Cognitive Composites Domain Scores Related to Neuroimaging Biomarkers within Probable-Amnestic Mild Cognitive Impairment-Storage Subtype
Abstract
The probable-amnestic (Pr-a) mild cognitive impairment (MCI)-storage subtype is a phenotype with 8.5 times more risk of conversion to dementia, mainly Alzheimer’s disease (AD), than the possible non-amnestic (Pss-na) MCI. The aim of this study was to find the optimized cognitive composites (CCs) domain scores most related to neuroimaging biomarkers within Pr-aMCI-storage subtype patients. The Fundació ACE (ACE) study with 20 Pr-aMCI-storage subtype subjects (MCI) were analyzed. All subjects underwent a neuropsychological assessment, a structural MRI, FDG-PET, and PIB-PET. The adjusted hippocampal volume (aHV) on MRI, the standard uptake value ratio (SUVR) on FDG-PET and PIB-PET SUVR measures were analyzed. The construction of the CCs domain scores, and the aHV on MRI and FDG-PET SUVR measures, were replicated in the parental AB255 study database (n = 133 MCI). Partial correlations adjusted by age, gender, and education were calculated with the associated p-value among every CC domain score and the neuroimaging biomarkers. The results were replicated in the “MCI due to AD” with memory storage impairments from ADNI. Delayed Recall CC domain score was significantly correlated with PIB-PET SUVR (β= –0.61, p = 0.003) in the ACE study and also with aHV on MRI (β= 0.27, p = 0.01) and FDG-PET SUVR (β= 0.27, p = 0.01) in the AB255 study. After a median survival time of 20.6 months, 85% from the ACE MCI converted to AD. The replication of our results in the ADNI dataset also confirmed our findings. Delayed Recall is the CC domain score best correlated with neuroimaging biomarkers associated with prodromal AD diagnosis.
INTRODUCTION
The search for reliable Alzheimer’s disease (AD) biomarkers to identify prodromal AD [1, 2], or mild cognitive impairment (MCI) due to AD [3], already implemented or that has been proposed should be used in clinical trials and clinical diagnosis [1, 3–7], has focused on expensive methods which are often poorly tolerated by patients (e.g., PET). By contrast, neuropsychological tests are non-invasive, and may be cost-effective, better predictors of disease than neuroimaging [8]. The identification of optimal cognitive composites (CCs) domains that are related to neuroimaging biomarkers and that characterize specific phenotypes will maximize the cost-effectiveness of clinical practice and management, and recruitment into clinical trials.
In a recent study [9], at The Memory Disorders Unit from Fundació ACE (Barcelona, Spain) [10] which involved the follow-up of 550 MCI subjects for an average of 26.6 months, the present authors found that the majority (45.5%) of those MCI individuals who subsequently developed dementia displayed the AD dementia phenotype.
The MCI subjects were classified as probable/possible in function of the presence of comorbidities that could otherwise explain their cognitive deficits [9, 11–13]. Among those probable-amnestic (Pr-a) MCI patients with memory storage impairment [14] (i.e., impaired recall and recognition), there was an 8.5 times greater risk to develop dementia, mainly AD, than those with the possible MCI condition, where cognitive deficits did not include memory.
The aim of the present study was to find the optimized CC domain scores that were most related to three brain imaging biomarkers, derived from structural magnetic resonance imaging (MRI), [18F]-fluorodeoxyglucose-positron emission tomography (FDG-PET), and Pittsburgh compound B-positron emission tomography (PiB-PET) within the Pr-aMCI storage phenotype.
MATERIALS AND METHODS
Subjects
For the purpose of this study, two groups of datasets, >64 years old, were analyzed: (i) The Fundació ACE (ACE) study (n = 59) with 20 Pr-a-MCI-storage subtype subjects (MCI, from now on) (60.0% men) all with multiple domains impaired and 39 healthy controls (HC) (51.3% men), were all recruited and assessed from 2010 to 2013 at The Memory Disorders Unit from Fundació ACE, Institut Català de Neurociènces Aplicades, Barcelona, Spain [10]. All subjects underwent a neuropsychological assessment including five cognitive domains and were subjected to a structural MRI, FDG-PET, and PIB-PET; and (ii) The parental AB255 study (n = 175) with 133 MCI all with multiple domains impaired and 42 HC, were all recruited and assessed at 19 clinical memory research sites in Spain, Italy, Sweden, and France and led by Araclon Biotech S.L., Zaragoza, Spain. The construction of the five CCs domain scores, and the adjusted hippocampal volume (aHV) on structural MRI, and the standard uptake value ratio (SUVR) on FDG-PET measures, were replicated in the parental AB255 study database.
The study was approved by the CEIC ethic committee 2009/5455, and all participants provided written informed consent prior to inclusion.
Clinical characteristics of the ACE study and the AB255 study
All had the following inclusion criteria: aged from 65 to 85; without lifetime history of psychiatric, neurological, or systemic illnesses; autonomy for instrumental activities of daily living; preserved global cognition (Mini-Mental State Examination, MMSE) [15, 16] (≥24 scores); with general good health; a Hachinski Ischemia Scale [17] score≤4; without depressive symptoms measured by self-rating Geriatric Depression Scale [18] (< 11 scores); at least minimal elementary school; without severe auditory or visual abnormalities including glaucoma and cataracts; and, DNA sample available. Those subjects with significant vascular pathology on MRI that could explain memory deficits, and/or with contraindications for neuroimaging administration, were excluded from the study.
Diagnostic adjudication
The HC subjects had a normal clinical history for their age, and no neurological signs or symptoms. They did not report problems with memory or other cognitive functions, and their performance on the MMSE [15, 16], and measures of memory function were normal. The Clinical Dementia Rating (CDR) [19] was 0, and none had a family history of dementia.
The MCI patients fulfilled Petersen’s diagnostic criteria [20], including subjective memory complaint, relatively preserved performance in activities of daily living, absence of dementia, and a measurable impairment in memory function, with or without a deficit in other cognitive domains [21]. They did not have significant clinical comorbidities (i.e., cerebrovascular disease, history of head trauma encephalopathy, infectious diseases, or developmental disabilities) that could themselves cause cognitive deficits [12, 13]. The memory loss was characterized as being one of impaired storage [14] because both verbal delayed recall and recognition memory were impaired. The CDR score was 0.5, with a 0.5 or 1 score for memory; the Interview for Deterioration in Daily Activities in Dementia score was less than 40 [22]. Those subjects who converted to dementia, that is, to AD [5, 23], and mixed dementia (AD with cerebrovascular disease) over the study period, were classified as MCI converters. All of them had a CDR [19] of 1. In contrast, those subjects who remained stable during follow-ups were classified as stable or non-MCI converters.
Neuropsychological assessment
All subjects underwent a neuropsychological battery for diagnostic purposes, including evaluation of i) global cognition using the MMSE [15, 16], once adjusted by age and educational level; ii) verbal learning and memory by The Word List Learning test from the Wechsler Memory Scale-Third Edition (WMS-III) [24], including delayed recall, and a recognition task) without list of interference [25, 26], and the Free and Cued Selective Reminding Test (FCSRT) [27] adjusted by age and educational level; and, iii) The Vocabulary test of the Wechsler Adult Intelligence Scale-Third Edition (WAIS-III) [28], as a previous cultural level estimation tool.
Comparison of demographic, genetic, and neuropsychological data of these subjects are detailed in Table 1. There were no statistically significant differences between HC and MCI subjects in education or gender, but they did differ in age (Cohen’s d = 1.00, p = 0.001) (Table 1). The MCI patients had a higher frequency of APOE ɛ4 allele carriers (presence of at least one ɛ4 allele) compared to the HC subjects (χ2 = 22.26, p < 0.001, Odds Ratio = 20.42). The MCI patients had significantly lower scores in the Global Cognition, Verbal Memory, and Vocabulary test compared to the HC group (Table 1).
Cognitive composites construction
All subjects underwent a neuropsychological battery with tests in five cognitive domains: 1) Memory: Rey’s Auditory-Verbal Learning Test (RAVLT) [29] and Alzheimer’s Disease Assessment Scale-Cognitive Subscale (ADAS-Cog) [30] (only immediate recall on memory condition); 2) Delayed Recall: both verbal RAVLT [29] and ADAS-Cog [30] also incorporating non-verbal (Rey-Osterrieth Complex Figure Test, ROCF) [29]; 3) Processing Speed, Attention, and Executive Functions: Digit Symbol coding and Digit spans forwards and backwards [29], Trail Making Test (part B-part A) [29]; Semantic Verbal Fluency (“animals” during one minute) [29], and Phonetic Verbal Fluency (words beginning with “P” during one minute) [29]; 4) Language: Boston Naming Test [29] and Commands item from the ADAS-Cog [30]; and 5) Praxis: the ROCF copy condition [29] and Block Design from WAIS-III [29].
