Association Between Kidney Disease Index and Decline in Cognitive Function with Mediation by Arterial Stiffness in Asians with Type 2 Diabetes
Abstract
Background:
Decline in renal function impairs systemic clearance of amyloid-β which characterizes Alzheimer’s disease while albuminuria is associated with blood-brain barrier disruption due to endothelial damage. Arterial stiffness adversely affects the brain with high pulsatile flow damaging cerebral micro-vessels.
Objective:
To examine association between a novel kidney disease index (KDI), which is a composite index of estimated glomerular filtration (eGFR) and urinary albumin-to-creatinine ratio (uACR), and cognitive function with potential mediation by arterial stiffness.
Methods:
This was a longitudinal multi-center study of participants with type 2 diabetes (T2D) aged 45 years and above. We assessed cognitive function with Repeatable Battery for the Assessment of Neuropsychological Status (RBANS). Pulse wave velocity (PWV), an index of arterial stiffness, was measured using applanation tonometry method. KDI was calculated as geometric mean of 1/eGFR and natural logarithmically-transformed (ln)(ACR*100).
Results:
There were 1,303 participants with mean age 61.3±8.0 years. LnKDI was associated with lower baseline RBANS total score with adjusted coefficient –2.83 (95% CI –4.30 to –1.35; p < 0.001). 590 participants were followed over up to 8.6 years. LnKDI was associated with lower follow-up RBANS score in total, immediate memory, visuo-spatial/construction and attention domains with corresponding adjusted coefficients –2.35 (95% CI –4.50 to –0.20; p = 0.032), –2.93 (95% CI –5.84 to –0.02; p = 0.049), –3.26 (95% CI –6.25 to –0.27; p = 0.033) and –4.88 (95% CI –7.95 to –1.82; p = 0.002). PWV accounted for 19.5% of association between and follow-up RBANS total score.
Conclusions:
KDI was associated with lower cognitive function globally, and in immediate memory, visuo-spatial/construction and attention domains. Arterial stiffness mediated the association between KDI and cognitive decline in patients with T2D.
INTRODUCTION
According to the World Health Organization, there are currently more than 55 million people living with dementia globally.1 Ten million new cases of dementia are reported each year.1 Dementia is also the seventh leading cause of mortality and is a major contributor to disability in older people worldwide.1 Hence there is a strong impetus to understand and prevent the development of dementia.
According to a systematic review by Biessels and Despa (2018), individuals with diabetes had relative risks of 1.53 to 2.27 of developing dementia.2 Moreover, there was a high prevalence (45%) of mild cognitive impairment in patients with type 2 diabetes (T2D).3 Another emerging risk factor of dementia is chronic kidney disease (CKD)3 which is characterized by estimated glomerular filtration (eGFR) less than 60 ml/min/1.73 m2 or urinary albumin-to-creatinine ratio (uACR) more than 30 mg/g.4 Interestingly, the brain and kidney have common hemodynamic characteristics as both are low-resistance end-organs with exposure to high blood flow and have rich vasculature.5 The brain tissues are penetrated by branches from anterior, middle and posterior cerebral arteries.5 Similarly, the kidneys have juxtamedullary afferent arterioles. These vessels depend on their autoregulation to respond to hemodynamic strain generated by large supplying arteries.5 It was also suggested that T2D leads to accumulation of advanced glycation end-products, chronic inflammation and oxidative stress which are substrates for development of CKD6 and cognitive impairment.7 Hence it is plausible that both kidneys and brain have shared pathophysiological pathways in patients with T2D.
While there were studies which investigated the relationship between renal function and cognitive function, the results were not consistent.8 Some studies found a positive association between worse kidney function and cognitive impairment, while others observed a null effect.8 The difference may be attributed to different measures of renal function being used.8 Some of the studies used categorical measures of GFR or uACR while other studies used continuous renal function as exposures of interest.8 A systematic review reported a positive association between worse categorical GFR and cerebral small vessel disease (cSVD) with relative risk 1.77 (95% Confidence Interval (CI) 1.40–2.24) in a pooled analysis of four studies. However, pooled analyses on studies using eGFR and uACR as separate continuous variables showed no evidence of association.8 The joint effect of low renal function and albuminuria on cognitive function is also unknown.
In recent years, there is an emerging interest in combining eGFR and uACR into a novel kidney disease index (KDI) which was calculated as a geometric mean of 1/eGFR and natural logarithmically transformed (ln) (100*uACR).9 The geometric mean is an approach which combines information from different dimensions of an underlying condition into a composite measure.10 Unlike arithmetic mean which can only combine measurements from the same scale, geometric mean can combine eGFR and uACR which come from different scales.10 The details of the calculation are covered under materials and methods. In a study by Gerstein and colleagues (2022), higher values of KDI were shown to be linearly associated with major cardiovascular events, kidney outcomes and mortality in patients with T2D.9 In contrast, there was a non-linear association between 1/eGFR and the outcomes in the same study.9 The findings suggested that KDI could aid in identifying high-risk individuals who might benefit from preventive strategies.9 These results provided a timely opportunity for us to examine the association between KDI and cognitive function in patients with T2D. KDI as a composite measure can better reflect two aspects of CKD: 1) abnormality of kidney function in terms of low eGFR; and 2) kidney damage as indicated by albuminuria.4 The dual aspects of CKD were taken into account in CKD definition and classification by 2012 Kidney Disease: Improving Global Outcomes (KDIGO) guidelines and classification.4
Besides the epidemiological link between KDI and cognitive decline in T2D, there is a strong impetus to understand the underlying pathophysiological mechanism. Of note, arterial stiffness, a hallmark of vascular aging, adversely affects the brain with high pulsatile flow damaging cerebral micro-vessels.11 The study by Novak N and Colleagues (2023) also demonstrated that arteriosclerosis markers such as N-terminal pro-B-type natriuretic peptide (NT-proBNP) and Growth Differentiation Factor 15 (GDF15) mediated the association between kidney function and cognitive decline in community-dwelling individuals.12 Hence it is plausible that arterial stiffness, which is also a marker of arteriosclerosis,13 mediates the relationship between KDI and decline in cognitive function.
In this study, we aimed to examine the association between KDI and follow-up cognitive function over time, and the role of arterial stiffness as a potential mediator in the association in patients with T2D.
