230] | Iraji A , Davoodi-Bojd E , Soltanian-Zadeh H , Hossein-Zadeh GA , Jiang Q ((2011) ) Diffusion kurtosis imaging discriminates patients with white matter lesions from healthy subjects. Conf Proc IEEE Eng Med Biol Soc 2011: , 2796–2799. |
[231] | Falangola MF , Jensen JH , Babb JS , Hu C , Castellanos FX , Di Martino A , Ferris SH , Helpern JA ((2008) ) Age-related non-Gaussian diffusion patterns in the prefrontal brain. J Magn Reson Imaging 28: , 1345–1350. |
[232] | Wang JJ , Lin WY , Lu CS , Weng YH , Ng SH , Wang CH , Liu HL , Hsieh RH , Wan YL , Wai YY ((2011) ) Parkinson disease: Diagnostic utility of diffusion kurtosis imaging. Radiology 261: , 210–217. |
[233] | Thompson PM , Ge T , Glahn DC , Jahanshad N , Nichols TE ((2013) ) Genetics of the connectome. Neuroimage 80: , 475–488. |
[234] | Baggio HC , Segura B , Junque C , de Reus MA , Sala-Llonch R , Van den Heuvel MP ((2015) ) Rich club organization and cognitive performance in healthy older participants. J Cogn Neurosci 27: , 1801–1810. |
[235] | Nakagawa TT , Jirsa VK , Spiegler A , McIntosh AR , Deco G ((2013) ) Bottom up modeling of the connectome: Linking structure and function in the resting brain and their changes in aging. Neuroimage 80: , 318–329. |
[236] | Fornito A , Zalesky A , Breakspear M ((2015) ) The connectomics of brain disorders. Nat Rev Neurosci 16: , 159–172. |
[237] | Fischer FU , Wolf D , Scheurich A , Fellgiebel A , Alzheimer’s Disease Neuroimaging Initiative ((2015) ) Altered whole-brain white matter networks in preclinical Alzheimer’s disease. Neuroimage Clin 8: , 660–666. |
[238] | Daianu M , Jahanshad N , Nir TM , Toga AW , Jack CR Jr , Weiner MW , Thompson PM; Alzheimer’s Disease Neuroimaging Initiative ((2013) ) Breakdown of brain connectivity between normal aging and Alzheimer’s disease: A structural k-core network analysis. Brain Connect 3: , 407–422. |
[239] | Logothetis NK , Pauls J , Augath M , Trinath T , Oeltermann A ((2001) ) Neurophysiological investigation of the basis of the fMRI signal. Nature 412: , 150–157. |
[240] | Machulda MM , Senjem ML , Weigand SD , Smith GE , Ivnik RJ , Boeve BF , Knopman DS , Petersen RC , Jack CR ((2009) ) Functional magnetic resonance imaging changes in amnestic and nonamnestic mild cognitive impairment during encoding and recognition tasks. J Int Neuropsychol Soc 15: , 372. |
[241] | Machulda MM , Ward HA , Borowski B , Gunter JL , Cha RH , O’Brien PC , Petersen RC , Boeve BF , Knopman D , Tang-Wai DF , Ivnik RJ , Smith GE , Tangalos EG , Jack CR ((2003) ) Comparison of memory fMRI response among normal, MCI, and Alzheimer’s patients. Neurology 61: , 500–506. |
[242] | Sperling RA ((2003) ) fMRI studies of associative encoding in young and elderly controls and mild Alzheimer’s disease. J Neurol Neurosurg Psychiatry 74: , 44–50. |
[243] | Chhatwal JP , Sperling RA ((2012) ) Functional MRI of mnemonic networks across the spectrum of normal aging, mild cognitive impairment, and Alzheimer’s disease. J Alzheimers Dis 31: , S155–S167. |
[244] | Dickerson BC , Miller SL , Greve DN , Dale AM , Albert MS , Schacter DL , Sperling RA ((2007) ) Prefrontal-hippocampal-fusiform activity during encoding predicts intraindividual differences in free recall ability: An event-related functional-anatomic MRI study. Hippocampus 17: , 1060–1070. |
[245] | Papp KV , Amariglio RE , Mormino EC , Hedden T , Dekhytar M , Johnson KA , Sperling RA , Rentz DM ((2015) ) Free and cued memory in relation to biomarker-defined abnormalities in clinically normal older adults and those at risk for Alzheimer’s disease. Neuropsychologia 73: , 169–175. |
[246] | Sperling RA , Dickerson BC , Pihlajamaki M , Vannini P , LaViolette PS , Vitolo OV , Hedden T , Becker JA , Rentz DM , Selkoe DJ , Johnson KA ((2010) ) Functional Alterations in memory networks in early Alzheimer’s disease. Neuromolecular Med 12: , 27–43. |
[247] | Pihlajamäki M , O’Keefe K , Bertram L , Tanzi RE , Dickerson BC , Blacker D , Albert MS , Sperling RA ((2010) ) Evidence of altered posteromedial cortical fMRI activity in subjects at risk for Alzheimer disease. Alzheimer Dis Assoc Disord 24: , 28–36. |
[248] | Rombouts SARB , Goekoop R , Stam CJ , Barkhof F , Scheltens P ((2005) ) Delayed rather than decreased BOLD response as a marker for early Alzheimer’s disease. Neuroimage 26: , 1078–1085. |
[249] | Celone KA , Calhoun VD , Dickerson BC , Atri A , Chua EF , Miller SL , DePeau K , Rentz DM , Selkoe DJ , Blacker D , Albert MS , Sperling RA ((2006) ) Alterations in memory networks in mild cognitive impairment and Alzheimer’s disease: An independent component analysis. J Neurosci 26: , 10222–10231. |
[250] | Bokde ALW , Karmann M , Born C , Teipel SJ , Omerovic M , Ewers M , Frodl T , Meisenzahl E , Reiser M , Möller H-J , Hampel H ((2010) ) Altered brain activation during a verbal working memory task in subjects with amnestic mild cognitive impairment. J Alzheimers Dis 21: , 103–118. |
[251] | Veltman DJ , Rombouts SARB , Dolan RJ ((2003) ) Maintenance versus manipulation in verbal working memory revisited: An fMRI study. Neuroimage 18: , 247–256. |
[252] | Kochan NA , Breakspear M , Valenzuela M , Slavin MJ , Brodaty H , Wen W , Trollor JN , Turner A , Crawford JD , Sachdev PS ((2011) ) Cortical responses to a graded working memory challenge predict functional decline in mild cognitive impairment. Biol Psychiatry 70: , 123–130. |
[253] | Teipel S , Ehlers I , Erbe A , Holzmann C , Lau E , Hauenstein K , Berger C ((2014) ) Structural connectivity changes underlying altered working memory networks in mild cognitive impairment: A three-way image fusion analysis. J Neuroimaging 25: , 634–642. |
[254] | Veltman DJ , De Ruiter MB , Rombouts SARB , Lazeron RHC , Barkhof F , Van Dyck R , Dolan RJ , Hans Phaf R ((2005) ) Neurophysiological correlates of increased verbal working memory in high-dissociative participants: A functional MRI study. Psychol Med 35: , 175–185. |
[255] | Kochan NA , Breakspear M , Slavin MJ , Valenzuela M , McCraw S , Brodaty H , Sachdev PS ((2010) ) Functional alterations in brain activation and deactivation in mild cognitive impairment in response to a graded working memory challenge. Dement Geriatr Cogn Disord 30: , 553–568. |
[256] | Bokde ALW , Dong W , Born C , Leinsinger G , Meindl T , Teipel SJ , Reiser M , Hampel H ((2005) ) Task difficulty in a simultaneous face matching task modulates activity in face fusiform area. Cogn Brain Res 25: , 701–710. |
[257] | Bokde ALW , Teipel SJ , Drzezga A , Thissen J , Bartenstein P , Dong W , Leinsinger G , Born C , Schwaiger M , Moeller HJ , Hampel H ((2005) ) Association between cognitive performance and cortical glucose metabolism in patients with mild Alzheimer’s disease. Dement Geriatr Cogn Disord 20: , 352–357. |
[258] | Barch DM , Braver TS , Nystrom LE , Forman SD , Noll DC , Cohen JD ((1997) ) Dissociating working memory from task difficulty in human prefrontal cortex. Neuropsychologia 35: , 1373–1380. |
[259] | Gould RL , Brown RG , Owen AM , ffytche DH , Howard RJ ((2003) ) FMRI BOLD response to increasing task difficulty during successful paired associates learning. Neuroimage 20: , 1006–1019. |
[260] | Grady CL , Horwitz B , Pietrini P , Mentis MJ , Ungerleider LG , Rapoport SI , Haxby JV ((1996) ) Effect of task difficulty on cerebral blood flow during perceptual matching of faces. Hum Brain Mapp 4: , 227–239. |
[261] | Gur RC , Gur RE , Skolnick BE , Resnick SM , Silver FL , Chawluk J , Muenz L , Obrist WD , Reivich M ((1988) ) Effects of task difficulty on regional cerebral blood flow: Relationships with anxiety and performance. Psychophysiology 25: , 392–399. |
[262] | Bokde ALW , Lopez-Bayo P , Born C , Ewers M , Meindl T , Teipel SJ , Faltraco F , Reiser MF , Möller H-J , Hampel H ((2010) ) Alzheimer disease: Functional abnormalities in the dorsal visual pathway. Radiology 254: , 219–226. |
[263] | Bokde ALW , Lopez-Bayo P , Born C , Dong W , Meindl T , Leinsinger G , Teipel SJ , Faltraco F , Reiser M , Möller H-J , Hampel H ((2008) ) Functional abnormalities of the visual processing system in subjects with mild cognitive impairment: An fMRI study. Psychiatry Res 163: , 248–259. |
[264] | Hao J , Li K , Li K , Zhang D , Wang W , Yang Y , Yan B , Shan B , Zhou X ((2005) ) Visual attention deficits in Alzheimer’s disease: An fMRI study. Neurosci Lett 385: , 18–23. |
[265] | Keightley ML , Winocur G , Graham SJ , Mayberg HS , Hevenor SJ , Grady CL ((2003) ) An fMRI study investigating cognitive modulation of brain regions associated with emotional processing of visual stimuli. Neuropsychologia 41: , 585–596. |
[266] | Matsumoto E , Ohigashi Y , Fujimori M , Mori E ((2000) ) The processing of global and local visual information in Alzheimer’s disease. Behav Neurol 12: , 119–125. |
[267] | Mentis MJ , Alexander GE , Krasuski J , Pietrini P , Furey ML , Schapiro MB , Rapoport SI ((1998) ) Increasing required neural response to expose abnormal brain function in mild versus moderate or severe Alzheimer’s disease: PET study using parametric visual stimulation. Am J Psychiatry 155: , 785–794. |
[268] | Mentis MJ , Horwitz B , Grady CL , Alexander GE , VanMeter JW , Maisog JM , Pietrini P , Schapiro MB , Rapoport SI ((1996) ) Visual cortical dysfunction in Alzheimer’s disease evaluated with a temporally graded “stress test” during PET. Am J Psychiatry 153: , 32–40. |
[269] | Pietrini P , Alexander GE , Furey ML , Dani A , Mentis MJ , Horwitz B , Guazzelli M , Shapiro MB , Rapoport SI ((2000) ) Cerebral metabolic response to passive audiovisual stimulation in patients with Alzheimer’s disease and healthy volunteers assessed by PET. J Nucl Med 41: , 575–583. |
[270] | McIntosh AR , Grady CL , Ungerleider LG , Haxby JV , Rapoport SI , Horwitz B ((1994) ) Network analysis of cortical visual pathways mapped with PET. J Neurosci 14: , 655–666. |
[271] | Pietrini P , Furey ML , Graff-Radford N , Freo U , Alexander GE , Grady CL , Dani A , Mentis MJ , Schapiro MB ((1996) ) Preferential metabolic involvement of visual cortical areas in a subtype of Alzheimer’s disease: Clinical implications. Am J Psychiatry 153: , 1261–1268. |
[272] | Prvulovic D , Hubl D , Sack AT , Melillo L , Maurer K , Frölich L , Lanfermann H , Zanella FE , Goebel R , Linden DEJ , Dierks T ((2002) ) Functional imaging of visuospatial processing in Alzheimer’s disease. Neuroimage 17: , 1403–1414. |
[273] | Bokde ALW , Cavedo E , Lopez-Bayo P , Lista S , Meindl T , Born C , Galluzzi S , Faltraco F , Dubois B , Teipel SJ , Reiser M , Möller H-J , Hampel H ((2016) ) Effects of rivastigmine on visual attention in subjects with amnestic mild cognitive impairment: A serial functional MRI activation pilot-study. Psychiatry Res 249: , 84–90. |
[274] | Hämäläinen A , Pihlajamäki M , Tanila H , Hänninen T , Niskanen E , Tervo S , Karjalainen PA , Vanninen RL , Soininen H ((2007) ) Increased fMRI responses during encoding in mild cognitive impairment. Neurobiol Aging 28: , 1889–1903. |
[275] | Bentley P , Driver J , Dolan RJ ((2008) ) Cholinesterase inhibition modulates visual and attentional brain responses in Alzheimer’s disease and health. Brain 131: , 409–424. |
[276] | Bentley P , Driver J , Dolan RJ ((2009) ) Modulation of fusiform cortex activity by cholinesterase inhibition predicts effects on subsequent memory. Brain 132: , 2356–2371. |
[277] | Bentley P , Husain M , Dolan RJ ((2004) ) Effects of cholinergic enhancement on visual stimulation, spatial attention, and spatial working memory. Neuron 41: , 969–982. |