In order to create the CCs domain scores, the data were first analyzed by a principal component analysis (PCA). Five separate PCAs were performed, one for each of the following cognitive domains: 1) learning, 2) delayed recall on memory, 3) executive functions, 4) language, and 5) praxis. Every PCA was forced to produce a unidimensional factorial solution, according to the expected unidimensional neuropsychological function assessed. The original variables contributed to the final score in a weighted way, based on the magnitude of the inter-correlations among the variables in the same composite domain. The stability of the PCA was evaluated by means of the Hotelling’s T2 test. When a neuropsychological variable obtained a factorial loading <0.3 in the one-dimension solution, the variable was excluded from the analysis [31], assuming that this variable has a poor empirical contribution to the corresponding inferred cognitive function. According this criterion, Digit Symbol coding, Digit spans forwards, and backwards subtests of WAIS-III [29], and Commands from ADAS-Cog [30], were each excluded from the corresponding PCA analyses. The linear function of the original variables from the factorial solution, was used as a final standardized domain score, for each subject, which we identified as a composite in this study. Each cognitive domain corresponded to a CC domain score that could be later analyzed using standardprocedures.
Neuroimaging acquisition and analysis
All subjects from the ACE study underwent a structural MRI, FDG-PET, and PiB-PET within 30 days following the neurological and neuropsychological visits. All subjects from the AB255 study underwent a structural MRI and FDG-PET. Imaging data were analyzed using the Fundació ACE Pipeline for Neuroimaging Analysis, available at http://detritus.fundacioace.com/.
MRI
All MRI scans were performed with a 1.5T MR scanner (Magnetom Symphony; Siemens Medical Solutions, Erlangen, Germany). The protocol for the acquisition of the MRI data was identical for all subjects and consisted of 3D T1-weighted Sagital MP-RAGE, 2D Axial T2-weighted TSE, 2D Axial Fluid-Attenuated Inversion Recovery (FLAIR), 2D Axial T2* Gradient Echo and 2D Axial Diffusion Weighted Imaging. Brain images were also visually inspected by experienced clinicians who were blinded to the participants’ demographic, anthropometric, and clinical data. All MRI were acquired before the PET and an expert neuroradiologist excluded any form of vascular pathology over participants. Subjects with MRI evidence of major stroke, white matter hyperintensities, leukoaraiosis, and lacunae were excluded.
Cortical reconstruction and volumetric segmentation was performed with the Freesurfer 5.3 image analysis suite, which is documented and freely available for download online (http://surfer.nmr.mgh.Harvard.edu/). The technical details of these procedures are also described in prior publications [32, 33]. Freesurfer morphometric procedures have been proven to show good test-retest reliability across scanner manufacturers and across field strengths [34, 35]. The procedures for the measurement of cortical thickness have been validated against histological analysis [34] and manual measurements [33, 36].
A residual approach was used to adjust hippocampal volume by total intracranial volume (ICV) [37]. The aHV was obtained with the following formula: aHV = HV-b (ICV-<ICV>), where HV is the mean value between right and left HV, <ICV>reflects the group mean ICV value and b is the regression slope between ICV and HV.
FDG-PET
The FDG-PET were acquired 60 min after intravenous injection of approximately 370 MBq of [18F]-FDG during 20 min. The imaging processing and calculation of mean value for the defined ROI were performed using the imaging processing suite FSL, free available online at http://fsl.fmrib.ox.ac.uk/fsl/fslwiki/. Every individual scan was averaged and corrected. Then, the resultant images were coregistered to Montreal National Institute (MNI) standard space and mean value of FDG-PET activity calculated for a composite region-of-interest (ROI). This composite ROI was built with a set of five ROIs (left and right angular gyri, bilateral posterior cingulate gyrus, and left middle and inferior temporal gyrus) based on coordinates cited frequently in other FDG studies comparing AD, MCI, and normal subjects in the ADNI (Alzheimer’s Disease Neuroimaging Initiative) database and available in http://adni.loni.usc.edu/methods/research-tools/. The SUVR was calculated normalizing by the value of vermis/pons as described in Landauet al. [38].
Notice that volumetric and FDG-PET analysis carried on our data were made using the same methods that ADNI site recommend. Information about these analyses are available in ADNI website (http://adni.loni.usc.edu/methods/).
PIB-PET from the ACE study
All these individuals also received a PIB-PET scan. The PIB-PET was acquired 50 min after intravenous injection of 400 MBq of the radiotracer. Every subject MRI was previously segmented using Freesurfer. A cortical composite ROI was built with the labels exported by Freesurfer segmentation on Desikan-Killiany Atlas. The composite ROI included four large cortical grey matter regions (frontal, anterior/posterior cingulate, lateral parietal, lateral temporal). The SUVR was calculated using the cerebellum as reference region [39, 40].
Apolipoprotein E (APOE) genotyping
APOE genotyping was performed for all subjects as previously described by Hixson et al. [41] using the amplification of genomic DNA, digestion with HhaI, and further analysis of the restriction fragments.
Alzheimer’s disease neuroimaging initiative data
Additional data used in the preparation of this study were obtained from the ADNI database (http://adni.loni.usc.edu). The ADNI was launched in 2003 as a public-private partnership, with the primary goal to assess relationships between serial MRI, PET, other biological markers, and clinical and neuropsychological assessment in the progression of MCI and early AD.
The ADNI repository was explored for MCI patients with PIB-PET images (n = 65) [42, 43], and who were similar in age, gender, and educational levels to the ACE study and in parental dataset the AB255 study. From those with MCI, 54 subjects (34 PIB positive and 16 PIB negative) were labeled as “MCI due to AD” with memory storage impairment. Moreover, those MCI with structural MRI (n = 477) and with FDG-PET (n = 336) were also downloaded from the ADNI repository for a later comparison of results obtained in this study.
The subtests used for the construction of the ADNI CC domain score were the delayed recall on memory scores from ADAS-Cog scale and RAVLT. ADNI PIB neuroimaging analysis was completed at University of Pittsburgh, PET Facility. The methods used in this analysis are described at the ADNI website (http://adni.loni.usc.edu/methods/pet-analysis).
An automated template-based method was used to sample multiple ROIs on the ADNI PIB SUVR image. The PIB SUVR was downloaded from the ADNI website along with its corresponding ADNI Processed 3 MR image. The MR image choice was scanner dependent. The PIB SUVR image has been co-registered to the first frame of the raw image file and averaged across frames (for dynamic acquisitions only), reoriented to Talairach space, intensity normalized so that the average of voxels within the mask was exactly 1, and smoothed to achieve a uniform isotropic resolution of 8 mm FWHM. A set of four ROIs were averaged to calculate the PiB SUVR in the frontal cortex, anterior cingulate, parietal cortex and precuneus. All the values were normalized to the cerebellum uptake value. A description of the other variables used is detailed in Supplementary Table 1. Notice that the procedures to calculate the PIB SUVR by the University of Pittsburgh at ADNI database and along this study are completely different. So the data could not be merged but any comparison must be done between the final results.
Statistical analysis
Partial correlations, adjusted by age, gender, and educational level, were carried out among every CC domain score and the three neuroimaging biomarkers, the aHV on MRI, FDG-PET SUVR, and PIB-PET SUVR. This procedure was performed for both the whole sample (the MCI and the HC groups) and the MCI group alone. The PIB-PET SUVR was compared to the aHV and FDG-PET SUVR in order to contrast amyloid-β (Aβ) burden within the MCI group, related to the significant CCs domain scores. Further, a Kaplan-Meier survival analysis was executed in order to estimate survival times in MCI from the ACE study. The aHV on MRI and FDG-PET results from the AB255 study and PIB-PET results from ACE study were replicated using data from the ADNI website (http://adni.loni.usc.edu) and specifically, R ADNIMERGE package (http://adni.bitbucket.org). Cox proportional hazards with adjustments for age, gender, and education were also completed from ADNI data.
