Precipitating Mechanisms of Falls in Preclinical Alzheimer’s Disease
Abstract
Background:
Individuals with Alzheimer’s disease (AD) are more than twice as likely to incur a serious fall as the general population of older adults. Although AD is commonly associated with cognitive changes, impairments in other clinical measures such as strength or functional mobility (i.e., gait and balance) may precede symptomatic cognitive impairment in preclinical AD and lead to increased fall risk.
Objective:
To examine mechanisms (i.e., functional mobility, cognition, AD biomarkers) associated with increased falls in cognitively normal older adults.
Methods:
This 1-year study was part of an ongoing longitudinal cohort study. We examined the relationships among falls, clinical measures of functional mobility and cognition, and neuroimaging AD biomarkers in cognitively normal older adults. We also investigated which domain(s) best predicted fall propensity and severity through multiple regression models.
Results:
A total of 182 older adults were included (mean age 75 years, 53% female). A total of 227 falls were reported over the year; falls per person ranged from 0–16 with a median of 1. Measures of functional mobility were the best predictors of fall propensity and severity. Cognition and AD biomarkers were associated with each other but not with the fall outcome measures.
Conclusion:
These results suggest that, although subtle changes in cognition may be more closely associated with AD neuropathology, functional mobility indicators better predict falls in cognitively normal older adults. This study adds to our understanding of the mechanisms underlying falls in older adults and could lead to the development of targeted fall prevention strategies.
INTRODUCTION
Falls are a leading cause of injury, long-term disability, premature institutionalization, and injury-related death among older adults [1]. Falls are highly prevalent among community-dwelling older adults, with approximately 1 in 3 experiencing a fall each year [1]. Individuals with Alzheimer’s disease (AD) have more than twice the risk of serious falls compared to the general population of older adults [2, 3].
AD is a gradual, progressive condition that occurs over several decades. During the preclinical phase, neuropathological changes accumulate prior to the onset of cognitive changes and clinical diagnosis (i.e., while individuals remain cognitively normal [CN]). The preclinical stage of AD is defined by the presence of amyloidosis and neurodegeneration [4, 5] measured using neuroimaging techniques (i.e., MRI, PET), fluid biomarker analysis (i.e., cerebrospinal fluid, plasma), and subtle declines in cognition (i.e., episodic memory and attentional control) [6]. Approximately 46 million Americans, or 22% , are in the preclinical stage of AD [7, 8]. CN older adults with higher levels of amyloidosis or greater evidence of neurodegeneration are at even greater risk for symptomatic AD [7, 8]. A 1-year study noted that higher levels of PET amyloid were associated with an increased risk of falls in older adults with no cognitive impairment, that falls also occur at higher rates during the preclinical phase of AD, and that the mechanisms underlying AD-related cognitive decline may be associated with declines in gait and balance [9].
Falls have multiple potential precipitating mechanisms, such as impaired functional mobility (i.e., gait and balance), reductions in strength and sensation, lack of precautionary behavior for potential fall hazards, reduced functional capacity and performance of daily activities, adverse medication effects, changes in cognition, and neuropathological changes [3, 10]. While these many factors make falls a complex phenomenon to study, a growing body of work has identified that motor impairments (i.e., functional mobility) and subsequent falls may precede symptomatic cognitive impairment in older adults [9, 11–13]. While relationships among falls, functional mobility, and cognition have been established among CN older adults [1–3, 10–12], to our knowledge, there has been no examination among AD biomarkers, cognition, functional mobility, and prospectively collected falls data while controlling for known fall risk factors among CN older adults. A better understanding of meaningful clinical manifestations (e.g., falls, functional mobility, cognition) of underlying AD biomarkers among CN older adults is necessary to understand and characterize this early stage of the disease for the millions of older adults in this stage and those at greater risk for symptomatic AD [7, 8].
