Distinct and Overlapping Metabolites Associated with Visual Impairment and Cognitive Impairment
Abstract
Background:
Previous studies found that visual impairment (VI) is associated with higher risk of cognitive impairment, but the molecular basis of these conditions is unknown.
Objective:
We aim to compare the metabolite associations of VI and cognitive impairment.
Methods:
The study population with comprehensive measurements was derived from the UK Biobank study. Visual acuity worse than 0.3 logMAR units were defined as VI. Failure in one or more of the four cognitive tests was defined as cognitive impairment. A panel of 249 metabolites was measured using a nuclear magnetic resonance metabolites profiling platform. Logistic regression models were applied to compare metabolite associations with VI and cognitive impairment.
Results:
23,775 participants with complete data on visual acuity, cognitive tests and metabolomics, and without a history of neurological disorders at baseline were included. After adjusting for confounding factors, VI was significantly associated with cognitive impairment (odds ratio[OR] = 1.49, 95% confidence interval [CI]: 1.27–1.74, p < 0.001). After multiple testing correction (p < 9×10–4), five metabolites including the ratio of omega-6 to omega-3 fatty acids (FAs) (OR = 1.18[1.10–1.27]), ratio of omega-3 to total FAs (OR = 0.84[0.77–0.91]), ratio of docosahexaenoic acid (DHA) to total FAs (OR = 0.86[0.80–0.94]), DHA (OR = 0.85[0.78–0.92]), and omega-3 FAs (OR = 0.84[0.77–0.91]) were uniquely associated with VI. Glycoprotein acetyls (OR = 1.06[1.03–1.10]) and alanine (OR = 0.95[0.92–0.98]) were exclusively associated with cognitive impairment. Albumin was identified as the common metabolite shared by the two phenotypes (OR = 0.90[0.85–0.95] for VI, and 0.95[0.92–0.98]) for cognitive impairment).
Conclusions:
We identified distinct and overlapping metabolites associated with VI and cognitive impairment, unveiling their distinct metabolic profiles and potential common pathophysiology.
INTRODUCTION
Cognitive impairment is an emerging public health concern with a prevalence ranging from 12.6% to 26.6%.1–3 Another leading condition in the aging population is visual impairment (VI)4 which affected over 400 million in 2015.5 Both comorbidities were associated with poorer quality of life and higher risk of functional disabilities.6–8 With an aging population, the disease burden of cognitive impairment and VI are projected to increase over time.3,9
Notably, growing evidence has suggested that VI is associated with cognitive impairment or dementia both in cross-sectional and longitudinal studies.10–16 Particularly, a recent systematic review and meta-analysis suggested that visually impaired individuals are at 35% increased risk of developing cognitive impairment and 47% increased risk of developing dementia. Moreover, VI accounted for an estimated 4.7% of the global disease burden of dementia.17 Several hypotheses have been developed to explain this association. First, the common cause theory posits that common risk factors or pathologies contribute to visual-debilitating eye diseases and neurodegenerative diseases.14 Secondly, the plausible causal theory suggests that VI may lead to decreased cognitive reserve (i.e., the brain’s ability to maintain normal cognitive function under damage or pathology) or trigger downstream consequences that result in cognitive decline.18,19 Despite these theoretical hypotheses, no study to date has investigated the molecular mechanisms underlying the VI-cognitive impairment association.
Metabolomics profiling quantitatively provides a functional readout of biochemical processes, thereby facilitating the linkage to phenotypes.20 Moreover, it has great potential to systematically evaluate complex diseases influenced by both genetic and environmental factors.21,22 The metabolomic profiling of diseases has shown increasing promise in its ability to detect novel biomarkers and provide insights into underlying mechanisms.23,24
Therefore, we aim to investigate the distinct and overlapping metabolites associated with VI and cognitive impairment in the middle-aged and older adults of the UK Biobank Study to understand 1) the common metabolites that contribute to the close relationship between the two phenotypes, and 2) their respective metabolic biomarkers.
METHODS
Study participants
UK Biobank is a large-scale biomedical database with over 500,000 individuals aged 40–69 recruited at 22 assessment centers across the United Kingdom. The detailed protocols for the recruitment and collection of biomedical data of the participants have been described elsewhere.25 Briefly, during baseline assessment from 2006 to 2010, questionnaires and verbal interviews on health-related conditions were conducted. Information on physical measurements, cognitive function, biological samples of blood, saliva, and urine, and genomic data were also obtained. Health outcomes were collected through data linkage to primary care, hospital inpatient records, and death registrations. In 2009, vision examinations were introduced at six assessment centers, including visual acuity, intraocular pressure, keratometry, fundus images, and optical coherence tomography.
In the present study, participants with a history of neurological disorders (Supplementary Table 1), and incomplete data on visual acuity, incomplete data on cognitive tests to define no cognitive impairment and incomplete data on metabolomics were excluded, leaving 23,775 participants included in the study (Supplementary Figure 1). The baseline characteristics of the included and excluded participants are shown in Supplementary Table 2.
Ethical approval
The UK Biobank Study received ethical approval from the Northwest Multi-Centre Research Ethics Committee, the National Information Governance Board for Health & Social Care (NIGB), and the Community Health Index Advisory Group (CHIAG). All researchers are able to apply for access to the data. All participants provided written informed consent. The study was conducted adhering to the tenets of the Declaration of Helsinki.