[278] | Bokde ALW , Karmann M , Teipel SJ , Born C , Lieb M , Reiser MF , Möller H-J , Hampel H ((2009) ) Decreased activation along the dorsal visual pathway after a 3-month treatment with galantamine in mild Alzheimer disease. J Clin Psychopharmacol 29: , 147–156. |
[279] | Balsters JH , O’Connell RG , Martin MP , Galli A , Cassidy SM , Kilcullen SM , Delmonte S , Brennan S , Meaney JF , Fagan AJ , Bokde ALW , Upton N , Lai R , Laruelle M , Lawlor B , Robertson IH ((2011) ) Donepezil impairs memory in healthy older subjects: Behavioural, EEG and simultaneous EEG/fMRI biomarkers. PLoS One 6: , e24126. |
[280] | Bentley P , Vuilleumier P , Thiel CM , Driver J , Dolan RJ ((2003) ) Cholinergic enhancement modulates neural correlates of selective attention and emotional processing. Neuroimage 20: , 58–70. |
[281] | Handjaras G , Ricciardi E , Szczepanik J , Pietrini P , Furey ML ((2013) ) Cholinergic enhancement differentially modulates neural response to encoding during face identity and face location working memory tasks. Exp Biol Med 238: , 999–1008. |
[282] | Ricciardi E , Handjaras G , Bernardi G , Pietrini P , Furey ML ((2013) ) Cholinergic enhancement reduces functional connectivity and BOLD variability in visual extrastriate cortex during selective attention. Neuropharmacology 64: , 305–313. |
[283] | Pizoli CE , Shah MN , Snyder AZ , Shimony JS , Limbrick DD , Raichle ME , Schlaggar BL , Smyth MD ((2011) ) Resting-state activity in development and maintenance of normal brain function. Proc Natl Acad Sci U S A 108: , 11638–11643. |
[284] | Chen S , Ross TJ , Zhan W , Myers CS , Chuang K-S , Heishman SJ , Stein EA , Yang Y ((2008) ) Group independent component analysis reveals consistent resting-state networks across multiple sessions. Brain Res 1239: , 141–151. |
[285] | Greicius MD , Krasnow B , Reiss AL , Menon V ((2002) ) Functional connectivity in the resting brain: A network analysis of the default mode hyothesis. Proc Natl Acad Sci U S A 100: , 253–258. |
[286] | Greicius MD , Supekar K , Menon V , Dougherty RF ((2008) ) Resting-state functional connectivity reflects structural connectivity in the default mode network. Cereb Cortex 19: , 72–78. |
[287] | Shah LM , Cramer JA , Ferguson MA , Birn RM , Anderson JS ((2016) ) Reliability and reproducibility of individual differences in functional connectivity acquired during task and resting state. Brain Behav 6: , e00456. |
[288] | Hutchison RM , Womelsdorf T , Allen EA , Bandettini PA , Calhoun VD , Corbetta M , Della Penna S , Duyn JH , Glover GH , Gonzalez-Castillo J ((2013) ) Dynamic functional connectivity: Promise, issues, and interpretations. Neuroimage 80: , 360–378. |
[289] | Power JD , Cohen AL , Nelson SM , Wig GS , Barnes KA , Church JA , Vogel AC , Laumann TO , Miezin FM , Schlaggar BL , Petersen SE ((2011) ) Functional network organization of the human brain. Neuron 72: , 665–678. |
[290] | Wen W , Zhu W , He Y , Kochan NA , Reppermund S , Slavin MJ , Brodaty H , Crawford J , Xia A , Sachdev P ((2011) ) Discrete neuroanatomical networks are associated with specific cognitive abilities in old age. J Neurosci 31: , 1204–1212. |
[291] | Cole MW , Bassett DS , Power JD , Braver TS , Petersen SE ((2014) ) Intrinsic and task-evoked network architectures of the human brain. Neuron 83: , 238–251. |
[292] | Connolly J , McNulty JP , Boran L , Roche RAP , Delany D , Bokde ALW ((2016) ) Identification of resting state networks involved in executive function. Brain Connect 6: , 365–374. |
[293] | Sala-Llonch R , Peña-Gómez C , Arenaza-Urquijo EM , Vidal-Piñeiro D , Bargalló N , Junqué C , Bartrés-Faz D ((2012) ) Brain connectivity during resting state and subsequent working memory task predicts behavioural performance. Cortex 48: , 1187–1196. |
[294] | Wang G-Z , Belgard TG , Mao D , Chen L , Berto S , Preuss Todd M , Lu H , Geschwind Daniel H , Konopka G ((2015) ) Correspondence between resting-state activity and brain gene expression. Neuron 88: , 659–666. |
[295] | Richiardi J , Altmann A , Milazzo AC , Chang C , Chakravarty MM , Banaschewski T , Barker GJ , Bokde ALW , Bromberg U , Buchel C , Conrod P , Fauth-Buhler M , Flor H , Frouin V , Gallinat J , Garavan H , Gowland P , Heinz A , Lemaitre H , Mann KF , Martinot JL , Nees F , Paus T , Pausova Z , Rietschel M , Robbins TW , Smolka MN , Spanagel R , Strohle A , Schumann G , Hawrylycz M , Poline JB , Greicius MD , IMAGEN consortium ((2015) ) Correlated gene expression supports synchronous activity in brain networks. Science 348: , 1241–1244. |
[296] | Craddock RC , Holtzheimer PE , Hu XP , Mayberg HS ((2009) ) Disease state prediction from resting state functional connectivity. Magn Reson Med 62: , 1619–1628. |
[297] | Greicius MD , Srivastava G , Reiss AL , Menon V ((2004) ) Default-mode network activity distinguishes Alzheimer’s disease from healthy aging: Evidence from functional MRI. Proc Natl Acad Sci U S A 101: , 4637–4642. |
[298] | Seeley WW , Crawford RK , Zhou J , Miller BL , Greicius MD ((2009) ) Neurodegenerative diseases target large-scale human brain networks. Neuron 62: , 42–52. |
[299] | Seeley WW , Menon V , Schatzberg AF , Keller J , Glover GH , Kenna H , Reiss AL , Greicius MD ((2007) ) Dissociable intrinsic connectivity networks for salience processing and executive control. J Neurosci 27: , 2349–2356. |
[300] | Zhou J , Greicius MD , Gennatas ED , Growdon ME , Jang JY , Rabinovici GD , Kramer JH , Weiner M , Miller BL , Seeley WW ((2010) ) Divergent network connectivity changes in behavioural variant frontotemporal dementia and Alzheimer’s disease. Brain 133: , 1352–1367. |
[301] | Dopper EG , Rombouts SA , Jiskoot LC , den Heijer T , de Graaf JR , de Koning I , Hammerschlag AR , Seelaar H , Seeley WW , Veer IM , van Buchem MA , Rizzu P , van Swieten JC ((2014) ) Structural and functional brain connectivity in presymptomatic familial frontotemporal dementia. Neurology 83: , e19–26. |
[302] | Thomas JB , Brier MR , Bateman RJ , Snyder AZ , Benzinger TL , Xiong C , Raichle M , Holtzman DM , Sperling RA , Mayeux R , Ghetti B , Ringman JM , Salloway S , McDade E , Rossor MN , Ourselin S , Schofield PR , Masters CL , Martins RN , Weiner MW , Thompson PM , Fox NC , Koeppe RA , Jack CR , Mathis CA , Oliver A , Blazey TM , Moulder K , Buckles V , Hornbeck R , Chhatwal J , Schultz AP , Goate AM , Fagan AM , Cairns NJ , Marcus DS , Morris JC , Ances BM ((2014) ) Functional connectivity in autosomal dominant and late-onset Alzheimer disease. JAMA Neurol 71: , 1111. |
[303] | Ward AM , Mormino EC , Huijbers W , Schultz AP , Hedden T , Sperling RA ((2015) ) Relationships between default-mode network connectivity, medial temporal lobe structure, and age-related memory deficits. Neurobiol Aging 36: , 265–272. |
[304] | Cole MW , Ito T , Bassett DS , Schultz DH ((2016) ) Activity flow over resting-state networks shapes cognitive task activations. Nat Neurosci 19: , 1718–1726. |
[305] | Mennes M , Kelly C , Zuo XN , Di Martino A , Biswal BB , Castellanos FX , Milham MP ((2010) ) Inter-individual differences in resting-state functional connectivity predict task-induced BOLD activity. Neuroimage 50: , 1690–1701. |
[306] | Mennes M , Zuo X-N , Kelly C , Di Martino A , Zang Y-F , Biswal B , Castellanos FX , Milham MP ((2011) ) Linking inter-individual differences in neural activation and behavior to intrinsic brain dynamics. Neuroimage 54: , 2950–2959. |
[307] | Lorenzi M , Beltramello A , Mercuri NB , Canu E , Zoccatelli G , Pizzini FB , Alessandrini F , Cotelli M , Rosini S , Costardi D , Caltagirone C , Frisoni GB ((2011) ) Effect of memantine on resting state default mode network activity in Alzheimer’s disease. Drugs Aging 28: , 205–217. |
[308] | Laumann TO , Gordon EM , Adeyemo B , Snyder AZ , Joo SJ , Chen M-Y , Gilmore AW , McDermott KB , Nelson SM , Dosenbach NU ((2015) ) Functional system and areal organization of a highly sampled individual human brain. Neuron 87: , 657–670. |
[309] | Dubois J , Adolphs R ((2016) ) Building a science of individual differences from fMRI. Trends Cogn Sci 20: , 425–443. |
[310] | Babiloni C , Lizio R , Marzano N , Capotosto P , Soricelli A , Triggiani AI , Cordone S , Gesualdo L , Del Percio C ((2016) ) Brain neural synchronization and functional coupling in Alzheimer’s disease as revealed by resting state EEG rhythms. Int J Psychophysiol 103: , 88–102. |
[311] | Stern Y ((2017) ) An approach to studying the neural correlates of reserve. Brain Imaging Behav 11: , 410–416. |
[312] | Babiloni C , Del Percio C , Boccardi M , Lizio R , Lopez S , Carducci F , Marzano N , Soricelli A , Ferri R , Triggiani AI , Prestia A , Salinari S , Rasser PE , Basar E , Famà F , Nobili F , Yener G , Emek-Savaş DD , Gesualdo L , Mundi C , Thompson PM , Rossini PM , Frisoni GB ((2015) ) Occipital sources of resting-state alpha rhythms are related to local gray matter density in subjects with amnesic mild cognitive impairment and Alzheimer’s disease. Neurobiol Aging 36: , 556–570. |
[313] | Dierks T , Ihl R , Frölich L , Maurer K ((1993) ) Dementia of the alzheimer type: Effects on the spontaneous EEG described by dipole sources. Psychiatry Res 50: , 151–162. |
[314] | Dierks T , Jelic V , Pascual-Marqui RD , Wahlund L-O , Julin P , Linden DEJ , Maurer K , Winblad B , Nordberg A ((2000) ) Spatial pattern of cerebral glucose metabolism (PET) correlates with localization of intracerebral EEG-generators in Alzheimer’s disease. Clin Neurophysiol 111: , 1817–1824. |
[315] | Huang C , Wahlund LO , Dierks T , Julin P , Winblad B , Jelic V ((2000) ) Discrimination of Alzheimer’s disease and mild cognitive impairment by equivalent EEG sources: A cross-sectional and longitudinal study. Clin Neurophysiol 111: , 1961–1967. |
[316] | Jelic V , Johansson SE , Almkvist O , Shigeta M , Julin P , Nordberg A , Winblad B , Wahlund LO ((2000) ) Quantitative electroencephalography in mild cognitive impairment: Longitudinal changes and possible prediction of Alzheimer’s disease. Neurobiol Aging 21: , 533–540. |
[317] | Ponomareva NV , Selesneva ND , Jarikov GA ((2003) ) EEG Alterations in Subjects at high familial risk for Alzheimer’s disease. Neuropsychobiology 48: , 152–159. |
[318] | Jeong J ((2004) ) EEG dynamics in patients with Alzheimer’s disease. Clin Neurophysiol 115: , 1490–1505. |
[319] | Babiloni C , Binetti G , Cassetta E , Forno GD , Percio CD , Ferreri F , Ferri R , Frisoni G , Hirata K , Lanuzza B , Miniussi C , Moretti DV , Nobili F , Rodriguez G , Romani GL , Salinari S , Rossini PM ((2006) ) Sources of cortical rhythms change as a function of cognitive impairment in pathological aging: A multicenter study. Clin Neurophysiol 117: , 252–268. |
[320] | Reeves RR , Struve FA , Patrick G ((2002) ) The effects of donepezil on quantitative EEG in patients with Alzheimer’s disease. Clin Electroencephalogr 33: , 93–96. |
[321] | Brassen S , Adler G ((2003) ) Short-term effects of acetylcholinesterase inhibitor treatment on EEG and memory performance in Alzheimer patients: An open, controlled trial. Pharmacopsychiatry 36: , 304–308. |
[322] | Onofrj M , Thomas A , Iacono D , Luciano AL , Di Iorio A ((2003) ) The effects of a cholinesterase inhibitor are prominent in patients with fluctuating cognition: A part 3 study of the main mechanism of cholinesterase inhibitors in dementia. Clin Neuropharmacol 26: , 239–251. |
[323] | Kogan EA , Korczyn AD , Virchovsky RG , Klimovizky SS , Treves TA , Neufeld MY ((2001) ) EEG changes during long-term treatment with donepezil in Alzheimer’s disease patients. J Neural Transm 108: , 1167–1173. |