The goal of the present study was to 1) examine the relationship between AD biomarkers and falls, and 2) determine which mechanism(s) (i.e., cognition, functional performance of daily activities, strength, fall precautionary behaviors, or functional mobility) best predict fall propensity and severity among a cohort of CN older adults. We hypothesized that AD biomarkers would be associated with greater numbers of falls and that both functional mobility and cognition would predict falls. Elucidating the specific mechanisms associated with falls in preclinical AD could enhance the precision of future fall prevention intervention studies.
MATERIALS AND METHODS
Participants
Participants in studies at the Knight Alzheimer’s Disease Research Center (Knight ADRC) at Washington University in St. Louis were invited to participate in this study, a 4-year longitudinal observational study of fall risk, functional mobility, and AD. Individuals were invited to participate if they were over the age of 65, cognitively normal as indicated by a Clinical Dementia Rating ® (CDR) [14] score of 0 at the time of assessment, and underwent amyloid PET and MRI scans within 2 years of the CDR and cognitive assessments. Additional study details have been described elsewhere [15]. This study was approved by the Institutional Review Board at Washington University in St. Louis (201807135). All participants provided written informed consent. All data used in the present study are from the first year of the ongoing parent study (see Fig. 1 for timeline of study procedures).
Fig. 1
Timeline of study procedures. ADRC, Alzheimer’s Disease Research Center.
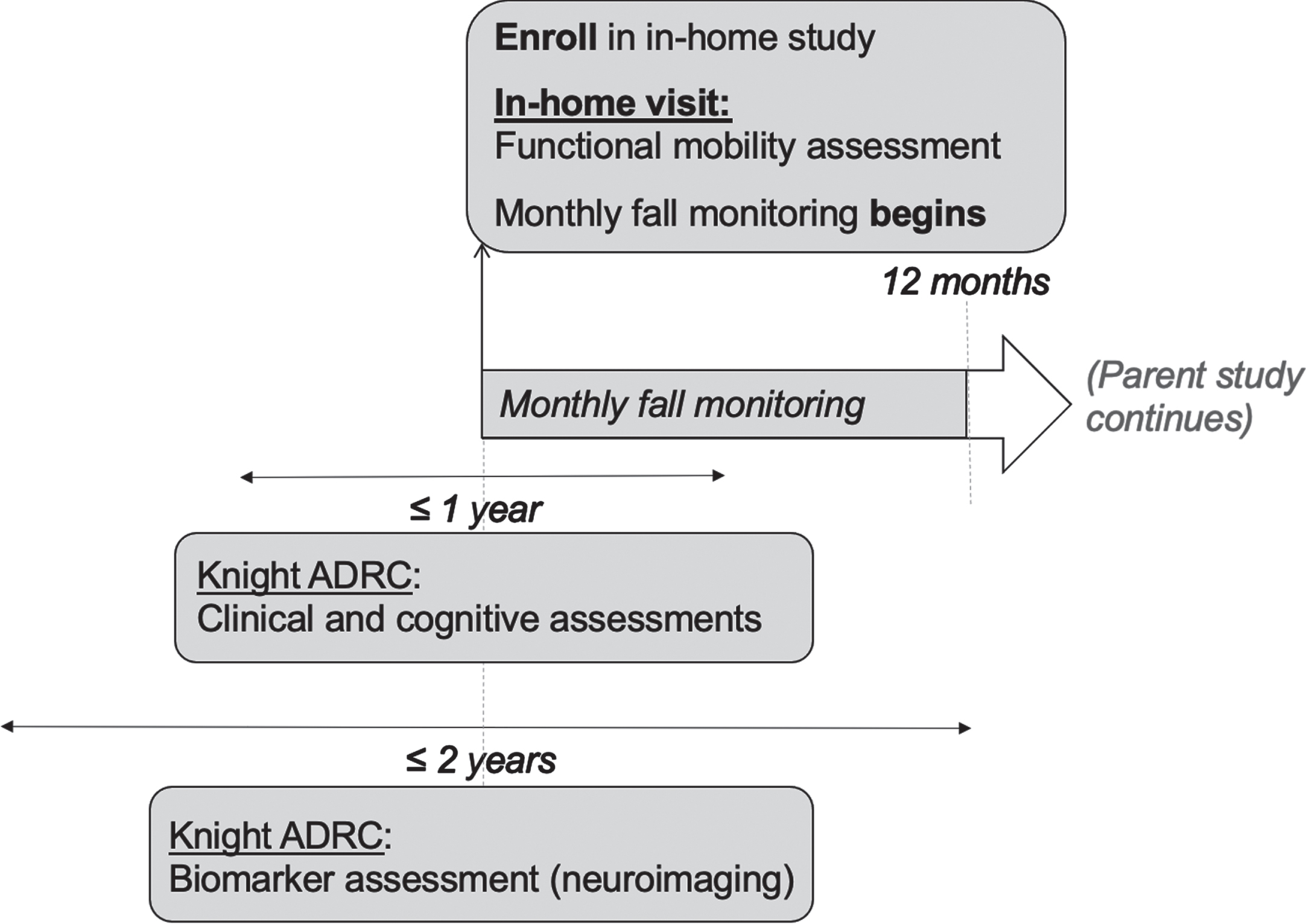
CDR status
The CDR uses a 5-point scale to characterize 6 domains of cognitive and functional performance that are applicable to AD and other dementias [14]. The domains include memory, orientation, judgment and problem solving, community affairs, home and hobbies, and personal care. CDR scores are determined through semi-structured interviews with a licensed clinician, the participant, and a reliable informant such as a family member or friend. A CDR score of 0 indicates cognitive normality, 0.5 = very mild dementia, 1 = mild dementia, 2 = moderate dementia, and 3 = severe dementia. All participants in this study were CDR 0 at the time of evaluation.
APOE status
DNA samples were obtained and genotyped according to methods published previously [16]. An Illumina 610 or Omniexpress chip was used for genotyping. For the purposes of these analyses, APOE status was converted from a genotype to a binary variable. If participants had at least one copy of the Apolipoprotein (APOE) ɛ4 allele, they were deemed “APOE positive” and “APOE negative”otherwise.
In-home visit