MATERIALS AND METHODS
Study population
This was a prospective cohort study of patients aged 45 years and above from the Singapore Study of Macro-angiopathy and Micro-vascular Reactivity in Type 2 Diabetes (SMART2D). The patients were recruited when they attended follow-up for T2D in the Diabetes Centre of a public hospital or primary-care polyclinics in the Northern region of Singapore. The following exclusion criteria were applied: active inflammation (e.g., systemic lupus erythematosus and overt untreated cancer), intake of non-steroidal anti-inflammatory medications on the same day of assessment, intake of oral steroids with amount equivalent to more than 7.5 mg of prednisolone, history of dementia based on case notes, and inability to give written informed consent. All the participants provided written informed consent. Ethics approval for the study was granted by the National Healthcare Group Domain Specific Review Board in Singapore. The study conforms to the ethical guidelines of the 1975 Declaration of Helsinki.
Data collection
Information on demographics, educational level and medical history was obtained from questionnaires administered to patients. We assessed for depressive symptoms with a 15-item Geriatric Depression Scale (GDS). Depressive symptoms were present if the score was 5 or more.14
Trained research coordinators measured blood pressure using a standard blood pressure monitor (HEM-C7011-C1, OMRON Corp., Kyoto, Japan) for the patients after a resting period of 10 minutes or more. Body mass index (BMI) was obtained from weight and height measured with standard weighing scale and stadiometer respectively. Skeletal muscle mass (SMM) was quantitated using Bio-impedance analysis (BIA) which involved attaching electrodes in a tetrapolar configuration with the patient lying supine followed by passing a low alternating current at 1, 5, 50, 250, 500, and 1000 kHz through the electrodes.15
Carotid-femoral pulse wave velocity (PWV) is the gold standard measure of arterial stiffness16 and was measured using SphygmoCor® (AtCor Medical, Sydney, Australia) in this study. The instrument utilizes foot-to-foot method to measure carotid-femoral PWV.17 This involves using an applanation tonometer to detect the pulse waveform at the carotid and femoral arteries, followed by recording of the mean transit period for the waves between carotid and femoral arteries.17 We calculated PWV as the ratio of the distance between the recording sites in meters divided by transit time in seconds.17 The instrument is well-validated with good reproducibility in various populations including those with diabetes.17
Fasting blood and urine samples were collected and sent to the hospital laboratory accredited by the College of the American Pathologists for the following measurements: fasting plasma glucose (FPG) using Hexokinase method, Hemoglobin A1c (HbA1c) using Tina-quant Hemoglobin A1c Gen.3, urinary albumin using immunoturbidimetric assay, and low-density lipoprotein-cholesterol (LDL-C), triglycerides (TG) and serum creatinine using enzymatic colorimetric test (COBAS-Roche, Mannheim, Germany). We detected the presence of Apolipoprotein E (APOE) ɛ4 allele using Illumina OmniExpress 24 array.
We collected data on eGFR, uACR and cognitive function at baseline between September 2014 and January 2019 and during one follow-up about three years later till April 2022. We used the latest available RBANS score in the follow-up.
We assessed cognitive function with the Repeatable Battery for the Assessment of Neuropsychological Status (RBANS).18 The tool has undergone translation for use in older subjects with validated norms in Singapore.19 There are 12 subsets of cognitive function, namely list learning, story memory, figure copy, line orientation, picture naming, semantic fluency, digit span, coding, list recall, list recognition, story recall and figure recall.18 They are grouped into five cognitive domains – immediate memory, delayed memory, visuo-spatial/construction, language and attention.18
We calculated eGFR based on the Chronic Kidney Disease Epidemiology Collaboration (CKD-EPI) equation.20 As eGFR and uACR have opposite relationships to CKD, we used the reciprocal of eGFR to reflect worse renal function before calculating the geometric mean.10 The skewed distribution of uACR necessitated the natural logarithmical transformation of this variable.9 We also multiplied uACR by 100 in order to avoid ln-transformed values of zero or negative.10 KDI was constructed by calculating the geometric mean of 1/eGFR and ln(uACR*100).9
Statistical analysis
Data was expressed as number with percentages for categorical variables, and mean±standard deviation (SD) or median with interquartile range (IQR) for continuous variables. We used linear regression to examine the cross-sectional association between the markers of kidney disease and RBANS score at baseline. Model 1 was adjusted for age, gender and ethnicity. Model 2 was additionally adjusted for education < 7 years, presence of APOE ɛ4 allele, GDS score, HbA1c, FPG, LDL-C, lnTG, systolic blood pressure (SBP), BMI and SMM. These covariates were included in the model in view of biological plausibility or p-value less than 0.1 in the univariate analysis.
Linear mixed model was used to examine the longitudinal association between the markers of kidney disease and follow-up RBANS score. The model consists of fixed covariates except for different cognitive function at baseline (random intercept). Model 1 was adjusted for follow-up period. Model 2 was additionally adjusted for age, gender and ethnicity. Model 3 included the variables in Model 2 and education <7 years, presence of APOE ɛ4 allele, GDS score, HbA1c, FPG, LDL-C, lnTG, SBP, BMI and SMM. Both KDI and 1/eGFR were natural logarithmically transformed as lnKDI and ln(1/eGFR) so as to ensure comparability with ln(uACR*100) (i.e., all exposures in natural logarithmic scales) in linear mixed model analysis. There was no evidence of multi-collinearity between the individual covariates, including HbA1c and FPG, as variance inflation factor (VIF) for each covariate was lessthan 5.
Linear mixed model stratified by progression of kidney disease was also performed, adjusting for the same covariates. The patients were deemed to have KDI progression if follow-up KDI was more than baseline KDI. eGFR progression was defined as deterioration across the eGFR categories coupled with drop of eGFR from baseline by more than 25%.4 uACR progression was defined as worsening of albuminuria from normoalbuminuria (uACR < 30 mg/g) to microalbuminuria (uACR 30–300 mg/g), normoalbuminuria to macroalbuminuria (uACR > 300 mg/g) or normoalbuminuria to macroalbminuria.21
Mediation analysis was done to test for mediation of the association between KDI and follow-up RBANS total score by arterial stiffness. Based on the framework by Baron and Kenny,22 the following criteria for mediation were required: 1) significant association between independent variable (KDI) and mediating variable (PWV) (pathway a); 2) significant association between mediating variable (PWV) and dependent variable (follow-up RBANS total score) (pathway b); 3) significant association between independent variable (KDI) and dependent variable (follow-up RBANS total score) (pathway c); and 4) weakening of the association between independent variable (KDI) and dependent variable (follow-up RBANS total score) when adjusted for the mediating variable (PWV) (pathway c’). We used generalized structural equation model (GSEM) for the mediation analysis. Mediation analysis was also performed to test if SBP, HbA1c, LDL, and TG mediated the association between KDI and follow-up RBANS total score. Statistical analysis was conducted with STATA version 14.0 (STATA Corporation, College Station, Texas). Results were considered statistically significant for p-values less than 0.05.