Metabolite quantification
A panel of 249 metabolites and indexes was measured in EDTA plasma samples from UK Biobank participants, using a high-throughput nuclear magnetic resonance (NMR) metabolites profiling platform developed by Nightingale Health Ltd. (Finland). The blood samples were drawn non-fasting but with a recommendation of at least four hours of fasting.26 NMR analytical platform demonstrated the strengths of high reproducibility, minimum sample preparation and non-destructiveness which enables repeat tests on the same sample and is subjective to large-scale analyses.27 The first release of this metabolomics data from approximately 120,000 UK Biobank participants was introduced in 2021. The panel includes lipoprotein lipids in 14 subclasses, fatty acids and their compositions, and various low-molecular-weight metabolites, such as amino acids, ketone bodies, and glycolysis metabolites. The detailed protocols of NMR metabolomics have been described elsewhere.26
VI ascertainment
The detailed protocols of UKB vision measures have been described previously.28 Presenting visual acuity was measured by the logarithm of the minimum angle of resolution (logMAR) chart (Precision Vision, LaSalle, IL, USA) at a distance of 4 meters or 1 meter if the participant cannot read the largest letters. Individuals read the letters from the top sequentially, and the lines identified correctly were converted into logMAR units. The test terminated when two letters or more were identified incorrectly. A visual acuity worse than 0.3 logMAR units in the better eye (if both are available) or the only eye with available data were determined as visual impairment in the present study. We have further divided VI into four categories based on severity, ranging between no VI, mild VI (logMAR >0.3 to 0.48), moderate VI (logMAR >0.48 to 1.0) and severe VI and worse (logMAR >1.0).29
Cognitive function and cognitive impairment ascertainment
Basic cognitive function was tested using a computerized program with a touchscreen interface and automated scoring. The UK Biobank cognitive impairment tests have been described and validated elsewhere.30 In brief, two cognitive tests (reaction time and pair matching) were included in the baseline protocol and three additional cognitive tests (fluid intelligence/reasoning, prospective memory and numeric memory) were added halfway to the baseline recruitment phase, but numeric memory was removed due to time constraints.30 Therefore, consistent with previous research,31 we used four tests including prospective memory, pair matching, fluid intelligence/reasoning, and reaction time to characterize cognitive function at baseline. Prospective memory test required the participants to complete the task “At the end of the games we will show you four colored symbols and ask you to touch the blue square. However, to test your memory, we want you to actually touch the Orange Circle instead.” A failed test of prospective memory was defined as incorrect recall of the command in the first attempt. Pair matching test showed the participants random cards with matching symbols. Participants were asked to memorize the position of the matching pairs and click on the pairs when the cards were turned over. More than two false matches of the paired cards was defined as failure in this test. Fluid intelligence/reasoning required the participants to select the correct choice to the numeric and verbal questions. Failure in this task is defined as less than three correct answers. In the reaction time test, participants completed symbol matching and the mean response time in milliseconds was documented. A reaction time of more than 770 milliseconds was defined as a failure in this test. Failure in one or more of the four tests indicated deficits in certain domain(s) of cognitive function and was defined as cognitive impairment in this study. For the severity of cognitive impairment, we have divided it into five categories, ranging from zero to four, based on the number of failed tests.
Covariates
The common covariates known to be associated with visual and cognitive impairment are listed as follows: age (continuous), gender (male/female), ethnicity (white/others), diabetes mellitus (previous/never), systolic blood pressure (currently/never on antihypertensive treatments), smoking status (never/prior and current), body mass index (BMI) (continuous), and hyperlipidemia (yes/no). In addition, education (with/without College or University degree), history of coronary heart disease (CHD) (yes/no), the presence of APOE ɛ4 allele(s) (yes/no) and taking psychotropic medications (yes/no) was adjusted for the cognitive impairment analysis.32–35
Diabetes mellitus was defined by participant-reported doctor-diagnosed diabetes, use of glucose-lowering medications, or with an HbA1c ≥6.5%. Antihypertensive treatment was defined by participant-reported treatment on antihypertensives. Participant-reported age when angina or heart attack were diagnosed was collected at baseline. An age of diagnosis younger than the baseline age was defined as prior CHD. Psychotropic medications include anti-depressants, anxiolytics and migraine pharmacological treatment.
Statistical analyses
We reported categorical variables with number and percentage, and continuous variables with mean and standard deviation (SD). Pearson’s chi-square test and unpaired t-test were used to examine the categorical and continuous variables, respectively. The association between visual and cognition impairment was analyzed using logistic regression models and estimated by odds ratio (OR) with a 95% confidence interval (CI). We adjusted for age, gender, and ethnicity only in Model I. In addition to the covariates adjusted for in Model I, Model II also adjusted for attainable education, APOE ɛ4 status, systolic blood pressure, the current treatment of hypertension, history of diabetes mellitus, smoking status, history of CHD, hyperlipidemia, psychotropic medications, and BMI. Two-sided p values of less than 0.05 were considered statistically significant for the aforementioned tests.
All metabolites were natural log-transformed (ln[x+1]) and then Z-transformed. To examine the associations between circulating metabolites and functional impairments, we performed univariable and multivariable logistic regressions. The multivariable model adjusted for age, gender, ethnicity, smoking status, systolic blood pressure, treatment of hypertension, history of diabetes mellitus, BMI, and hyperlipidemia for both functional impairments. For metabolite associations with cognitive impairment, we further adjusted for education, APOE ɛ4 status, psychotropic medications, and coronary heart disease. To explore the relationship between metabolite levels (continuous variables) and the severity of VI and cognitive impairment (ordinal categorical variables), we adopted polyserial correlation coefficients. For the associations or correlations between metabolites and visual or cognitive impairment, a p value less than 0.05 was considered as nominally significant. Due to the high potential for covariance between metabolites, principal component analyses were performed in order to extract the most salient features and mitigate the effect of redundant information causing an overfitted model.36 The results showed that 55 principal components were able to account for 99.5% of the variations, hence a corrected p value of 9×10–4 for multiple testing correction, was deemed statistically significant. Multivariate mediation analysis was performed to examine the indirect effect of VI in the association between metabolic alterations and cognitive impairment. Sobel-Goodman mediation tests were used to examine the mediation effects. A p value of <0.05 for the indirect effect suggests a significant mediation effect.
All analyses were conducted using Stata version 13 (StataCorp LLC, College Station, TX, USA) and R version 4.4.0 (R Foundation for Statistical Computing, Vienna, Austria).
RESULTS
Study sample
Of the total 23,775 participants meeting the inclusion criteria (age mean[SD]: 56.8[8.12], 54.2% female), 771 (3.24%) participants had VI and 7,341 (30.9%) participants had cognitive impairment at baseline. The baseline characteristics of participants stratified by VI and cognitive impairment are shown in Tables 1 and 2, respectively.