RESULTS
As can be seen in Supplementary Table 2, the correlations between the three neuroimaging biomarkers and the five CCs domain scores are strong for the whole sample from the ACE study and the AB255 study.
This was expected since MCI and HC groups are quite different for biomarkers and neuropsychological CCs domains scores.
Within the ACE MCI study, there was only a single significant partial correlation between PIB-PET SUVR and the Delayed Recall CC domain score (β= –0.61, p = 0.003) (Table 2C).
In our replication MCI sample from parental dataset AB255, there was a significant partial correlation between the aHV and the Delayed Recall CC domain score (β= 0.27, p = 0.01) (Table 2A), and between the FDG-PET SUVR and Delayed Recall CC domain score (β= 0.27, p = 0.01) (Table 2B).
MCI subjects from the ACE study demonstrated an Aβ burden related to the Delayed Recall CC domain score as established by aHV on MRI (Fig. 1) and FDG-PET (Fig. 2). They were classified into aHV+ (n = 17) and aHV- (n = 3) with a threshold of aHV = 3.2 cm3, and into FDG+ (n = 18) or FDG- (n = 2) with a threshold of FDG-PET SUVR = 1.3. Those thresholds were the biomarker values that better separated the HC and MCI groups for each case. The Aβ burden cut-off was taken as PIB-PET SUVR = 1.5, for 12 PIB+ and 8 PIB- subjects. The Delayed Recall CC domain score, naturally divided the sample into positive and negative subjects by its mean value of zero. Indeed, this is the threshold that best separated HC and MCI groups.
After a median survival time of 20.6 months (SD: 15.5; range: 6–68), 17/20 (85%) of the ACE MCI patients developed dementia; 15 (88.2%) with AD and 2 (11.8%) with a Mixed Dementia (AD with cerebrovascular disease) (Figs. 1 and 2). Over the course of the observation, one of the MCI patients died and was censored for the analysis of dementia incidence. Among this group, Kaplan-Meier analyses showed that the Delayed Recall CC was the best domain score to predict conversion to dementia compared to the other CCs (see Supplementary Table 3 and Supplementary Figure 1 for details); however, this only showed a tendency to approach significance (Wald = 3.49; p = 0.06; Odds Ratio = 7.40) (0.91–58.82 [95% CI]).
Finally, the correlation between the five CCs domain scores and the three biomarkers was replicated using the ADNI dataset (Table 3). The partial correlations between the Delayed Recall CC domain score and aHV on MRI (Table 3A), FDG-PET (Table 3B), and PIB (Table 3C) were the most significant in MCI from the ADNI dataset.
After a median survival time of 6.5 months (SD: 5.4; range: 6 months-4years), 26/54 (48%) of the ADNI MCI patients developed dementia, specifically AD.
Within MCI with PIB-PET, Cox proportional hazards with adjustments for age, gender, and education showed that the Delayed Recall CC significantly predicted conversion to AD dementia (Wald = 11.32; p = 0.001; Odds Ratio = 2.51) (1.46 – 4.29 [95% CI]) (see Supplementary Figure 2 for details).
DISCUSSION
This work explored the relationship between five CCs domain scores, and three AD neuroimaging biomarkers covering neurodegeneration and Aβ deposition, within probable MCI [12, 13] subjects with memory storage impairment [9]. As previously reported [9], the probable MCI condition had higher risk of early conversion to dementia, mainly AD than the rest of MCI individuals. Remarkably, thosePr-aMCI subjects with storage memory impairment had the most and closest risk of conversion to dementia, mainly AD compared to the other MCI subtypes.
Of note, since the aim of our study was include only the Pr-aMCI-storage subtype subjects, we reported as a unique condition that they must all have a memory loss characterized as being one of impaired storage, that is, independently if they had single or multiple domains impaired. However, all MCI had deficits in multiple cognitive domains. Our data supports that the traditional amnestic single-domain aMCI (aMCI-sd) [20] is rarely diagnosed when a comprehensive neuropsychological battery is applied, because other cognitive deficits are frequently found when neuropsychological evaluation is expanded [44].
One of our central goals was to extend the limited literature of CCs domain scores combined with neuroimaging biomarkers in this population. In particular, focus on the relation with Aβ burden, discussed in more detail later. Then we analyzed the correlation between these two magnitudes. We found that only poorer performances on Delayed Recall CC domain score are related to reduced hippocampal volume, greater hypometabolism, and also greater Aβ burden. Other CCs domain scores, such as executive functions, language, and praxis, were not found to be related to neuroimaging biomarkers used. Our results are according to previous studies that reported that poorer Delayed Recall was related to reduced hippocampal volume as well as greater hypometabolism in MCI patients who were near to AD dementia conversion [45–51].
However, our main finding is that the Aβ burden of the MCI subjects, related to the Delayed Recall CC domain score performances, did not allow classifying all MCI subjects as Prodromal AD in either aHV on MRI or FDG-PET. Instead, some of them had high and other low Aβ burden. Importantly, that as mentioned above, we included subjects in the worst case situation in order to distinguish it, understanding that the MCI sample, all Pr-aMCI-storage subtype, has a high homogeneity phenotype, and besides this, contains some members with closely AD-like cognitive and biomarker pattern who are likely to convert in the near future. Furthermore, observed APOE ɛ4 allele enrichment in the ACE study (70.0%), closely resembled histopathological series reported in AD [52]. In fact, almost all of MCI converted to dementia, mainly developed clinical AD.
As a fact, while all subjects were positive at baseline for at least one neuroimaging biomarker, only 11 (55%) subjects were positive for the three biomarkers at the same time. In contrast, 17 (85%) subjects were positive for aHV, 18 (90%) were positive for FDG-PET, and 12 (60%) were positive for PIB-PET. Also, 15 (75%) subjects were positive for aHV and FDG-PET, 11 (55%) were positive for aHV and PIB-PET, and 11 (55%) were positive for FDG-PET and PIB-PET. Regarding the Delayed Recall CC domain score, all subjects had a negative value.
Hence, Pr-aMCI-storage subjects with higher Aβ burden could be considered canonical prodromal AD (represented in the right bottom corner of both figures). All of the subjects with both greater hippocampal atrophy and hypometabolism could find representation in the biomarker model of pure AD [53–56]. In contrast, it is likely that MCI subjects with lower Aβ burden behave like SNAP (suspected non-Alzheimer’s pathology) subjects, i.e., those individuals with neuroimaging/biomarker evidence of AD-like neurodegeneration without clinical amyloidosis [57] (represented in the left bottom corner of both figures). All of these subjects could find representation on the neurodegeneration-first biomarker model of late-onset AD [53–56]. Note that one subject might be showing low performances on the Delayed Recall CC domain score, even before showing high Aβ burden. In addition, other subjects with high Aβ burden do not show enough hippocampal atrophy or hypometabolism to be classified as prodromal AD (top right corner of both figures). All of them could find representation in the amyloid-first biomarker model of late-onset AD [53–56].
Nevertheless, two of our MCI subjects could not find representation in any of the three Jack’s models [53–56] (left top corner of both figures). Note that although both developed AD dementia, were in the boundaries to be considered with high Aβ burden based on aHV on MRI, and on FDG-PET SUVR.
For a big fraction of our MCI subjects, clinical and cognitive features that are: the “probable” clinical condition, the memory storage impairment, and the impaired Delayed Recall CC domain score precedes in this study amyloid and neurodegeneration biomarkers. This finding was unexpected given the fact that previous studies with MCI reported that the core biomarker pattern provided clinical evidence of the AD model in patients with MCI [58, 59]. The selection of this MCI population in clinical trials with the poorer performances on the Delayed Recall CC domain score could avoid substantial heterogeneity in biomarkers previously reported within amnestic MCI who were clinically similar [60].
Our main finding of this specific clinical-cognitive phenotype MCI observed before amyloidosis is the first reported in the literature. That is, a big fraction of our MCI subjects that converted to clinical AD dementia have not found representation in line with the current pathophysiologic model of AD; even two of our MCI subjects have not found it in any of the three different sets of biomarker models Jack and colleagues proposed [53–56].
After a median survival time of 20.6 months (SD: 15.5; range: 6–68), 17/20 (85%) of the ACE MCI patients developed dementia; 15 (88.2%) AD type; and 2 (11.8%) mixed dementia (AD with cerebrovascular disease). This points out the importance of follow-up in this MCI group. However, further studies including a more extensive longitudinal clinical follow-up and anatomopathological data would be needed to corroborate these results.