RESULTS
The baseline characteristics of patients were shown in Table 1. The mean age of the patients was 61.3±8.0 years. There was a slight male predominance at 51.0%. The distribution of patients by ethnicity was as follows: Chinese, 54.1%; Malay, 19.1%; Indian, 22.8%; and Other 3.9%. The proportion of patients with < 7 years of education was 32.1%. The median values for eGFR, uACR, and KDI were 82.1 ml/min/1.73 m2 (IQR 60.4–99.2), 26.5 mg/g (IQR 7.7–123.7) and 0.30 (0.26–0.37) respectively. The proportion of CKD stage 3 and above (i.e., eGFR < 60 ml/min/1.73 m2) was 24.6%.
Table 1
Baseline characteristics of patients (N = 1,303)
Variable | Mean±SD or N (%) |
Age (y) | 61.3±8.0 |
Male (%) | 664 (51.0) |
Ethnicity (%) | |
Chinese | 704 (54.1) |
Malay | 249 (19.1) |
Indian | 297 (22.8) |
Other | 51 (3.9) |
<7 y education (%) | 418 (32.1) |
GDS score≥5 | 50 (3.9) |
DM duration (y) | 15.0±9.0 |
BMI (kg/m2) | 27.2±4.9 |
SMM (kg) | 24.3±7.3 |
SBP (mmHg) | 141.3±18.3 |
HbA1c (%) | 7.9±1.6 |
FPG (mmol/l) | 8.8±3.0 |
LDL-C (mmol/l) | 2.6±0.8 |
TG (mmol/l) | 1.3 (1.0–1.9) |
Presence of APOE ɛ4 allele (%) | 183 (17.2) |
eGFR (ml/min/1.73 m2) | 82.1 (60.4–99.2) |
CKD stage 3 and above (%) | 320 (24.6) |
uACR (mg/g) | 26.5 (7.7–123.7) |
KDI | 0.30 (0.26–0.37) |
PWV (m/s) | 10.3±2.9 |
RBANS score | |
Total | 100.6±8.7 |
Immediate memory | 101.7±11.5 |
Delayed memory | 100.3±11.9 |
Visuo-spatial/ construction | 100.3±11.7 |
Language | 100.3±11.3 |
Attention | 100.3±12.1 |
GDS, Geriatric Depression Scale, DM, diabetes mellitus; BMI, body mass index; SMM, skeletal muscle mass; SBP, systolic blood pressure; HbA1c, hemoglobin A1c; FPG, fasting plasma glucose; LDL-C, low density lipoprotein-cholesterol; TG, triglycerides; eGFR, estimated glomerular filtration rate; CKD, chronic kidney disease; uACR, urinary albumin-to-creatinine ratio; KDI, kidney disease index; PWV, pulse wave velocity; RBANS, Repeatable Battery for the Assessment of NeuropsychologicalStatus.
In Table 2, baseline KDI was positively correlated with age, GDS score, DM duration, BMI, SMM, SBP, HbA1c, FPG, TG, and PWV. It was negatively correlated with baseline RBANS scores for total and all cognitive domains.
Table 2
Spearman correlation between KDI and baseline characteristics
Variable | Correlation coefficient | p |
Age (y) | 0.279 | <0.001 |
GDS score | 0.074 | 0.008 |
DM duration (y) | 0.284 | <0.001 |
BMI (kg/m2) | 0.142 | <0.001 |
SMM (kg) | 0.116 | <0.001 |
SBP (mmHg) | 0.353 | <0.001 |
HbA1c (%) | 0.084 | 0.002 |
FPG (mmol/l) | 0.079 | 0.005 |
LDL-C (mmol/l) | –0.043 | 0.123 |
TG (mmol/l) | 0.274 | <0.001 |
eGFR (ml/min/1.73 m2) | –0.803 | <0.001 |
uACR (mg/g) | 0.828 | <0.001 |
PWV (m/s) | 0.376 | <0.001 |
RBANS score | ||
Total | –0.237 | <0.001 |
Immediate memory | –0.203 | <0.001 |
Delayed memory | –0.211 | <0.001 |
Visuo-spatial/construction | –0.161 | <0.001 |
Language | –0.099 | <0.001 |
Attention | –0.202 | <0.001 |
GDS, Geriatric Depression Scale, DM, diabetes mellitus; BMI, body mass index; SMM, skeletal muscle mass; SBP, systolic blood pressure; HbA1c, hemoglobin A1c; FPG, fasting plasma glucose; LDL-C, low density lipoprotein-cholesterol; TG, triglycerides; eGFR, estimated glomerular filtration rate; uACR, urinary albumin-to-creatinine ratio; KDI, kidney disease index; PWV, pulse wave velocity; RBANS, Repeatable Battery for the Assessment of Neuropsychological Status.
In the unadjusted analysis in Table 3, baseline lnKDI was cross-sectionally associated with lower RBANS total score (indicative of lower cognitive function) with coefficient –5.59 (95% CI –6.97 to –4.20; p < 0.001) at baseline. The association remained in Model 1 and Model 2 with coefficients –3.55 (95% CI –4.86 to –2.24; p < 0.001) and –2.59 (95% CI –4.17 to –1.20; p < 0.001) respectively. Baseline ln(1/eGFR) and ln(ACR*100) were also cross-sectionally associated with lower RBANS total score in the unadjusted analysis with corresponding coefficients –2.81 (95% CI –3.62 to –1.99; p < 0.001) and –0.62 (95% CI –0.85 to –0.40; p < 0.001) at baseline. Their associations remained in Models 1 and 2. In the adjusted Model 2, the coefficients for ln(1/eGFR) and ln(uACR*100) were –1.53 (95% CI –2.48 to –0.59; p = 0.001) and –0.28 (95% CI –0.52 to –0.05; p = 0.019) respectively. Hence the association between lnKDI with RBANS total score appeared stronger than the associations between ln(1/eGFR) and ln(uACR*100) with RBANS total score.