As described in Bollinger et al. [15], an occupational therapist blinded to participants’ AD biomarker levels completed an in-home visit lasting approximately 120–180 min. The occupational therapist conducted assessments related to functional mobility, the peripheral nervous system, and fall covariates (Table 1). The visit was typically completed in 1 session but was completed over 2 sessions for 4 participants due to participant fatigue or request. Participants received their results from the home visit and fall risk assessments based on established fall risk cutoff scores [17].
Table 1
Clinically informed composites
Composite | Rationale | Measure | Description |
Sensation* | Reduced sensation, particularly in lower extremities and feet, can lead to increased fall risk [38, 39], for example, lack of feedback of changes in surface or feet placement in time to correct and prevent a fall | Tuning Forkr [38, 39] | Sensation testing (vibration, bilateral big toes) |
Sharpr | Sensation testing (sharp, arms and legs) | ||
Sensation questionnairer | 8-Item questionnaire | ||
Fall Risk Factors/Comorbidities* | Risk factors known to be associated with falls such as alcoholism, depression, pain, previous falls, and vision [40, 41]. | Short Michigan Alcoholism Screening Test-Geriatric Version (SMAST-G)r [42] | 10-Item interview |
Patient Health Questionnaire (PHQ-9)r [43] | 10-Item questionnaire assessing depression, frequency of symptoms | ||
Self-reported painr [44] | Pain Scale from 12-Item Short Form Survey | ||
Previous fallsr [17] | Total falls in the past 12 months, self-reported | ||
Early Treatment Diabetic Retinopathy Study (ETDRS) test [45] | Visual acuity score; number of correct letters read | ||
Pelli-Robson Test [46] | Contrast sensitivity; letter-by-letter | ||
Cognition | Certain domains of cognitive functioning such as episodic and semantic memory and processing speed are sensitive to preclinical changes in AD [47–51] and may be associated with an increased risk of falls among individuals at risk for AD, such as through impaired navigation of complex environments and stimuli. | Free and Cued Selective Reminding Test Free Recall [47] | Test of memory |
Animal Verbal Fluency Naming Total Score [48] | Test of semantic memory | ||
Craft Story 21 Delayed Recall [49] | Test of memory (delayed recall) | ||
Total correct score from the Number Symbol Test [see] [50, 51] | Processing speed; a computerized task developed and validated at the Knight ADRC that assesses similar constructs to the Wechsler Adult Intelligence Scale Digit Symbol Substitution task [51] | ||
Activities of Daily Living [22] | Decreased performance of ADL (such as requiring assistance for daily tasks) and presence of environmental hazards where daily activities are performed (loose rugs, clutter) are associated with increased fall risk [52-54]. | Older Adults Resources and Services Activities of Daily Living (OARS ADL) Scale [52] | Functional capacity; ability to perform 14 activities |
Performance Assessment of Self-Care Skills (PASS)r [53] | Performance of 3 complex daily activities: shopping, checkbook balancing, and medication management (independence mean score) | ||
Westmead Home Safety Assessment (WeHSA)r [54] | Rates 72 environmental home hazards | ||