Table 1
Baseline characteristics of participants stratified by visual impairment
Baseline characteristics | Total | Non-visual impairment | Visual impairment | p |
N | 23,775 | 23,004 | 771 | |
Age, mean (SD), y | 56.8 (8.12) | 56.7 (8.13) | 58.8 (7.60) | <0.001 |
Gender, N (%) | ||||
Female | 12,896 (54.2) | 12,460 (54.2) | 436 (56.6) | |
Male | 10,879 (45.8) | 10,544 (45.8) | 335 (43.5) | 0.191 |
Ethnicity, N (%) | ||||
White | 21,516 (90.5) | 20,854 (90.7) | 662 (85.9) | |
Others | 2,259 (9.5) | 2,150 (9.35) | 109 (14.1) | <0.001 |
Smoking status, N (%) | ||||
Never | 13,168 (55.6) | 12,737 (55.6) | 431 (56.3) | |
Prior/current | 10,519 (44.4) | 10,185 (44.4) | 334 (43.7) | 0.672 |
History of diabetes mellitus, N (%) | ||||
No | 22,279 (93.7) | 21,580 (93.8) | 699 (90.7) | |
Yes | 1,496 (6.29) | 1,424 (6.19) | 72 (9.34) | <0.001 |
Cholesterol level, mean (SD), mmol/L | 5.69 (1.14) | 5.69 (1.14) | 5.65 (1.17) | 0.355 |
Systolic blood pressure, mean (SD), mmHg | 137.1 (18.2) | 137.0 (18.2) | 139.5 (18.7) | <0.001 |
Currently on anti-hypertensive treatments, N (%) | ||||
No | 21,517 (90.5) | 20,850 (90.6) | 667 (86.5) | |
Yes | 2,258 (9.50) | 2,154 (9.36) | 104 (13.5) | <0.001 |
BMI, mean (SD), kg/m2 | 27.4 (4.75) | 27.4 (4.73) | 28.0 (5.25) | <0.001 |
Hyperlipidemia, N (%) | ||||
No | 12,736 (53.6) | 12,347 (53.7) | 389 (50.5) | |
Yes | 11,039 (46.4) | 10, 657 (46.3) | 382 (49.6) | 0.078 |
BMI, body mass index; SD, standard deviation.
Table 2
Baseline characteristics of participants stratified by cognitive impairment
Baseline characteristics | Total | No cognitive impairment | Cognitive impairment | p |
N | 23,775 | 16,434 | 7,341 | |
Age, mean (SD), y | 56.8 (8.12) | 56.1 (8.10) | 58.2 (7.98) | <0.001 |
Gender, N (%) | ||||
Female | 12,896 (54.2) | 8,787 (53.5) | 4,109 (56.0) | |
Male | 10,879 (45.8) | 7,647 (46.5) | 3,232 (44.0) | <0.001 |
Ethnicity, N (%) | ||||
White | 21,516 (90.5) | 15,608 (95.0) | 5,908 (80.5) | |
Others | 2,259 (9.5) | 826 (5.03) | 1,433 (19.5) | <0.001 |
Education, N (%) | ||||
College/University degree | 8,340 (35.1) | 6,469 (39.4) | 1,871 (25.5) | |
Without College/University degree | 15,435 (64.9) | 9,965 (60.6) | 5,470 (74.5) | <0.001 |
APOE ɛ4, N (%) | ||||
Absent | 18,054 (76.5) | 12,434 (76.2) | 5,620 (77.1) | |
Present | 5,546 (23.5) | 3,880 (23.8) | 1,666 (22.9) | 0.125 |
Systolic blood pressure, mean (SD), mmHg | 137.1 (18.2) | 136.6 (18.0) | 138.2 (18.5) | <0.001 |
Currently on anti-hypertensive treatments, N (%) | ||||
No | 21,517 (90.5) | 15,067 (91.7) | 6,450 (87.9) | |
Yes | 2,258 (9.50) | 1,367 (8.32) | 891 (12.14) | <0.001 |
History of diabetes mellitus, N (%) | ||||
No | 22,279 (93.7) | 15,570 (94.7) | 6,709 (91.4) | |
Yes | 1,496 (6.29) | 864 (5.26) | 632 (8.61) | <0.001 |
Smoking status, N (%) | ||||
Never | 13,168 (55.6) | 9,125 (55.7) | 4,043 (55.4) | |
Prior/current | 10,519 (44.4) | 7,267 (44.3) | 3,252 (44.6) | 0.725 |
History of CHD, N (%) | ||||
No | 22,898 (96.3) | 15,901 (96.8) | 6,997 (95.3) | |
Yes | 877 (3.69) | 533 (3.24) | 344 (4.69) | <0.001 |
BMI, mean (SD), kg/m2 | 27.4 (4.75) | 27.3 (4.66) | 27.7 (4.93) | <0.001 |
Hyperlipidemia, N (%) | ||||
No | 12,736 (53.6) | 9,037 (55.0) | 3,699 (50.4) | |
Yes | 11,039 (46.4) | 7,397 (45.0) | 3,642 (49.6) | <0.001 |
Use of psychotropic medication, N (%) | ||||
No | 22,017 (92.6) | 15,302 (93.1) | 6,715 (91.5) | |
Yes | 1,758 (7.39) | 1,132 (6.89) | 626 (8.53) | <0.001 |
CHD, coronary heart disease; BMI, body mass index; SD, standard deviation.
Phenotype association
Table 3 shows the association between VI and cognitive impairment. In the age-, gender- and ethnicity-adjusted model, VI was associated with cognitive impairment (OR = 1.54, 95% CI: 1.32–1.79, p < 0.001). After adjusting for sociodemographic factors, chronic diseases, APOE ɛ4 status, and other confounding factors, the association remains significant (OR = 1.49, 95% CI: 1.27–1.74, p < 0.001).
Table 3
Association between VI and cognitive impairment
Visual impairment status | Model Ia | Model IIb | ||
OR (95% CI) | p | OR (95% CI) | p | |
Non-VI | 1 [Reference] | 1 [Reference] | ||
VI | 1.54 (1.32,1.79) | <0.001 | 1.49 (1.27,1.74) | <0.001 |
VI, visual impairment; OR, odds ratio; CI, confidence interval. aModel I adjusted for age, gender and ethnicity. bModel II adjusted for age, gender, ethnicity, attainable education, APOE ɛ4 status, systolic blood pressure, current treatment of hypertension, history of diabetes mellitus, smoking status, history of coronary heart disease, hyperlipidemia, body mass index and psychotropic medications.
Distinct and overlapping circulating metabolites associated with VI and cognitive impairment
The univariate association of circulating metabolites with VI and cognitive impairment is shown in Supplementary Table 3. The results for the multivariable model are shown in Table 4 and Fig. 1. At a p value less than 0.05, 12 metabolites or indexes (ratios of metabolites) spanning phospholipids to total lipids ratio in large LDL (OR = 1.08, 95% CI: 1.01–1.15, p = 0.023), omega-3 fatty acids (FAs) (OR = 0.84, 95% CI: 0.77–0.91, p < 0.001), ratio of omega-3 FAs to total FAs (OR = 0.84, 95% CI: 0.77–0.91, p < 0.001), docosahexaenoic acid (DHA) (OR = 0.85, 95% CI: 0.78–0.92, p < 0.001), free cholesterol to total lipids ratio in very small VLDL (OR = 1.09, 95% CI: 1.01–1.18, p = 0.032), ratio of omega-6 FAs to omega-3 FAs (OR = 1.18, 95% CI: 1.10–1.27, p < 0.001), ratio of DHA to total FAs (OR = 0.86, 95% CI: 0.80–0.94, p < 0.001), ratio of omega-6 FAs to total FAs (OR = 1.11, 95% CI: 1.01–1.22, p = 0.031), triglycerides in medium HDL (OR = 0.91, 95% CI: 0.84–0.99, p = 0.021), triglycerides in large HDL (OR = 0.90, 95% CI: 0.83–0.98, p = 0.010), triglycerides in HDL (OR = 0.91, 95% CI: 0.84–0.99, p = 0.024) and acetoacetate (OR = 1.08, 95% CI: 1.01–1.17, p = 0.033) were uniquely associated with VI.