[324] | Rodriguez G , Vitali P , de Leo C , De Carli F , Girtler N , Nobili F ((2002) ) Quantitative EEG changes in Alzheimer patients during long-term donepezil therapy. Neuropsychobiology 46: , 49–56. |
[325] | Valladares-Neto DC , Buchsbaum MS , Evans WJ , Nguyen D , Nguyen P , Siegel BV , Stanley J , Starr A , Guich S , Rice D ((1995) ) EEG delta, positron emission tomography, and memory deficit in Alzheimer’s disease. Neuropsychobiology 31: , 173–181. |
[326] | Babiloni C , Del Percio C , Lizio R , Noce G , Cordone S , Lopez S , Soricelli A , Ferri R , Pascarelli MT , Nobili F , Arnaldi D , Aarsland D , Orzi F , Buttinelli C , Giubilei F , Onofrj M , Stocchi F , Stirpe P , Fuhr P , Gschwandtner U , Ransmayr G , Caravias G , Garn H , Sorpresi F , Pievani M , Frisoni GB , D’Antonio F , De Lena C , Güntekin B , Hanoğlu L , Başar E , Yener G , Emek-Savaş DD , Triggiani AI , Franciotti R , De Pandis MF , Bonanni L ((2017) ) Abnormalities of cortical neural synchronization mechanisms in patients with dementia due to Alzheimer’s and Lewy body diseases: An EEG study. Neurobiol Aging 55: , 143–158. |
[327] | Adler G , Brassen S , Jajcevic A ((2003) ) EEG coherence in Alzheimer’s dementia. J Neural Transm 110: , 1051–1058. |
[328] | Anghinah R , Kanda PAM , Jorge MS , Lima EEPD , Pascuzzi L , Melo ACDEP ((2000) ) Estudo da coerência do eletrencefalograma para a banda de frequência alfa em indivíduos adultos normais e com provável demência do tipo Alzheimer. Arq Neuropsiquiatr 58: , 272–275. |
[329] | Besthorn C , Förstl H , Geiger-Kabisch C , Sattel H , Gasser T , Schreiter-Gasser U ((1994) ) EEG coherence in Alzheimer disease. Electroencephalogr Clin Neurophysiol 90: , 242–245. |
[330] | Dunkin JJ , Leuchter AF , Newton TF , Cook IA ((1994) ) Reduced EEG coherence in dementia: State or trait marker? Biol Psychiatry 35: , 870–879. |
[331] | Fonseca LC , Tedrus GMAS , Prandi LR , Almeida AM , Furlanetto DS ((2011) ) Alzheimer’s disease: Relationship between cognitive aspects and power and coherence EEG measures. Arq Neuropsiquiatr 69: , 875–881. |
[332] | Fonseca LC , Tedrus GMAS , Carvas PN , Machado ECFA ((2013) ) Comparison of quantitative EEG between patients with Alzheimer’s disease and those with Parkinson’s disease dementia. Clin Neurophysiol 124: , 1970–1974. |
[333] | Jelic V , Julin P , Shigeta M , Nordberg A , Lannfelt L , Winblad B , Wahlund LO ((1997) ) Apolipoprotein E epsilon 4 allele decreases functional connectivity in Alzheimer’s disease as measured by EEG coherence. J Neurol Neurosurg Psychiatry 63: , 59–65. |
[334] | Knott V , Engeland C , Mohr E , Mahoney C , Ilivitsky V ((2000) ) Acute nicotine administration in Alzheimer’s disease: An exploratory EEG study. Neuropsychobiology 41: , 210–220. |
[335] | Leuchter AF ((1987) ) Electroencephalographic spectra and coherence in the diagnosis of Alzheimer’s-type and multi-infarct dementia. Arch Gen Psychiatry 44: , 993. |
[336] | Leuchter AF , Newton TF , Cook IA , Walter DO , Rosenberg-Thompson S , Lachenbruch PA ((1992) ) Changes in brain functional connectivity in Alzheimer-type and multi-infarct dementia. Brain 115: , 1543–1561. |
[337] | Locatelli T , Cursi M , Liberati D , Franceschi M , Comi G ((1998) ) EEG coherence in Alzheimer’s disease. Electroencephalogr Clin Neurophysiol 106: , 229–237. |
[338] | Pogarell O ((2005) ) EEG coherence reflects regional corpus callosum area in Alzheimer’s disease. J Neurol Neurosurg Psychiatry 76: , 109–111. |
[339] | Sloan EP , Fenton GW , Kennedy NSJ , MacLennan JM ((1994) ) Neurophysiology and SPECT cerebral blood flow patterns in dementia. Electroencephalogr Clin Neurophysiol 91: , 163–170. |
[340] | Leuchter AF , Dunkin JJ , Lufkin RB , Anzai Y , Cook IA , Newton TF ((1994) ) Effect of white matter disease on functional connections in the aging brain. J Neurol Neurosurg Psychiatry 57: , 1347–1354. |
[341] | Babiloni C , Frisoni GB , Vecchio F , Pievani M , Geroldi C , De Carli C , Ferri R , Vernieri F , Lizio R , Rossini PM ((2010) ) Global functional coupling of resting EEG rhythms is related to white-matter lesions along the cholinergic tracts in subjects with amnesic mild cognitive impairment. J Alzheimers Dis 19: , 859–871. |
[342] | Sankari Z , Adeli H , Adeli A ((2011) ) Intrahemispheric, interhemispheric, and distal EEG coherence in Alzheimer’s disease. Clin Neurophysiol 122: , 897–906. |
[343] | Babiloni C , Babiloni F , Carducci F , Cincotti F , Vecchio F , Cola B , Rossi S , Miniussi C , Rossini PM ((2004) ) Functional frontoparietal connectivity during short-term memory as revealed by high-resolution EEG coherence analysis. Behav Neurosci 118: , 687–697. |
[344] | Stam CJ , Nolte G , Daffertshofer A ((2007) ) Phase lag index: Assessment of functional connectivity from multi channel EEG and MEG with diminished bias from common sources. Hum Brain Mapp 28: , 1178–1193. |
[345] | de Haan W , van der Flier WM , Wang H , Van Mieghem PFA , Scheltens P , Stam CJ ((2012) ) Disruption of functional brain networks in Alzheimer’s disease: What can we learn from graph spectral analysis of resting-state magnetoencephalography? Brain Connectivity 2: , 45–55. |
[346] | Vecchio F , Miraglia F , Marra C , Quaranta D , Vita MG , Bramanti P , Rossini PM ((2014) ) Human brain networks in cognitive decline: A graph theoretical analysis of cortical connectivity from EEG data. J Alzheimers Dis 41: , 113–127. |
[347] | Morabito FC , Campolo M , Labate D , Morabito G , Bonanno L , Bramanti A , de Salvo S , Marra A , Bramanti P ((2015) ) A longitudinal EEG study of Alzheimer’s disease progression based on a complex network approach. Int J Neural Syst 25: , 1550005. |
[348] | Drago V , Babiloni C , Bartres-Faz D , Caroli A , Bosch B , Hensch T , Didic M , Klafki HW , Pievani M , Jovicich J , Venturi L , Spitzer P , Vecchio F , Schoenknecht P , Wiltfang J , Redolfi A , Forloni G , Blin O , Irving E , Davis C , Hardemark HG , Frisoni GB ((2011) ) Disease tracking markers for Alzheimer’s disease at the prodromal (MCI) stage. J Alzheimers Dis 26: , 159–199. |
[349] | Coben LA , Danziger W , Storandt M ((1985) ) A longitudinal EEG study of mild senile dementia of Alzheimer type: Changes at 1 year and at 2.5 years. Electroencephalogr Clin Neurophysiol 61: , 101–112. |
[350] | Soininen H , Partanen J , Laulumaa V , Helkala EL , Laakso M , Riekkinen PJ ((1989) ) Longitudinal EEG spectral analysis in early stage of Alzheimer’s disease. Electroencephalogr Clin Neurophysiol 72: , 290–297. |
[351] | Soininen H , Partanen J , Laulumaa V , Pääkkönen A , Helkala EL , Riekkinen PJ ((1991) ) Serial EEG in Alzheimer’s disease: 3 year follow-up and clinical outcome. Electroencephalogr Clin Neurophysiol 79: , 342–348. |
[352] | Babiloni C , Bartrés-Faz D , Didic M , Forloni G , Jovicich J , Nobili F , Payoux P , Schönknecht P , Wiltfang J , Blin O , Frisoni G ((2013) ) Cross-sectional clinical, neuropsychological, neuroimaging, neurophysiological and biochemical characterization of people with mild cognitive impairment in the WP5 PharmaCog/E-ADNI study. Alzheimers Dement 9: , P48. |
[353] | Babiloni C , Del Percio C , Lizio R , Marzano N , Infarinato F , Soricelli A , Salvatore E , Ferri R , Bonforte C , Tedeschi G , Montella P , Baglieri A , Rodriguez G , Famà F , Nobili F , Vernieri F , Ursini F , Mundi C , Frisoni GB , Rossini PM ((2014) ) Cortical sources of resting state electroencephalographic alpha rhythms deteriorate across time in subjects with amnesic mild cognitive impairment. Neurobiol Aging 35: , 130–142. |
[354] | Adler G , Brassen S ((2001) ) Short-term rivastigmine treatment reduces EEG slow-wave power in Alzheimer patients. Neuropsychobiology 43: , 273–276. |
[355] | Balkan S , Yaras N , Mihci E , Dora B , Agar A , Yargicoglu P ((2003) ) Effect of donepezil on EEG spectral analysis in Alzheimer’s disease. Acta Neurol Belg 103: , 164–169. |
[356] | Gianotti LRR , Künig G , Faber PL , Lehmann D , Pascual-Marqui RD , Kochi K , Schreiter-Gasser U ((2008) ) Rivastigmine effects on EEG spectra and three-dimensional LORETA functional imaging in Alzheimer’s disease. Psychopharmacology (Berl) 198: , 323–332. |
[357] | Adler G , Brassen S , Chwalek K , Dieter B , Teufel M ((2004) ) Prediction of treatment response to rivastigmine in Alzheimer’s dementia. J Neurol Neurosurg Psychiatry 75: , 292–294. |
[358] | Agnoli A , Martucci N , Manna V , Conti L , Fioravanti M ((1983) ) Effect of cholinergic and anticholinergic drugs on short-term memory in Alzheimer’s dementia. Clin Neuropharmacol 6: , 311–324. |
[359] | Alhainen K , Partanen J , Reinikainen K , Laulumaa V , Soininen H , Airaksinen M , Riekkinen P ((1991) ) Discrimination of tetrahydroaminoacridine responders by a single dose pharmaco-EEG in patients with Alzheimer’s disease. Neurosci Lett 127: , 113–116. |
[360] | Alhainen K , Riekkinen PJ ((1993) ) Discrimination of Alzheimer patients responding to cholinesterase inhibitor therapy. Acta Neurol Scand 88: , 16–21. |
[361] | Rodriguez G , Vitali P , Canfora M , Calvini P , Girtler N , De Leo C , Piccardo A , Nobili F ((2004) ) Quantitative EEG and perfusional single photon emission computed tomography correlation during long-term donepezil therapy in Alzheimer’s disease. Clin Neurophysiol 115: , 39–49. |
[362] | Kai T , Asai Y , Sakuma K , Koeda T , Nakashima K ((2005) ) Quantitative electroencephalogram analysis in dementia with Lewy bodies and Alzheimer’s disease. J Neurol Sci 237: , 89–95. |
[363] | Passero S , Rocchi R , Vatti G , Burgalassi L , Battistini N ((1995) ) Quantitative EEG mapping, regional cerebral blood flow, and neuropsychological function in Alzheimer’s disease. Dement Geriatr Cogn Disord 6: , 148–156. |
[364] | Valladares-Neto DC , Buchsbaum MS , Evans J , Nguyen D , Nguyen P , Siegel BV , Stanley J , Starr A , Guich S , Rice D ((2008) ) EEG delta, positron emission tomography, and memory deficit in Alzheimer’s disease. Neuropsychobiology 31: , 173–181. |
[365] | Niedermeyer E ((1997) ) Alpha rhythms as physiological and abnormal phenomena. Int J Psychophysiol 26: , 31–49. |
[366] | Rodriguez G , Nobili F , Copello F , Vitali P , Gianelli MV , Taddei G , Catsafados E , Mariani G ((1999) ) 99mTc-HMPAO regional cerebral blood flow and quantitative electroencephalography in Alzheimer’s disease: A correlative study. J Nucl Med 40: , 522–529. |
[367] | Fernandez A , Arrazola J , Maestu F , Amo C , Gil-Gregorio P , Wienbruch C , Ortiz T ((2003) ) Correlations of hippocampal atrophy and focal low-frequency magnetic activity in Alzheimer disease: Volumetric MR imaging-magnetoencephalographic study. AJNR Am J Neuroradiol 24: , 481–487. |
[368] | Graff-Radford NR , Besser LM , Crook JE , Kukull WA , Dickson DW ((2016) ) Neuropathologic differences by race from the National Alzheimer’s Coordinating Center. Alzheimers Dement 12: , 669–677. |
[369] | Sarro L , Senjem ML , Lundt ES , Przybelski SA , Lesnick TG , Graff-Radford J , Boeve BF , Lowe VJ , Ferman TJ , Knopman DS , Comi G , Filippi M , Petersen RC , Jack CR , Kantarci K ((2016) ) Amyloid-β deposition and regional grey matter atrophy rates in dementia with Lewy bodies. Brain 139: , 2740–2750. |
[370] | Hughes SW , Crunelli V ((2005) ) Thalamic mechanisms of EEG alpha rhythms and their pathological implications. The Neuroscientist 11: , 357–372. |
[371] | Lorincz ML , Crunelli V , Hughes SW ((2008) ) Cellular dynamics of cholinergically induced (8 13Hz) rhythms in sensory thalamic nuclei in vitro. J Neurosci 28: , 660–671. |
