Latent Class Analysis of Sleep in Mild Cognitive Impairment Patients and its Influencing Factors
Abstract
Background:
Individuals with mild cognitive impairment (MCI) frequently experience sleep disorders, which may elevate the risk of developing Alzheimer’s disease. Yet, sleep types in MCI patients and the factors influencing them have not been sufficiently investigated.
Objective:
The objective of this study was to explore potential sleep typing and its influencing factors in patients with MCI using latent class analysis.
Methods:
A cross-sectional survey was conducted in Jiangsu Province, China. Cognitive function in older adults was assessed using neuropsychological tests, including the Montreal Cognitive Assessment Scale-Beijing version (MoCA), the Mini-Mental State Examination (MMSE), the Activities of Daily Living Scale (ADL), and the Clinical Dementia Rating Scale (CDR). Sleep quality was evaluated using the Pittsburgh Sleep Quality Index (PSQI). Latent class analysis based on PSQI scores and multinomial logistic regression analyses were employed to explore the influencing factors of sleep typing.
Results:
The study included a total of 611 patients with MCI. Latent class analysis identified three latent classes to categorize the sleep patterns of MCI patients: the good sleep type (56.6%), the insufficient sleep type (29.6%), and the difficulty falling asleep type (13.7%). Potential sleep typing is influenced by gender, chronic disease, physical exercise, social activity, brain exercise, smoking, frailty, subjective cognitive status, and global cognitive function.
Conclusions:
The findings of this study underscore the notable heterogeneity in the sleep patterns of patients with MCI. Future research may provide targeted prevention and interventions to address the characteristics and influencing factors of patients with different subtypes of sleep MCI.
INTRODUCTION
Alzheimer’s disease (AD) is a neurodegenerative disorder characterized by progressive memory loss and cognitive decline, representing the most prevalent cause of dementia. The neuropathological hallmark of AD involves the gradual accumulation of amyloid-β peptides into extracellular plaques and the aggregation of tau proteins into intracellular neurofibrillary tangles [1]. Projections suggest that by 2050, the global prevalence of AD will soar to 131.5 million individuals [2]. Unfortunately, no definitive therapeutic intervention has yet been discovered to halt or reverse the progression of AD. Consequently, there is an urgent need for early identification of pre-AD stages and identification of modifiable risk factors to enable effective prevention strategies. Mild cognitive impairment (MCI) is a syndrome between normal cognitive decline and dementia, characterized by a progressive decline in cognitive functions, including memory, attention, and language ability. The prevalence of MCI in Chinese individuals aged over 60 is approximately 15.5% , with an annual conversion rate to AD of 10% –15% [3]. Notably, the cognitive trajectory in the MCI stage varies significantly, and cognitive function decline can be reversible [4]. Therefore, timely detection and effective intervention of MCI can prevent or delay conversion to AD.
Sleep disturbances are common psychobehavioral symptoms in the early stage of MCI. MCI patients often experience more severe sleep disturbances, with a prevalence rate more than triple that of cognitively healthy older adults, ranging from 57.52% to 70% [5, 6]. MCI patients may exhibit abnormal changes in subjective and objective sleep characteristics, such as total sleep time, sleep latency, sleep efficiency, wake time, and daytime sleepiness [7]. Sleep is significantly associated with cognitive function in MCI patients, and sleep disorders can negatively impact life quality, accelerate cognitive decline and psychobehavioral impairment, increase the risk of dementia, and worsen the burden of disease. Moreover, the relationship between sleep disorders and cognitive impairment can be bidirectional, potentially forming a vicious cycle. On the one hand, sleep disorders can exacerbate cognitive and psychobehavioral impairments, increase dementia risk, and add to the disease burden in MCI patients. On the other hand, disruption of sleep-wake centers by neurodegenerative disorders can also lead to sleep disorders. The interplay between sleep and cognitive function is complex and may involve multiple factors and mechanisms.
Existing research has established that functional abnormalities in the suprachiasmatic nucleus (SCN) of the hypothalamus are linked to sleep disturbances and cognitive decline in patients with MCI. Initially, the dysfunctional SCN may lead to insomnia and disrupted sleep rhythms, which can directly impact cognitive functions such as attention, memory, and executive functioning. Additionally, the SCN modulates brain regions associated with memory, learning, and cognitive functions. Sleep disorders may indirectly impair cognitive functions by disrupting the normal functioning of the SCN [8]. Furthermore, studies indicate that increased interstitial space in the brain during sleep aids in the removal of substances like amyloid-β. However, abnormalities in the exchange between cerebrospinal and tissue fluids during sleep disorders may lead to abnormal deposition of amyloid-β, impairing cognitive function [9]. Neuroimaging research has shown that disruption of sleep-wake homeostasis and circadian rhythm disturbances affect cortical and pro-arousal subcortical brain regions associated with cognitive function, resulting in neuronal damage in the hippocampus similar to early changes in MCI [10, 11]. Therefore, sleep characteristics may serve as a potential target for preventing cognitive decline. Improving sleep in patients with MCI may be a viable, effective, and manageable strategy to delay the progression of their cognitive decline.
Previous studies predominantly utilized a “variable-centered” approach to investigate the relationship between different sleep characteristics and cognitive function. However, the sleep problems of MCI patients are complex, with individual sleep comprising multiple characteristics and being influenced by social, economic, and cultural factors, resulting in evident population heterogeneity. The Pittsburgh Sleep Quality Index (PSQI) is a standardized scale widely used in clinical and epidemiological investigations of sleep medicine due to its high sensitivity and specificity in distinguishing between individuals with good and poor sleep quality [12]. The PSQI consists of seven dimensions: sleep quality, sleep latency, sleep duration, sleep efficiency, sleep disorders, sleep medication use, and daytime dysfunction. The scores of different dimensions reflect variations in sleep characteristics. However, most studies have categorized sleep quality levels based on scale scores, often neglecting differences in sleep characteristics among individuals. Latent Class Analysis (LCA) is an individual-centered statistical method that identifies potential subgroups through pattern matching on exogenous variables. It objectively categorizes research subjects into different latent classes, revealing potential heterogeneity across classes [13]. LCA is widely employed in psychology and sociology research [14]. While previous research has explored the association between sleep characteristics and impairments in different cognitive domains among MCI patients, there is a research gap in understanding the various sleep typologies of MCI patients and their influencing factors.
The objective of this study was to characterize the sleep patterns of patients with MCI using latent class analysis and to explore the factors influencing these sleep classifications. This approach facilitates a deeper understanding of the individual differences and characteristics related to sleep in MCI patients. By categorizing MCI patients into distinct sleep subtypes, we can identify various sleep problems and performance patterns. This classification provides a theoretical basis for the development of precise clinical treatment and nursing care plans tailored to MCI patients with different sleep subtypes. Such an approach holds significant clinical importance and value for improving sleep disorders and decelerating the progression of cognitive decline in MCI patients.