Table 3
Association between per unit increase in marker of kidney disease and RBANS scores at baseline
Coefficient (95% CI) p-value | |||
Unadjusted | Model 1a | Model 2b | |
Total | |||
LnKDI | –5.59 (–6.97 to –4.20) p < 0.001 | –3.55 (–4.86 to –2.24) p < 0.001 | –2.69 (–4.17 to –1.20) p < 0.001 |
Ln(1/eGFR) | –2.81 (–3.62 to –1.99) p < 0.001 | –1.67 (–2.44 to –0.91) p < 0.001 | –1.53 (–2.48 to –0.59) p = 0.001 |
Ln(uACR*100) | –0.62 (–0.85 to –0.40) p < 0.001 | –0.57 (–0.77 to –0.36) p < 0.001 | –0.28 (–0.52 to –0.05) p = 0.019 |
Immediate memory | |||
LnKDI | –6.30 (–8.15 to –4.45) p < 0.001 | –3.61 (–5.46 to –1.76) p < 0.001 | –2.65 (–4.88 to –0.42) p = 0.020 |
Ln(1/eGFR) | –3.10 (–4.19 to –2.00) p < 0.001 | –1.59 (–2.67 to –0.51) p = 0.004 | –1.38 (–2.80 to 0.03) p = 0.055 |
Ln(uACR*100) | –0.76 (–1.06 to –0.46) p < 0.001 | –0.63 (–0.92 to –0.34) p < 0.001 | –0.35 (–0.70 to 0.01) p = 0.056 |
Delayed memory | |||
LnKDI | –6.41 (–8.31 to –4.51) p < 0.001 | –3.25 (–5.14 to –1.37) p = 0.001 | –2.76 (–5.04 to –0.48) p = 0.018 |
Ln(1/eGFR) | –3.09 (–4.22 to –1.97) p < 0.001 | –1.28 (–2.38 to –0.18) p = 0.022 | –1.37 (–2.81 to 0.08) p = 0.064 |
Ln(uACR*100) | –0.74 (–1.05 to –0.44) p < 0.001 | –0.60 (–0.89 to –0.31) p < 0.001 | –0.40 (–0.76 to –0.04) p = 0.031 |
Visuo-spatial/Construction | |||
LnKDI | –6.18 (–8.07 to –4.29) p < 0.001 | –5.00 (–6.88 to –3.11) p < 0.001 | –4.71 (–6.73 to –2.68) p < 0.001 |
Ln(1/eGFR) | –3.33 (–4.44 to –2.22) p < 0.001 | –2.76 (–3.85 to –1.66) p < 0.001 | –3.17 (–4.45 to –1.89) p < 0.001 |
Ln(uACR*100) | –0.66 (–0.96 to –0.35) p < 0.001 | –0.64 (–0.93 to –0.34) p < 0.001 | –0.31 (–0.64 to 0.01) p = 0.061 |
Language | |||
LnKDI | –2.58 (–4.42 to –0.74) p = 0.006 | –1.63 (–3.51 to 0.24) p = 0.088 | –0.58 (–2.81 to 1.65) p = 0.610 |
Ln(1/eGFR) | –1.26 (–2.34 to –0.17) p = 0.023 | –0.64 (–1.73 to 0.45) p = 0.252 | –0.31 (–1.73 to 1.10) p = 0.666 |
Ln(uACR*100) | –0.25 (–0.54 to 0.05) p = 0.101 | –0.30 (–0.59 to –0.01) p = 0.042 | –0.03 (–0.38 to 0.33) p = 0.888 |
Attention | |||
LnKDI | –6.47 (–8.42 to –4.52) p < 0.001 | –4.24 (–6.06 to –2.43) p < 0.001 | –2.74 (–4.89 to –0.59) p = 0.013 |
Ln(1/eGFR) | –3.33 (–4.41 to –2.12) p < 0.001 | –0.64 (–1.73 to 0.45) p = 0.252 | –1.44 (–2.81 to –0.08) p = 0.038 |
Ln(uACR*100) | –0.72 (–1.03 to –0.40) p < 0.001 | –0.66 (–0.94 to –0.57) p < 0.001 | –0.34 (–0.68 to 0.00) p = 0.051 |
RBANS, Repeatable Battery for the Assessment of Neuropsychological Status. aModel 1: age, gender and ethnicity. bModel 2: Model 1 + education<7 years, presence of APOE ɛ4 allele, geriatric depression scale score, hemoglobin A1c, fasting plasma glucose, low-density lipoprotein-cholesterol, natural logarithmically transformed triglyceride, systolic blood pressure, body mass index and skeletal muscle mass.
In Table 3, baseline lnKDI was negatively associated with all the RBANS domain scores in the unadjusted analysis at baseline. In the adjusted Model 2, lnKDI remained significantly associated with lower RBANS score in immediate memory, delayed memory, visuo-spatial/construction and attention domains with adjusted coefficients –2.65 (95% CI –4.88 to –0.42; p = 0.020), –2.76 (95% CI –5.04 to –0.48; p = 0.018), –4.71 (95% CI –6.73 to –2.68; p < 0.001) and –2.74 (95% CI –4.89 to –0.59; p = 0.013) respectively at baseline. Ln(1/eGFR) was associated with lower RBANS scores in visuo-spatial/construction and attention, while ln(uACR*100) was associated with lower RBANS scores in delayed memory in the adjusted Model 2.
There were 590 participants who were followed up over a mean period of 5.0±1.1 years (up to 8.6 years). Table 4 showed that the mean follow-up RBANS total decreased from baseline by 2.04 from 102.8±7.6 to 100.8±8.5 (p < 0.001). Similar findings were noted for all cognitive domains.
Table 4
Baseline and follow-up RBANS scores (N = 590)
Baseline (A) | Follow-up (B) | Difference (A-B) | p | |
Total | 102.8±7.6 | 100.8±8.5 | 2.04 | <0.001 |
Immediate memory | 104.3±10.3 | 102.9±10.4 | 1.32 | <0.001 |
Delayed memory | 102.8±10.4 | 100.1±12.1 | 2.66 | <0.001 |
Visuospatial/ Construction | 101.9±10.2 | 100.7±11.7 | 1.23 | 0.004 |
Language | 102.2±10.6 | 99.8±11.7 | 2.37 | 0.001 |
Attention | 102.8±11.4 | 100.4±11.7 | 2.39 | <0.001 |
RBANS, Repeatable Battery for the Assessment of Neuropsychological Status.
Table 5 showed the longitudinal analysis on the association between the markers of kidney disease and RBANS total score at follow-up. In Model 1 which was adjusted for follow-up period, baseline lnKDI was associated with reduced RBANS total score with coefficient –5.71 (95% CI –7.70 to –3.71; p < 0.001). The negative association between lnKDI and follow-up RBANS total score persisted in Models 2 and 3 with corresponding coefficients –4.27 (95% CI –6.16 to –2.38; p < 0.001) and –2.35 (95% CI –4.50 to –0.20; p = 0.032). Although baseline ln(1/eGFR) and ln(uACR*100) were significantly associated with lower follow-up RBANS total score in Model 1 with coefficients –3.41 (95% CI –4.71 to –2.11; p < 0.001) and –0.47 (95% CI –0.75 to –0.18; p = 0.001) respectively, their associations were attenuated and lost statistical significance in the fully adjusted Model 3.