[372] | Lőrincz ML , Kékesi KA , Juhász G , Crunelli V , Hughes SW ((2009) ) Temporal framing of thalamic relay-mode firing by phasic inhibition during the alpha rhythm. Neuron 63: , 683–696. |
[373] | Andersson M , Hansson O , Minthon L , Rosén I , Londos E ((2008) ) Electroencephalogram variability in dementia with Lewy bodies, Alzheimer’s disease and controls. Dement Geriatr Cogn Disord 26: , 284–290. |
[374] | Engedal K , Snaedal J , Hoegh P , Jelic V , Bo Andersen B , Naik M , Wahlund L-O , Oeksengaard A-R ((2015) ) Quantitative EEG applying the statistical recognition pattern method: A useful tool in dementia diagnostic workup. Dement Geriatr Cogn Disord 40: , 1–12. |
[375] | Garn H , Coronel C , Waser M , Caravias G , Ransmayr G ((2017) ) Differential diagnosis between patients with probable Alzheimer’s disease, Parkinson’s disease dementia, or dementia with Lewy bodies and frontotemporal dementia, behavioral variant, using quantitative electroencephalographic features. J Neural Transm 124: , 569–581. |
[376] | Dauwan M , van der Zande JJ , van Dellen E , Sommer IEC , Scheltens P , Lemstra AW , Stam CJ ((2016) ) Random forest to differentiate dementia with Lewy bodies from Alzheimer’s disease. Alzheimers Dement (Amst) 4: , 99–106. |
[377] | Babiloni C , Del Percio C , Lizio R , Noce G , Cordone S , Lopez S , Soricelli A , Ferri R , Pascarelli MT , Nobili F , Arnaldi D , Famà F , Aarsland D , Orzi F , Buttinelli C , Giubilei F , Onofrj M , Stocchi F , Stirpe P , Fuhr P , Gschwandtner U , Ransmayr G , Caravias G , Garn H , Sorpresi F , Pievani M , D’Antonio F , De Lena C , Güntekin B , Hanoğlu L , Başar E , Yener G , Emek-Savaş DD , Triggiani AI , Franciotti R , Frisoni GB , Bonanni L , De Pandis MF ((2017) ) Abnormalities of cortical neural synchronization mechanisms in subjects with mild cognitive impairment due to Alzheimer’s and Parkinson’s diseases: An EEG study. J Alzheimers Dis 59: , 339–358. |
[378] | Rossini PM , Del Percio C , Pasqualetti P , Cassetta E , Binetti G , Dal Forno G , Ferreri F , Frisoni G , Chiovenda P , Miniussi C , Parisi L , Tombini M , Vecchio F , Babiloni C ((2006) ) Conversion from mild cognitive impairment to Alzheimer’s disease is predicted by sources and coherence of brain electroencephalography rhythms. Neuroscience 143: , 793–803. |
[379] | van der Hiele K , Bollen ELEM , Vein AA , Reijntjes RHAM , Westendorp RGJ , van Buchem MA , Middelkoop HAM , van Dijk JG ((2008) ) EEG markers of future cognitive performance in the elderly. J Clin Neurophysiol 25: , 83–89. |
[380] | Luckhaus C , Grass-Kapanke B , Blaeser I , Ihl R , Supprian T , Winterer G , Zielasek J , Brinkmeyer J ((2008) ) Quantitative EEG in progressingvsstable mild cognitive impairment (MCI): Results of a 1-year follow-up study. Int J Geriatr Psychiatry 23: , 1148–1155. |
[381] | Walla P , Püregger E , Lehrner J , Mayer D , Deecke L , Dal Bianco P ((2004) ) Depth of word processing in Alzheimer patients and normal controls: A magnetoencephalographic (MEG) study. J Neural Transm 112: , 713–730. |
[382] | Garrido MI , Kilner JM , Stephan KE , Friston KJ ((2009) ) The mismatch negativity: A review of underlying mechanisms. Clin Neurophysiol 120: , 453–463. |
[383] | Näätänen R , Paavilainen P , Rinne T , Alho K ((2007) ) The mismatch negativity (MMN) in basic research of central auditory processing: A review. Clin Neurophysiol 118: , 2544–2590. |
[384] | Cheng C-H , Wang P-N , Hsu W-Y , Lin Y-Y ((2012) ) Inadequate inhibition of redundant auditory inputs in Alzheimer’s disease: An MEG study. Biol Psychol 89: , 365–373. |
[385] | Pekkonen E ((2000) ) Mismatch negativity in aging and in Alzheimer’s and Parkinson’s diseases. Audiol Neurotol 5: , 216–224. |
[386] | Deeny SP , Poeppel D , Zimmerman JB , Roth SM , Brandauer J , Witkowski S , Hearn JW , Ludlow AT , Contreras-Vidal JL , Brandt J , Hatfield BD ((2008) ) Exercise, APOE, and working memory: MEG and behavioral evidence for benefit of exercise in epsilon4 carriers. Biol Psychol 78: , 179–187. |
[387] | Maestú F , Campo P , Gil-Gregorio P , Fernández S , Fernández A , Ortiz T ((2006) ) Medial temporal lobe neuromagnetic hypoactivation and risk for developing cognitive decline in elderly population: A 2-year follow-up study. Neurobiol Aging 27: , 32–37. |
[388] | Stam CJ ((2010) ) Use of magnetoencephalography (MEG) to study functional brain networks in neurodegenerative disorders. J Neurol Sci 289: , 128–134. |
[389] | Raichle ME , MacLeod AM , Snyder AZ , Powers WJ , Gusnard DA , Shulman GL ((2001) ) A default mode of brain function. Proc Natl Acad Sci U S A 98: , 676–682. |
[390] | Brookes MJ , Hale JR , Zumer JM , Stevenson CM , Francis ST , Barnes GR , Owen JP , Morris PG , Nagarajan SS ((2011) ) Measuring functional connectivity using MEG: Methodology and comparison with fcMRI. Neuroimage 56: , 1082–1104. |
[391] | Brookes MJ , Tewarie PK , Hunt BAE , Robson SE , Gascoyne LE , Liddle EB , Liddle PF , Morris PG ((2016) ) A multi-layer network approach to MEG connectivity analysis. Neuroimage 132: , 425–438. |
[392] | Marzetti L , Della Penna S , Snyder AZ , Pizzella V , Nolte G , de Pasquale F , Romani GL , Corbetta M ((2013) ) Frequency specific interactions of MEG resting state activity within and across brain networks as revealed by the multivariate interaction measure. Neuroimage 79: , 172–183. |
[393] | de Pasquale F , Della Penna S , Snyder AZ , Lewis C , Mantini D , Marzetti L , Belardinelli P , Ciancetta L , Pizzella V , Romani GL , Corbetta M ((2010) ) Temporal dynamics of spontaneous MEG activity in brain networks. Proc Natl Acad Sci U S A 107: , 6040–6045. |
[394] | de Pasquale F , Della Penna S , Sporns O , Romani GL , Corbetta M ((2015) ) A dynamic core network and global efficiency in the resting human brain. Cereb Cortex 26: , 4015–4033. |
[395] | Tewarie P , Hillebrand A , van Dijk BW , Stam CJ , O’Neill GC , Van Mieghem P , Meier JM , Woolrich MW , Morris PG , Brookes MJ ((2016) ) Integrating cross-frequency and within band functional networks in resting-state MEG: A multi-layer network approach. Neuroimage 142: , 324–336. |
[396] | Sockeel S , Schwartz D , Pélégrini-Issac M , Benali H ((2016) ) Large-scale functional networks identified from resting-state EEG using spatial ICA. PLoS One 11: , e0146845. |
[397] | Besga A , Ortiz L , Fernández A , Maestu F , Arrazola J , Gil-Gregorio P , Fuentes M , Ortiz T ((2010) ) Structural and functional patterns in healthy aging, mild cognitive impairment, and Alzheimer disease. Alzheimer Dis Assoc Disord 24: , 1–10. |
[398] | Fernández A , Hornero R , Mayo A , Poza J , Gil-Gregorio P , Ortiz T ((2006) ) MEG spectral profile in Alzheimer’s disease and mild cognitive impairment. Clin Neurophysiol 117: , 306–314. |
[399] | Fernández A , Turrero An , Zuluaga P , Gil-Gregorio P , Maestu F , Campo P , Ortiz T ((2006) ) IC-P-046. Alzheimers Dement 2: , S673. |
[400] | Fernandez A , Turrero A , Zuluaga P , Gil-Gregorio P , del Pozo F , Maestu F , Moratti S ((2013) ) MEG delta mapping along the healthy aging-Alzheimer’s disease continuum: Diagnostic implications. J Alzheimers Dis 35: , 495–507. |
[401] | de Haan W , Stam CJ , Jones BF , Zuiderwijk I , van Dijk BW , Scheltens P ((2008) ) P1-388: Resting-state oscillatory brain dynamics in Alzheimer’s disease. Alzheimers Dement 4: , T332–T333. |
[402] | Osipova D , Rantanen K , Ahveninen J , Ylikoski R , Häppölä O , Strandberg T , Pekkonen E ((2006) ) Source estimation of spontaneous MEG oscillations in mild cognitive impairment. Neurosci Lett 405: , 57–61. |
[403] | Guillon J , Attal Y , Colliot O , La Corte V , Dubois B , Schwartz D , Chavez M , De Vico Fallani F ((2017) ) Loss of brain inter-frequency hubs in Alzheimer’s disease. Sci Rep 7: , 10879. |
[404] | López ME , Cuesta P , Garcés P , Castellanos PN , Aurtenetxe S , Bajo R , Marcos A , Delgado ML , Montejo P , López-Pantoja JL , Maestú F , Fernandez A ((2014) ) MEG spectral analysis in subtypes of mild cognitive impairment. AGE 36: , 9624. |
[405] | Zamrini E , Maestu F , Pekkonen E , Funke M , Makela J , Riley M , Bajo R , Sudre G , Fernandez A , Castellanos N , del Pozo F , Stam CJ , van Dijk BW , Bagic A , Becker JT ((2011) ) Magnetoencephalography as a putative biomarker for Alzheimer’s disease. Int J Alzheimers Dis 2011: , 1–10. |
[406] | Hall SD , Barnes GR , Furlong PL , Seri S , Hillebrand A ((2010) ) Neuronal network pharmacodynamics of GABAergic modulation in the human cortex determined using pharmaco-magnetoencephalography. Hum Brain Mapp 31: , 581–594. |
[407] | Muthukumaraswamy SD ((2014) ) The use of magnetoencephalography in the study of psychopharmacology (pharmaco-MEG). J Psychopharmacol 28: , 815–829. |
[408] | Cuesta P , Barabash A , Aurtenetxe S , Garces P , Lopez ME , Bajo R , Llanero-Luque M , Ancin I , Cabranes JA , Marcos A , Sancho M , Nakamura A , Maestu F , Fernandez A ((2015) ) Source analysis of spontaneous magnetoencephalograpic activity in healthy aging and mild cognitive impairment: Influence of apolipoprotein E polymorphism. J Alzheimers Dis 43: , 259–273. |
[409] | López ME , Turrero A , Cuesta P , López-Sanz D , Bruña R , Marcos A , Gil P , Yus M , Barabash A , Cabranes JA , Maestú F , Fernández A ((2016) ) Searching for primary predictors of conversion from mild cognitive impairment to Alzheimer’s disease: A multivariate follow-up study. J Alzheimers Dis 52: , 133–143. |
[410] | Deeb W , Giordano JJ , Rossi PJ , Mogilner AY , Gunduz A , Judy JW , Klassen BT , Butson CR , Van Horne C , Deny D , Dougherty DD , Rowell D , Gerhardt GA , Smith GS , Ponce FA , Walker HC , Bronte-Stewart HM , Mayberg HS , Chizeck HJ , Langevin J-P , Volkmann J , Ostrem JL , Shute JB , Jimenez-Shahed J , Foote KD , Wagle Shukla A , Rossi MA , Oh M , Pourfar M , Rosenberg PB , Silburn PA , de Hemptine C , Starr PA , Denison T , Akbar U , Grill WM , Okun MS ((2016) ) Proceedings of the Fourth Annual Deep Brain Stimulation Think Tank: A review of emerging issues and technologies. Front Integr Neurosci 10: , 38. |
[411] | Bick SKB , Eskandar EN ((2016) ) Neuromodulation for restoring memory. Neurosurg Focus 40: , E5. |
[412] | Suthana N , Haneef Z , Stern J , Mukamel R , Behnke E , Knowlton B , Fried I ((2012) ) Memory enhancement and deep-brain stimulation of the entorhinal area. N Engl J Med 366: , 502–510. |
[413] | Kuhn J , Hardenacke K , Lenartz D , Gruendler T , Ullsperger M , Bartsch C , Mai J , Zilles K , Bauer A , Matusch A ((2015) ) Deep brain stimulation of the nucleus basalis of Meynert in Alzheimer’s dementia. Mol Psychiatry 20: , 353–360. |
[414] | Laxton AW , Tang-Wai DF , McAndrews MP , Zumsteg D , Wennberg R , Keren R , Wherrett J , Naglie G , Hamani C , Smith GS , Lozano AM ((2010) ) A phase I trial of deep brain stimulation of memory circuits in Alzheimer’s disease. Ann Neurol 68: , 521–534. |
[415] | Fontaine D , Deudon A , Lemaire JJ , Darcourt J , Paquis P , Robert P ((2012) ) Traitement symptomatique des troubles mnésiques de la maladie d’Alzheimer par stimulation électrique chronique du fornix dans l’hypothalamus. Neurochirurgie 58: , 411. |
[416] | Sankar T , Chakravarty MM , Bescos A , Lara M , Obuchi T , Laxton AW , McAndrews MP , Tang-Wai DF , Workman CI , Smith GS , Lozano AM ((2015) ) Deep brain stimulation influences brain structure in Alzheimer’s disease. Brain Stimul 8: , 645–654. |
[417] | Ponce FA , Asaad W , Foote KD , Anderson WS , Cosgrove R , Baltuch GH , Beasley KD , Reymers DE , Oh ES , Targum SD , Smith G , Lyketsos CG , Lozano AM ((2015) ) Bilateral fornix deep brain stimulation for Alzheimer disease. Neurosurgery 62: , 207. |