Strength | Decreased strength in both upper and lower extremities has been associated with overall frailty and fall risk [55–57]. | Grip Strength [55] | Handheld dynamometer; pounds of strength in dominant hand |
Chair Stand Test [56, 57] | 30-second test of lower extremity strength and function | ||
Fall Precaution | Fear of falling and lack of preventative behaviors have been associated with falls [58, 59]. | Falls Behavioral Scale of Older People (FaB) [58] | Protective falls behaviors; 30-item questionnaire rating behaviors to prevent falls |
Falls Efficacy Scale-International Short Form (FES-ISF)r [59] | Concern of falling during 7 daily activities | ||
Functional Mobility | Gait, static and dynamic balance, and vestibular function capture how well a person can move around, and reduced functioning in this area is associated with falls [22, 60–63]. | Balance Tracking System (BTrackS)r [60, 61] | Balance and vestibular function; standing on BTrackS with feet hip-width apart with eyes open for 20 seconds x 3 trials. Average center of pressure (path length) from 3 trials used |
Performance-Oriented Mobility Assessment (POMA) [62] | Dynamic balance and mobility assessment; a task-oriented assessment of 9 balance tasks and 7 items to assess gait | ||
Timed Up and Go (TUG) Taskr [22, 63] | Gait speed; timed task of standing from a chair, walking 3 meters, turning, walking back, and sitting down |
rIndicates that the measure was reverse scored during composite score creation. *Treated as covariates in regression models. AD, Alzheimer’s disease; Knight ADRC, Knight Alzheimer’s Disease Research Center.
Fall measures
A 12-month calendar-journal was given to each participant to record whether or not a fall occurred. Falls were reported monthly via an automated call or e-mail [16]. Participants were compensated with $5 on a reloadable gift card after they completed their fall report each month. A fall was defined as any unintentional movement to the floor, ground, or an object below knee level [18]. If a fall was reported, an interviewer called the participant to verify that the fall met this operational definition. The measures of interest included days to first reported fall, total number of falls reported over the year (i.e., fall propensity), and highest severity of falls reported over the year [1]. These graded fall outcomes were chosen because they capture and retain important information on subtleties of falls. Fall severity was measured using a published algorithm [1]: 0, no falls; 1, one fall without serious injury that did not require medical attention; 2, a fall with minor injury that received medical attention but not a hospital admittance or more than one fall; and 3, a fall with major injury that prompted a hospital admission. Additional details about monthly fall monitoring have been published elsewhere [15]. Fall monitoring for the present study began at enrollment and continued for the first 12 months of the parent study.