Table 4
Circulating metabolites associated with VI/cognitive impairment after multiple testing corrections
Metabolites | OR (95% CI) | pa |
VI | ||
Albumin | 0.90 (0.85,0.95) | 1.47×10–4 |
Ratio of omega-6 fatty acids to omega-3 fatty acids | 1.18 (1.10,1.27) | 6.29×10–6 |
Ratio of omega-3 fatty acids to total fatty acids | 0.84 (0.77,0.91) | 1.61×10–5 |
Ratio of docosahexaenoic acid to total fatty acids | 0.86 (0.80,0.94) | 4.03×10–4 |
Docosahexaenoic Acid | 0.85 (0.78,0.92) | 6.75×10–5 |
Omega-3 Fatty Acids | 0.84 (0.77,0.91) | 1.91×10–5 |
Cognitive impairment | ||
Albumin | 0.95 (0.92,0.98) | 4.84×10–4 |
Glycoprotein Acetyls | 1.06 (1.03,1.10) | 1.24×10–4 |
Alanine | 0.95 (0.92,0.98) | 7.86×10–4 |
aAfter multiple testing corrections.
Fig. 1
Forest plot for metabolite associations with visual impairment and cognitive impairment. This forest plot shows the estimated odds ratios (OR) and 95% confidence intervals (CIs) for the associations of distinct and overlapping circulating metabolites with visual impairment shown in green and cognitive impairment shown in purple.
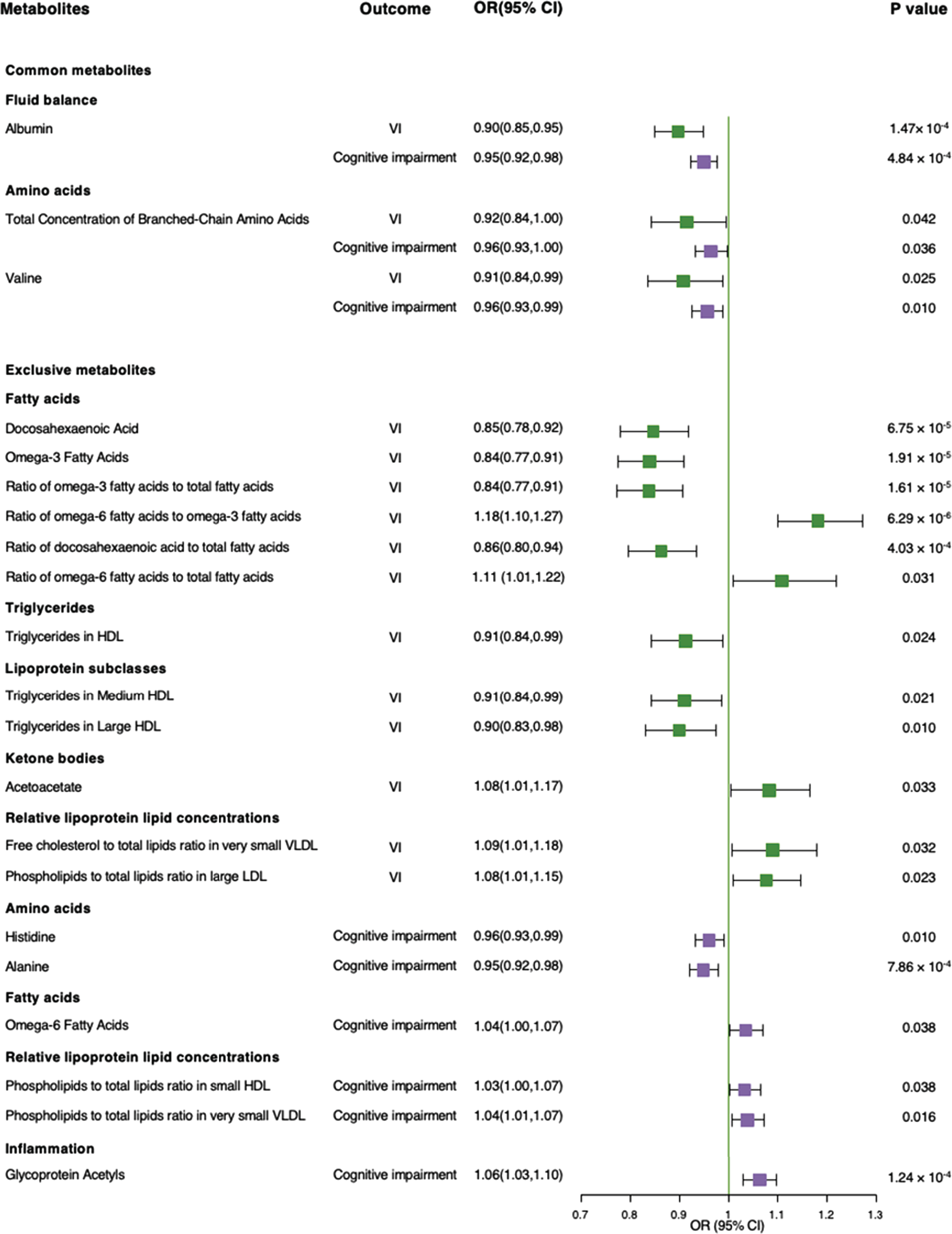
Six metabolites or indexes, including phospholipids to total lipids ratio in small HDL (OR = 1.03, 95% CI: 1.00–1.07, p = 0.038), phospholipids to total lipids ratio in very small VLDL (OR = 1.04, 95% CI: 1.01–1.07, p = 0.016), alanine (OR = 0.95, 95% CI: 0.92–0.98, p < 0.001), glycoprotein acetyls (OR = 1.06, 95% CI: 1.03–1.10, p < 0.001), omega-6 FAs (OR = 1.04, 95% CI: 1.00–1.07, p = 0.038), histidine (OR = 0.96, 95% CI: 0.93–0.99, p = 0.010) were uniquely associated with cognitive impairment. A total of three metabolites spanning branched-chain amino acids [BCAAs], valine and albumin were found to be common across these two phenotypes (Fig. 1).