With regard to ADNI, the procedures for the calculation of PIB SUVR and the Delayed Recall CC domain score slightly differed between samples. Also, the composite scores calculated on both datasets are not exactly the same. The corresponding CC domain score for the Delayed Recall differed on the visual memory by the ROCF test from the ADNI and the ACE and the AB255 studies, but both reflects the performance on the same cognitive domain. So although data cannot be merged across datasets, any comparison should be done between the statistical results. In addition, with our ADNI replication, we confirm that the Delayed Recall is the optimal CC domain score to look for prodromal AD, when compared to the other CC domains. Other CCs such as Learning and Executive functions domain scores were found related to hippocampal volume and FDG-PET, previously associated in MCI and AD patients with increasing disease severity [61].
One of the limitations of this study is firstly the lack of amyloid PET in parental dataset AB255; thus, the sample size of subjects with amyloid PET in ACE study was small. This fact could explain that the Delayed Recall CC domain score only showed a tendency to approached significance toward superiority with respect to the other CCs domain scores, as a predictor of conversion to dementia. However, in our replication from ADNI, the Delayed Recall was the best CC domain score as a predictor of conversion to AD. Secondly, we were not able to measure sensitivity and specificity values because of the small sample sizes. Our results are limited to those Pr-aMCI-storage subtype subjects and therefore, are not applicable or extendable to the majority of MCI cases. Further studies incorporating a more heterogeneous MCI group phenotype, i.e., including the possible/probable and amnestic/non-amnestic MCI subtypes [9] could be considered.
In conclusion, according to our results, we strongly suggest selecting those subjects within Pr-aMCI-storage subtype with the worst performances on Delayed Recall CC domain score in order to maximize cost-benefits of clinical trial recruitment.
ACKNOWLEDGMENTS
We thank the patients and control subjects who participated in this project. We are indebted to Trinitat Port-Carbó and her family, who support Fundació ACE Memory Clinic research programs. This work was funded by Araclon Biotech, Recercalia and Fundació ACE Memory Clinic, as well as the Spanish Ministry of Health through Instituto de Salud Carlos III (Madrid) (FISS PI10/00954) and by Agència d’Avaluació de Tecnologia i Recerca Mèdiques, Departament de Salut de la Generalitat de Catalunya (grant 390/06/2009). JTB was supported in part by funds from the National Institute of Aging (P50-AG005133).
Data collection and sharing for this project was funded by the Alzheimer’s Disease Neuroimaging Initiative (ADNI) (National Institutes of Health Grant U01AG024904) and DOD ADNI (Department of Defense award number W81XWH-12-2-0012). ADNI is funded by the National Institute on Aging, the National Institute of Biomedical Imaging and Bioengineering, and through generous contributions from the following: AbbVie, Alzheimer’s Association; Alzheimer’s Drug Discovery Foundation; Araclon Biotech; BioClinica, Inc.; Biogen; Bristol-Myers Squibb Company; CereSpir, Inc.; Cogstate; Eisai Inc.; Elan Pharmaceuticals, Inc.; Eli Lilly and Company; EuroImmun; F. Hoffmann-La Roche Ltd and its affiliated company Genentech, Inc.; Fujirebio; GE Healthcare; IXICO Ltd.; Janssen Alzheimer Immunotherapy Research & Development, LLC.; Johnson & Johnson Pharmaceutical Research & Development LLC.; Lumosity; Lundbeck; Merck & Co., Inc.; Meso Scale Diagnostics, LLC.; NeuroRx Research; Neurotrack Technologies; Novartis Pharmaceuticals Corporation; Pfizer Inc.; Piramal Imaging; Servier; Takeda Pharmaceutical Company; and Transition Therapeutics. The Canadian Institutes of Health Research is providing funds to support ADNI clinical sites in Canada. Private sector contributions are facilitated by the Foundation for the National Institutes of Health (http://www.fnih.org). The grantee organization is the Northern California Institute for Research and Education, and the study is coordinated by the Alzheimer’s Therapeutic Research Institute at the University of Southern California. ADNI data are disseminated by the Laboratory for Neuro Imaging at the University of Southern California.
The present study has been performed as part of the doctoral programme of Ana Espinosa at the University of Barcelona.
Authors’ disclosures available online (http://j-alz.com/manuscript-disclosures/16-1223r1).
Notes
[1] †Co-investigators from the AB255 Study Group:
Principal Investigator: Mercè Boada, MD, PhD (Fundació ACE, Institut Català de Neurociències Aplicades, Alzheimer Research Center and Memory Clinic, Barcelona, Spain); Collaborator Investigator: Lluís Tárraga, MSc (Fundació ACE, Institut Català de Neurociències Aplicades, Alzheimer Research Center and Memory Clinic, Barcelona, Spain); Coordinator: Manuel Sarasa, MD, PhD (Araclon Biotech SL, Zaragoza, Spain); Collaborators: Miguel Goñi, MD (Hospital DivinoValles, Burgos, Spain), Francesc Pujadas, MD (Hospital Universitari Vall d’Hebrón, Barcelona, Spain); Alberto Villarejo, MD (Hospital Doce de Octubre, Madrid, Spain); Ana Frank, MD, PhD (Hospital La Paz, Madrid, Spain); Jordi Peña-Casanova, MD, PhD (Hospital del Mar, Barcelona, Spain); Pablo Martínez-Lage, MD, PhD (Centro CITA Alzheimer, San Sebastián, Spain); Manuel Fernández, MD, PhD (CAE Oroitu Algorta, Vizcaya, Spain); Gerard Piñol, MD, PhD (Hospital Santa Maria de Lleida, Lleida, Spain); Rafael Blesa, MD, PhD (Hospital de la Santa Creu i Sant Pau, Barcelona, Spain); Pedro Gil, MD, PhD (Hospital Clínico San Carlos, Madrid, Spain); Luis F. Pascual, MD (Hospital Lozano Blesa, Zaragoza, Spain); Miquel Aguilar, MD (Hospital Universitari Mútua Terrassa, Terrassa, Spain); Giovanni B Frisoni, MD (IRCCS Centro San Giovanni di Dio FBF, Brescia, Italy); Jorge Matias-Guiu, MD, PhD (Hospital Clínico San Carlos, Madrid, Spain); Niels Andreasen, MD, PhD (Karolinska Institutet, Stockholm, Sweden); Carmen Antúnez, MD (Hospital Virgen de la Arrixaca, Fundación Alzheimur, Murcia, Spain); Bruno Vellas, MD, PhD (Hôpital CHU La Grave, Casselardit, Toulouse, France); Touchon Jacques, MD, PhD (Hôpital Gui de Chauliac, CHU, Montpellier, France); Neuroimaging coordinators: Josep Munnuera, MD, PhD (Hospital Universitari Germans Trias i Pujol, Unitat RM Badalona, Institut de diagnòstic per la imatge, Badalona, Spain) and Javier Arbizu, MD, PhD (Clínica Universitaria de Pamplona, Pamplona, Spain).