[418] | Smith GS , Laxton AW , Tang-Wai DF , McAndrews MP , Diaconescu AO , Workman CI , Lozano AM ((2012) ) Increased cerebral metabolism after 1 year of deep brain stimulation in Alzheimer disease. Arch Neurol 69: , 1141–1148. |
[419] | Ponce FA , Asaad WF , Foote KD , Anderson WS , Rees Cosgrove G , Baltuch GH , Beasley K , Reymers DE , Oh ES , Targum SD , Smith GS , Lyketsos CG , Lozano AM , for The ADvance Research Group ((2016) ) Bilateral deep brain stimulation of the fornix for Alzheimer’s disease: Surgical safety in the ADvance trial. J Neurosurg 125: , 75–84. |
[420] | Lozano AM , Fosdick L , Chakravarty MM , Leoutsakos J-M , Munro C , Oh E , Drake KE , Lyman CH , Rosenberg PB , Anderson WS ((2016) ) A phase II study of fornix deep brain stimulation in mild Alzheimer’s disease. J Alzheimers Dis 54: , 777–787. |
[421] | Gonsalvez I , Baror R , Fried P , Santarnecchi E , Pascual-Leone A ((2017) ) Therapeutic noninvasive brain stimulation in Alzheimer’s disease. Curr Alzheimer Res 14: , 362–376. |
[422] | Caliandro P , Padua L , Rossi A , Rossini PM , Stalberg E , Feurra M , Ulivelli M , Bartalini S , Giannini F , Rossi S ((2014) ) Jitter of corticospinal neurons during repetitive transcranial magnetic stimulation. Method and possible clinical implications. Brain Stimul 7: , 580–586. |
[423] | Wagner T , Fregni F , Fecteau S , Grodzinsky A , Zahn M , Pascual-Leone A ((2007) ) Transcranial direct current stimulation: A computer-based human model study. Neuroimage 35: , 1113–1124. |
[424] | Lefaucheur J-P , André-Obadia N , Antal A , Ayache SS , Baeken C , Benninger DH , Cantello RM , Cincotta M , de Carvalho M , De Ridder D , Devanne H , Di Lazzaro V , Filipović SR , Hummel FC , Jääskeläinen SK , Kimiskidis VK , Koch G , Langguth B , Nyffeler T , Oliviero A , Padberg F , Poulet E , Rossi S , Rossini PM , Rothwell JC , Schönfeldt-Lecuona C , Siebner HR , Slotema CW , Stagg CJ , Valls-Sole J , Ziemann U , Paulus W , Garcia-Larrea L ((2014) ) Evidence-based guidelines on the therapeutic use of repetitive transcranial magnetic stimulation (rTMS). Clin Neurophysiol 125: , 2150–2206. |
[425] | Lefaucheur J-P , Antal A , Ayache SS , Benninger DH , Brunelin J , Cogiamanian F , Cotelli M , De Ridder D , Ferrucci R , Langguth B , Marangolo P , Mylius V , Nitsche MA , Padberg F , Palm U , Poulet E , Priori A , Rossi S , Schecklmann M , Vanneste S , Ziemann U , Garcia-Larrea L , Paulus W ((2017) ) Evidence-based guidelines on the therapeutic use of transcranial direct current stimulation (tDCS). Clin Neurophysiol 128: , 56–92. |
[426] | Ridding MC , Rothwell JC ((2007) ) Is there a future for therapeutic use of transcranial magnetic stimulation? Nat Rev Neurosci 8: , 559–567. |
[427] | Ahmed MA , Darwish ES , Khedr EM , El serogy YM , Ali AM ((2011) ) Effects of low versus high frequencies of repetitive transcranial magnetic stimulation on cognitive function and cortical excitability in Alzheimer’s dementia. J Neurol 259: , 83–92. |
[428] | Rabey JM , Dobronevsky E , Aichenbaum S , Gonen O , Marton RG , Khaigrekht M ((2013) ) Repetitive transcranial magnetic stimulation combined with cognitive training is a safe and effective modality for the treatment of Alzheimer’s disease: A randomized, double-blind study. J Neural Transm (Vienna) 120: , 813–819. |
[429] | Rabey JM , Dobronevsky E ((2016) ) Repetitive transcranial magnetic stimulation (rTMS) combined with cognitive training is a safe and effective modality for the treatment of Alzheimer’s disease: Clinical experience. J Neural Transm 123: , 1449–1455. |
[430] | Toschi N , Keck ME , Welt T , Guerrisi M ((2012) ) Quantifying uncertainty in Transcranial Magnetic Stimulation - A high resolution simulation study in ICBM space. Conf Proc IEEE Eng Med Biol Soc 2012: , 1218–1221. |
[431] | Toschi N , Welt T , Guerrisi M , Keck ME ((2009) ) Transcranial magnetic stimulation in heterogeneous brain tissue: Clinical impact on focality, reproducibility and true sham stimulation. J Psychiatr Res 43: , 255–264. |
[432] | Toschi N , Welt T , Guerrisi M , Keck ME ((2008) ) A reconstruction of the conductive phenomena elicited by transcranial magnetic stimulation in heterogeneous brain tissue. Phys Med 24: , 80–86. |
[433] | Tatti E , Rossi S , Innocenti I , Rossi A , Santarnecchi E ((2016) ) Non-invasive brain stimulation of the aging brain: State of the art and futureerspectives. Ageing Res Rev 29: , 66–89. |
[434] | Goutagny R , Gu N , Cavanagh C , Jackson J , Chabot J-G , Quirion R , Krantic S , Williams S ((2013) ) Alterations in hippocampal network oscillations and theta-gamma coupling arise before Aβ overproduction in a mouse model of Alzheimer’s disease. Eur J Neurosci 37: , 1896–1902. |
[435] | Iaccarino HF , Singer AC , Martorell AJ , Rudenko A , Gao F , Gillingham TZ , Mathys H , Seo J , Kritskiy O , Abdurrob F , Adaikkan C , Canter RG , Rueda R , Brown EN , Boyden ES , Tsai L-H ((2016) ) Gamma frequency entrainment attenuates amyloid load and modifies microglia. Nature 540: , 230–235. |
[436] | Musaeus CS , Shafi MM , Santarnecchi E , Herman ST , Press DZ ((2017) ) Levetiracetam alters oscillatory connectivity in Alzheimer’s disease. J Alzheimers Dis 58: , 1065–1076. |
[437] | Antal A , Alekseichuk I , Bikson M , Brockmöller J , Brunoni AR , Chen R , Cohen LG , Dowthwaite G , Ellrich J , Flöel A , Fregni F , George MS , Hamilton R , Haueisen J , Herrmann CS , Hummel FC , Lefaucheur JP , Liebetanz D , Loo CK , McCaig CD , Miniussi C , Miranda PC , Moliadze V , Nitsche MA , Nowak R , Padberg F , Pascual-Leone A , Poppendieck W , Priori A , Rossi S , Rossini PM , Rothwell J , Rueger MA , Ruffini G , Schellhorn K , Siebner HR , Ugawa Y , Wexler A , Ziemann U , Hallett M , Paulus W ((2017) ) Low intensity transcranial electric stimulation: Safety, ethical, legal regulatory and application guidelines. Clin Neurophysiol 128: , 1774–1809. |
[438] | Fröhlich F , McCormick DA ((2010) ) Endogenous electric fields may guide neocortical network activity. Neuron 67: , 129–143. |
[439] | Reato D , Rahman A , Bikson M , Parra LC ((2010) ) Low-intensity electrical stimulation affects network dynamics by modulating population rate and spike timing. J Neurosci 30: , 15067–15079. |
[440] | Feurra M , Bianco G , Del Testa M , Santarnecchi E , Rossi A , Rossi S ((2011) ) P14.21 Frequency-dependent tuning of human motor system induced by transcranial oscillatory potentials. Clin Neurophysiol 122: , S126. |
[441] | Feurra M , Pasqualetti P , Bianco G , Santarnecchi E , Rossi A , Rossi S ((2013) ) State-dependent effects of transcranial oscillatory currents on the motor system: What you think matters. J Neurosci 33: , 17483–17489. |
[442] | Santarnecchi E , Polizzotto Nicola R , Godone M , Giovannelli F , Feurra M , Matzen L , Rossi A , Rossi S ((2013) ) Frequency-dependent enhancement of fluid intelligence induced by transcranial oscillatory potentials. Curr Biol 23: , 1449–1453. |
[443] | Santarnecchi E , Muller T , Rossi S , Sarkar A , Polizzotto NR , Rossi A , Cohen Kadosh R ((2016) ) Individual differences and specificity of prefrontal gamma frequency-tACS on fluid intelligence capabilities. Cortex 75: , 33–43. |
[444] | Santarnecchi E , Biasella A , Tatti E , Rossi A , Prattichizzo D , Rossi S ((2017) ) High-gamma oscillations in the motor cortex during visuo-motor coordination: A tACS interferential study. Brain Res Bull 131: , 47–54. |
[445] | Jack CR , Wiste HJ , Weigand SD , Therneau TM , Lowe VJ , Knopman DS , Gunter JL , Senjem ML , Jones DT , Kantarci K , Machulda MM , Mielke MM , Roberts RO , Vemuri P , Reyes DA , Petersen RC ((2017) ) Defining imaging biomarker cut points for brain aging and Alzheimer’s disease. Alzheimers Dement 13: , 205–216. |
[446] | Foster NL , Heidebrink JL , Clark CM , Jagust WJ , Arnold SE , Barbas NR , DeCarli CS , Scott Turner R , Koeppe RA , Higdon R , Minoshima S ((2007) ) FDG-PET improves accuracy in distinguishing frontotemporal dementia and Alzheimer’s disease. Brain 130: , 2616–2635. |
[447] | Lehmann M , Ghosh PM , Madison C , Laforce R Jr , Corbetta-Rastelli C , Weiner MW , Greicius MD , Seeley WW , Gorno-Tempini ML , Rosen HJ ((2013) ) Diverging patterns of amyloid deposition and hypometabolism in clinical variants of probable Alzheimer’s disease. Brain 136: , 844–858. |
[448] | Dowling NM , Johnson SC , Gleason CE , Jagust WJ , Alzheimer’s Disease Neuroimaging Initiative ((2015) ) The mediational effects of FDG hypometabolism on the association between cerebrospinal fluid biomarkers and neurocognitive function. Neuroimage 105: , 357–368. |
[449] | Morbelli S , Perneczky R , Drzezga A , Frisoni GB , Caroli A , van Berckel BNM , Ossenkoppele R , Guedj E , Didic M , Brugnolo A , Naseri M , Sambuceti G , Pagani M , Nobili F ((2013) ) Metabolic networks underlying cognitive reserve in prodromal Alzheimer disease: A European Alzheimer Disease Consortium Project. J Nucl Med 54: , 894–902. |
[450] | Chételat G , Ossenkoppele R , Villemagne VL , Perrotin A , Landeau B , Mézenge F , Jagust WJ , Dore V , Miller BL , Egret S ((2016) ) Atrophy, hypometabolism and clinical trajectories in patients with amyloid-negative Alzheimer’s disease. Brain 139: , 2528–2539. |
[451] | Tan RH , Kril JJ , Yang Y , Tom N , Hodges JR , Villemagne VL , Rowe CC , Leyton CE , Kwok JBJ , Ittner LM , Halliday GM ((2017) ) Assessment of amyloid β in pathologically confirmed frontotemporal dementia syndromes. Alzheimers Dement (Amst) 9: , 10–20. |
[452] | Ossenkoppele R , Jansen WJ , Rabinovici GD , Knol DL , van der Flier WM , van Berckel BNM , Scheltens P , Visser PJ , Verfaillie SCJ , Zwan MD , Adriaanse SM , Lammertsma AA , Barkhof F , Jagust WJ , Miller BL , Rosen HJ , Landau SM , Villemagne VL , Rowe CC , Lee DY , Na DL , Seo SW , Sarazin M , Roe CM , Sabri O , Barthel H , Koglin N , Hodges J , Leyton CE , Vandenberghe R , van Laere K , Drzezga A , Forster S , Grimmer T , Sánchez-Juan P , Carril JM , Mok V , Camus V , Klunk WE , Cohen AD , Meyer PT , Hellwig S , Newberg A , Frederiksen KS , Fleisher AS , Mintun MA , Wolk DA , Nordberg A , Rinne JO , Chételat G , Lleo A , Blesa R , Fortea J , Madsen K , Rodrigue KM , Brooks DJ ((2015) ) Prevalence of amyloid PET positivity in dementia syndromes. JAMA 313: , 1939. |
[453] | Brosch JR , Farlow MR , Risacher SL , Apostolova LG ((2016) ) Tau imaging in Alzheimer’s disease diagnosis and clinical trials. Neurotherapeutics 14: , 62–68. |
[454] | Janssen B , Vugts DJ , Funke U , Molenaar GT , Kruijer PS , van Berckel BNM , Lammertsma AA , Windhorst AD ((2016) ) Imaging of neuroinflammation in Alzheimer’s disease, multiple sclerosis and stroke: Recent developments in positron emission tomography. Biochim Biophys Acta 1862: , 425–441. |
[455] | Hamelin L , Lagarde J , Dorothée G , Leroy C , Labit M , Comley RA , De Souza LC , Corne H , Dauphinot L , Bertoux M ((2016) ) Early and protective microglial activation in Alzheimer’s disease: A prospective study using 18 F-DPA-714 PET imaging. Brain 139: , 1252–1264. |
[456] | Hiraoka K , Okamura N , Funaki Y , Hayashi A , Tashiro M , Hisanaga K , Fujii T , Takeda A , Yanai K , Iwata R , Mori E ((2012) ) Cholinergic deficit and response to donepezil therapy in Parkinson’s disease with dementia. Eur Neurol 68: , 137–143. |
[457] | Ziebell M , Andersen BB , Pinborg LH , Knudsen GM , Stokholm J , Thomsen G , Karlsborg M , Hogh P , Mork ML , Hasselbalch SG ((2013) ) Striatal dopamine transporter binding does not correlate with clinical severity in dementia with Lewy bodies. J Nucl Med 54: , 1072–1076. |