Table 5
Association between per unit increase in baseline marker of kidney disease and RBANS scores at follow-up
Coefficient (95% CI) p-value | |||
Model 1a | Model 2b | Model 3c | |
Total | |||
LnKDI | –5.71 (–7.70 to –3.71) p < 0.001 | –4.27 (–6.16 to –2.38) p < 0.001 | –2.35 (–4.50 to –0.20) p = 0.032 |
Ln(1/eGFR) | –3.41 (–4.71 to –2.11) p < 0.001 | –1.89 (–3.13 to –0.65) p = 0.003 | –1.25 (–2.69 to 0.19) p = 0.089 |
Ln(uACR*100) | –0.47 (–0.75 to –0.18) p = 0.001 | –0.57 (–0.84 to –0.31) p < 0.001 | –0.27 (––0.58 to 0.04) p = 0.089 |
Immediate memory | |||
LnKDI | –6.96 (–9.47 to –4.45) p < 0.001 | –4.47 (–6.93 to –2.02) p < 0.001 | –2.93 (–5.84 to –0.02) p = 0.049 |
Ln(1/eGFR) | –3.94 (–5.58 to –2.31) p < 0.001 | –1.78 (–3.38 to –0.18) p = 0.030 | –1.63 (–3.58 to 0.32) p = 0.101 |
Ln(uACR*100) | –0.61 (–0.97 to –0.25) p = 0.001 | –0.61 (–0.96 to –0.27) p < 0.001 | –0.32 (–0.74 to 0.10) p = 0.134 |
Delayed memory | |||
LnKDI | –5.51 (–8.28 to –2.74) p < 0.001 | –2.60 (–5.31 to 0.10) p = 0.059 | –0.00 (–3.23 to 3.22) p = 0.999 |
Ln(1/eGFR) | –3.34 (–5.13 to –1.54) p < 0.001 | –0.89 (–2.66 to 0.87) p = 0.320 | 0.03 (–2.12 to 2.19) p = 0.977 |
Ln(uACR*100) | –0.40 (–0.80 to –0.00) p = 0.049 | –0.37 (–0.75 to 0.01) p = 0.053 | 0.03 (–0.44 to 0.49) p = 0.903 |
Visuo-spatial/Construction | |||
LnKDI | –5.94 (–8.54 to –3.34) p < 0.001 | –5.29 (–7.84 to –2.74) p < 0.001 | –3.26 (–6.25 to –0.27) p = 0.033 |
Ln(1/eGFR) | –3.65 (–5.34 to –1.97) p < 0.001 | –2.92 (–4.59 to –1.26) p = 0.001 | –2.39 (–4.39 to –0.39) p = 0.019 |
Ln(uACR*100) | –0.45 (–0.82 to –0.07) p = 0.019 | –0.55 (–0.91 to –0.19) p = 0.003 | –0.16 (–0.60 to 0.27) p = 0.474 |
Language | |||
LnKDI | –2.11 (–4.81 to –0.59) p = 0.126 | –1.41 (–4.14 to 1.32) p = 0.323 | –0.67 (–3.94 to 2.60) p = 0.687 |
Ln(1/eGFR) | –1.00 (–2.75 to 0.75) p = 0.261 | 0.12 (–1.65 to 1.90) p = 0.894 | 0.32 (–1.87 to 2.51) p = 0.775 |
Ln(uACR*100) | –0.18 (–0.57 to 0.20) p = 0.349 | –0.36 (–0.74 to 0.02) p = 0.065 | –0.29 (–0.76 to 0.18) p = 0.231 |
Attention | |||
LnKDI | –8.64 (–11.44 to –5.84) p < 0.001 | –7.30 (–9.92 to –4.68) p < 0.001 | –4.88 (–7.95 to –1.82) p = 0.002 |
Ln(1/eGFR) | –5.36 (–7.18 to –3.53) p < 0.001 | –3.77 (–5.48 to –2.05) p < 0.001 | –2.58 (–4.63 to –0.52) p = 0.014 |
Ln(uACR*100) | –0.76 (–1.16 to –0.36) p < 0.001 | –0.93 (–1.30 to –0.56) p < 0.001 | –0.60 (–1.05 to –0.16) p = 0.007 |
RBANS, Repeatable Battery for the Assessment of Neuropsychological Status. aModel 1: follow-up period. bModel 2: Model 1 + age, gender and ethnicity. cModel 3: Model 2 + education<7 years, presence of APOE ɛ4 allele, geriatric depression scale score, hemoglobin A1c, fasting plasma glucose, low-density lipoprotein-cholesterol natural logarithmically transformed triglyceride, systolic blood pressure, body mass index and skeletal muscle mass.
In Table 5, lnKDI was negatively associated with follow-up RBANS score in immediate memory, delayed memory, visuo-spatial/construction and attention in Model 1 with corresponding coefficients –6.96 (95% CI –9.47 to –4.45; p < 0.001), –5.51 (95% CI –8.28 to –2.74; p < 0.001), –5.94 (95% CI –8.54 to –3.34; p < 0.001) and –8.64 (95% CI –11.44 to –5.84; p < 0.001) in Model 1. In the adjusted Model 3, the negative association between lnKDI with follow-up RBANS score for immediate memory, visuo-spatial/construction and attention domains persisted with coefficients –2.93 (95% CI –5.84 to –0.02; p = 0.049), –3.26 (95% CI –6.25 to –0.27; p = 0.033) and –4.88 (95% CI –7.95 to –1.82; p = 0.002) respectively. The association between lnKDI and immediate memory domain appeared weaker than that between lnKDI and visuospatial/construction domain or attention domain.
Supplementary Table 1 shows the results of the linear mixed model stratified by progression of kidney disease. lnKDI was associated with lower follow-up RBANS total score in patients with KDI progression with coefficients –7.41 (95% CI –9.93 to –4.89; p < 0.001), –5.30 (95% CI –7.71 to –2.89; p < 0.001) and –3.05 (95% CI –5.85 to –0.24; p = 0.034) in Models 1, 2 and 3 respectively. However, there was no evidence of association between lnKDI and follow-up RBANS total score among patients with no KDI progression. In the adjusted Model 2, lnKDI was also associated with lower follow-up RBANS total score in patients with no uACR progression but not in those with uACR progression. There was no evidence of association between lnKDI and follow-up RBANS total score in the analysis stratified by eGFR progression.