After correction for multiple testing (Table 4), the ratio of omega-6 to omega-3 FAs was positively associated with VI (OR = 1.18, 95% CI = 1.10–1.27, P = 6.29×10–6) and four metabolites or indexes were inversely associated with VI (OR = 0.84, 95% CI = 0.77–0.91, p = 1.61×10–5 for ratio of omega-3 FAs to total FAs; OR = 0.86, 95% CI = 0.80–0.94, p = 4.03×10–4 for ratio of DHA to total FAs; OR = 0.85, 95% CI = 0.78–0.92, p = 6.75×10–5 for DHA; and OR = 0.84, 95% CI = 0.77–0.91, p = 1.91×10–5 for omega-3 FAs). With respect to cognitive impairment, glycoprotein acetyls showed a positive association (OR = 1.06, 95% CI = 1.03–1.10, p = 1.24×10–4) while alanine showed a negative association (OR = 0.95, 95% CI: 0.92–0.98, p = 7.86×10–4). Albumin was identified as the common metabolite inversely associated with both VI and cognitive impairment (OR = 0.90, 95% CI: 0.85–0.95, p = 1.47×10–4 for VI, and OR = 0.95, 95% CI: 0.92–0.98, p = 4.84×10–4 for cognitive impairment). Similar results were found in the polyserial correlation between metabolites and severity of VI and cognitive impairment (Supplementary Table 4). Albumin remained the only metabolite that was negatively correlated with the severity of both VI and cognitive impairment after multiple testing correction.
Mediation effects
Among the metabolites that were associated with both VI and cognitive impairment, we found a significant medication effect of VI of 3.6% (p = 0.023) in the association between total concentration of BCAAs and cognitive impairment. The mediation effects of VI in the respective association of valine and albumin with cognitive impairment were 3.3% (p = 0.013) and 3.7% (p = 0.002).
DISCUSSION
We validated in the present study that VI was significantly associated with cognitive impairment based on the UK Biobank dataset. Further, using high-throughput metabolomics data, we demonstrated that VI and cognitive impairment have metabolic signatures that are, in part, shared (albumin, BCAAs, and valine) but also unique to each phenotype. These findings suggested that disrupted fluid and electrolyte balance (albumin) and disrupted protein anabolism (BCAAs including valine) are the common factors that contribute to VI and cognitive impairment. Moreover, part of the associations between these shared metabolites and cognitive impairment could be mediated by VI. For metabolites exclusively associated with VI or cognitive impairment, they added to the limited knowledge of metabolic profiles of the phenotypes and may provide novel biomarkers.
To the best of our knowledge, this is the first study that characterized the metabolic associations with VI and cognitive impairment. The plausible mechanisms under the metabolite associations may be explained by evidence from previous studies. Omega-3 FAs and DHA are essential nutrients to vision health.37,38 Omega-3 FAs are protective against inflammation, oxidative stress, and neovascularization which are commonly involved in the pathogenesis of ophthalmic diseases such as age-related macular degeneration and diabetic retinopathy.39–41 DHA is one of the primary type of omega-3 FA that is critical in maintaining the photoreceptor membrane function, visual processing capacity, retinal cell signaling, and retinal gene expression and differentiation.42 Plasma DHA was also found to be able to cross the blood-retina barrier given the availability of specific transporters.43 This not only supported the observed negative association of omega-3 and DHA with VI, but also partially supported our findings that higher ratio of omega-3 and DHA in total FAs would be protective against VI. In contrast, the ratio of omega-6 FAs to total omega-3 FAs exhibited a positive association with VI and ratio of omega-6 FAs to total FAs also exhibited nominal significance. Particularly, excessive omega-6 FAs could exert oxidative stress, which may lead to endothelial dysfunction of the retinal vasculature. An unbalanced ratio of omega-6 FAs to omega-3 FAs has also become an emerging biomarker for systemic inflammation and chronic diseases.44,45 Growing evidence has suggested an interplay between ocular diseases and altered lipid or lipoprotein profiles,46–48 suggesting the differential effects of lipoprotein subclasses and particle sizes in mediating visual-threatening ocular diseases. Our findings also demonstrated differed effect of different sizes and components of lipoprotein subclasses. As these metabolites only demonstrated nominal significance, further studies are needed to validate the effect of these metabolites.
As for the metabolites exclusively associated with cognitive impairment, glycoprotein acetyls are an emerging biomarker for systemic inflammation and predictor of severe infection,49 cardiovascular diseases,50 diabetes,51 and autoimmune diseases.52 These conditions are risk factors for cognitive impairment or dementia,53–56 thus explaining the positive association between glycoprotein acetyls and cognitive impairment. The potential protective role of alanine has been evidenced by its rescue of cognitive function in mice with Alzheimer’s disease.57 In contrast, omega-6 FAs were identified as a risk factor for cognitive impairment in the present study, probably due to the pro-inflammatory effects of arachidonic acid.58,59 In addition, our findings suggested the potential detrimental role of multiple phospholipid constituents of lipoprotein subclasses in cognitive ability. Specifically, phospholipid levels may be altered as the pathological process of Alzheimer’s disease progresses,60 which might explain the association between phospholipids to total lipids ratio in small HDL and very small VLDL and cognitive decline.
The overlapping metabolites shared by both VI and cognitive impairment provided insights into the common factors that contribute to the association between these two impairments. Albumin is the metabolite that showed significant inverse association with both VI and cognitive impairment. As an essential nutrient comprising the body protein pool,61 serum albumin is the major substrate for Aβ sequestering, and hypoalbuminemia may promote amyloid accumulation and thus increase the risk of brain Aβ deposition in individuals without dementia.62 Lower levels of serum albumin contribute to visual impairment plausibly by increasing the risk of developing cataracts due to the lack of required nutrients for the lens.63 BCAAs, including valine, leucine, and isoleucine, also showed negative association with both functional impairments at nominal significance, probably because it is essential in maintaining normal neurological functions as a critical component for neurotransmitter synthesis.64 Taken together, as the retina is anatomically and embryologically an extension of the central nervous system, deficits in the metabolites that play neuroprotective roles could contribute to the impairment in both the central nervous system and the vision system. As evidenced in previous studies, BCAA metabolic dysfunction would be implicated in both neurological diseases (cerebrovascular diseases and Alzheimer’s disease) and retinal degenerative diseases.65,66
Our findings of the VI-cognitive impairment cross-sectional association was observed in previous longitudinal studies, suggesting VI is a significant risk factor for cognitive impairment.10–16 One theory focuses on cognitive load, proposing that VI individuals required more cognitive resources to proceed visual information and compromise the brain’s resilience to external stress.19 In addition, VI may lead to social isolation and physical inactivity, which are established risk factors for cognitive impairment.18 Another theory was the common cause theory that shared risk factors or pathophysiology may contribute to both functional impairments.14 Our findings of the overlapping metabolites shared by VI and cognitive impairment potentially provided evidence on the molecular level for the common cause theory.