MATERIALS AND METHODS
Participants
A cross-sectional survey study using the convenience sampling method was conducted from May 2022 to November 2023. Study participants were primarily recruited from Nanjing, Changzhou, Nantong, and Xuzhou cities in Jiangsu Province, China. The inclusion criteria were as follows: (1) age≥60 years; (2) elementary school education or higher, with effective reading, writing, and communication skills; (3) diagnosed with MCI. The diagnostic criteria for MCI were based on the guidelines developed by the National Institute on Aging and Alzheimer’s Disease Association Diagnostic Guidelines Writing Group [15] and the 2018 Chinese Guidelines for the Diagnosis and Treatment of Dementia and Cognitive Impairment [16]: (1) cognitive impairment reported by the patient or an informed individual, or detected by an experienced clinician; (2) objective evidence of impairment in one or more cognitive domains (neuropsychological testing), with situational memory impairment being the most common; (3) mild impairment in complex instrumental daily living skills, while independent daily living skills are maintained; and (4) absence of dementia. The exclusion criteria were as follows: (1) cognitive decline caused by other diseases, such as neurodegenerative diseases other than MCI, traumatic brain injury, vascular-related disorders, infection-related cognitive disorders, or psychiatric cognitive disorders; (2) use of neurological, psychotropic drugs, or other medications affecting cognitive function; (3) presence of severe physical illnesses or other conditions affecting sleep, such as anxiety, depression, asthma, or nocturia; (4) use of medications that affect sleep, such as tranquilizers or hypnotics; (5) significant hearing, visual impairments, or speech communication disorders that hinder cooperation with the survey; and (6) participants who discontinued the survey or had incomplete data collection. The study was conducted in accordance with the Declaration of Helsinki and approved by the Ethical Review Committee of Nanjing Hospital of Traditional Chinese Medicine (registration number: ChiCTR2300075195). All subjects provided informed consent by signing a consent form.
Neuropsychological assessment
(1) The Mini-Mental State Examination (MMSE) is a widely used and user-friendly cognitive screening scale that assesses orientation, memory, attention, calculation, recall, and language abilities [17]. It provides a total score of 30, with higher scores indicating better cognitive function. (2) The Beijing version of the Montreal Cognitive Assessment (MoCA) is a more sensitive and specific cognitive screening scale for MCI patients than the MMSE [18]. It primarily evaluates visuospatial and executive abilities, naming, attention, language, abstraction, delayed recall, and orientation abilities. The total score is 30, and higher scores indicate better overall cognitive functioning. If the respondent’s education is≤12 years, the total score is increased by 1 point. A total score of less than 26 indicates MCI [19]. (3) Activities of Daily Living Scale (ADL) include basic daily living skills (toileting, eating, dressing, grooming, walking, and bathing) and instrumental daily living abilities (phone calls, shopping, meal preparation, housework, laundry, use of transport, medication, and money management). It consists of 20 items, each scored on a 4-point Likert scale. The total score ranges from 20 to 80. Lower scores indicate better daily living skills [20]. (4) The Clinical Dementia Rating (CDR) scale is a comprehensive assessment tool for the severity of cognitive impairment and is used to differentiate between normal cognition and various stages of dementia. The ratings are 0 for cognitively normal, 0.5 for suspected dementia, 1.0 for mild dementia, 2.0 for moderate dementia, and 3.0 for severe dementia [21].
Sleep assessment
Subjective sleep quality was assessed using the PSQI, which consists of 18 items covering seven dimensions: subjective sleep quality, sleep latency, sleep duration, sleep efficiency, sleep disorders, medication use, and daytime dysfunction. The total score ranges from 0 to 21 points, with higher scores indicating worse sleep quality [22].
Sociodemographic factors
Sociodemographic variables included gender, age, education level, income level, nature of occupation, retirement status, and marital status. Lifestyle factors included physical exercise (at least once a week for more than 30 min), social activities, brain exercise (includes playing puzzles, practising calligraphy, drawing, playing musical instruments, etc.), smoking, and alcohol consumption. Disease history encompassed family history of dementia and a history of chronic diseases (such as hypertension, diabetes mellitus, heart disease, etc.). In addition, the degree of subjective cognitive decline was assessed using the Subjective Cognitive Decline Questionnaire (SCD-Q9) [23], and the level of frailty was evaluated using the 5-item FRAIL scale (FRAIL) [24].
Statistical analysis
Statistical descriptions of the study population were performed using SPSS 25.0. Non-normally distributed continuous variables were reported as median (M) and quartiles (QR), while categorical variables were presented as frequency (n) and percentage (%). Subsequently, the seven-dimensional scores of the PSQI were used as observational variables for LCA using Mplus 8.3. Model fit was assessed using metrics such as the Akaike Information Criterion (AIC), Bayesian Information Criterion (BIC), and corrected Bayesian Information Criterion (aBIC), with smaller values indicating better model fit. The Lo-Mendell-Rubin likelihood ratio test (LMR) and Bootstrapped likelihood ratio test (BLRT) were performed, and p-values less than 0.05 indicated that the k-class model fit better than the K-1 class model. Entropy was used as an indicator to evaluate the accuracy of categorization, with a value of ≥0.8 indicating a categorization accuracy of over 90%. Furthermore, sociodemographic variables, lifestyle factors, and disease history were compared among different sleep subtypes using the Kruskal-Wallis H test and the χ2 test. Multinomial logistic regression analyses were conducted to explore the influencing factors of the different sleep subtypes, considering indicators that exhibited statistical significance in the univariate analyses. A p-value of less than 0.05 was considered statistically significant.
RESULTS
Sample characteristics
This study included a total of 611 patients with MCI, with a mean age of (71.65±7.45) years. Among the patients, 331 (54.2%) were males and 280 (45.8%) were females. The majority of patients had primary education (30.0%) or junior high school education (34.0%), with a mean education duration of (9.28±3.63) years. Additionally, 59.7% of MCI patients reported having one or more chronic diseases. Details are shown in Table 1.