Table 5 also showed that ln(1/eGFR) and ln(uACR*100) were negatively associated with follow-up RBANS score in immediate memory, delayed memory, visuo-spatial/construction and attention domains in Model 1. However, ln(1/eGFR) remained associated with lower RBANS score in visuo-spatial/construction and attention domains in the adjusted Model 3 with corresponding coefficients –2.39 (95% CI –4.39 to –0.39; p = 0.019) and –2.58 (95% CI –4.63 to –0.52; p = 0.014). Ln(uACR*100) only remained associated with lower RBANS score in attention domain with coefficient –0.60 (95% CI –1.05 to –0.16; p = 0.007) in the fully adjustedModel 3.
In Fig. 1, mediation analysis revealed that per unit increase in baseline lnKDI was positively associated with baseline PWV with coefficient for pathway a 2.01 (p < 0.001); per unit increase in baseline PWV was negatively associated with follow-up RBANS total score with coefficient for pathway b –0.27 (p = 0.006); per unit increase in baseline lnKDI was negatively associated with follow-up RBANS total score with coefficient for pathway c –2.75 (p = 0.003), and the association between baseline lnKDI and follow-up RBANS total score was attenuated upon addition of baseline PWV to the model with coefficient for pathway c’ –2.21 (p = 0.019). Baseline PWV accounted for 19.5% of the association between baseline lnKDI and follow-up RBANS total score (p = 0.010). There was no evidence of mediation by SBP, HbA1c, LDL and TG in the association between lnKDI and follow-up RBANS total score in Supplementary Figures 1–4.
Fig. 1
Mediation of baseline PWV between lnKDI and RBANS total score at follow-up Adjusted for age, gender, ethnicity, education < 7 years, hemoglobin A1c, fasting plasma glucose, low-density lipoprotein-cholesterol, natural logarithmically transformed triglyceride, body mass index, and skeletal musclemass.
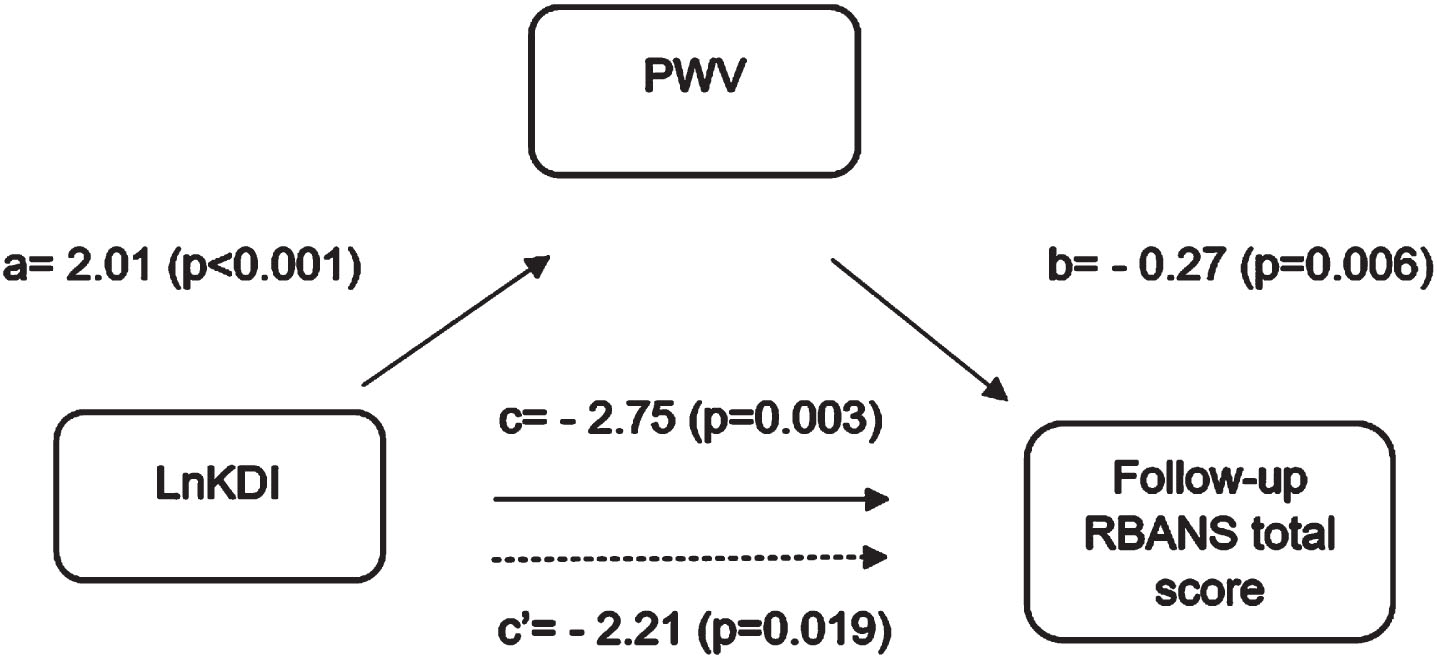
DISCUSSION
Our study demonstrated that baseline KDI, a composite index of eGFR and uACR, was independently associated with lower RBANS score globally and in the domains of immediate memory, delayed memory, visuo-spatial/construction and attention at baseline. In the longitudinal analysis, baseline KDI was also independently associated with lower follow-up RBANS score globally and in the domains of immediate memory, visuo-spatial/construction and attention. On the other hand, the reciprocal of eGFR and uACR were independently associated with RBANS total score at baseline but not at follow-up. Baseline PWV mediated the association between baseline lnKDI and follow-up RBANS total score.
The systematic review by Tang X and Colleagues (2022) showed mixed findings regarding the relationship between kidney function and cognitive impairment or dementia 8. Based on ten longitudinal studies conducted in the European, American and Asian populations, a decline in eGFR as a continuous variable was associated with an elevated risk of cognitive impairment and dementia with risk ratio of 1.04 (95% CI 1.00–1.08).8 However, there was no evidence of association between decline in eGFR with all-cause dementia (RR 1.01, 95% CI 0.97–1.06) and AD (RR 0.96, 95% CI 0.92–1.02) in subgroup analyses.8 On the other hand, an increase in uACR as a continuous variable was associated with an elevated risk of all-cause dementia and cognitive impairment.8,23,24 However, another study demonstrated a null effect of uACR increase on mild cognitive impairment (RR 1.54; 95% CI 0.92–2.58).8,25 The pooled risk ratio of these three studies on the association between continuous uACR and cognitive impairment and dementia was 1.02 (95% C 1.00–1.04) in the systematic review.8 Nevertheless, the few available studies for comparison were acknowledged as a limitation in the meta-analysis.8 Notably, eGFR and uACR were studied as separate exposures of interest in this systematic review and in other previousstudies.