Assessment of cognition
Participants were given a series of computerized and paper-and-pencil tasks to measure different facets of cognition. A cognitive composite similar to the Preclinical Alzheimer’s Cognitive Composite (PACC) [19, 20] was created by averaging the standardized scores from the following tests: Free and Cued Selective Reminding Test Free Recall, Animal Verbal Fluency Naming Total Score, Craft Story 21 Delayed Recall, and the total correct score from the Number Symbol Test (a computerized task developed and validated at the Knight ADRC that assesses similar constructs to the Wechsler Adult Intelligence Scale Digit Symbol Substitution task). Specifically, each participant’s raw score on each task was standardized to the mean and standard deviation of the cohort’s baseline completion of that particular test. The z-scores were then averaged together such that higher scores indicated better performance [21].
Clinically informed composites
We used our team members’ expertise in occupational therapy, neurology, and psychology to group the potential fall mechanisms collected into clinically meaningful categories. We refer to these groupings as composites, with each capturing different facets of functioning associated with falls (see Table 1) [3, 10]. The composites were: sensation, fall risk factors/comorbidities, cognition, activities of daily living [22], strength, fall precautionary behaviors, and functional mobility. Each composite was created by averaging the standardized scores from the measures listed in Table 1. Scores with the superscript “r” were reverse scored (standardized scores multiplied by –1) so that higher scores indicated better performance across all composites. Descriptions of measures used to create each composite and rationales are provided in Table 1.
Neuroimaging AD biomarkers
Amyloid PET imaging was performed with [11C] Pittsburgh Compound B (PiB) or [18F]-Florbetapir (AV45) and was acquired on a Biograph mMR scanner (Siemens Medical Solutions, Malvern, PA). PiB PET scans included data 30–60 min post-injection, and AV45 PET scans included data 50–70 min post-injection. All data were converted to standardized uptake value ratios with the cerebellar cortex used as a reference region before then being converted to the Centiloid scale [23, 24]. PET data were processed with an in-house pipeline using regions of interest (ROIs) derived from FreeSurfer (https://github.com/ysu001/PUP) [25]. This approach corrects for the spillover signal from adjacent ROIs and non-brain tissue on the basis of the scanner point spread function and the relative distance between regions. This partial volume correction approach accounts for spillover not only from different areas in the brain, but also from non-brain regions into the brain. Amyloid deposition was quantified with the average across the left and right lateral orbitofrontal, medial orbitofrontal, rostral middle frontal, superior frontal, superior temporal, middle temporal, and precuneus regions.
Structural MRI data were acquired on a Siemens Biograph mMR or Trio 3T scanner. T1-weighted images were acquired with a magnetization-prepared rapid acquisition gradient echo sequence acquired in the sagittal orientation with a repetition time of 2300 ms, an echo time of 2.95 ms, a flip angle of 9°, 176 slices, an in-plane resolution of 240×256, and a slice thickness of 1.2 mm. Images underwent volumetric segmentation with FreeSurfer 5.3 (http://freesurfer.net) to identify ROIs for further analysis. Hippocampal volumes were adjusted for head size with a regression approach and summed across hemispheres [26, 27]. Cortical thickness values were obtained for each hemisphere for a limited number of ROIs reflecting brain atrophy patterns in AD [28]. Cortical thickness was calculated as the shortest distance between the cortical gray/white boundary to the gray/cerebrospinal fluid boundary [29]. Neuroimaging AD biomarkers measured within 2 years of enrollment in the present study, which is also when the monthly fall monitoring began, were used for the analysis (see Fig. 1).
Statistical analysis
We used Pearson correlations to examine associations among the fall measures, clinically informed composites, and neuroimaging AD biomarkers (as continuous variables). We also conducted multiple regression models to test for the contribution of each clinically informed composite above and beyond that of the covariates (i.e., age, gender, education, APOE4 status, polypharmacy, sensation, and comorbidities of alcoholism, depression, and pain; see Table 1). Variables with significant correlations with one another were not included in the same models, as they would be subject to potential multicollinearity issues. This applied to the clinically informed and cognitive composites (Fig. 2). Therefore, non-nested models were used, and model fit was evaluated based on the R2 and Akaike information criterion (AIC). For all models, reference groups are indicated in the output tables (Tables 3 and 4). For all results reported, statistical significance was set at p < 0.05 two-tailed, unless otherwise noted. Cohen’s d [30], a measure of effect size, was reported for significant t-tests. Adjusted degrees of freedom were reported such that unequal variances were assumed for comparisons, and the Welsh approximation was applied. All analyses were conducted using the R statistical computing environment [31].