Table 1
General characteristics of the study population (n = 611)
Variables | n (%)/mean±SD |
Age | 71.65±7.45 |
Gender | |
Male | 253 (41.4) |
Female | 358 (58.6) |
Educational attainment (y) | 9.28±3.63 |
Education | |
Elementary school | 183 (30.0) |
Junior high school | 208 (34.0) |
Senior high school | 119 (19.5) |
University and higher | 101 (16.5) |
Monthly disposable income | |
<3000 CNY | 275 (45.0) |
3000–5000 CNY | 182 (29.8) |
≥5000 CNY | 154 (25.2) |
Nature of occupation | |
mental work | 221 (36.2) |
physical work | 235 (38.5) |
both are combined | 155 (25.4) |
Retirement | |
Yes | 577 (94.4) |
No | 34 (5.6) |
Marriage status | |
Unmarried | 5 (0.8) |
Married | 533 (87.2) |
Divorce or separation | 2 (0.3) |
Widowhood or other | 71 (11.6) |
Family history of dementia | |
Yes | 41 (6.7) |
No | 570 (93.3) |
Chronic disease history | |
Yes | 393 (64.3) |
No | 218 (35.6) |
Physical exercise | |
Yes | 472 (77.2) |
No | 139 (22.7) |
Social activities | |
Yes | 453 (74.1) |
No | 158 (25.8) |
Brain exercise | |
Yes | 393 (64.3) |
No | 218 (35.6) |
Smoke | |
Yes | 158 (25.8) |
No | 453 (74.1) |
Drink | |
Yes | 152 (24.9) |
No | 459 (75.1) |
FRAIL | 0.59±0.76 |
PSQI | 7.53±3.91 |
MoCA | 22.36±2.32 |
SCD-Q9 | 4.91±2.16 |
ADL | 20.27±0.73 |
SD, standard deviation; CNY, Chinese Yuan; FRAIL, 5-item FRAIL scale; PSQI, Pittsburgh Sleep Quality Index; MoCA, Montreal Cognitive Assessment; SCD-Q9, Subjective Cognitive Decline Questionnaire; ADL, Activities of Daily Living Scale.
Results of latent class analysis
Sequentially fitting 1–5 latent class models using the scores on each dimension of the PSQI as exogenous variables resulted in fit indices for each class model, as presented in Table 2. As the number of classes increased, the values of AIC, BIC, and aBIC gradually decreased. The entropy was highest in the 4-class model, and the p-value of the BLRT was < 0.05 in all fitted models. After analysis, the 5-class model was excluded when the number of people in a category was less than 50, and the LMR p-value of the 4-class model was > 0.05. The entropy of the 3-class model was close to 1, and both the LMR and BLRT had p-values < 0.05. Considering the practical significance and interpretability of the typing results, the 3-class model was selected as the best-fitting model, with a probability of 100% for each subgroup’s classification, indicating accurate and reasonable delineation results.
Table 2
Fit indices for latent class modeling of sleep in patients with MCI (n = 611)
Classes | k | AIC | BIC | aBIC | Entory | LMR | BLRT |
1 | 14 | 11018.141 | 11079.952 | 11035.505 | — | — | — |
2 | 22 | 10204.117 | 10301.249 | 10231.404 | 0.851 | 0.0000 | 0.0000 |
3 | 30 | 9283.408 | 9415.861 | 9320.618 | 0.999 | 0.0002 | 0.0000 |
4 | 38 | 7433.540 | 7601.314 | 7480.672 | 1.000 | 0.9975 | 0.0000 |
5 | 46 | 7209.444 | 7412.538 | 7266.498 | 0.971 | 0.0164 | 0.0000 |
k, free parameters; AIC, Akaike information criterion; BIC, Bayesian information criterion; aBIC, sample size adjusted BIC; LMR, Lo-Mendell-Rubin likelihood ratio test; BLRT, Bootstrapped likelihood ratio test.
Naming of latent class
Figure 1 presents the scores on the seven dimensions of the PSQI scale for each potential sleep type of MCI patients, based on the results of the LCA. The first class, named the “good sleep type” (n = 346, 56.6%), exhibited higher scores in the sleep duration and sleep disorder dimensions, but lower scores in all seven dimensions compared to the remaining two classes. The second class, named the “insufficient sleep type” (n = 181, 29.6%), demonstrated higher scores in the dimensions of sleep duration, sleep efficiency, and sleep disorders. The third class, named the “difficulty falling asleep type” (n = 84, 13.7%), exhibited significantly higher scores on the dimensions of falling asleep time and taking hypnotic medication compared to the remaining two groups.
Fig. 1
Characteristics of each potential sleep typing in patients with MCI. Class 1, Good sleep type; Class 2, Insufficient sleep type; Class 3, Difficulty falling asleep type.
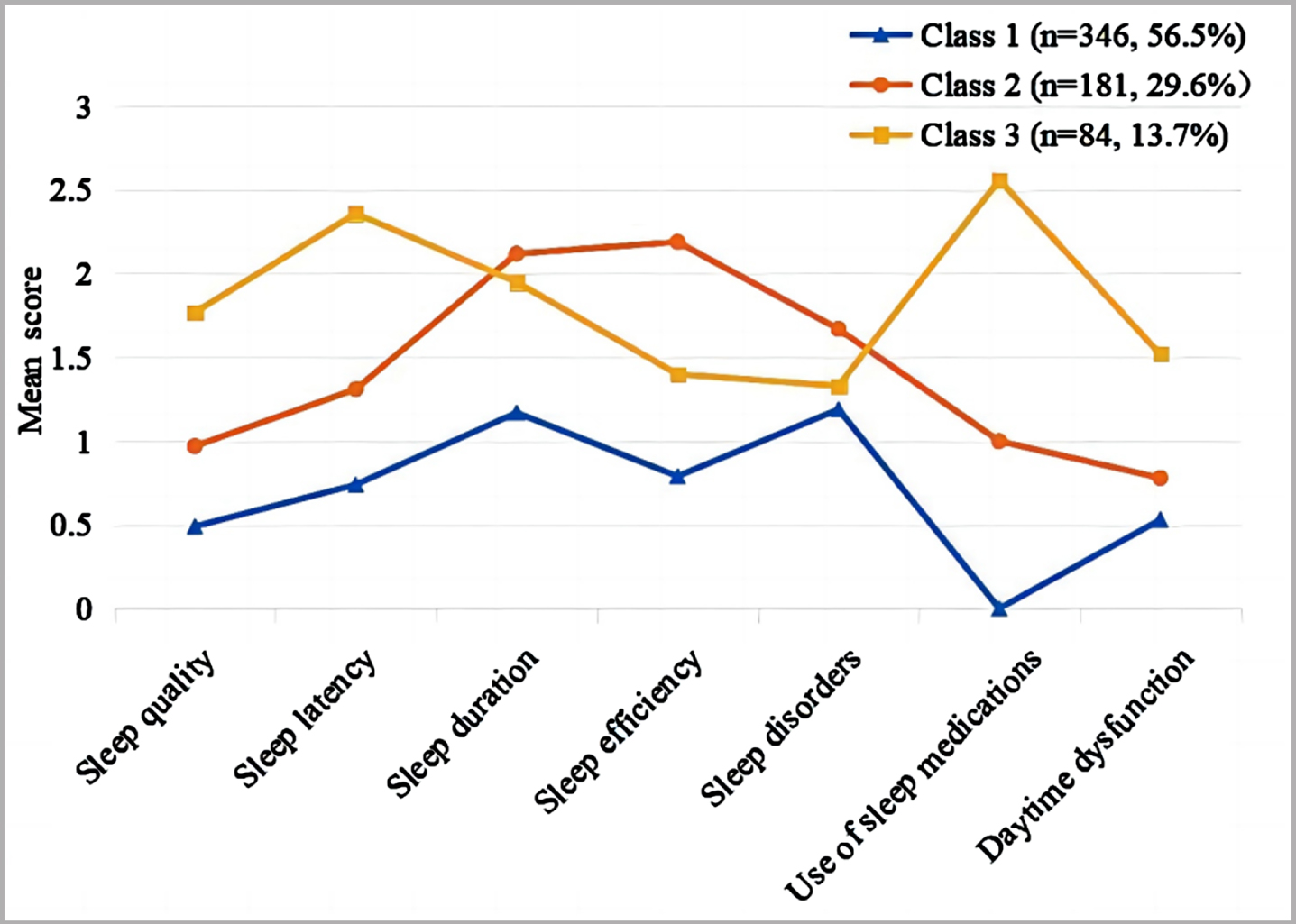
Characteristic differences between classes
Differences in demographic characteristics, frailty, cognitive level, PSQI scores, and daily life abilities were compared among patients with the three potential sleep subtypes of MCI (Table 3). Compared to the good sleep type of MCI, the insufficient sleep type and the difficulty falling asleep type of MCI patients were predominantly female and had lower levels of education. They also had a higher prevalence of chronic illnesses, lower participation rates in physical exercise, social activities, and brain exercise, and a relatively high percentage of smokers. Moreover, patients with the difficulty falling asleep type of MCI had higher scores on the FRAIL, PSQI, and SCD-Q9 scales compared to the other classes. The MoCA scores were in descending order among good sleepers, insufficient sleepers, and those who had difficulty falling asleep.