To our knowledge, there is no study which examined the joint effect of eGFR and uACR on cognitive function. Hence our study has provided novel findings on the association between a composite index of renal function and albuminuria with cognitive function. Our study revealed that the association between lnKDI and cognitive function is stronger than the association between its individual components, ln(1/eGFR) and ln(uACR*100), with cognitive function at baseline and follow-up. This suggests a possible synergistic effect between renal function and albuminuria on cognitive function.
The pathophysiological mechanisms underlying the relationship between KDI and uACR remain elusive. However, there are a few proposed explanations for the relationship between eGFR and uACR with cognitive function. First, there are similarities between brain and kidney as they are both considered low-resistance end-organs with exposure to high volume of vascular flow.5 The vasculatures in both the brain and kidneys are therefore highly susceptible to blood pressure fluctuations.5 Of note, diabetes and hypertension can contribute to damage of blood vessels, compromising vascular autoregulation and vascular tone in the brain and kidneys.5 Thus the brain and kidneys are highly vulnerable to reduced perfusion and fluctuations in blood pressure.5 Second, albuminuria is considered a marker of endothelial dysfunction which damages the systemic microcirculation.5 Notably, endothelial damage stimulates blood-brain barrier (BBB) breakdown which itself contributes to the development of cSVD.5 After the occurrence of BBB breakdown, there is increased inflammation which may account for the link between albuminuria and cognitive dysfunction.5 Several circulating inflammatory biomarkers in the blood, such as C-reactive protein (CRP), interleukin-1 (IL-1) and tumor-necrosis factor-α (TNF-α) have been linked to both cSVD and CKD.5 This suggests a shared inflammatory pathway in the pathogenesis of cognitive and renal dysfunctions.5 Third, the loss of renal function may impede the renal clearance of amyloid-β (Aβ), resulting in accumulation of Aβ peptide which characterizes Alzheimer’s disease (AD).5 It is plausible that the combined effects of albuminuria and reduced renal function, as represented by KDI, exert a more detrimental impact than either component alone. This is in line with our current findings which show that the association of KDI is more marked than either component analyzed separately.
It was suggested that arterial stiffness promotes transmission of excessive pressure and flow pulsatility into the renal microvasculature, resulting in microvascular ischemia and renal damage.26 Studies have demonstrated that arterial stiffness is a risk factor for kidney function decline.26,27 There is accumulating evidence that arterial stiffness, which is measured as PWV, is a risk factor of cognitive impairment 11. For the first time, we demonstrated that PWV mediated the association between KDI and cognitive function. This mechanistic pathway suggested that renal dysfunction may precede cognitive dysfunction. Furthermore, our results support an earlier study by Nowak and colleagues (2023) which revealed that the association between kidney function and cognitive dysfunction was mediated by arteriosclerosis biomarkers which included NT-proBNP and GDF15 in community-dwelling individuals.12. These findings suggested potential vascular pathways which underline the association between kidney function and cognitive decline.12
The association between KDI and follow-up cognitive function was evident in patients with KDI progression but not in those without KDI progression. The results suggested that patients with KDI progression or deterioration of kidney function involving both eGFR and uACR might be more susceptible to reduction of cognitive function. This could be attributed to continuing vascular damage leading to reduction of cognitive function.
KDI was associated with decreased cognitive function in immediate memory domain though the association was weaker than that in visuospatial/construction and attention domain. The results were suggestive of cognitive changes in vascular dementia rather than those in AD where memory impairment is more pronounced.28,29 Nevertheless, it is possible for both vascular dementia and AD to co-exist. Further studies with longer follow-up may be needed to determine the type of dementia developed. Other possible speculations are benefit of ongoing metabolic interventions (e.g., insulin has been thought to be neurotrophic30) which may have mitigated progressive deterioration in some cognitive domains, and availability of prospective observation only in a smaller subset (N = 590) which may have limited the study power to observe minor effect size.
There are a few clinical implications arising from our study. First, CKD is highly prevalent in patients with T2D.31 Patients with T2D are also susceptible to development and progression of CKD.6 Hence a composite index of kidney disease may be relevant to future clinical practice as both eGFR and uACR are routinely available for monitoring of diabetic complication in patients with T2D. KDI may potentially be an inexpensive and convenient marker for monitoring cognitive function in patients with T2D. However, it is not possible to conclude if KDI can be used as a marker to predict cognitive decline or in intervention for slowing of cognitive decline. Second, arterial stiffness may be considered a potential target for preventing cognitive dysfunction in patients with T2D.
This is the first study to examine the combined effect of eGFR and uACR on cognitive function over time. The longitudinal study was conducted with a fairly long follow-up period. We also adjusted for a comprehensive range of covariates including demographics, education, APOE ɛ4 allele and clinical parameters. Nevertheless, there are some limitations in our study. First, our study was performed on patients with T2D and hence we were unable to generalize the findings to the general population. Second, we did not collect information on tau, amyloid and neurodegeneration biomarkers. Further studies are needed to study the effect of KDI on these biomarkers. Third, RBANS does not assess executive function directly. Future studies may incorporate executive function tests for a more complete cognitive evaluation. We also lacked brain imaging which can inform us on brain regions involved in cognitive function reduction.
In conclusion, KDI was associated with lower cognitive function in patients globally and in the domains of immediate memory, visuo-spatial/construction and attention. Arterial stiffness also played a mediating role in the association between KDI and cognitive decline in patients with T2D.
AUTHOR CONTRIBUTIONS
Kiat Mun Serena Serena Low (Conceptualization; Formal analysis; Investigation; Methodology; Writing – original draft); Angela Moh (Data curation; Formal analysis; Methodology); Kiat Sern Goh (Supervision; Writing – review & editing); Jonathon Khoo (Formal analysis); Keven Ang (Data curation; Project administration); Allen Yan Lun Liu (Writing – review & editing); Wern Ee Tang (Writing – review & editing); Ziliang Lim (Writing – review & editing); Tavintharan Subramaniam (Writing – review & editing); Chee Fang Sum (Writing – review & editing); Su Chi Lim (Conceptualization; Funding acquisition; Resources; Writing – review & editing).
ACKNOWLEDGMENTS
The authors have no acknowledgments to report.
FUNDING
This work was supported by Singapore National Medical Research Council (MOH-000066, MOH-0000714 and MOH-001327-02).
CONFLICT OF INTEREST
The authors have no conflict of interest to report.
DATA AVAILABILITY
Restrictions apply to the availability of some, or all data generated or analyzed during this study to preserve patient confidentiality. The corresponding author will on request detail the restrictions and any condition under which access to some data may be provided. The data are not publicly available due to privacy or ethical restrictions.
SUPPLEMENTARY MATERIAL
[1] The supplementary material is available in the electronic version of this article: https://dx.doi.org/10.3233/ADR-240067.