[458] | Chang LYL , Lowe J , Ardiles A , Lim J , Grey AC , Robertson K , Danesh-Meyer H , Palacios AG , Acosta ML ((2014) ) Alzheimer’s disease in the human eye. Clinical tests that identify ocular and visual information processing deficit as biomarkers. Alzheimers Dement 10: , 251–261. |
[459] | Sadun AA , Borchert M , DeVita E , Hinton DR , Bassi CJ ((1987) ) Assessment of visual impairment in patients with Alzheimer’s disease. Am J Ophthalmol 104: , 113–120. |
[460] | Gilmore GC , Wenk HE , Naylor LA , Koss E ((1994) ) Motion perception and Alzheimer’s disease. J Gerontol 49: , P52–P57. |
[461] | Trick GL , Trick LR , Morris P , Wolf M ((1995) ) Visual field loss in senile dementia of the Alzheimer’s type. Neurology 45: , 68–74. |
[462] | Risacher SL , WuDunn D , Pepin SM , MaGee TR , McDonald BC , Flashman LA , Wishart HA , Pixley HS , Rabin LA , Pare N , Englert JJ , Schwartz E , Curtain JR , West JD , O’Neill DP , Santulli RB , Newman RW , Saykin AJ ((2013) ) Visual contrast sensitivity in Alzheimer’s disease, mild cognitive impairment, and older adults with cognitive complaints. Neurobiol Aging 34: , 1133–1144. |
[463] | Bambo MP , Garcia-Martin E , Otin S , Pinilla J , Larrosa JM , Polo V , Pablo LE ((2015) ) Visual function and retinal nerve fibre layer degeneration in patients with Alzheimer disease: Correlations with severity of dementia. Acta Ophthalmol 93: , e507–508. |
[464] | Tzekov R , Mullan M ((2014) ) Vision function abnormalities in Alzheimer disease. Surv Ophthalmol 59: , 414–433. |
[465] | Ju Y-ES , McLeland JS , Toedebusch CD , Xiong C , Fagan AM , Duntley SP , Morris JC , Holtzman DM ((2013) ) Sleep quality and preclinical Alzheimer disease. JAMA Neurol 70: , 587. |
[466] | La Morgia C , Ross-Cisneros FN , Koronyo Y , Hannibal J , Gallassi R , Cantalupo G , Sambati L , Pan BX , Tozer KR , Barboni P , Provini F , Avanzini P , Carbonelli M , Pelosi A , Chui H , Liguori R , Baruzzi A , Koronyo-Hamaoui M , Sadun AA , Carelli V ((2016) ) Melanopsin retinal ganglion cell loss in Alzheimer disease. Ann Neurol 79: , 90–109. |
[467] | Chen J-C , Espeland MA , Brunner RL , Lovato LC , Wallace RB , Leng X , Phillips LS , Robinson JG , Kotchen JM , Johnson KC , Manson JE , Stefanick ML , Sarto GE , Mysiw WJ ((2016) ) Sleep duration, cognitive decline, and dementia risk in older women. Alzheimers Dement 12: , 21–33. |
[468] | Chwiszczuk L , Breitve M , Hynninen M , Gjerstad MD , Aarsland D , Rongve A ((2015) ) Higher frequency and complexity of sleep disturbances in dementia with Lewy bodies as compared to Alzheimer’s disease. Neurodegener Dis 16: , 152–160. |
[469] | Emamian F , Khazaie H , Tahmasian M , Leschziner GD , Morrell MJ , Hsiung G-YR , Rosenzweig I , Sepehry AA ((2016) ) The association between obstructive sleep apnea and Alzheimer’s disease: A meta-analysis perspective. Front Aging Neurosci 8: , 78. |
[470] | Katsunuma H , Shimizu T , Ogawa K , Kubo H , Ishida H , Yoshihama A ((1998) ) Treatment of insomnia by concomitant therapy with Zopiclone and Aniracetam in patients with cerebral infarction, cerebroatrophy, Alzheimer’s disease and Parkinson’s disease. Psychiatry Clin Neurosci 52: , 198–200. |
[471] | Vitiello MV , Borson S ((2001) ) Sleep disturbances in patients with Alzheimer’s disease: Epidemiology, pathophysiology and treatment. CNS Drugs 15: , 777–796. |
[472] | Hart NJ , Koronyo Y , Black KL , Koronyo-Hamaoui M ((2016) ) Ocular indicators of Alzheimer’s: Exploring disease in the retina. Acta Neuropathol 132: , 767–787. |
[473] | Armstrong R , Kergoat H ((2015) ) Oculo-visual changes and clinical considerations affecting older patients with dementia. Ophthalmic Physiol Opt 35: , 352–376. |
[474] | Bayhan HA , Aslan Bayhan S , Celikbilek A , Tanik N , Gurdal C ((2015) ) Evaluation of the chorioretinal thickness changes in Alzheimer’s disease using spectral-domain optical coherence tomography. Clin Exp Ophthalmol 43: , 145–151. |
[475] | Blanks JC , Hinton DR , Sadun AA , Miller CA ((1989) ) Retinal ganglion cell degeneration in Alzheimer’s disease. Brain Res 501: , 364–372. |
[476] | Blanks JC , Schmidt SY , Torigoe Y , Porrello KV , Hinton DR , Blanks RHI ((1996) ) Retinal pathology in Alzheimer’s disease. II. Regional neuron loss and glial changes in GCL. Neurobiol Aging 17: , 385–395. |
[477] | Coppola G , Di Renzo A , Ziccardi L , Martelli F , Fadda A , Manni G , Barboni P , Pierelli F , Sadun AA , Parisi V ((2015) ) Optical coherence tomography in Alzheimer’s disease: A meta-analysis. PLoS One 10: , e0134750. |
[478] | Feke GT , Hyman BT , Stern RA , Pasquale LR ((2015) ) Retinal blood flow in mild cognitive impairment and Alzheimer’s disease. Alzheimers Dement (Amst) 1: , 144–151. |
[479] | Frost S , Kanagasingam Y , Sohrabi H , Vignarajan J , Bourgeat P , Salvado O , Villemagne V , Rowe CC , Lance Macaulay S , Szoeke C , Ellis KA , Ames D , Masters CL , Rainey-Smith S , Martins RN ((2013) ) Retinal vascular biomarkers for early detection and monitoring of Alzheimer’s disease. Transl Psychiatry 3: , e233. |
[480] | Gharbiya M , Trebbastoni A , Parisi F , Manganiello S , Cruciani F , D’Antonio F , De Vico U , Imbriano L , Campanelli A , De Lena C ((2014) ) Choroidal thinning as a new finding in Alzheimer’s disease: Evidence from enhanced depth imaging spectral domain optical coherence tomography. J Alzheimers Dis 40: , 907–917. |
[481] | Goldstein LE , Muffat JA , Cherny RA , Moir RD , Ericsson MH , Huang X , Mavros C , Coccia JA , Faget KY , Fitch KA , Masters CL , Tanzi RE , Chylack LT , Bush AI ((2003) ) Cytosolic β-amyloid deposition and supranuclear cataracts in lenses from people with Alzheimer’s disease. Lancet 361: , 1258–1265. |
[482] | Hinton DR , Sadun AA , Blanks JC , Miller CA ((1986) ) Optic-nerve degeneration in Alzheimer’s disease. N Engl J Med 315: , 485–487. |
[483] | Kergoat H , Kergoat M-J , Justino L , Chertkow H , Robillard A , Bergman H ((2001) ) An evaluation of the retinal nerve fiber layer thickness by scanning laser polarimetry in individuals with dementia of the Alzheimer type. Acta Ophthalmol Scand 79: , 187–191. |
[484] | Kirbas S , Turkyilmaz K , Anlar O , Tufekci A , Durmus M ((2013) ) Retinal nerve fiber layer thickness in patients with Alzheimer disease. J Neuroophthalmol 33: , 58–61. |
[485] | Koronyo Y , Biggs D , Barron E , Boyer DS , Pearlman JA , Au WJ , Kile SJ , Blanco A , Fuchs D-T , Ashfaq A , Frautschy S , Cole GM , Miller CA , Hinton DR , Verdooner SR , Black KL , Koronyo-Hamaoui M ((2017) ) Retinal amyloid pathology and proof-of-concept imaging trial in Alzheimer’s disease. JCI Insight 2: , 93621. |
[486] | Paquet C , Boissonnot M , Roger F , Dighiero P , Gil R , Hugon J ((2007) ) Abnormal retinal thickness in patients with mild cognitive impairment and Alzheimer’s disease. Neurosci Lett 420: , 97–99. |
[487] | Patton N , Aslam T , MacGillivray T , Pattie A , Deary IJ , Dhillon B ((2005) ) Retinal vascular image analysis as a potential screening tool for cerebrovascular disease: A rationale based on homology between cerebral and retinal microvasculatures. J Anat 206: , 319–348. |
[488] | Sadun AA , Bassi CJ ((1990) ) Optic nerve damage in Alzheimer’s disease. Ophthalmology 97: , 9–17. |
[489] | Schön C , Hoffmann NA , Ochs SM , Burgold S , Filser S , Steinbach S , Seeliger MW , Arzberger T , Goedert M , Kretzschmar HA , Schmidt B , Herms J ((2012) ) Long-term in vivo imaging of fibrillar tau in the retina of P301S transgenic mice. PLoS One 7: , e53547. |
[490] | Koronyo-Hamaoui M , Koronyo Y , Ljubimov AV , Miller CA , Ko MK , Black KL , Schwartz M , Farkas DL ((2011) ) Identification of amyloid plaques in retinas from Alzheimer’s patients and noninvasive in vivo optical imaging of retinal plaques in a mouse model. Neuroimage 54: , S204–S217. |
[491] | Berisha F , Feke GT , Trempe CL , McMeel JW , Schepens CL ((2007) ) Retinal abnormalities in early Alzheimer’s disease. Invest Ophthalmol Vis Sci 48: , 2285. |
[492] | Cheung CY-l , Ong YT , Ikram MK , Ong SY , Li X , Hilal S , Catindig J-AS , Venketasubramanian N , Yap P , Seow D , Chen CP , Wong TY ((2014) ) Microvascular network alterations in the retina of patients with Alzheimer’s disease. Alzheimers Dement 10: , 135–142. |
[493] | Curcio CA , Drucker DN ((2004) ) Retinal ganglion cells in Alzheimer’s disease and aging. Ann Neurol 33: , 248–257. |
[494] | Frost S , Martins RN , Kanagasingam Y ((2010) ) Ocular biomarkers for early detection of Alzheimer’s disease. J Alzheimers Dis 22: , 1–16. |
[495] | Gao L , Liu Y , Li X , Bai Q , Liu P ((2015) ) Abnormal retinal nerve fiber layer thickness and macula lutea in patients with mild cognitive impairment and Alzheimer’s disease. Arch Gerontol Geriatr 60: , 162–167. |
[496] | Guo L , Duggan J , Cordeiro M ((2010) ) Alzheimer’s disease and retinal neurodegeneration. Curr Alzheimer Res 7: , 3–14. |
[497] | Kapasi A , Schneider JA ((2016) ) Vascular contributions to cognitive impairment, clinical Alzheimer’s disease, and dementia in older persons. Biochim Biophys Acta 1862: , 878–886. |
[498] | Kromer R , Serbecic N , Hausner L , Froelich L , Aboul-Enein F , Beutelspacher SC ((2014) ) Detection of retinal nerve fiber layer defects in Alzheimer’s disease using SD-OCT. Front Psychiatry 5: , 22. |
[499] | Liu D , Zhang L , Li Z , Zhang X , Wu Y , Yang H , Min B , Zhang X , Ma D , Lu Y ((2015) ) Thinner changes of the retinal nerve fiber layer in patients with mild cognitive impairment and Alzheimer’s disease. BMC Neurol 15: , 14. |
[500] | Marziani E , Pomati S , Ramolfo P , Cigada M , Giani A , Mariani C , Staurenghi G ((2013) ) Evaluation of retinal nerve fiber layer and ganglion cell layer thickness in Alzheimer’s disease using spectral-domain optical coherence tomography. Invest Ophthalmol Vis Sci 54: , 5953. |
[501] | Moreno-Ramos T , Benito-Leon J , Villarejo A , Bermejo-Pareja F ((2013) ) Retinal nerve fiber layer thinning in dementia associated with Parkinson’s disease, dementia with Lewy bodies, and Alzheimer’s disease. J Alzheimers Dis 34: , 659–664. |
[502] | Moschos MM , Markopoulos I , Chatziralli I , Rouvas A , Papageorgiou SG , Ladas I , Vassilopoulos D ((2012) ) Structural and functional impairment of the retina and optic nerve in Alzheimer’s disease. Curr Alzheimer Res 9: , 782–788. |
[503] | Nolan JM , Loskutova E , Howard AN , Moran R , Mulcahy R , Stack J , Bolger M , Dennison J , Akuffo KO , Owens N , Thurnham DI , Beatty S ((2014) ) Macular pigment, visual function, and macular disease among subjects with Alzheimer’s disease: An exploratory study. J Alzheimers Dis 42: , 1191–1202. |
[504] | Parisi V , Restuccia R , Fattapposta F , Mina C , Bucci MG , Pierelli F ((2001) ) Morphological and functional retinal impairment in Alzheimer’s disease patients. Clin Neurophysiol 112: , 1860–1867. |
[505] | Shi ZY , Wu YJ , Wang MJ , Cao J , Feng W , Cheng Y , Li CB , Shen Y ((2014) ) Greater attenuation of retinal nerve fiber layer thickness in Alzheimer’s disease patients. J Alzheimers Dis 40: , 277–283. |
[506] | Trick GL , Barris MC , Bickler-Bluth M ((1989) ) Abnormal pattern electroretinograms in patients with senile dementia of the alzheimer type. Ann Neurol 26: , 226–231. |
[507] | Williams MA , McGowan AJ , Cardwell CR , Cheung CY , Craig D , Passmore P , Silvestri G , Maxwell AP , McKay GJ ((2015) ) Retinal microvascular network attenuation in Alzheimer’s disease. Alzheimers Dement (Amst) 1: , 229–235. |