Table 3
Comparison of the characteristics of patients with three sleep subtypes of MCI
Variables | Class 1 (n = 346) | Class 2 (n = 181) | Class 3 (n = 84) | p |
Age | 71.75±7.63 | 71.26±6.95 | 72.11±7.81 | 0.704 |
Gender | < 0.001* | |||
Male | 175 (50.6) | 58 (32.0) | 20 (23.8) | |
Female | 171 (49.4) | 123 (68.0) | 64 (76.2) | |
Educational attainment (y) | 9.65±3.85 | 8.80±3.26 | 8.81±3.28 | 0.042* |
Education | 0.075 | |||
Elementary school | 93 (26.9) | 61 (33.7) | 29 (34.5) | |
Junior high school | 110 (31.8) | 66 (36.5) | 32 (38.1) | |
Senior high school | 75 (21.7) | 33 (18.2) | 11 (13.1) | |
University and higher | 68 (19.7) | 21 (11.6) | 12 (14.3) | |
Monthly income | 0.469 | |||
<3000CNY | 156 (45.1) | 83 (45.9) | 36 (42.9) | |
3000–5000CNY | 95 (27.5) | 60 (33.1) | 27 (32.1) | |
≥5000CNY | 95 (27.5) | 38 (21.0) | 21 (25.0) | |
Career | 0.194 | |||
mental work | 133 (38.4) | 60 (33.1) | 28 (33.3) | |
physical work | 137 (39.6) | 64 (35.4) | 34 (40.5) | |
both are combined | 76 (22.0) | 57 (31.5) | 22 (26.2) | |
Retirement | 0.471 | |||
Yes | 329 (95.1) | 171 (94.5) | 77 (91.7) | |
No | 17 (4.9) | 10 (5.5) | 7 (8.3) | |
Marriage status | 0.176 | |||
Unmarried | 3 (0.9) | 2 (1.1) | 0 | |
Married | 306 (88.4) | 160 (88.4) | 67 (79.8) | |
Divorce or separation | 1 (0.3) | 0 | 1 (1.2) | |
Widowhood or other | 36 (10.4) | 19 (10.5) | 16 (19.0) | |
Family history of dementia | 0.948 | |||
Yes | 24 (6.9) | 12 (6.6) | 5 (6.0) | |
No | 322 (93.1) | 169 (93.4) | 79 (94.0) | |
Chronic disease history | 0.023* | |||
Yes | 204 (59.0) | 126 (69.6) | 63 (75.0) | |
No | 142 (41.0) | 55 (30.4) | 21 (25.0) | |
Physical activity habits | 0.001* | |||
Yes | 281 (81.2) | 139 (76.8) | 52 (61.9) | |
No | 65 (18.8) | 42 (23.2) | 32 (38.1) | |
Social activities | < 0.001* | |||
Yes | 274 (79.2) | 132 (72.9) | 47 (56.0) | |
No | 72 (20.8) | 49 (27.1) | 37 (44.0) | |
Brain exercise | 0.001* | |||
Yes | 240 (69.4) | 113 (62.4) | 40 (47.6) | |
No | 106 (30.6) | 68 (37.6) | 44 (52.4) | |
Smoke | 0.002* | |||
Yes | 76 (22.0) | 48 (26.5) | 34 (40.5) | |
No | 270 (78.0) | 133 (73.5) | 50 (59.5) | |
Drink | 0.252 | |||
Yes | 92 (26.6) | 45 (24.9) | 15 (17.9) | |
No | 254 (73.4) | 136 (75.1) | 69 (82.1) | |
FRAIL | 0.51±0.73 | 0.60±0.49 | 0.82±0.84 | 0.001* |
PSQI | 4.91±2.63 | 10.04±1.81 | 12.90±2.25 | < 0.001* |
MoCA | 22.77±2.09 | 22.06±2.43 | 21.29±2.54 | < 0.001* |
SCD-Q9 | 4.58±2.16 | 5.22±1.98 | 5.59±2.30 | < 0.001* |
ADL | 20.26±0.76 | 20.28±0.69 | 20.30±0.67 | 0.555 |
SD, standard deviation; Class 1, Good sleep type; Class 2, Insufficient sleep type; Class 3, Difficulty falling asleep type; CNY, Chinese Yuan; PSQI, Pittsburgh Sleep Quality Index; MoCA, Montreal Cognitive Assessment; SCD-Q9, Subjective Cognitive Decline Questionnaire; ADL, Activities of Daily Living Scale; *p < 0.05.
Analysis of factors influencing latent sleep typing
Multinomial logistic regression analyses was performed to investigate the factors influencing potential sleep typing in patients with MCI. The good sleep type served as the reference class (Table 4). Specifically, compared to the good sleep type, MCI patients with the insufficient sleep type were more likely to be female, smokers, have lower levels of overall cognitive functioning, and experience more severe subjective cognitive decline. MCI patients with the difficulty falling asleep type were more likely to be female, have chronic diseases, not participate in physical exercise and brain exercise, not engage in socialization, smoke, exhibit frailty, have worse overall cognitive functioning, and experience more severe subjective cognitive decline.