Appendices
The supplementary material is available in the electronic version of this article: http://dx.doi.org/10.3233/JAD-161223
REFERENCES
[1] | Dubois B , Feldman HH , Jacova C , Cummings JL , DeKosky ST , Barberger-Gateau P , Delacourte A , Frisoni G , Fox NC , Galasko D , Gauthier S , Hampel H , Jicha GA , Meguro K , O’Brien J , Pasquier F , Robert P , Rossor M , Salloway S , Sarazin M , de Souza LC , Stern Y , Visser PJ , Scheltens P ((2010) ) Revising the definition of Alzheimer’s disease: A new lexicon. Lancet Neurol 9: , 1118–1127. |
[2] | Dubois B , Feldman HH , Jacova C , Hampel H , Molinuevo JL , Blennow K , DeKosky ST , Gauthier S , Selkoe D , Bateman R , Cappa S , Crutch S , Engelborghs S , Frisoni GB , Fox NC , Galasko D , Habert M-O , Jicha GA , Nordberg A , Pasquier F , Rabinovici G , Robert P , Rowe C , Salloway S , Sarazin M , Epelbaum S , de Souza LC , Vellas B , Visser PJ , Schneider L , Stern Y , Scheltens P , Cummings JL ((2014) ) Advancing research diagnostic criteria for Alzheimer’s disease: The IWG-2 criteria. Lancet Neurol 13: , 614–629. |
[3] | Albert MS , DeKosky ST , Dickson D , Dubois B , Feldman HH , Fox NC , Gamst A , Holtzman DM , Jagust WJ , Petersen RC , Snyder PJ , Carrillo MC , Thies B , Phelps CH ((2011) ) The diagnosis of mild cognitive impairment due to Alzheimer’s disease: Recommendations from the National Institute on Aging-Alzheimer’s Association workgroups on diagnostic guidelines for Alzheimer’s disease. Alzheimers Dement 7: , 270–279. |
[4] | Jack CR Jr , Albert MS , Knopman DS , McKhann GM , Sperling RA , Carrillo MC , Thies B , Phelps CH ((2011) ) Introduction to the recommendations from the National Institute on Aging-Alzheimer’s Association workgroups on diagnostic guidelines for Alzheimer’s disease. Alzheimers Dement 7: , 257–262. |
[5] | McKhann GM , Knopman DS , Chertkow H , Hyman BT , Jack CR , Kawas CH , Klunk WE , Koroshetz WJ , Manly JJ , Mayeux R , Mohs RC , Morris JC , Rossor MN , Scheltens P , Carrillo MC , Thies B , Weintraub S , Phelps CH ((2011) ) The diagnosis of dementia due to Alzheimer’s disease: Recommendations from the National Institute on Aging-Alzheimer’s Association workgroups on diagnostic guidelines for Alzheimer’s disease. Alzheimers Dement 7: , 263–269. |
[6] | Sperling RA , Aisen PS , Beckett LA , Bennett DA , Craft S , Fagan AM , Iwatsubo T , Jack CR Jr , Kaye J , Montine TJ , Park DC , Reiman EM , Rowe CC , Siemers E , Stern Y , Yaffe K , Carrillo MC , Thies B , Morrison-Bogorad M , Wagster MV , Phelps CH ((2011) ) Toward defining the preclinical stages of Alzheimer’s disease: Recommendations from the National Institute on Aging and the Alzheimer’s Association workgroup. Alzheimers Dement 7: , 280–292. |
[7] | Vos SJB , Verhey F , Frölich L , Kornhuber J , Wiltfang J , Maier W , Peters O , Rüther E , Nobili F , Morbelli S , Frisoni GB , Drzezga A , Didic M , van Berckel BNM , Simmons A , Soininen H , Kłoszewska I , Mecocci P , Tsolaki M , Vellas B , Lovestone S , Muscio C , Herukka S-K , Salmon E , Bastin C , Wallin A , Nordlund A , de Mendonça A , Silva D , Santana I , Lemos R , Engelborghs S , Van der Mussele S , Freund-Levi Y , Wallin ÅK , Hampel H , van der Flier W , Scheltens P , Visser PJ ((2015) ) Prevalence and prognosis of Alzheimer’s disease at the mild cognitive impairment stage. Brain 138: , 1327–1338. |
[8] | Gomar JJ , Bobes-Bascaran MT , Conejero-Goldberg C , Davies P , Goldberg TE , Alzheimer’s Disease Neuroimaging, Initiative ((2011) ) Utility of combinations of biomarkers, cognitive markers, and risk factors to predict conversion from mild cognitive impairment to Alzheimer disease in patients in the Alzheimer’s disease neuroimaging initiative. Arch Gen Psychiatry 68: , 961–969. |
[9] | Espinosa A , Alegret M , Valero S , Vinyes-Junqué G , Hernández I , Mauleón A , Rosende-Roca M , Ruiz A , López O , Tárraga L , Boada M ((2013) ) A longitudinal follow-up of 550 mild cognitive impairment patients: Evidence for large conversion to dementia rates and detection of major risk factors involved. J Alzheimers Dis 34: , 769–780. |
[10] | Boada M , Tárraga L , Hernández I , Valero S , Alegret M , Ruiz A , Lopez OL , Becker JT ((2014) ) Design of a comprehensive Alzheimer’s disease clinic and research center in Spain to meet critical patient and family needs. Alzheimers Dement 10: , 409–415. |
[11] | Petersen RC ((2004) ) Mild cognitive impairment as a diagnostic entity. J Intern Med 256: , 183–194. |
[12] | Lopez OL , Jagust WJ , DeKosky ST , Becker JT , Fitzpatrick A , Dulberg C , Breitner J , Lyketsos C , Jones B , Kawas C , Carlson M , Kuller LH ((2003) ) Prevalence and classification of mild cognitive impairment in the Cardiovascular Health Study Cognition Study: Part 1. Arch Neurol 60: , 1385–1389. |
[13] | Lopez OL , Kuller LH , Becker JT , Dulberg C , Sweet RA , Gach HM , Dekosky ST ((2007) ) Incidence of dementia in mild cognitive impairment in the cardiovascular health study cognition study. Arch Neurol 64: , 416–420. |
[14] | Delis DC , Massman PJ , Butters N , Salmon DP , Cermak LS , Kramer JH ((1991) ) Profiles of demented and amnesic patients on the California Verbal Learning Test: Implications for the assessment of memory disorders. Psychol Assess 3: , 19–26. |
[15] | Folstein MF , Folstein SE , McHugh PR ((1975) ) Mini-mental state. A practical method for grading the cognitive state of patients for the clinician. J Psychiatr Res 12: , 189–198. |
[16] | Blesa R , Pujol M , Aguilar M , Santacruz P , Bertran-Serra I , Hernández G , Sol JM , Peña-Casanova J , NORMACODEM Group ((2001) ) Clinical validity of the “mini-mental state” for Spanish speaking communities. Neuropsychologia 39: , 1150–1157. |
[17] | Hachinski VC , Lassen NA , Marshall J ((1974) ) Multi-infarct dementia. A cause of mental deterioration in the elderly. Lancet 2: , 207–210. |
[18] | Sheikh JI , Yesavage JA ((1986) ) Geriatric depression scale (GDS): Recent findings and development of a shorter version. In Clinical Gerontology: A Guide to Assessment and Intervention. The Haworth Press, Inc, NY, pp. 165–173. |
[19] | Morris JC ((1993) ) The Clinical Dementia Rating (CDR): Current version and scoring rules. Neurology 43: , 2412–2414. |
[20] | Petersen RC , Smith GE , Waring SC , Ivnik RJ , Tangalos EG , Kokmen E ((1999) ) Mild cognitive impairment: Clinical characterization and outcome. Arch Neurol 56: , 303–308. |
[21] | Petersen RC ((2004) ) Mild cognitive impairment as a diagnostic entity. J Intern Med 256: , 183–194. |
[22] | Teunisse S , Derix MM , van Crevel H ((1991) ) Assessing the severity of dementia. Patient and caregiver. Arch Neurol 48: , 274–277. |
[23] | McKhann G , Drachman D , Folstein M , Katzman R , Price D , Stadlan EM ((1984) ) Clinical diagnosis of Alzheimer’s disease: Report of the NINCDS-ADRDA Work Group under the auspices of Department of Health and Human Services Task Force on Alzheimer’s Disease. Neurology 34: , 939–944. |
[24] | Wechsler D ((1997) ) WMS–III. Wechsler Memory Scale–Third Edition. Administration and scoring manual. The Psychological Corporation, San Antonio, TX. |
[25] | Alegret M , Espinosa A , Vinyes-Junqué G , Valero S , Hernández I , Tárraga L , Becker JT , Boada M ((2012) ) Normative data of a brief neuropsychological battery for Spanish individuals older than 49. J Clin Exp Neuropsychol 34: , 209–219. |
[26] | Alegret M , Espinosa A , Valero S , Vinyes-Junqué G , Ruiz A , Hernández I , Rosende-Roca M , Mauleón A , Becker JT , Tárraga L , Boada M ((2013) ) Cut-off scores of a Brief Neuropsychological Battery (NBACE) for Spanish individual adults older than 44 years old. PLoS One 8: , e:76436. |
[27] | Buschke H ((1984) ) Cued recall in amnesia. J Clin Neuropsychol 6: , 433–440. |
[28] | Wechsler D ((1997) ) WAIS–III. Wechsler Adult Intelligence Scale–Third Edition. Technical manual. The Psychological Corporation, San Antonio, TX. |
[29] | Lezak MD ((2004) ) Neuropsychological Assessment, 4th ed, Oxford University Press, New York. |
[30] | Rosen WG , Mohs RC , Davis KL ((1984) ) A new rating scale for Alzheimer’s disease. Am J Psychiatry 141: , 1356–1364. |
[31] | Kline P ((1994) ) An easy guide to factor analysis, Routledge, London. |