Fig. 2
Relationships Among Age, Composites, Neuroimaging Biomarkers, and Falls. Significant correlations at *p < 0.05, **p < 0.01, and ***p < 0.001. ADL, Activities of Daily Living.
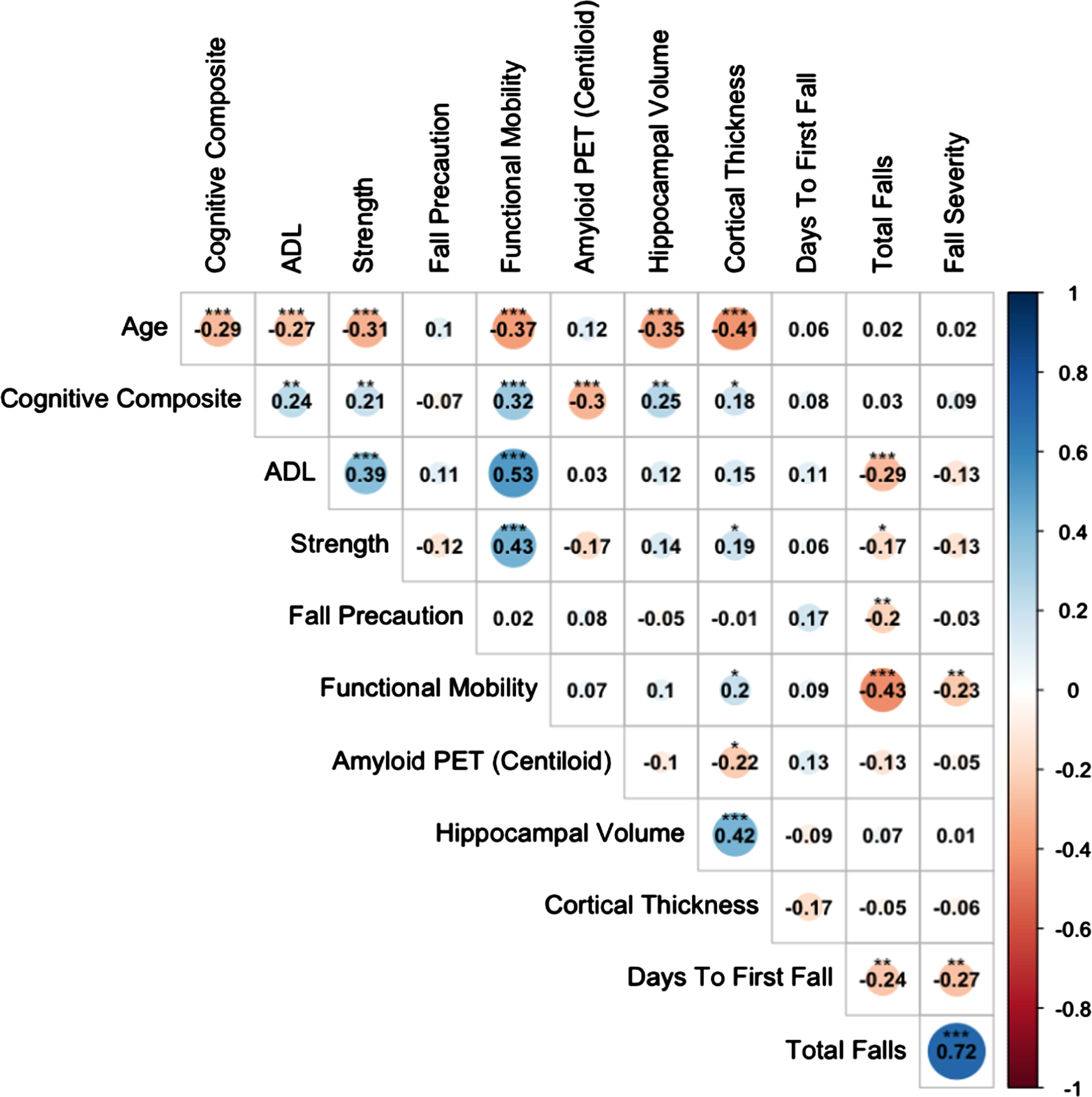
RESULTS
Sample characteristics
A total of 182 participants were included in the study. Demographic information and sample characteristics are displayed in Table 2. A total of 227 falls were reported over the 12-month monitoring period. Falls per person ranged from 0–16; the median number of falls per person was 1.