[508] | Purves D , Augustine GJ , Fitzpatrick D , Katz LC , LaMantia A-S , McNamara JO , Williams SM ((2001) ) Sinauer, Associates Sunderland (MA). The Retina. |
[509] | Byerly MS , Blackshaw S ((2009) ) Vertebrate retina and hypothalamus development. Wiley Interdiscip Rev Syst Biol Med 1: , 380–389. |
[510] | Trost A , Lange S , Schroedl F , Bruckner D , Motloch KA , Bogner B , Kaser-Eichberger A , Strohmaier C , Runge C , Aigner L , Rivera FJ , Reitsamer HA ((2016) ) Brain and retinal pericytes: Origin, function and role. Front Cell Neurosci 10: , 20. |
[511] | Vecino E , Rodriguez FD , Ruzafa N , Pereiro X , Sharma SC ((2016) ) Glia– neuron interactions in the mammalian retina. Prog Retin Eye Res 51: , 1–40. |
[512] | Morin PJ , Abraham CR , Amaratunga A , Johnson RJ , Huber G , Sandell JH , Fine RE ((2006) ) Amyloid precursor protein is synthesized by retinal ganglion cells, rapidly transported to the optic nerve plasma membrane and nerve terminals, and metabolized. J Neurochem 61: , 464–473. |
[513] | Cai J , Qi X , Kociok N , Skosyrski S , Emilio A , Ruan Q , Han S , Liu L , Chen Z , Bowes Rickman C , Golde T , Grant MB , Saftig P , Serneels L , de Strooper B , Joussen AM , Boulton ME ((2012) ) β-Secretase (BACE1) inhibition causes retinal pathology by vascular dysregulation and accumulation of age pigment. EMBO Mol Med 4: , 980–991. |
[514] | Li L , Luo J , Chen D , Tong JB , Zeng LP , Cao YQ , Xiang J , Luo XG , Shi JM , Wang H , Huang JF ((2016) ) BACE1 in the retina: A sensitive biomarker for monitoring early pathological changes in Alzheimer’s disease. Neural Regen Res 11: , 447–453. |
[515] | Alexandrov PN , Pogue A , Bhattacharjee S , Lukiw WJ ((2011) ) Retinal amyloid peptides and complement factor H in transgenic models of Alzheimer’s disease. Neuroreport 22: , 623–627. |
[516] | Tsai Y , Lu B , Ljubimov AV , Girman S , Ross-Cisneros FN , Sadun AA , Svendsen CN , Cohen RM , Wang S ((2014) ) Ocular changes in TgF344-AD rat model of Alzheimer’s disease. Invest Ophthalmol Vis Sci 55: , 523. |
[517] | Ho CY , Troncoso JC , Knox D , Stark W , Eberhart CG ((2014) ) Beta-amyloid, phospho-tau and alpha-synuclein deposits similar to those in the brain are not identified in the eyes of Alzheimer’s and Parkinson’s disease patients. Brain Pathol 24: , 25–32. |
[518] | Kesler A , Vakhapova V , Korczyn AD , Naftaliev E , Neudorfer M ((2011) ) Retinal thickness in patients with mild cognitive impairment and Alzheimer’s disease. Clin Neurol Neurosurg 113: , 523–526. |
[519] | Lu Y , Li Z , Zhang X , Ming B , Jia J , Wang R , Ma D ((2010) ) Retinal nerve fiber layer structure abnormalities in early Alzheimer’s disease: Evidence in optical coherence tomography. Neurosci Lett 480: , 69–72. |
[520] | Cordeiro MF , Guo L , Luong V , Harding G , Wang W , Jones HE , Moss SE , Sillito AM , Fitzke FW ((2004) ) Real-time imaging of single nerve cell apoptosis in retinal neurodegeneration. Proc Natl Acad Sci U S A 101: , 13352–13356. |
[521] | Du LY , Chang LYL , Ardiles AO , Tapia-Rojas C , Araya J , Inestrosa NC , Palacios AG , Acosta ML ((2015) ) Alzheimer’s disease-related protein expression in the retina of octodon degus. PLoS One 10: , e0135499. |
[522] | Dutescu RM , Li Q-X , Crowston J , Masters CL , Baird PN , Culvenor JG ((2009) ) Amyloid precursor protein processing and retinal pathology in mouse models of Alzheimer’s disease. Graefes Arch Clin Exp Ophthalmol 247: , 1213–1221. |
[523] | Edwards MM , Rodríguez JJ , Gutierrez-Lanza R , Yates J , Verkhratsky A , Lutty GA ((2014) ) Retinal macroglia changes in a triple transgenic mouse model of Alzheimer’s disease. Exp Eye Res 127: , 252–260. |
[524] | Gao L , Chen X , Tang Y , Zhao J , Li Q , Fan X , Xu H , Yin ZQ ((2015) ) Neuroprotective effect of memantine on the retinal ganglion cells of APPswe/PS1ΔE9 mice and its immunomodulatory mechanisms. Exp Eye Res 135: , 47–58. |
[525] | Gupta VK , Chitranshi N , Gupta VB , Golzan M , Dheer Y , Wall RV , Georgevsky D , King AE , Vickers JC , Chung R , Graham S ((2016) ) Amyloid β accumulation and inner retinal degenerative changes in Alzheimer’s disease transgenic mouse. Neurosci Lett 623: , 52–56. |
[526] | He Y , Zhao H , Su G ((2013) ) Ginsenoside Rg1 decreases neurofibrillary tangles accumulation in retina by regulating activities of neprilysin and PKA in retinal cells of AD mice model. J Mol Neurosci 52: , 101–106. |
[527] | Koronyo Y , Salumbides BC , Black KL , Koronyo-Hamaoui M ((2012) ) Alzheimer’s disease in the retina: Imaging retinal Aβ Plaques for early diagnosis and therapy assessment. Neurodegener Dis 10: , 285–293. |
[528] | Liu B , Rasool S , Yang Z , Glabe CG , Schreiber SS , Ge J , Tan Z ((2009) ) Amyloid-peptide vaccinations reduce beta-amyloid plaques but exacerbate vascular deposition and inflammation in the retina of Alzheimer’s transgenic mice. Am J Pathol 175: , 2099–2110. |
[529] | More SS , Vince R ((2015) ) Hyperspectral imaging signatures detect amyloidopathy in Alzheimer’s mouse retina well before onset of cognitive decline. ACS Chem Neurosci 6: , 306–315. |
[530] | Ning A , Cui J , To E , Ashe KH , Matsubara J ((2008) ) Amyloid-β deposits lead to retinal degeneration in a mouse model of Alzheimer disease. Invest Ophthalmol Vis Sci 49: , 5136. |
[531] | Park SW , Kim JH , Mook-Jung I , Kim K-W , Park WJ , Park KH , Kim JH ((2014) ) Intracellular amyloid beta alters the tight junction of retinal pigment epithelium in 5XFAD mice. Neurobiol Aging 35: , 2013–2020. |
[532] | Parthasarathy R , Chow KM , Derafshi Z , Fautsch MP , Hetling JR , Rodgers DW , Hersh LB , Pepperberg DR ((2015) ) Reduction of amyloid-beta levels in mouse eye tissues by intra-vitreally delivered neprilysin. Exp Eye Res 138: , 134–144. |
[533] | Perez SE , Lumayag S , Kovacs B , Mufson EJ , Xu S ((2009) ) β-amyloid deposition and functional impairment in the retina of the APPswe/PS1ΔE9 transgenic mouse model of Alzheimer’s disease. Invest Ophthalmol Vis Sci 50: , 793. |
[534] | Pogue AI , Dua P , Hill JM , Lukiw WJ ((2015) ) Progressive inflammatory pathology in the retina of aluminum-fed 5xFAD transgenic mice. J Inorg Biochem 152: , 206–209. |
[535] | Williams PA , Thirgood RA , Oliphant H , Frizzati A , Littlewood E , Votruba M , Good MA , Williams J , Morgan JE ((2013) ) Retinal ganglion cell dendritic degeneration in a mouse model of Alzheimer’s disease. Neurobiol Aging 34: , 1799–1806. |
[536] | Yang Y , Shiao C , Hemingway JF , Jorstad NL , Shalloway BR , Chang R , Keene CD ((2013) ) Suppressed retinal degeneration in aged wild type and APPswe/PS1ΔE9 mice by bone marrow transplantation. PLoS One 8: , e64246. |
[537] | Zhao H , Chang R , Che H , Wang J , Yang L , Fang W , Xia Y , Li N , Ma Q , Wang X ((2013) ) Hyperphosphorylation of tau protein by calpain regulation in retina of Alzheimer’s disease transgenic mouse. Neurosci Lett 551: , 12–16. |
[538] | Begum AN , Jones MR , Lim GP , Morihara T , Kim P , Heath DD , Rock CL , Pruitt MA , Yang F , Hudspeth B , Hu S , Faull KF , Teter B , Cole GM , Frautschy SA ((2008) ) Curcumin structure-function, bioavailability, and efficacy in models of neuroinflammation and Alzheimer’s disease. J Pharmacol Exp Ther 326: , 196–208. |
[539] | Dadhaniya P , Patel C , Muchhara J , Bhadja N , Mathuria N , Vachhani K , Soni MG ((2011) ) Safety assessment of a solid lipid curcumin particle preparation: Acute and subchronic toxicity studies. Food Chem Toxicol 49: , 1834–1842. |
[540] | Garcia-Alloza M , Borrelli LA , Rozkalne A , Hyman BT , Bacskai BJ ((2007) ) Curcumin labels amyloid pathology in vivo, disrupts existing plaques, and partially restores distorted neurites in an Alzheimer mouse model. J Neurochem 102: , 1095–1104. |
[541] | Goozee KG , Shah TM , Sohrabi HR , Rainey-Smith SR , Brown B , Verdile G , Martins RN ((2015) ) Examining the potential clinical value of curcumin in the prevention and diagnosis of Alzheimer’s disease. Br J Nutr 115: , 449–465. |
[542] | Gota VS , Maru GB , Soni TG , Gandhi TR , Kochar N , Agarwal MG ((2010) ) Safety and pharmacokinetics of a solid lipid curcumin particle formulation in osteosarcoma patients and healthy volunteers. J Agric Food Chem 58: , 2095–2099. |
[543] | Kumaraswamy P , Sethuraman S , Krishnan UM ((2013) ) Mechanistic insights of curcumin interactions with the core-recognition motif of β-amyloid peptide. J Agric Food Chem 61: , 3278–3285. |
[544] | Masuda Y , Fukuchi M , Yatagawa T , Tada M , Takeda K , Irie K , Akagi K-i , Monobe Y , Imazawa T , Takegoshi K ((2011) ) Solid-state NMR analysis of interaction sites of curcumin and 42-residue amyloid β-protein fibrils. Bioorg Med Chem 19: , 5967–5974. |
[545] | Mutsuga M , Chambers JK , Uchida K , Tei M , Makibuchi T , Mizorogi T , Takashima A , Nakayama H ((2012) ) Binding of curcumin to senile plaques and cerebral amyloid angiopathy in the aged brain of various animals and to neurofibrillary tangles in Alzheimer’s brain. J Vet Med Sci 74: , 51–57. |
[546] | Yang F , Lim GP , Begum AN , Ubeda OJ , Simmons MR , Ambegaokar SS , Chen PP , Kayed R , Glabe CG , Frautschy SA , Cole GM ((2005) ) Curcumin inhibits formation of amyloid beta oligomers and fibrils, binds plaques, and reduces amyloid in vivo. J Biol Chem 280: , 5892–5901. |
[547] | Ryu EK , Choe YS , Lee K-H , Choi Y , Kim B-T ((2006) ) Curcumin and dehydrozingerone derivatives: Synthesis, radiolabeling, and evaluation for β-amyloid plaque imaging. J Med Chem 49: , 6111–6119. |
[548] | Tei M , Uchida K , Mutsuga M , Chambers JK , Nakayama H ((2012) ) The binding of curcumin to various types of canine amyloid proteins. J Vet Med Sci 74: , 481–483. |
[549] | Yanagisawa D , Amatsubo T , Morikawa S , Taguchi H , Urushitani M , Shirai N , Hirao K , Shiino A , Inubushi T , Tooyama I ((2011) ) In vivo detection of amyloid β deposition using 19F magnetic resonance imaging with a 19F-containing curcumin derivative in a mouse model of Alzheimer’s disease. Neuroscience 184: , 120–127. |
[550] | Yanagisawa D , Shirai N , Amatsubo T , Taguchi H , Hirao K , Urushitani M , Morikawa S , Inubushi T , Kato M , Kato F , Morino K , Kimura H , Nakano I , Yoshida C , Okada T , Sano M , Wada Y , Wada K-n , Yamamoto A , Tooyama I ((2010) ) Relationship between the tautomeric structures of curcumin derivatives and their Aβ-binding activities in the context of therapies for Alzheimer’s disease. Biomaterials 31: , 4179–4185. |
[551] | Yanagisawa D , Taguchi H , Yamamoto A , Shirai N , Hirao K , Tooyama I ((2011) ) Curcumin has binding activity to β-amyloid oligomer. Alzheimers Dement 7: , S476–S477. |
[552] | Butovsky O , Koronyo-Hamaoui M , Kunis G , Ophir E , Landa G , Cohen H , Schwartz M ((2006) ) Glatiramer acetate fights against Alzheimer’s disease by inducing dendritic-like microglia expressing insulin-like growth factor 1. Proc Natl Acad Sci U S A 103: , 11784–11789. |
[553] | Koronyo Y , Salumbides BC , Sheyn J , Pelissier L , Li S , Ljubimov V , Moyseyev M , Daley D , Fuchs D-T , Pham M , Black KL , Rentsendorj A , Koronyo-Hamaoui M ((2015) ) Therapeutic effects of glatiramer acetate and grafted CD115+ monocytes in a mouse model of Alzheimer’s disease. Brain 138: , 2399–2422. |
[554] | Kayabasi U ((2014) ) Retinal examination for the diagnosis of Alzheimer’s disease. Int J Ophthalmic Pathol 3: , 4. |
[555] | Snyder PJ , Johnson LN , Lim YY , Santos CY , Alber J , Maruff P , Fernández B ((2016) ) Nonvascular retinal imaging markers of preclinical Alzheimer’s disease. Alzheimers Dement (Amst) 4: , 169–178. |
[556] | Einarsdottir AB , Hardarson SH , Kristjansdottir JV , Bragason DT , Snaedal J , Stefánsson E ((2015) ) Retinal oximetry imaging in Alzheimer’s disease. J Alzheimers Dis 49: , 79–83. |