Table 4
Multinomial logistic regression analyses of latent sleep subtypes
Class 2 (ref = Class 1) | Class 3 (ref = Class 1) | |||||
Variables | OR | (95% CI) | p | OR | (95% CI) | p |
Gender | ||||||
Male | 0.390 | (0.246; 0.619) | < 0.001* | 0.182 | (0.091; 0.366) | < 0.001* |
Female | ref | |||||
Educational attainment (y) | 0.982 | (0.926; 1.041) | 0.548 | 1.036 | (0.954; 1.125) | 0.404 |
Chronic disease history | ||||||
Yes | ref | |||||
No | 0.631 | (0.422; 0.941) | 0.631 | 0.522 | (0.287; 0.947) | 0.033* |
Physical exercise | ||||||
Yes | ref | |||||
No | 1.213 | (0.756; 1.947) | 0.423 | 2.091 | (1.147; 3.81) | 0.016* |
Social activities | ||||||
Yes | ref | |||||
No | 1.290 | (0.813; 2.049) | 0.280 | 2.261 | (1.264; 4.044) | 0.006* |
Brain exercise | ||||||
Yes | ref | |||||
No | 0.947 | (0.611; 1.468) | 0.808 | 1.179 | (0.648; 2.146) | 0.59 |
Smoke | ||||||
Yes | ref | |||||
No | 0.493 | (0.298; 0.814) | 0.006* | 0.190 | (0.098; 0.369) | < 0.001* |
FRAIL | 1.221 | (0.93; 1.604) | 0.151 | 1.469 | (1.035; 2.084) | 0.031* |
MoCA | 0.886 | (0.809; 0.969) | 0.008* | 0.807 | (0.717; 0.909) | < 0.001* |
SCD-Q9 | 1.108 | (1.009; 1.217) | 0.031* | 1.182 | (1.033; 1.353) | 0.015* |
OR, odds ratio; 95% CI, 95% Confidence Interval. Ref, reference group; Class 1, Good sleep type; Class 2, Insufficient sleep type; Class 3, Difficulty falling asleep type; MoCA, Montreal Cognitive Assessment; SCD-Q9, Subjective Cognitive Decline Questionnaire; *p < 0.05.
DISCUSSION
The purpose of this study was to investigate the potential sleep subtypes of MCI patients and identify their influencing factors. Based on the characteristic differences in the seven dimensions of the PSQI scale, we classified MCI patients’ sleep into three potential classes: good sleep, insufficient sleep, and difficulty falling asleep type. In particular, more than half of the MCI patients belonged to the good sleep type, and the mean PSQI scores of such MCI patients were the lowest, and the scores of all dimensions were lower than those of the remaining two categories, indicating that the overall sleep status of such patients was better. However, it is important to note that patients with good sleep MCI still require attention, as they display a significant upward trend in the scores of sleep duration and sleep disorder dimensions. This suggests a potential risk for further deterioration in their sleep and cognitive status, considering the interrelationship between cognitive decline and sleep disorders. Lifestyle interventions, such as maintaining a reasonable diet, engaging in moderate physical activity, avoiding overstimulation, improving the sleep environment, and establishing regular sleep habits, may help improve sleep in these patients. Additionally, regular assessment and monitoring of patients’ sleep and cognitive functions are necessary to allow for timely interventions to prevent deterioration.
29.6% of MCI sufferers belonged to the insufficient sleep type. Based on specific entries and scoring criteria of the PSQI, it was observed that patients in this type had an average sleep duration of less than 6 hours, an average sleep efficiency of less than 75% , and may experience disturbances such as frequent awakenings, early morning awakenings, nocturia, and nightmares. The American Sleep Foundation recommends that older adults should aim for 7–8 hours of sleep, highlighting a significant sleep duration deficit in this population. Self-reported sleep duration was found to be U-shapedly associated with the risk of MCI in older adults, and the mechanism of association between sleep duration and the risk of MCI may be related to elevated levels of inflammatory cytokines such as C-reactive protein and interleukin 6. Longitudinal studies have shown that sleep duration correlates with cognitive function, and appropriately increasing sleep duration for those sleeping less than 6 hours reduces the risk of MCI [25].Therefore, for patients with insufficient sleep, measures to improve sleep quality and prolong sleep duration may be an effective strategy to prevent and delay the progression of MCI.
Additionally, 13.7% (n = 84) of the MCI patients belonged to the difficulty falling asleep type. Patients in this class took longer to fall asleep and more frequently used hypnotic drugs for sleep induction. Hypnotic medications have numerous adverse effects, yet they remain commonly prescribed for the treatment of sleep disorders in older adults. Studies have found an association between the use of hypnotic drugs and cognitive impairment [26], with long-term use increasing the risk of cognitive decline by 33% and the risk of dementia by 79% [27, 28]. Furthermore, insomniacs taking hypnotic medications continue to experience poorer sleep quality, and the residual sedative effects of these medications can exacerbate daytime dysfunction [29]. It is evident that relying solely on hypnotic medication is not the ideal approach to treating sleep disorders in the elderly. Univariate analysis revealed that patients in this group exhibited poorer performance in terms of frailty, subjective cognitive decline, and overall cognitive functioning compared to the remaining two groups. Therefore, it is crucial to prioritize this group and provide targeted interventions as early as possible.
Our study identified gender, chronic disease, physical exercise, social activities, mental exercise, smoking, debility, overall cognitive level, and level of subjective cognitive decline as influential factors in the potential sleep subtyping of MCI patients. Due to various physiological and psychological factors, females, especially older women, are more susceptible to sleep problems compared to males. Their risk of developing insomnia is 1.73 times higher than that of males [30, 31]. This increased susceptibility may be attributed to lower estrogen levels in older women, which can affect the sleep-wake cycle [32]. Additionally, women are more prone to negative emotions such as anxiety and depression, or may be overly preoccupied with sleep problems, which could further contribute to the higher prevalence of sleep issues among this group. Emerging evidence suggests that gender moderates self-reported sleep status and objective cognitive performance, with women being more vulnerable to the impact of poor sleep quality on cognitive decline [33]. The differences in cognitive performance related to sleep disorders between males and females may be attributed to underlying mechanisms such as changes in brain structure and anatomy, synaptic and structural plasticity, decreased estrogen levels, and alterations in neurotransmitter systems in the hippocampus [34]. Future research should delve deeper into the mechanisms by which gender influences sleep disorders and cognitive function to provide more effective prevention and intervention strategies. Healthcare professionals should conduct more detailed and comprehensive sleep assessments for female MCI patients to gain a thorough understanding of their sleep disturbances and provide comprehensive and effective treatment strategies.