Table 2
Sample characteristics
Characteristic | N | Mean (SD); n (% ) |
Demographic | ||
Age (y) | 182 | 75 (6) |
Gender (Female) | 182 | 97 (53% ) |
Race | 182 | |
Black | 19 (10% ) | |
White | 162 (89% ) | |
No response | 1 (<1% ) | |
Education (y) | 182 | 16 (2) |
APOE4 (Negative) | 178 | 125 (69% ) |
Polypharmacy | 182 | 89 (49% ) |
Clinically Informed Composites | ||
Sensation* | 181 | 0.00 (0.93) |
Fall Risk Factors* | 182 | 0.03 (0.45) |
Cognition | 171 | –0.03 (0.67) |
ADL | 182 | 0.01 (0.69) |
Strength | 182 | 0.05 (0.79) |
Fall Precaution | 182 | 0.00 (0.55) |
Functional Mobility | 182 | 0.02 (0.69) |
Alzheimer’s Disease Biomarkers | ||
Amyloid PET (Centiloid) | 125 | 24.5 (36) |
Hippocampal Volume, Standardized | 125 | –0.01 (0.99) |
Cortical Thickness | 125 | 0.02 (1.02) |
Falls | Median (Range); n (%) | |
Days to First Fall | 182 | 49 (0–357) |
Fall Propensity (Total falls per person, over 1 y) | 182 | 1 (0–16) |
Fall Severity [1] (over 1 y) | 182 | |
0, No falls | 69 (38% ) | |
1, One fall without injury | 63 (35% ) | |
2, 1 + falls or 1 with minor injury | 41 (22% ) | |
3, Fall with major injury requiring hospitalization | 9 (5% ) |
*Treated as covariates in regression models. ADL, Activities of Daily Living.
Relationships among composites, neuroimaging biomarkers, and falls
Cortical thickness, a measure of neurodegeneration, was associated with functional mobility (r = 0.2, p < 0.05). Although the cognitive composite was associated with AD biomarkers and clinical composites, we found that the activities of daily living (ADL), strength, fall precaution, and functional mobility composites all significantly correlated with the fall outcome measures, particularly fall propensity (rs≤–0.4, ps < 0.01; see Fig. 2). The cognitive composite, in contrast, was not associated with any of the fall outcome measures (rs = 0.03–0.09). Participants with higher ADL function, strength, fall precaution, and functional mobility reported fewer total falls and less severe falls over the 12-month monitoring period. The same relationships appeared for fewer days to first fall; however, they were weaker and did not reach significance.
Predicting fall propensity and severity
ADL and functional mobility composites significantly predicted the total number of falls reported by participants over the 12-month monitoring period (fall propensity; see Table 3). Furthermore, examination of R2 and AIC values suggested that functional mobility was the domain that best predicted fall propensity compared to the other domains in the analysis.