[557] | Stefánsson E , Olafsdottir OB , Einarsdottir AB , Eliasdottir TS , Eysteinsson T , Vehmeijer W , Vandewalle E , Bek T , Hardarson SH ((2017) ) Retinal oximetry discovers novel biomarkers in retinal and brain diseases. Invest Ophthalmol Vis Sci 58: , BIO227. |
[558] | Archibald NK , Clarke MP , Mosimann UP , Burn DJ ((2009) ) The retina in Parkinson’s disease. Brain 132: , 1128–1145. |
[559] | Bodis-Wollner I , Kozlowski PB , Glazman S , Miri S ((2014) ) α-synuclein in the inner retina in parkinson disease. Ann Neurol 75: , 964–966. |
[560] | Calabresi PA , Balcer LJ , Frohman EM ((2010) ) Retinal pathology in multiple sclerosis: Insight into the mechanisms of neuronal pathology. Brain 133: , 1575–1577. |
[561] | Ong YT , De Silva DA , Cheung CY , Chang HM , Chen CP , Wong MC , Wong TY , Ikram MK ((2013) ) Microvascular structure and network in the retina of patients with ischemic stroke. Stroke 44: , 2121–2127. |
[562] | Scinto LF , Daffner KR , Dressler D , Ransil BI , Rentz D , Weintraub S , Mesulam M , Potter H ((1994) ) A potential noninvasive neurobiological test for Alzheimer’s disease. Science 268: , 1051–1054. Erratum in: Science (1995) 268. |
[563] | Scinto L , Wu C , Firla K , Daffner K , Saroff D , Geula C ((1999) ) Focal pathology in the Edinger-Westphal nucleus explains pupillary hypersensitivity in Alzheimer’s disease. Acta Neuropathol 97: , 557–564. |
[564] | Nowak W , Pieniazek M , Nakayama M , Hachol A ((2016) ) Feature analyses of pupil light reflex to chromatic stimuli in Alzheimer’s patients. Frontiers of Signal Processing (ICFSP), International Conference on, IEEE, pp. 58–62. |
[565] | Granholm E , Morris S , Galasko D , Shults C , Rogers E , Vukov B ((2003) ) Tropicamide effects on pupil size and pupillary light reflexes in Alzheimer’s and Parkinson’s disease. Int J Psychophysiol 47: , 95–115. |
[566] | Durrleman S , Pennec X , Trouvé A , Ayache N ((2009) ) Statistical models of sets of curves and surfaces based on currents. Med Image Anal 13: , 793–808. |
[567] | Durrleman S , Prastawa M , Charon N , Korenberg JR , Joshi S , Gerig G , Trouvé A ((2014) ) Morphometry of anatomical shape complexes with dense deformations and sparse parameters. Neuroimage 101: , 35–49. |
[568] | Gori P , Colliot O , Marrakchi-Kacem L , Worbe Y , Routier A , Poupon C , Hartmann A , Ayache N , Durrleman S ((2015) ) Joint morphometry of fiber tracts and gray matter structures using double diffeomorphisms. Inf Process Med Imaging 24: , 275–287. |
[569] | Gori P , Colliot O , Marrakchi-Kacem L , Worbe Y , Poupon C , Hartmann A , Ayache N , Durrleman S ((2017) ) A Bayesian framework for joint morphometry of surface and curve meshes in multi-object complexes. Med Image Anal 35: , 458–474. |
[570] | Schiratti J-B , Allassonniere S , Colliot O , Durrleman S ((2015) ) Learning spatiotemporal trajectories from manifold-valued longitudinal data. Adv Neural Inf Process Syst, pp. 2404–2412. |
[571] | Koval I , Schiratti JB , Routier A , Bacci M , Colliot O , Allassonnière S , Durrleman S ((2017) ) Statistical learning of spatiotemporal patterns from longitudinal manifold-valued networks. Medical Image Computing and Computer Assisted Intervention – MICCAI 2017, Springer International Publishing, pp. 451–459. |
[572] | Allassonnière S , Durrleman S , Kuhn E ((2015) ) Bayesian mixed effect atlas estimation with a diffeomorphic deformation model. SIAM J Imaging Sci 8: , 1367–1395. |
[573] | Durrleman S , Pennec X , Trouvé A , Gerig G , Ayache N ((2009) ) Spatiotemporal atlas estimation for developmental delay detection in longitudinal datasets. Medical Image Computing and Computer-Assisted Intervention – MICCAI 2009, Springer Berlin Heidelberg, pp. 297–304. |
[574] | Durrleman S , Pennec X , Trouve A , Braga J , Gerig G , Ayache N ((2013) ) Toward a comprehensive framework for the spatiotemporal statistical analysis of longitudinal shape data. Int J Comput Vis 103: , 22–59. |
[575] | Fishbaugh J , Prastawa M , Durrleman S , Piven J , Gerig G ((2012) ) Analysis of longitudinal shape variability via subject specific growth modeling. Medical ImageComputing and Computer-Assisted Intervention – MICCAI 2012 Springer Berlin Heidelberg, pp. 731–738. |
[576] | Fishbaugh J , Prastawa M , Gerig G , Durrleman S ((2013) ) Geodesic shape regression in the framework of currents. Lecture Notes in Computer Science, Springer Berlin Heidelberg, pp. 718–729. |
[577] | Schiratti JB , Allassonnière S , Colliot O , Durrleman S ((2017) ) A Bayesian mixed-effects model to learn trajectories of changes from repeated manifold-valued observations. J Mach Learn Res 3: , 1–48. |
[578] | Cummings JL , Morstorf T , Zhong K ((2014) ) Alzheimer’s disease drug-development pipeline: Few candidates, frequent failures. Alzheimers Res Ther 6: , 37. |
[579] | Cummings J , Morstorf T , Lee G ((2016) ) Alzheimer’s drug-development pipeline: 2016. Alzheimers Dement (N Y) 2: , 222–232. |
[580] | Berger SI , Iyengar R ((2009) ) Network analyses in systems pharmacology. Bioinformatics 25: , 2466–2472. |
[581] | Danhof M ((2016) ) Systems pharmacology – Towards the modeling of network interactions. Eur J Pharm Sci 94: , 4–14. |
[582] | Rask-Andersen M , Almén MS , Schiöth HB ((2011) ) Trends in the exploitation of novel drug targets. Nat Rev Drug Discov 10: , 579–590. |
[583] | Hopkins AL ((2008) ) Network pharmacology: The next paradigm in drug discovery. Nat Chem Biol 4: , 682–690. |
[584] | Campillos M , Kuhn M , Gavin AC , Jensen LJ , Bork P ((2008) ) Drug target identification using side-effect similarity. Science 321: , 263–266. |
[585] | Xie L , Ge X , Tan H , Xie L , Zhang Y , Hart T , Yang X , Bourne PE ((2014) ) Towards structural systems pharmacology to study complex diseases and personalized medicine. PLoS Comput Biol 10: , e1003554. |
[586] | Nikolic K , Mavridis L , Djikic T , Vucicevic J , Agbaba D , Yelekci K , Mitchell JBO ((2016) ) Drug design for CNS diseases: Polypharmacological profiling of compounds using cheminformatic, 3D-QSAR and virtual screening methodologies. Front Neurosci 10: , 265. |
[587] | Shahid M , Shahzad Cheema M , Klenner A , Younesi E , Hofmann-Apitius M ((2013) ) SVM based descriptor selection and classification of neurodegenerative disease drugs for pharmacological modeling. Mol Inform 32: , 241–249. |
[588] | Issa NT , Kruger J , Wathieu H , Raja R , Byers SW , Dakshanamurthy S ((2016) ) DrugGenEx-Net: A novel computational platform for systems pharmacology and gene expression-based drug repurposing. BMC Bioinformatics 17: , 202. |
[589] | Fujita KA , Ostaszewski M , Matsuoka Y , Ghosh S , Glaab E , Trefois C , Crespo I , Perumal TM , Jurkowski W , Antony PMA , Diederich N , Buttini M , Kodama A , Satagopam VP , Eifes S , del Sol A , Schneider R , Kitano H , Balling R ((2013) ) Integrating pathways of Parkinson’s disease in a molecular interaction map. Mol Neurobiol 49: , 88–102. |
[590] | Mizuno S , Iijima R , Ogishima S , Kikuchi M , Matsuoka Y , Ghosh S , Miyamoto T , Miyashita A , Kuwano R , Tanaka H ((2012) ) AlzPathway: A comprehensive map of signaling pathways of Alzheimer’s disease. BMC Syst Biol 6: , 52. |
[591] | Iyappan A , Gündel M , Shahid M , Wang J , Li H , Mevissen H-T , Müller B , Fluck J , Jirsa V , Domide L , Younesi E , Hofmann-Apitius M ((2016) ) Towards a pathway inventory of the human brain for modeling disease mechanisms underlying neurodegeneration. J Alzheimers Dis 52: , 1343–1360. |
[592] | Mueller SG , Weiner MW , Thal LJ , Petersen RC , Jack C , Jagust W , Trojanowski JQ , Toga AW , Beckett L ((2005) ) The Alzheimer’s Disease Neuroimaging Initiative. Neuroimaging Clin N Am 15: , 869–877. |
[593] | Malhotra A , Younesi E , Gündel M , Müller B , Heneka MT , Hofmann-Apitius M ((2014) ) ADO: A disease ontology representing the domain knowledge specific to Alzheimer’s disease. Alzheimers Dement 10: , 238–246. |
[594] | Kodamullil AT , Younesi E , Naz M , Bagewadi S , Hofmann-Apitius M ((2015) ) Computable cause-and-effect models of healthy and Alzheimer’s disease states and their mechanistic differential analysis. Alzheimers Dement 11: , 1329–1339. |
[595] | Emon MAEK , Kodamullil AT , Karki R , Younesi E , Hofmann-Apitius M ((2017) ) Using drugs as molecular probes: A computational chemical biology approach in neurodegenerative diseases. J Alzheimers Dis 56: , 677–686. |
[596] | Geerts H , Dacks PA , Devanarayan V , Haas M , Khachaturian ZS , Gordon MF , Maudsley S , Romero K , Stephenson D ((2016) ) Big data to smart data in Alzheimer’s disease: The brain health modeling initiative to foster actionable knowledge. Alzheimers Dement 12: , 1014–1021. |
[597] | Balch WE , Morimoto RI , Dillin A , Kelly JW ((2008) ) Adapting proteostasis for disease intervention. Science 319: , 916–919. |
[598] | Lim J , Yue ZY ((2015) ) Neuronal aggregates: Formation, clearance, and spreading. Dev Cell 32: , 491–501. |
[599] | Calandra-Buonaura G , Toschi N , Provini F , Corazza I , Bisulli F , Barletta G , Vandi S , Montagna P , Guerrisi M , Tinuper P , Cortelli P ((2012) ) Physiologic autonomic arousal heralds motor manifestations of seizures in nocturnal frontal lobe epilepsy: Implications for pathophysiology. Sleep Med 13: , 252–262. |
[600] | Valenza G , Orsolini S , Diciotti S , Citi L , Scilingo EP , Guerrisi M , Danti S , Lucetti C , Tessa C , Barbieri R ((2016) ) Assessment of spontaneous cardiovascular oscillations in Parkinson’s disease. Biomed Signal Process Control 26: , 80–89. |
[601] | Valenza G , Citi L , Garcia RG , Taylor JN , Toschi N , Barbieri R ((2017) ) Complexity variability assessment of nonlinear time-varying cardiovascular control. Sci Rep 7: , 42779. |
[602] | Duggento A , Bianciardi M , Passamonti L , Wald LL , Guerrisi M , Barbieri R , Toschi N ((2016) ) Globally conditioned Granger causality in brain-brain and brain-heart interactions: A combined heart rate variability/ultra-high-field (7 T) functional magnetic resonance imaging study. Philos Trans A Math Phys Eng Sci 374: , 20150185. |
[603] | Toschi N , Duggento A , Canichella A , Coniglione F , Dauri M , Sabato AF , Guerrisi M ((2011) ) Intra- and inter-beat modeling of cardiovascular dynamics and control: Assessing haemodynamic stability and responsiveness. Conf Proc IEEE Eng Med Biol Soc 2011: , 8440–8443. |
[604] | Duggento A , Toschi N , Guerrisi M ((2012) ) Modeling of human baroreflex: Considerations on the Seidel-Herzel model. Fluctuation Noise Lett 11: , 1240017. |
[605] | Poldrack RA ((2017) ) Precision neuroscience: Dense sampling of individual brains. Neuron 95: , 727–729. |
[606] | Gordon EM , Laumann TO , Gilmore AW , Newbold DJ , Greene DJ , Berg JJ , Ortega M , Hoyt-Drazen C , Gratton C , Sun H , Hampton JM , Coalson RS , Nguyen AL , McDermott KB , Shimony JS , Snyder AZ , Schlaggar BL , Petersen SE , Nelson SM , Dosenbach NUF ((2017) ) Precision functional mapping of individual human brains. Neuron 95: , 791–807.e7. |
[607] | Braga RM , Buckner RL ((2017) ) Parallel interdigitated distributed networks within the individual estimated by intrinsic functional connectivity. Neuron 95: , 457–471.e5. |
[608] | Dubois B , Hampel H , Feldman HH , Scheltens P , Aisen P , Andrieu S , Bakardjian H , Benali H , Bertram L , Blennow K ((2016) ) Preclinical Alzheimer’s disease: Definition, natural history, and diagnostic criteria. Alzheimers Dement 12: , 292–323. |
[609] | Petersen SE , Sporns O ((2015) ) Brain networks and cognitive architectures. Neuron 88: , 207–219. |