[32] | Salat DH , Buckner RL , Snyder AZ , Greve DN , Desikan RSR , Busa E , Morris JC , Dale AM , Fischl B ((2004) ) Thinning of the cerebral cortex in aging. Cereb Cortex 14: , 721–730. |
[33] | Han X , Jovicich J , Salat D , van der Kouwe A , Quinn B , Czanner S , Busa E , Pacheco J , Albert M , Killiany R , Maguire P , Rosas D , Makris N , Dale A , Dickerson B , Fischl B ((2006) ) Reliability of MRI-derived measurements of human cerebral cortical thickness: The effects of field strength, scanner upgrade and manufacturer. Neuroimage 32: , 180–194. |
[34] | Rosas HD , Liu AK , Hersch S , Glessner M , Ferrante RJ , Salat DH , van der Kouwe A , Jenkins BG , Dale AM , Fischl B ((2002) ) Regional and progressive thinning of the cortical ribbon in Huntington’s disease. Neurology 58: , 695–701. |
[35] | Kuperberg GR , Broome MR , McGuire PK , David AS , Eddy M , Ozawa F , Goff D , West WC , Williams SCR , van der Kouwe AJW , Salat DH , Dale AM , Fischl B ((2003) ) Regionally localized thinning of the cerebral cortex in schizophrenia. Arch Gen Psychiatry 60: , 878–888. |
[36] | Reuter M , Schmansky NJ , Rosas HD , Fischl B ((2012) ) Within-subject template estimation for unbiased longitudinal image analysis. Neuroimage 61: , 1402–1418. |
[37] | Mathalon DH , Sullivan EV , Rawles JM , Pfefferbaum A ((1993) ) Correction for head size in brain-imaging measurements. Psychiatry Res 50: , 121–139. |
[38] | Landau SM , Harvey D , Madison CM , Koeppe RA , Reiman EM , Foster NL , Weiner MW , Jagust WJ , Alzheimer’s Disease Neuroimaging Initiative ((2011) ) Associations between cognitive, functional, and FDG-PET measures of decline in AD and MCI. Neurobiol Aging 32: , 1207–1218. |
[39] | Mormino EC , Kluth JT , Madison CM , Rabinovici GD , Baker SL , Miller BL , Koeppe RA , Mathis CA , Weiner MW , Jagust WJ , Alzheimer’s Disease Neuroimaging Initiative ((2009) ) Episodic memory loss is related to hippocampal-mediated β-amyloid deposition in elderly subjects. Brain 132: , 1310–1323. |
[40] | Jagust WJ1 , Landau SM , Shaw LM , Trojanowski JQ , Koeppe RA , Reiman EM , Foster NL , Petersen RC , Weiner MW , Price JC , Mathis CA , Alzheimer’s Disease Neuroimaging Initiative ((2009) ) Relationships between biomarkers in aging and dementia. Neurology 73: , 1193–1199. |
[41] | Hixson JE , Vernier DT ((1990) ) Restriction isotyping of human apolipoprotein E by gene amplification and cleavage with HhaI. J Lipid Res 31: , 545–548. |
[42] | Jagust WJ , Bandy D , Chen K , Foster NL , Landau SM , Mathis CA , Price JC , Reiman EM , Skovronsky D , Koeppe RA , Alzheimer’s Disease Neuroimaging Initiative ((2010) ) The Alzheimer’s Disease Neuroimaging Initiative positron emission tomography core. Alzheimers Dement 6: , 221–229. |
[43] | Swaminathan S , Shen L , Risacher SL , Yoder KK , West JD , Kim S , Nho K , Foroud T , Inlow M , Potkin SG , Huentelman MJ , Craig DW , Jagust WJ , Koeppe RA , Mathis CA , Jack CR , Weiner MW , Saykin AJ , Alzheimer’s Disease Neuroimaging Initiative ((2012) ) Amyloid pathway-based candidate gene analysis of [11C]PiB-PET in the Alzheimer’s Disease Neuroimaging Initiative (ADNI) cohort. Brain Imaging Behav 6: , 1–15. |
[44] | Nordlund A , Rolstad S , Hellström P , Sjögren M , Hansen S , Wallin A ((2005) ) The Goteborg MCI study: Mild cognitive impairment is a heterogeneous condition. J Neurol Neurosurg Psychiatry 76: , 1485–1490. |
[45] | Wolk DA , Dickerson BC ((2011) ) Fractionating verbal episodic memory in Alzheimer’s disease. Neuroimage 54: , 1530–1539. |
[46] | Chang Y-L , Bondi MW , Fennema-Notestine C , McEvoy LK , Hagler DJ , Jacobson MW , Dale AM ((2010) ) Brain substrates of learning and retention in mild cognitive impairment diagnosis and progression to Alzheimer’s disease. Neuropsychologia 48: , 1237–1247. |
[47] | Risacher S , Saykin A , Wes J , Shen L , Firpi H , McDonald B , Alzheimer’s Disease Neuroimaging Initiative (ADNI) ((2009) ) Baseline MRI predictors of conversion from MCI to probable AD in the ADNI Cohort. Curr Alzheimer Res 6: , 347–361. |
[48] | McEvoy LK , Fennema-Notestine C , Roddey JC , Hagler DJ , Holland D , Karow DS , Pung CJ , Brewer JB , Dale AM , Alzheimer’s Disease Neuroimaging Initiative ((2009) ) Alzheimer disease: Quantitative structural neuroimaging for detection and prediction of clinical and structural changes in mild cognitive impairment. Radiology 251: , 195–205. |
[49] | Misra C , Fan Y , Davatzikos C ((2009) ) Baseline and longitudinal patterns of brain atrophy in MCI patients, and their use in prediction of short-term conversion to AD: Results from ADNI. Neuroimage 44: , 1415–1422. |
[50] | Habeck C , Risacher S , Lee GJ , Glymour MM , Mormino E , Mukherjee S , Kim S , Nho K , DeCarli C , Saykin AJ , Crane PK , Alzheimer’s Disease Neuroimaging Initiative ((2012) ) Relationship between baseline brain metabolism measured using [18F]FDG PET and memory and executive function in prodromal and early Alzheimer’s disease. Brain Imaging Behav 6: , 568–583. |
[51] | Crane PK , Carle A , Gibbons LE , Insel P , Mackin RS , Gross A , Jones RN , Mukherjee S , Curtis SM , Harvey D , Weiner M , Mungas D , Alzheimer’s Disease Neuroimaging Initiative ((2012) ) Development and assessment of a composite score for memory in the Alzheimer’s Disease Neuroimaging Initiative (ADNI). Brain Imaging Behav 6: , 502–516. |
[52] | Nielsen AS , Ravid R , Kamphorst W , Jørgensen OS ((2003) ) Apolipoprotein E epsilon 4 in an autopsy series of various dementing disorders. J Alzheimers Dis 5: , 119–125. |
[53] | Jack CR , Knopman DS , Jagust WJ , Petersen RC , Weiner MW , Aisen PS , Shaw LM , Vemuri P , Wiste HJ , Weigand SD , Lesnick TG , Pankratz VS , Donohue MC , Trojanowski JQ ((2013) ) Tracking pathophysiological processes in Alzheimer’s disease: An updated hypothetical model of dynamic biomarkers. Lancet Neurol 12: , 207–216. |
[54] | Jack CR , Wiste HJ , Lesnick TG , Weigand SD , Knopman DS , Vemuri P , Pankratz VS , Senjem ML , Gunter JL , Mielke MM , Lowe VJ , Boeve BF , Petersen RC ((2013) ) Brain β-amyloid load approaches a plateau. Neurology 80: , 890–896. |
[55] | Jack CR , Wiste HJ , Weigand SD , Knopman DS , Lowe V , Vemuri P , Mielke MM , Jones DT , Senjem ML , Gunter JL , Gregg BE , Pankratz VS , Petersen RC ((2013) ) Amyloid-first and neurodegeneration-first profiles characterize incident amyloid PET positivity. Neurology 81: , 1732–1740. |
[56] | Jack CR , Holtzman DM ((2013) ) Biomarker modeling of Alzheimer’s disease. Neuron 80: , 1347–1358. |
[57] | Jack CR ((2014) ) PART and SNAP. Acta Neuropathol 128: , 773–776. |
[58] | Wolk DA , Price JC , Saxton JA , Snitz BE , James JA , Lopez OL , Aizenstein HJ , Cohen AD , Weissfeld LA , Mathis CA , Klunk WE , De-Kosky ST ((2009) ) Amyloid imaging in mild cognitive impairment subtypes. Ann Neurol 65: , 557–568. |
[59] | Prestia A , Caroli A , Van Der Flier WM , Ossenkoppele R , Van Berckel B , Barkhof F , Teunissen CE , Wall AE , Carter SF , Schöll M , Choo IH , Nordberg A , Scheltens P , Frisoni GB ((2013) ) Prediction of dementia in MCI patients based on core diagnostic markers for Alzheimer disease. Neurology 80: , 1048–1056. |
[60] | Nettiksimmons J , DeCarli C , Landau S , Beckett L , Alzheimer’s Disease Neuroimaging Initiative ((2014) ) Biological heterogeneity in ADNI amnestic mild cognitive impairment. Alzheimers Dement 10: , 511–521.e1. |
[61] | Weiner MW , Veitch DP , Aisen PS , Beckett LA , Cairns NJ , Green RC , Harvey D , Jack CR , Jagust W , Liu E , Morris JC , Petersen RC , Saykin AJ , Schmidt ME , Shaw L , Shen L , Siuciak JA , Soares H , Toga AW , Trojanowski JQ , Alzheimer’s Disease Neuroimaging Initiative ((2013) ) The Alzheimer’s Disease Neuroimaging Initiative: A review of papers published since its inception. Alzheimers Dement 9: , e111–e194. |
Figures and Tables
Fig.1
PIB SUVR related to the Delayed Recall CC established by aHV in the MCI group. aThe MCI subjects with the best performances on the Delayed Recall CC domain score are shown with circles, bthose with intermediate performances are represented with triangles, cwhile those with the worst performances are shown with squares. dAfter a median survival time of 20.6 months (SD: 15.5; range: 6–68), 17/20 (85%) of the MCI patients developed dementia; 15 (88.2%) with AD; and 2 (11.8%) with a mixed dementia (AD with cerebrovascular disease).