The results of this study indicate that MCI patients with one or more chronic diseases have a higher likelihood of experiencing sleep problems. Previous studies have consistently demonstrated the interaction between chronic diseases and sleep disorders. It has been reported that dementia patients with chronic physical multiple disorders had more than twice the likelihood of experiencing sleep disorders compared to patients with no or only one chronic disease [35]. Additionally, chronic diseases are recognized as risk factors for cognitive impairment, and community-dwelling older adults with chronic diseases may experience cognitive decline independent of their gender, age, education, and comorbidities [36]. Therefore, timely assessment and management of sleep in older adults at risk for debilitation are necessary. Individualized sleep management and intervention plans should be tailored to the specific circumstances and needs of each older adult. Healthcare professionals should be knowledgeable about the type, severity, and treatment of chronic illnesses in patients with MCI. They should also be aware of the manifestation and impact of sleep problems and provide psychological support and behavioral interventions to enhance the patient’s ability to self-manage. Additionally, medication regimens for chronic conditions may need to be adjusted to minimize medication-related adverse effects.
There is a bidirectional relationship between sleep disorders and debility, both of which may share common risk factors such as chronic illness, social isolation, and substance use [37]. Patients with the difficulty falling asleep type of MCI have a higher predisposition to debilitation compared to those in the good sleep type. There is a cumulative effect between sleep problems and debility, and the mechanism of interaction may involve overactivation of the neuroendocrine system, enhanced inflammatory responses, and psychological factors such as depression and anxiety [38]. Therefore, timely assessment and management of sleep status in older adults at risk for debility is critical. Individualized sleep management and intervention plans should be developed for each older person’s specific situation and needs in order to improve their sleep quality and reduce the risk of debility. Therefore, timely assessment and management of sleep are crucial for older adults at risk of debilitation. Individualized sleep management and intervention plans should be developed to address the specific circumstances and needs of each individual.
Smoking is widely recognized as a risk factor for sleep problems, and nicotine in tobacco stimulates nicotinic-acetylcholine receptors and promotes the release of a variety of neurotransmitters, thereby affecting the sleep-wake cycle [39]. A meta-analysis that included cohort studies examining the impact of smoking on the prevalence of insomnia revealed a significantly higher prevalence of sleep disorders among smokers [40]. Compared to non-smokers, smokers have shorter sleep duration, longer sleep onset latency, and a higher likelihood of developing sleep apnea [41]. Previous research has demonstrated that physical activity can serve as an effective non-pharmacological intervention to improve sleep quality [42]. Findings from a randomized clinical trial indicated that tai chi or regular physical activity significantly enhanced objective sleep efficiency and decreased the duration and frequency of awakenings after sleep onset in patients with insomnia [43]. A large-scale study demonstrated that physically inactive adults who were smokers were at a higher risk of developing insomnia [44]. Consistent with these studies, the present study found that patients with MCI who smoked and did not engage in physical activity were more likely to experience sleep deprivation and difficulty falling asleep.
There is a clear association between social interactions and sleep, as indicated by several studies demonstrating that social isolation in older adults is inversely associated with sleep quality and predicts poorer sleep quality in subsequent years [45, 46]. Study found that restricting sleep duration in adults could diminish their inclination to engage in socialization and reduce positive social emotions [47]. Similarly, the present study revealed lower engagement in social activities among patients with the difficulty falling asleep type of MCI. These various influencing factors underscore the significance of maintaining a healthy lifestyle, which can be promoted through health education and behavioral interventions. Strategies such as smoking cessation, increased physical activity, and active participation in social activities are crucial for enhancing sleep and overall well-being.
Furthermore, this study found that patients with insufficient sleep and difficulty falling asleep types of MCI experienced more severe subjective cognitive decline and cognitive impairment. Consistent with previous studies, subjective sleep problems and objective sleep structure in patients with sleep disorders are associated with multiple cognitive domains, including executive function and processing speed [48, 49]. Improving sleep in patients with MCI provides an opportunity to delay further cognitive decline, highlighting the importance of breaking the vicious cycle between sleep disorders and cognitive decline.
Our study has several limitations. The use of convenience sampling in this study may introduce selection bias, potentially limiting the representativeness of the outcomes across the elderly population with MCI. Furthermore, the established inclusion criteria could affect the generalizability of the research conclusions. In terms of data collection, the exclusion of participants who failed to complete the survey or provided incomplete data may lead to the loss of potentially valuable information, thereby impacting the study’s integrity and accuracy. Future research should consider employing random sampling techniques and including a broader spectrum of ages, educational backgrounds, and MCI patients from various regions to enhance the generalizability of the findings. Implementing strategies to minimize data loss and investigating appropriate statistical methods for handling incomplete data are crucial steps toward enhancing the reliability and representativeness of the research. Additionally, this study predominantly relied on subjective sleep assessment scales, which may be subject to individual perceptual and recall biases. The cross-sectional design employed further complicates the establishment of a causal relationship between sleep disturbances and MCI. Future studies should consider the use of objective tools such as polysomnography or actigraphy to evaluate sleep quality and patterns. Integrating multimodal data collection methods, including biomarkers, cognitive tests, and imaging studies, and conducting longitudinal studies will allow for a more comprehensive exploration of the impact of different sleep phenotypes on long-term cognitive change and disease progression in patients with MCI. Given the potential association between sleep disturbances and the progression of MCI, subsequent research might investigate interventions targeting sleep issues to assess their efficacy in improving cognitive functions.
Conclusions
This study is the first to identify three distinct sleep phenotypes in patients with MCI, highlighting significant sleep heterogeneity. Our results underscore the importance of personalized sleep management in MCI patients. Although current research has focused on sleep interventions for MCI patients, such as cognitive-behavioral therapy, light therapy, and exercise interventions, there is a lack of targeted intervention strategies based on different sleep characteristics. Comparing the three sleep categories and analyzing influencing factors deepen our understanding of sleep issues in MCI patients, providing a basis for personalized interventions, predicting disease progression, and guiding clinical decisions. Additionally, this study also lays an important foundation for further exploring the biological mechanisms and intervention measures for sleep disorders in MCI patients. In summary, this research is significant for understanding sleep disturbances in MCI patients and offers a solid scientific basis for developing more precise and personalized treatment methods.
AUTHOR CONTRIBUTIONS
Yamei Bai (Data curation; Investigation; Methodology; Writing – original draft); Meng Tian (Data curation; Investigation; Methodology; Writing – original draft); Yuqing Chen (Data curation; Investigation; Methodology); Yulei Song (Data curation; Supervision; Writing – review & editing); Xueqing Zhang (Data curation; Supervision); Haiyan Yin (Data curation; Supervision); Dan Luo (Supervision; Writing – review & editing); Guihua Xu (Resources; Software; Writing – review & editing).
ACKNOWLEDGMENTS
The authors extend their sincere appreciation to all study participants, study team members, and relevant community staff for their invaluable contributions. We are also grateful for the constructive suggestions and insights provided by the anonymous reviewers. Additionally, the authors would like to acknowledge the assistance of ChatGPT-3.5, an AI language model developed by OpenAI (https://www.openai.com), which was used for the language refinement of this manuscript.