REFERENCES
1. | World Health Organization. Dementia: key facts. Switzerland, World Health Organization, 2023. Available at https://www.who.int/news-room/fact-sheets/detail/dementia. (2023, accessed 20 December 2023). |
2. | Biessels GJ and Despa F. Cognitive decline and dementia in diabetes mellitus: mechanisms and clinical implications. Nat Rev Endocrinol (2018) ; 14: : 591–604. |
3. | You Y , Liu Z , Chen Y , et al. The prevalence of mild cognitive impairment in type 2 diabetes mellitus patients: a systematic review and meta-analysis. Acta Diabetol (2021) ; 58: : 671–685. |
4. | Kidney Disease: Improving Global Outcomes (KDIGO) CKD Work Group. KDIGO 2012 clinical practice guideline for the evaluation and management of chronic kidney disease. Kidney Int Suppl (2012) ; 2013: : 1–150. |
5. | Ariton DM , Jiménez-Balado J , Maisterra O , et al. Diabetes, albuminuria and the kidney-brain axis. J Clin Med (2021) ; 10: : 2364. |
6. | Chaudhuri A , Ghanim H and Arora P. Improving the residual risk of renal and cardiovascular outcomes in diabetic kidney disease: A review of pathophysiology, mechanisms, and evidence from recent trials. Diabetes Obesity Metab (2022) ; 24: : 365–376. |
7. | Rizzo MR , Di Meo I , Polito R , et al. Cognitive impairment and type 2 diabetes mellitus: Focus of SGLT2 inhibitors treatment. Pharmacol Res (2022) ; 176: : 106062. |
8. | Tang X , Han YP , Chai YH , et al. Association of kidney function and brain health: A systematic review and meta-analysis of cohort studies. Ageing Res Rev (2022) ; 82: : 101762. |
9. | Gerstein HC , Ramasundarahettige C , Avezum A , et al. A novel kidney disease index reflecting both the albumin-to-creatinine ratio and estimated glomerular filtration rate, predicted cardiovascular and kidney outcomes in type 2 diabetes. Cardiovasc Diabetol (2022) ; 21: : 158. |
10. | Gerstein HC , Ramasundarahettige C and Bangdiwala SI. Creating composite indices from continuous variables for research: the geometric mean. Diabetes Care (2021) ; 44: : e85–e86. |
11. | Winder NR , Reeve EH and Walker AE. Large artery stiffness and brain health: insights from animal models. Am J Physiol Heart Circ Physiol (2021) ; 320: : H424–H431. |
12. | Nowak N , De Looze C , O’Halloran A , et al. The association between kidney function, cognitive function, and structural brain abnormalities in community-dwelling individuals aged 50+ is mediated by age and biomarkers of cardiovascular disease. Cardiovasc Res (2023) ; 119: : 2106–2116. |
13. | Liu XN , Gao HQ , Li BY , et al. Pulse wave velocity as a marker of arteriosclerosis and its comorbidities in Chinese patients. Hypertens Res (2007) ; 30: : 237–242. |
14. | Ng TP , Feng L , Yap KB , et al. Long-term metformin usage and cognitive function among older adults with diabetes. J Alzheimers Dis (2014) ; 41: : 61–68. |
15. | Moh A , Neelam K , Zhang X , et al. Excess visceral adiposity is associated with diabetic retinopathy in a multiethnic Asian cohort with longstanding type 2 diabetes. Endocrine Res (2018) ; 43: : 186–194. |
16. | Hwang CL , Muchira J , Hibner BA , et al. Alcohol consumption: a new risk factor for arterial stiffness? Cardiovasc Toxicol (2022) ; 22: : 236–245. |
17. | Zhang X , Liu JJ , Sum CF , et al. Ethnic disparity in central arterial stiffness and its determinants among Asians with type 2 diabetes. Atherosclerosis (2015) ; 242: : 22–28. |
18. | Randolph C , Tierney MC , Mohr E , et al. The Repeatable Battery for the Assessment of Neuropsychological Status (RBANS): preliminary clinical validity. J Clin Exp Neuropsychol (1998) ; 20: : 310–319. |
19. | Ng TP , Nyunt MSZ , Shuvo FK , et al. The neighborhood built environment and cognitive function of older persons: results from the Singapore Longitudinal Ageing Study. Gerontology (2018) ; 64: : 149–156. |
20. | Levey AS , Stevens LA , Schmid CH , et al. A new equation to estimate glomerular filtration rate. Ann Intern Med (2009) ; 150: : 604–612. |
21. | Low S , Zhang X , Ang K , et al. Discovery and validation of serum creatinine variability as novel biomarker for predicting onset of albuminuria in Type 2 diabetes mellitus. Diabetes Res Clin Pract (2018) ; 138: : 8–15. |
22. | Baron RM and Kenny DA. The moderator-mediator variable distinction in social psychological research: conceptual, strategic, and statistical considerations. J Pers Soc Psychol (1986) ; 51: : 1173–1182. |
23. | Kurella Tamura M , Muntner P , Wadley V , et al. Albuminuria, kidney function, and the incidence of cognitive impairment among adults in the United States. Am J Kidney Dis (2011) ; 58: : 756–763. |
24. | Scheppach JB , Coresh J , Wu A , et al. Albuminuria and estimated GFR as risk factors for dementia in midlife and older age: findings from the ARIC Study. Am J Kidney Dis (2020) ; 76: : 775–783. |
25. | Jiménez-Balado J , Riba-Llena I , Pizarro J , et al. Kidney function changes and their relation with the progression of cerebral small vessel disease and cognitive decline. J Neurol Sci (2020) ; 409: : 116635. |
26. | Sedaghat S , Mattace-Raso FU , Hoorn EJ , et al. Arterial stiffness and decline in kidney function. Clin J Am J Nephrol (2015) ; 10: : 2190–2197. |
27. | Kim CS , Kim HY , Kang YU , et al. Association of pulse wave velocity and pulse pressure with decline in kidney function. J Clin Hypertens (2014) ; 16: : 372–377. |
28. | Elahi FM and Miller BL. A clinicopathological approach to the diagnosis of dementia. Nat Rev Neurol (2017) ; 13: : 457–476. |
29. | Karantzoulis S and Galvin JE. Distinguishing Alzheimer’s disease from other major forms of dementia. Expert Rev Neurotherap (2011) ; 11: : 1579–1591. |
30. | Derakhshan F and Toth C. Insulin and the brain. Curr Diabetes Rev (2013) ; 9: : 102–116. |
31. | Low SK , Sum CF , Yeoh LY , et al. Prevalence of chronic kidney disease in adults with type 2 diabetes mellitus. Ann Acad Med Singap (2015) ; 44: : 164–171. |