In addition, the results need to be interpreted taking into account the cognitive impairment definition in this study. As cognitive impairment is a broad term and different definitions were used in various studies,14,67–69 we adopted the definition of failure in one or more of the cognitive tests in this study indicative of deficits in different cognitive domains.68,69 This results in a relatively high prevalence of cognitive impairment in this study and it warrants to be highlighted that this prevalence represents the proportion of individuals with impaired cognitive function indexed by cognitive performance deficits in at least one cognitive domain.
Strengths and limitations
The strengths of the present study included a large sample size, the standardized metabolomics platform for the measurement of all samples, and the full adjustment of confounding factors. However, there are also several limitations to mention. Firstly, the cross-sectional design of this study prevented us from knowing the longitudinal relationship between the metabolites and functional impairments. Further exploration is needed to confirm the findings through longitudinal studies or interventional studies. Secondly, the participants of the UK Biobank predominantly consisted of white ethnicity and of better overall health, and the selected sample had slightly different baseline characteristics from the whole UK Biobank sample. The potential selection bias may limit the generalizability of the results. However, the associations between the metabolites and health outcomes were not affected.70 Lastly, medications may affect the metabolic profile. Although we have adjusted for lipid-lowering medications, diabetic medications, and psychotropic medications, we cannot exclude the possible residual confounders. Further studies in external datasets are warranted to validate the findings from our study.
In conclusion, using the high-throughput metabolomics data of the UK Biobank, we identified distinct metabolites associated with VI and cognitive impairment, which added to the knowledge to understand the molecular basis under VI and cognitive impairment. In addition, the overlapping metabolites found to be associated with both VI and cognitive impairment provided insights into the mechanisms underlying the association between the two phenotypes.
AUTHOR CONTRIBUTIONS
Wenyi Hu (Conceptualization; Formal analysis; Investigation; Methodology; Writing – original draft); Tiancheng Chu (Writing – review & editing); Huan Liao (Writing – review & editing); Wei Wang (Conceptualization; Funding acquisition; Investigation; Resources; Writing – review & editing); Jason Ha (Writing – review & editing); Katerina Kiburg (Writing – review & editing); Xiayin Zhang (Writing – review & editing); Xianwen Shang (Data curation; Supervision; Writing – review & editing); Yu Huang (Writing – review & editing); Xueli Zhang (Writing – review & editing); Shulin Tang (Writing – review & editing); Yijun Hu (Writing – review & editing); Honghua Yu (Funding acquisition; Writing – review & editing); Xiaohong Yang (Funding acquisition; Writing – review & editing); Mingguang He (Funding acquisition; Project administration; Resources; Supervision; Writing – review & editing); Lisa Zhu (Conceptualization; Formal analysis; Funding acquisition; Investigation; Project administration; Resources; Supervision; Validation; Writing – original draft; Writing – review & editing).
ACKNOWLEDGMENTS
The sponsor or funding organization had no role in the design or conduct of this research.
FUNDING
This project received grant funding from the Australian Government: the National Critical Research Infrastructure Initiative, Medical Research Future Fund (MRFAI00035) and the NHMRC Investigator Grant (APP1175405, APP2010072). The contents of the published material are solely the responsibility of the Administering Institution, a participating institution or individual authors and do not reflect the views of the NHMRC. This work was supported by the Global STEM Professorship Scheme (P0046113), the Fundamental Research Funds of the State Key Laboratory of Ophthalmology, Project of Investigation on Health Status of Employees in Financial Industry in Guangzhou, China (Z012014075), National Natural Science Foundation of China (82000901, 82101173, 81870663, 82171075), Outstanding Young Talent Trainee Program of Guangdong Provincial People’s Hospital (KJ012019087), Guangdong Provincial People’s Hospital Scientific Research Funds for Leading Medical Talents and Distinguished Young Scholars in Guangdong Province (KJ012019457), High-level Talent Flexible Introduction Fund of Guangdong Provincial People’s Hospital (KJ012019530), Talent Introduction Fund of Guangdong Provincial People’s Hospital (Y012018145), Science and Technology Program of Guangzhou, China (202002020049), Project of Special Research on Cardiovascular Diseases (2020XXG007), and Research Foundation of Medical Science and Technology of Guangdong Province (B2021237). The Centre for Eye Research Australia receives Operational Infrastructure Support from the Victorian State Government. W.H. is supported by the Melbourne Research Scholarship established by the University of Melbourne. The funding source had no role in the design and conduct of the study; collection, management, analysis, and interpretation of the data; preparation, review, or approval of the manuscript; and decision to submit the manuscript for publication.
CONFLICT OF INTEREST
The authors have no conflict of interest to report.
DATA AVAILABILITY
All data used in this study is available through application to the UK Biobank. The current study was conducted using UK Biobank data under Application ID 94372.
SUPPLEMENTARY MATERIAL
[1] The supplementary material is available in the electronic version of this article: http://dx.doi.org/10.3233/ADR-230154.