FUNDING
This work was supported by the the National Natural Science Foundation of China under Grant 72174095; Social Science Foundation of Jiangsu Province under Grant 20GLB018; Jiangsu Province Social Development Project Fund under Grant BE2022802; Postgraduate Research & Practice Innovation Program of Jiangsu Province under Grant SJCX23_0832.
CONFLICT OF INTEREST
The authors have no conflict of interest to report.
DATA AVAILABILITY
Data can be obtained upon request.
REFERENCES
[1] | Scheltens P , De Strooper B , Kivipelto M , Holstege H , Chételat G , Teunissen CE , Cummings J , van der Flier WM ((2021) ) Alzheimer’sdisease. Lancet 397: , 1577–1590. |
[2] | ((2020) ) 2020 Alzheimer’s disease facts and figures. Alzheimers Dement 16: , 391–460. |
[3] | Jia L , Du Y , Chu L , Zhang Z , Li F , Lyu D , Li Y , Li Y , Zhu M , Jiao H , Song Y , Shi Y , Zhang H , Gong M , Wei C , Tang Y , Fang B , Guo D , Wang F , Zhou A , Chu C , Zuo X , Yu Y , Yuan Q , Wang W , Li F , Shi S , Yang H , Zhou C , Liao Z , Lv Y , Li Y , Kan M , Zhao H , Wang S , Yang S , Li H , Liu Z , Wang Q , Qin W , Jia J ((2020) ) Prevalence, risk factors, andmanagement of dementia and mild cognitive impairment in adults aged60 years or older in China: A cross-sectional study. LancetPublic Health 5: , e661–e671. |
[4] | Iraniparast M , Shi Y , Wu Y , Zeng L , Maxwell CJ , Kryscio RJ , St JP , SantaCruz KS , Tyas SL ((2022) ) Cognitive reserve and mild cognitive impairment: Predictors and rates of reversion to intact cognition vs progression to dementia. Neurology 98: , e1114–e1123. |
[5] | Song D , Yu D , Sun Q , He G ((2020) ) Correlates of sleep disturbance among older adults with mild cognitive impairment: A cross-sectional study. Int J Environ Res Public Health 17: , 4862. |
[6] | Gao F , Wei S , Dang L , Gao Y , Gao L , Shang S , Chen C , Huo K , Wang J , Wang J , Qu Q ((2022) ) Sleep disturbance is associated with mild cognitive impairment: A community population-based cross-sectional study. BMC Public Health 22: , 2000. |
[7] | Liang Y , Liu W , Wang M ((2023) ) Characteristics of macroscopic sleep structure in patients with mild cognitive impairment: A systematic review. Front Psychiatry 14: , 1212514. |
[8] | Van Erum J , Van Dam D , De Deyn PP ((2018) ) Sleep and Alzheimer’s disease: A pivotal role for the suprachiasmatic nucleus. Sleep Med Rev 40: , 17–27. |
[9] | Xie L , Kang H , Xu Q , Chen MJ , Liao Y , Thiyagarajan M , O’Donnell J , Christensen DJ , Nicholson C , Iliff JJ , Takano T , Deane R , Nedergaard M ((2013) ) Sleep drives metabolite clearance from the adult brain. Science 342: , 373–377. |
[10] | Schmidt C , Peigneux P , Cajochen C ((2012) ) Age-related changes in sleep and circadian rhythms: Impact on cognitive performance and underlying neuroanatomical networks. Front Neurol 3: , 118. |
[11] | Ali A , von Gall C ((2022) ) Adult neurogenesis under control of the circadian system. Cells 11: , 764. |
[12] | Tsai PS , Wang SY , Wang MY , Su CT , Yang TT , Huang CJ , Fang SC ((2005) ) Psychometric evaluation of the Chinese version of the Pittsburgh Sleep Quality Index (CPSQI) in primary insomnia and control subjects. Qual Life Res 14: , 1943–1952. |
[13] | Lubke GH , Muthén B ((2005) ) Investigating population heterogeneity with factor mixture models. Psychol Methods 10: , 21–39. |
[14] | Lim K , Smucny J , Barch DM , Lam M , Keefe R , Lee J ((2021) ) Cognitive subtyping in schizophrenia: A latent profile analysis. Schizophr Bull 47: , 712–721. |
[15] | Albert MS , DeKosky ST , Dickson D , Dubois B , Feldman HH , Fox NC , Gamst A , Holtzman DM , Jagust WJ , Petersen RC , Snyder PJ , Carrillo MC , Thies B , Phelps CH ((2011) ) The diagnosis of mild cognitive impairment due to Alzheimer’s disease: Recommendations from the National Institute on Aging-Alzheimer’s Association workgroups on diagnostic guidelines for Alzheimer’s disease. Alzheimers Dement 7: , 270–279. |
[16] | Chinese Guidelines for the Diagnosis and Treatment of Dementia and Cognitive Impairments Writing Group, Cognitive Impairments Professional Committee of the Neurology Branch of the Chinese Medical Association ((2018) ) Guidelines for the diagnosis and treatment of dementia and cognitive impairments in China (2018) Part 5: Diagnosis and treatment of mild cognitive impairment. Chin Med J 98: , 1294–1301. |
[17] | Gao MY , Yang M , Kuang WH , Qiu PY ((2015) ) Factors and validity analysis of Mini-Mental State Examination in Chinese elderly people. Beijing Da Xue Xue Bao Yi Xue Ban 47: , 443–449. |
[18] | Yu J , Li J , Huang X ((2012) ) The Beijing version of the Montreal Cognitive Assessment as a brief screening tool for mild cognitive impairment: A community-based study. BMC Psychiatry 12: , 156. |
[19] | Nasreddine ZS , Phillips NA , Bédirian V , Charbonneau S , Whitehead V , Collin I , Cummings JL , Chertkow H ((2005) ) The Montreal Cognitive Assessment, MoCA: A brief screening tool for mild cognitive impairment. J Am Geriatr Soc 53: , 695–699. |
[20] | He Y , Qu G , Xiong X , Chi Y , Zhang M , Zhang M ((1990) ) Evaluation of the ability of the elderly to perform activities of daily living. Chin J Geriatr 28: , 266–269. |
[21] | Woolf C , Slavin MJ , Draper B , Thomassen F , Kochan NA , Reppermund S , Crawford JD , Trollor JN , Brodaty H , Sachdev PS ((2016) ) Can the Clinical Dementia Rating Scale identify mild cognitive impairment and predict cognitive and functional decline? Dement Geriatr Cogn Disord 41: , 292–302. |
[22] | Buysse DJ , Reynolds CR , Monk TH , Berman SR , Kupfer DJ ((1989) ) The Pittsburgh Sleep Quality Index: A new instrument for psychiatric practice and research. Psychiatry Res 28: , 193–213. |
[23] | Gifford KA , Liu D , Romano RR , Jones RN , Jefferson AL ((2015) ) Development of a subjective cognitive decline questionnaire using item response theory: A pilot study. Alzheimers Dement (Amst) 1: , 429–439. |