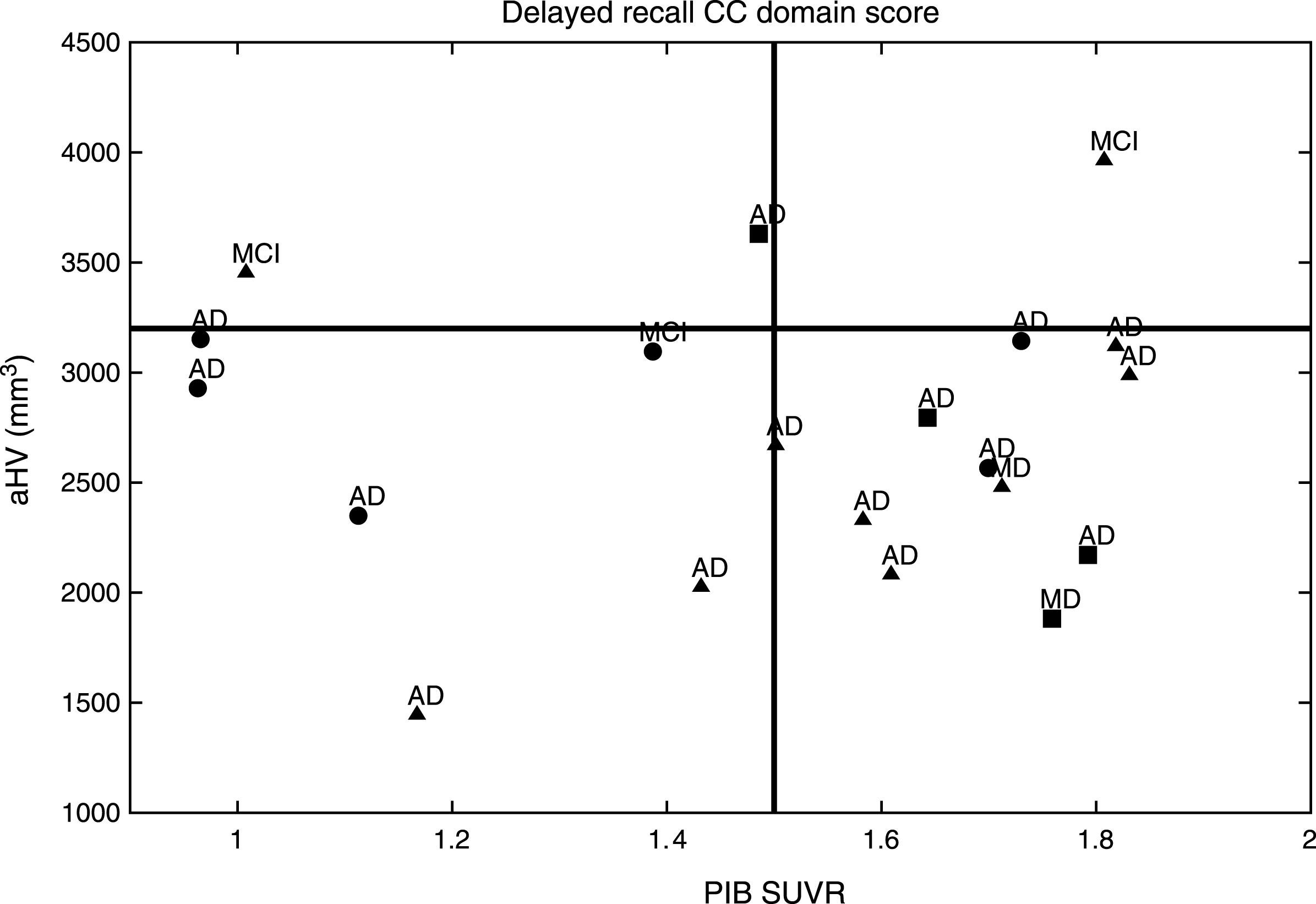
Fig.2
PIB SUVR related to the Delayed Recall CC established by FDG in the MCI group. aThe MCI subjects with the best performances on the Delayed Recall CC domain score are shown with circles, bthose with intermediate performances are represented with triangles, cwhile those with the worst performances are shown with squares. dAfter a median survival time of 20.6 months (SD: 15.5; range: 6–68), 17/20 (85%) of the MCI patients developed dementia; 15 (88.2%) with AD; and 2 (11.8%) with a mixed dementia (AD with cerebrovascular disease).