REFERENCES
1. | Plassman BL , Langa KM , Fisher GG , et al. Prevalence of cognitive impairment without dementia in the United States. Ann Intern Med (2008) ; 148: : 427–434. |
2. | Petersen RC , Roberts RO , Knopman DS , et al. Prevalence of mild cognitive impairment is higher in men. The Mayo Clinic Study of Aging. Neurology (2010) ; 75: : 889–897. |
3. | Unverzagt FW , Gao S , Baiyewu O , et al. Prevalence of cognitive impairment: data from the Indianapolis Study of Health and Aging. Neurology (2001) ; 57: : 1655–1662. |
4. | Causes of blindness and vision impairment in 2020 and trends over 30 years, and prevalence of avoidable blindness in relation to VISION 2020: the Right to Sight: an analysis for the Global Burden of Disease Study. Lancet Global Health (2021) ; 9: : e144–e160. |
5. | Bourne RRA , Flaxman SR , Braithwaite T , et al. Magnitude, temporal trends, and projections of the global prevalence of blindness and distance and near vision impairment: a systematic review and meta-analysis. Lancet Global Health (2017) ; 5: : e888–e897. |
6. | Bárrios H , Narciso S , Guerreiro M , et al. Quality of life in patients with mild cognitive impairment. Aging Mental Health (2013) ; 17: : 287–292. |
7. | Whitson HE , Cousins SW , Burchett BM , et al. The combined effect of visual impairment and cognitive impairment on disability in older people. J Am Geriatr Soc (2007) ; 55: : 885–891. |
8. | Patel N , Stagg BC , Swenor BK , et al. Association of co-occurring dementia and self-reported visual impairment with activity limitations in older adults. JAMA Ophthalmol (2020) ; 138: : 756–763. |
9. | Murray CJL , Vos T , Lozano R , et al. Disability-adjusted life years (DALYs) for 291 diseases and injuries in 21 regions, 1990-2010: a systematic analysis for the Global Burden of Disease Study 2010. Lancet (2012) ; 380: : 2197–2223. |
10. | Shang X , Zhu Z , Wang W , et al. The association between vision impairment and incidence of dementia and cognitive impairment: a systematic review and meta-analysis. Ophthalmology (2021) ; 128: : 1135–1149. |
11. | Lim ZW , Chee M-L , Soh ZD , et al. Association between visual impairment and decline in cognitive function in a multiethnic Asian population. JAMA Netw Open (2020) ; 3: : e203560. |
12. | Varadaraj V , Munoz B , Deal JA , et al. Association of vision impairment with cognitive decline across multiple domains in older adults. JAMA Netw Open (2021) ; 4: : e2117416. |
13. | Ong SY , Cheung CY , Li X , et al. Visual impairment, age-related eye diseases, and cognitive function: the Singapore Malay Eye study. Arch Ophthalmol (2012) ; 130: : 895–900. |
14. | Fischer ME , Cruickshanks KJ , Schubert CR , et al. Age-related sensory impairments and risk of cognitive impairment. J Am Geriatr Soc (2016) ; 64: : 1981–1987. |
15. | Hajek A , Brettschneider C , Lühmann D , et al. Effect of visual impairment on physical and cognitive function in old age: findings of a population-based prospective cohort study in Germany. J Am Geriatr Soc (2016) ; 64: : 2311–2316. |
16. | Zheng DD , Swenor BK , Christ SL , et al. Longitudinal associations between visual impairment and cognitive functioning: The Salisbury Eye Evaluation Study. JAMA Ophthalmol (2018) ; 136: : 989–995. |
17. | Shang X , Zhu Z , Wang W , et al. The association between vision impairment and incidence of dementia and cognitive impairment: a systematic review and meta-analysis. Ophthalmology (2021) ; 128: : 1135–1149. |
18. | Clemons TE , Rankin MW and McBee WL. Cognitive impairment in the Age-Related Eye Disease Study: AREDS report no 16. Arch Ophthalmol (2006) ; 124: : 537–543. |
19. | Uhlmann RF , Larson EB , Koepsell TD , et al. Visual impairment and cognitive dysfunction in Alzheimer’s disease. J Gen Intern Med (1991) ; 6: : 126–132. |
20. | Patti GJ , Yanes O and Siuzdak G. Innovation: Metabolomics: the apogee of the omics trilogy. Nat Rev Mol Cell Biol (2012) ; 13: : 263–269. |
21. | Collino S , Martin F-PJ and Rezzi S. Clinical metabolomics paves the way towards future healthcare strategies. Br J Clin Pharmacol (2013) ; 75: : 619–629. |
22. | Moco S , Collino S , Rezzi S , et al. Metabolomics perspectives in pediatric research. Pediatr Res (2013) ; 73: : 570–576. |
23. | Shah SH , Kraus WE and Newgard CB. Metabolomic profiling for the identification of novel biomarkers and mechanisms related to common cardiovascular diseases: form and function. Circulation (2012) ; 126: : 1110–1120. |
24. | Wilkins JM and Trushina E. Application of metabolomics in Alzheimer’s disease. Front Neurol (2017) ; 8: : 719. |
25. | Sudlow C , Gallacher J , Allen N , et al. UK biobank: an open access resource for identifying the causes of a wide range of complex diseases of middle and old age. PLoS Med (2015) ; 12: : e1001779. |
26. | Julkunen H , Cichonska A , Tiainen M , et al. Atlas of plasma NMR biomarkers for health and disease in 118,461 individuals from the UK Biobank. Nat Commun (2023) ; 14: : 604. |
27. | Emwas AH , Roy R , McKay RT , et al. NMR spectroscopy for metabolomics research. Metabolites (2019) ; 9: : 123. |
28. | Chua SYL , Thomas D , Allen N , et al. Cohort profile: design and methods in the eye and vision consortium of UK Biobank. BMJ Open (2019) ; 9: : e025077. |
29. | Killeen OJ , Zhou Y and Ehrlich JR. Objectively measured visual impairment and dementia prevalence in older adults in the US. JAMA Ophthalmol (2023) ; 141: : 786–790. |
30. | Lyall DM , Cullen B , Allerhand M , et al. Cognitive test scores in UK Biobank: Data reduction in 480,416 participants and longitudinal stability in 20,346 participants. PloS One (2016) ; 11: : e0154222. |
31. | Ko F , Muthy ZA , Gallacher J , et al. Association of retinal nerve fiber layer thinning with current and future cognitive decline a study using optical coherence tomography. JAMA Neurol (2018) ; 75: : 1198–1205. |
32. | Gharbi-Meliani A , Dugravot A , Sabia S , et al. The association of APOE epsilon4 with cognitive function over the adult life course and incidence of dementia: 20 years follow-up of the Whitehall II study. Alzheimers Res Ther (2021) ; 13: : 5. |
33. | Deckers K , Schievink SHJ , Rodriquez MMF , et al. Coronary heart disease and risk for cognitive impairment or dementia: Systematic review and meta-analysis. PLoS One (2017) ; 12: : e0184244. |
34. | Wang YC , Tai PA , Poly TN , et al. Increased risk of dementia in patients with antidepressants: a meta-analysis of observational studies. Behav Neurol (2018) ; 2018: : 5315098. |