[24] | Morley JE , Malmstrom TK , Miller DK ((2012) ) A simple frailty questionnaire (FRAIL) predicts outcomes in middle aged African Americans. J Nutr Health Aging 16: , 601–608. |
[25] | Wang X , Chen Y , Yue B , Li S , Liu Q , Li Q , Li L , Sun J ((2021) ) Association of changes in self-reported sleep duration with mild cognitive impairment in the elderly: A longitudinal study. Aging (Albany NY) 13: , 14816–14828. |
[26] | Jeong W , Joo JH , Kim H , Kim YK , Park EC , Jang SI ((2021) ) Association between the use of hypnotics and the risk of Alzheimer’s disease. J Alzheimers Dis 81: , 1381–1389. |
[27] | Lee JE , Ju YJ , Chun KH , Lee SY ((2020) ) The frequency of sleep medication use and the risk of subjective cognitive decline (SCD) or SCD with functional difficulties in elderly individuals without dementia. J Gerontol A Biol Sci Med Sci 75: , 1693–1698. |
[28] | Tseng LY , Huang ST , Peng LN , Chen LK , Hsiao FY ((2020) ) Benzodiazepines, z-hypnotics, and risk of dementia: Special considerations of half-lives and concomitant use. Neurotherapeutics 17: , 156–164. |
[29] | Tanielian M , Antoun J , Sidani M , Halabi A , Hoballah M , Hawatian K , Assaf G ((2022) ) Sleep pattern and predictors of daily versus as-needed hypnotics use in middle-aged and older adults with insomnia. BMC Prim Care 23: , 98. |
[30] | Suh S , Cho N , Zhang J ((2018) ) Sex differences in insomnia: From epidemiology and etiology to intervention. Curr Psychiatry Rep 20: , 69. |
[31] | Zhang B , Wing YK ((2006) ) Sex differences in insomnia: A meta-analysis. Sleep 29: , 85–93. |
[32] | Morssinkhof M , van Wylick DW , Priester-Vink S , van der Werf YD , den Heijer M , van den Heuvel OA , Broekman B ((2020) ) Associations between sex hormones, sleep problems and depression: A systematic review. Neurosci Biobehav Rev 118: , 669–680. |
[33] | Curtis AF , Costa AN , Musich M , Schmiedeler A , Jagannathan S , Connell M , Atkinson A , Miller MB , McCrae CS ((2024) ) Sex as a moderator of the sleep and cognition relationship in middle-aged and older adults: A preliminary investigation. Behav Sleep Med 22: , 14–27. |
[34] | Zhou L , Kong J , Li X , Ren Q ((2023) ) Sex differences in the effects of sleep disorders on cognitive dysfunction. Neurosci Biobehav Rev 146: , 105067. |
[35] | Bosak K , Filipčić I , Bajić Ž , Grošić V ((2023) ) Moderating effect of sleep quality on the association betweenhospital anxiety and quality of life in patients with mild tomoderate dementia; A cross-sectional study. Eur Psychiatry 66: , S1103–S1104. |
[36] | Kim J , Park E , An M ((2019) ) The cognitive impact of chronic diseases on functional capacity in community-dwelling adults. J Nurs Res 27: , 1–8. |
[37] | Tang JY , Luo H , Tse M , Lum TY , Wong GH , Li SX ((2021) ) The relationship between insomnia symptoms and frailty in community-dwelling older persons: A path analysis. Sleep Med 84: , 237–243. |
[38] | Piovezan RD , Abucham J , Dos SR , Mello MT , Tufik S , Poyares D ((2015) ) The impact of sleep on age-related sarcopenia: Possible connections and clinical implications. Ageing Res Rev 23: , 210–220. |
[39] | McKenzie M , Olsson CA , Jorm AF , Romaniuk H , Patton GC ((2010) ) Association of adolescent symptoms of depression and anxiety with daily smoking and nicotine dependence in young adulthood: Findings from a 10-year longitudinal study. Addiction 105: , 1652–1659. |
[40] | Hu N , Wang C , Liao Y , Dai Q , Cao S ((2021) ) Smoking and incidence of insomnia: A systematic review and meta-analysis of cohort studies. Public Health 198: , 324–331. |
[41] | Jaehne A , Unbehaun T , Feige B , Lutz UC , Batra A , Riemann D ((2012) ) How smoking affects sleep: A polysomnographical analysis. Sleep Med 13: , 1286–1292. |
[42] | Solis-Navarro L , Masot O , Torres-Castro R , Otto-Yáñez M , Fernández-Jané C , Solà-Madurell M , Coda A , Cyrus-Barker E , Sitjà-Rabert M , Pérez LM ((2023) ) Effects on sleep qualityof physical exercise programs in older adults: A systematic review and meta-analysis. Clocks Sleep 5: , 152–166. |
[43] | Siu PM , Yu AP , Tam BT , Chin EC , Yu DS , Chung KF , Hui SS , Woo J , Fong DY , Lee PH , Wei GX , Irwin MR ((2021) ) Effects of Tai Chi or exercise on sleep in older adults with insomnia: A randomized clinical trial. JAMA Netw Open 4: , e2037199. |
[44] | Chen LJ , Steptoe A , Chen YH , Ku PW , Lin CH ((2017) ) Physical activity, smoking, and the incidence of clinically diagnosed insomnia. Sleep Med 30: , 189–194. |
[45] | Yu B , Steptoe A , Niu K , Ku PW , Chen LJ ((2018) ) Prospective associations of social isolation and loneliness with poor sleep quality in older adults. Qual Life Res 27: , 683–691. |
[46] | Benson JA , McSorley VE , Hawkley LC , Lauderdale DS ((2021) ) Associations of loneliness and social isolation with actigraph and self-reported sleep quality in a national sample of older adults. Sleep 44: , 140. |
[47] | Palmer CA , John-Henderson NA , Bawden H , Massey A , Powell SL , Hilton A , Carter JR ((2023) ) Sleep restriction reduces positive social emotions and desire to connect with others. Sleep 46: , 265. |
[48] | Basta M , Simos P , Vgontzas A , Koutentaki E , Tziraki S , Zaganas I , Panagiotakis S , Kapetanaki S , Fountoulakis N , Lionis C ((2019) ) Associations between sleep duration and cognitive impairment in mild cognitive impairment. J Sleep Res 28: , e12864. |
[49] | Djonlagic I , Mariani S , Fitzpatrick AL , Van Der Klei V , Johnson DA , Wood AC , Seeman T , Nguyen HT , Prerau MJ , Luchsinger JA , Dzierzewski JM , Rapp SR , Tranah GJ , Yaffe K , Burdick KE , Stone KL , Redline S , Purcell SM ((2021) ) Macro and micro sleep architecture and cognitive performance in older adults. Nat Hum Behav 5: , 123–145. |