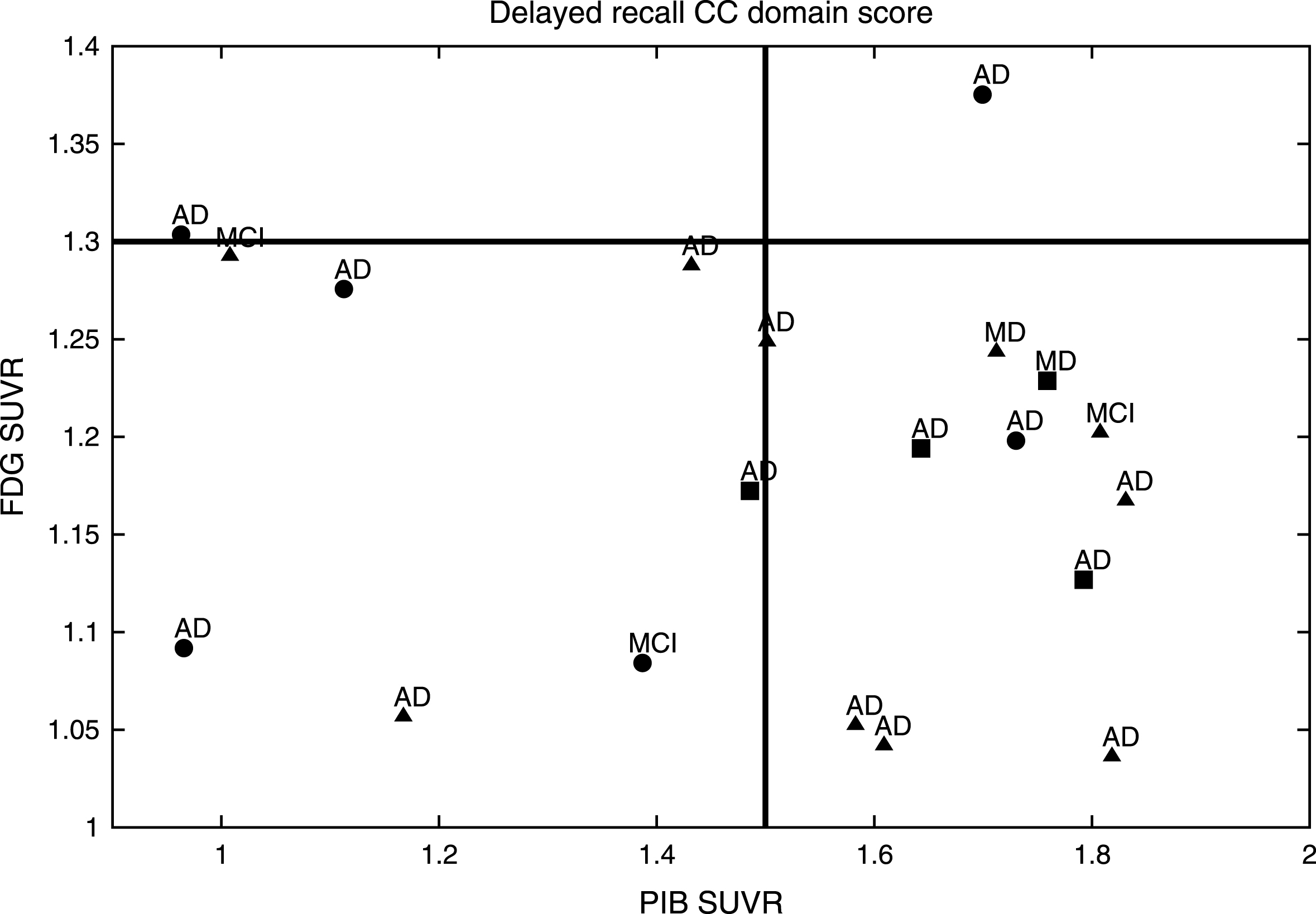
Table 1
Demographic, genetic and neuropsychological data between HC and MCI subjects from the ACE study and the AB255 study
HC | MCI | Statistics | p | Effect size3 | |
N (%) | 39 (66.1) | 20 (33.9) | |||
42 (24.0) | 133 (76.0) | ||||
Gender n (%) Male | 20 (51.3) | 12 (60.0) | 0.591 | 0.360 | 0.70 |
21 (50.0) | 64 (48.1) | 0.041 | 0.832 | 1.08 | |
Education in years | 12.3±4.1 | 10.6±4.1 | 2.012 | 0.161 | 0.42 |
12.2±3.9 | 15.4±22.9 | 0.812 | 0.370 | 0.24 | |
Age in years | 71.3±4.4 | 75.7±4.4 | 13.152 | 0.001** | 1.00 |
71.0±4.5 | 74.0±5.1 | 11.892 | 0.001** | 0.63 | |
APOE 4 n (%) | 4 (10.3) | 14 (70.0) | 22.261 | 0.001** | 20.42 |
(presence of ɛ4 or ɛ4/ɛ4) | 4 (9.5) | 73 (54.9) | 26.661 | 0.001** | 11.56 |
Neuropsychological battery | |||||
Global Cognition | |||||
MMSE | 29.7±0.6 | 26.0±1.9 | 43.092 | 0.001** | 2.96 |
29.7±0.6 | 26.2±2.2 | 104.092 | 0.001** | 2.50 | |
Verbal Memory | |||||
Verbal Learning and Memory WMS-III | |||||
Learning (Trials 1+2+3+4) | 30.0±5.2 | 16.0±4.9 | 32.792 | 0.001** | 2.77 |
29.6±5.1 | 16.6±4.8 | 227.902 | 0.001** | 2.63 | |
Delayed Recall | 6.3±2.8 | 0.3±0.7 | 33.85 2 | 0.001** | 3.43 |
6.1±2.5 | 1.2±1.7 | 210.04 2 | 0.001** | 2.33 | |
Recognition memory | 22.3±1.8 | 14.6±2.7 | 53.842 | 0.001** | 3.42 |
22.1±1.8 | 15.3±2.1 | 350.732 | 0.001** | 3.49 | |
Buschke Selective Reminding Test | |||||
Free recall (Trials 1+2+3) | 23.7±6.7 | 3.1±3.0 | 58.802 | 0.001** | 4.25 |
23.7±6.7 | 6.1±4.7 | 359.542 | 0.001** | 3.09 | |
Cued Recall (Trials 1+2+3) | 18.9±5.2 | 10.6±6.2 | 12.112 | 0.001** | 1.46 |
18.5±5.0 | 11.9±6.1 | 40.902 | 0.001** | 1.19 | |
Free + Cued Recall | 42.5±4.4 | 13.8±7.9 | 107.152 | 0.001** | 4.67 |
42.7±4.4 | 17.9±8.9 | 289.762 | 0.001** | 3.73 | |
Vocabulary test (WAIS-III) | 13.9±2.1 | 12.4±2.4 | 4.112 | 0.011* | 0.88 |
(Scalar Scores) | 13.8±2.1 | 11.8±2.5 | 32.582 | 0.001** | 1.05 |
Values in regular print correspond to ACE study and values in bold correspond to AB255 study; HC, healthy controls; MCI, amnestic mild cognitive impairment-storage type; MMSE, Mini-Mental State Examination; WMS-III, Wechsler Memory Scale, Third Edition; Recognition memory, correct answers WMS-III; WAIS-III, Wechsler Adult Intelligence Scale-Third Edition. Values reported are means:±; SD, standard deviation; 1: χ2; 2: F; 3: Cohen’s d for continuous variables, and Odds ratio for categorical data are reported. *Statistically significant p≤0.01; **p≤0.001.
Table 2
Partial correlations between neuroimaging biomarkers and the five CCs domain scores in MCI subjects from the ACE study (n = 20) and the AB255 study (n = 133)
CCs related to: | (A) aHV | (B) FDG-PET | (C) PIB-PET | |||
β | p-value | β | p-value | β | p-value | |
Learning CC | 0.09 | 0.74 | 0.06 | 0.82 | – 0.02 | 0.92 |
0.13 | 0.12 | 0.05 | 0.59 | NA | NA | |
Delayed Recall CC | – 0.02 | 0.94 | 0.06 | 0.82 | – 0.61 | 0.003* |
0.27 | 0.01* | 0.27 | 0.01* | NA | NA | |
Executive Functions CC | 0.29 | 0.24 | 0.23 | 0.37 | – 0.19 | 0.45 |
0.01 | 0.92 | 0.21 | 0.03 | NA | NA | |
Language CC | 0.23 | 0.37 | – 0.04 | 0.87 | 0.21 | 0.40 |
0.06 | 0.51 | 0.04 | 0.65 | NA | NA | |
Praxis CC | 0.19 | 0.46 | – 0.01 | 0.97 | – 0.07 | 0.79 |
– 0.08 | 0.33 | 0.13 | 0.14 | NA | NA |
Values in regular print correspond to ACE study and values in bold correspond to AB255 study; CC, Cognitive Composite domain score; aHV, adjusted hippocampal volume; β, partial correlations adjusted by age, gender, and education; NA, not applicable; *Statistically significant after Bonferroni’s correction p≤0.01.
Table 3
Partial correlations between neuroimaging biomarkers and the five CCs domain scores in MCI subjects from the ADNI dataset
CCs related to: | (A) aHV | (B) FDG-PET | (C) PIB-PET | |||
β | p-value | β | p-value | β | p-value | |
Learning CC | 0.35 (n = 477) | 2.95 e–15** | 0.23 (n = 336) | 1.51 e–05** | – 0.39 (n = 54) | 0.004 |
Delayed Recall CC | 0.50 (n = 477) | 1.56 e–31** | 0.30 (n = 336) | 2.17 e–08** | – 0.42 (n = 54) | 0.002* |
Executive Functions CC | 0.18 (n = 479) | 5.98 e–05** | 0.29 (n = 334) | 4.88 e–08** | – 0.35 (n = 54) | 0.01 |
Language CC | 0.14 (n = 478) | 0.002* | 0.14 (n = 337) | 0.01 | – 0.19 (n = 54) | 0.19 |
Praxis CC | NA | NA | NA | NA | NA | NA |
CC, Cognitive Composite domain score; aHV, adjusted hippocampal volume; β, partial correlations adjusted by age, gender, and education; *Statistically significant after Bonferroni’s correction p≤0.01; **p≤0.001; NA, not applicable on ADNI.