35. | Mula M . Topiramate and cognitive impairment: evidence and clinical implications. Ther Adv Drug Saf (2012) ; 3: : 279–289. |
36. | Gao X , Starmer J and Martin ER. A multiple testing correction method for genetic association studies using correlated single nucleotide polymorphisms. Genet Epidemiol (2008) ; 32: : 361–369. |
37. | Neuringer M , Connor WE , Van Petten C , et al. Dietary omega-3 fatty acid deficiency and visual loss in infant rhesus monkeys. J Clin Invest (1984) ; 73: : 272–276. |
38. | Merle BMJ , Benlian P , Puche N , et al. Circulating omega-3 Fatty acids and neovascular age-related macular degeneration. Invest Ophthalmol Vis Sci (2014) ; 55: : 2010–2019. |
39. | Lawrenson JG and Evans JR. Omega 3 fatty acids for preventing or slowing the progression of age-related macular degeneration. Cochrane Database Syst Rev (2015) ; 2015: : CD010015. |
40. | Delyfer M-N , Buaud B , Korobelnik J-F , et al. Association of macular pigment density with plasma ω-3 fatty acids: the PIMAVOSA study. Invest Ophthalmol Vis Sci (2012) ; 53: : 1204–1210. |
41. | Behl T and Kotwani A. Omega-3 fatty acids in prevention of diabetic retinopathy. J Pharm Pharmacol (2017) ; 69: : 946–954. |
42. | SanGiovanni JP and Chew EY. The role of omega-3 long-chain polyunsaturated fatty acids in health and disease of the retina. Prog Retin Eye Res (2005) ; 24: : 87–138. |
43. | Sugasini D , Yalagala PCR and Subbaiah PV. Efficient enrichment of retinal DHA with dietary lysophosphatidylcholine-DHA: potential application for retinopathies. Nutrients (2020) ; 12: : 3114. |
44. | Shetty SS , Kumari NS and Shetty PK. ω-6/ω-3 fatty acid ratio as an essential predictive biomarker in the management of type 2 diabetes mellitus. Nutrition (2020) ; 79–80: : 110968. |
45. | DiNicolantonio JJ and O’Keefe JH. Importance of maintaining a low omega-6/omega-3 ratio for reducing inflammation. Open Heart (2018) ; 5: : e000946. |
46. | Lyons TJ , Jenkins AJ , Zheng D , et al. Diabetic retinopathy and serum lipoprotein subclasses in the DCCT/EDIC cohort. Invest Ophthalmol Vis Sci (2004) ; 45: : 910–918. |
47. | Chang Y-C and Wu W-C. Dyslipidemia and diabetic retinopathy. Rev Diabet Stud (2013) ; 10: : 121–132. |
48. | Betzler BK , Rim TH , Sabanayagam C , et al. High-density lipoprotein cholesterol in age-related ocular diseases. Biomolecules (2020) ; 10: : 645. |
49. | Ritchie SC , Würtz P , Nath AP , et al. The biomarker GlycA is associated with chronic inflammation and predicts long-term risk of severe infection. Cell Syst (2015) ; 1: : 293–301. |
50. | Connelly MA , Otvos JD , Shalaurova I , et al. GlycA, a novel biomarker of systemic inflammation and cardiovascular disease risk. J Transl Med (2017) ; 15: : 219. |
51. | Akinkuolie AO , Pradhan AD , Buring JE , et al. Novel protein glycan side-chain biomarker and risk of incident type 2 diabetes mellitus. Arterioscler Thromb Vasc Biol (2015) ; 35: : 1544–1550. |
52. | Durcan L , Winegar DA , Connelly MA , et al. Longitudinal evaluation of lipoprotein variables in systemic lupus erythematosus reveals adverse changes with disease activity and prednisone and more favorable profiles with hydroxychloroquine therapy. J Rheumatol (2016) ; 43: : 745–750. |
53. | Katan M , Moon YP , Paik MC , et al. Infectious burden and cognitive function: the Northern Manhattan Study. Neurology (2013) ; 80: : 1209–1215. |
54. | Leritz EC , McGlinchey RE , Kellison I , et al. Cardiovascular disease risk factors and cognition in the elderly. Curr Cardiovasc Risk Rep (2011) ; 5: : 407–412. |
55. | Luchsinger JA , Reitz C , Patel B , et al. Relation of diabetes to mild cognitive impairment. Arch Neurol (2007) ; 64: : 570–575. |
56. | Oláh C , Schwartz N , Denton C , et al. Cognitive dysfunction in autoimmune rheumatic diseases. Arthritis Res Ther (2020) ; 22: : 78. |
57. | Herculano B , Tamura M , Ohba A , et al. β-alanyl-L-histidine rescues cognitive deficits caused by feeding a high fat diet in a transgenic mouse model of Alzheimer’s disease. J Alzheimers Dis (2013) ; 33: : 983–997. |
58. | Tsoukalas D , Alegakis AK , Fragkiadaki P , et al. Application of metabolomics part II: Focus on fatty acids and their metabolites in healthy adults. Int J Mol Med (2019) ; 43: : 233–242. |
59. | Innes JK and Calder PC. Omega-6 fatty acids and inflammation. Prostaglandins Leukot Essent Fatty Acids (2018) ; 132: : 41–48. |
60. | Kosicek M and Hecimovic S. Phospholipids and Alzheimer’s disease: alterations, mechanisms and potential biomarkers. Int J Mol Sci (2013) ; 14: : 1310–1322. |
61. | Omran ML and Morley JE. Assessment of protein energy malnutrition in older persons, Part II: Laboratory evaluation. Nutrition (2000) ; 16: : 131–140. |
62. | Kim JW , Byun MS , Lee JH , et al. Serum albumin and beta-amyloid deposition in the human brain. Neurology (2020) ; 95: : e815–e826. |
63. | Delcourt C , Dupuy A-M , Carriere I , et al. Albumin and transthyretin as risk factors for cataract: the POLA study. Arch Ophthalmol (2005) ; 123: : 225–232. |
64. | Shimomura Y and Kitaura Y. Physiological and pathological roles of branched-chain amino acids in the regulation of protein and energy metabolism and neurological functions. Pharmacol Res (2018) ; 133: : 215–217. |
65. | Polis B and Samson AO. Role of the metabolism of branched-chain amino acids in the development of Alzheimer’s disease and other metabolic disorders. Neural Regen Res (2020) ; 15: : 1460–1470. |
66. | Hasegawa T , Ikeda HO , Iwai S , et al. Branched chain amino acids attenuate major pathologies in mouse models of retinal degeneration and glaucoma. Heliyon (2018) ; 4: : e00544. |
67. | Dhakal A and Bobrin BD. Cognitive deficits. In StatPearls. Treasure Island (FL): StatPearls Publishing; (2023) . |
68. | Cullen B , Deary IJ , Evans JJ , et al. Understanding cognitive impairment in mood disorders: mediation analyses in the UK Biobank cohort. Br J Psychiatry (2019) ; 215: : 683–690. |
69. | Shen J , Tozer DJ , Markus HS , et al. Network efficiency mediates the relationship between vascular burden and cognitive impairment: a diffusion tensor imaging study in UK Biobank. Stroke (2020) ; 51: : 1682–1689. |
70. | Fry A , Littlejohns TJ , Sudlow C , et al. Comparison of sociodemographic and health-related characteristics of UK Biobank participants with those of the general population. Am J Epidemiol (2017) ; 186: : 1026–1034. |