Table 3
Identifying best predictor of fall propensity
DV =Total Falls | ||||||||||
Cognition | ADL | Strength | Fall Precaution | Functional | ||||||
Mobility | ||||||||||
Predictors | Estimate | p | Estimate | p | Estimate | p | Estimate | p | Estimate | p |
(Intercept) | 0.11 | 0.875 | 0.53 | 0.413 | 0.12 | 0.852 | 0.01 | 0.984 | 0.81 | 0.202 |
Age | 0.00 | 0.842 | –0.01 | 0.184 | 0.00 | 0.678 | 0.00 | 0.892 | –0.01 | 0.087 |
Gender [Male] | –0.06 | 0.479 | –0.04 | 0.645 | –0.05 | 0.594 | –0.09 | 0.286 | –0.11 | 0.161 |
Race [White] | 0.22 | 0.142 | 0.26 | 0.045 | 0.24 | 0.067 | 0.25 | 0.055 | 0.25 | 0.046 |
Education | 0.00 | 0.885 | 0.01 | 0.433 | 0.01 | 0.680 | 0.00 | 0.783 | 0.01 | 0.575 |
APOE4 | –0.03 | 0.733 | –0.08 | 0.364 | –0.03 | 0.703 | –0.05 | 0.532 | –0.03 | 0.713 |
Polypharmacy | 0.20 | 0.021 | 0.14 | 0.079 | 0.18 | 0.027 | 0.20 | 0.014 | 0.13 | 0.089 |
Sensation | –0.01 | 0.809 | 0.00 | 0.989 | –0.01 | 0.869 | –0.02 | 0.687 | 0.01 | 0.898 |
Fall Risk Factors | –0.28 | 0.002 | –0.26 | 0.002 | –0.27 | 0.002 | –0.24 | 0.007 | –0.20 | 0.021 |
Cognition | 0.04 | 0.606 | ||||||||
ADL | –0.17 | 0.004 | ||||||||
Strength | –0.04 | 0.578 | ||||||||
Fall Precaution | –0.13 | 0.079 | ||||||||
Functional Mobility | –0.24 | <0.001 | ||||||||
Observations | 165 | 176 | 176 | 176 | 176 | |||||
R2 R2adjusted | 0.123 0.072 | 0.170 0.126 | 0.129 0.082 | 0.144 0.097 | 0.208 0.165 | |||||
AIC | 268.109 | 271.134 | 279.657 | 276.706 | 262.961 |
ADL, Activities of Daily Living; AIC, Akaike information criterion.
Next, we performed a similar analysis to identify the domain that best predicted fall severity. Examination of R2 and AIC values again indicated that functional mobility was the domain that best predicted fall severity compared to the other predictors in the model (See Table 4).
Table 4
Identifying best predictor of fall severity
DV =Total Falls | ||||||||||
Cognition | ADL | Strength | Fall Precaution | Functional | ||||||
Mobility | ||||||||||
Predictors | Estimate | p | Estimate | p | Estimate | p | Estimate | p | Estimate | p |
(Intercept) | 0.65 | 0.55 | 0.62 | 0.563 | 0.26 | 0.805 | 0.37 | 0.725 | 1.18 | 0.27 |
Age | 0.00 | 0.974 | 0 | 0.776 | 0 | 0.849 | 0 | 0.953 | –0.01 | 0.389 |
Gender [Male] | –0.29 | 0.037 | –0.32 | 0.019 | –0.38 | 0.019 | –0.35 | 0.011 | –0.37 | 0.005 |
Race [White] | 0.51 | 0.028 | 0.65 | 0.003 | 0.66 | 0.003 | 0.65 | 0.003 | 0.65 | 0.002 |
Education | –0.01 | 0.627 | 0 | 0.994 | 0 | 0.892 | 0 | 0.912 | 0 | 0.982 |
APOE4 | 0.03 | 0.837 | –0.04 | 0.793 | –0.03 | 0.857 | –0.02 | 0.908 | –0.01 | 0.958 |
Polypharmacy | 0.39 | 0.005 | 0.36 | 0.007 | 0.4 | 0.003 | 0.39 | 0.004 | 0.33 | 0.012 |
Sensation | 0.04 | 0.612 | 0.02 | 0.805 | 0.01 | 0.932 | –0.31 | 0.035 | –0.22 | 0.124 |
Fall Risk Factors | –0.32 | 0.027 | –0.3 | 0.035 | –0.31 | 0.03 | 0.01 | 0.87 | 0.03 | 0.679 |
Cognition | 0.1 | 0.371 | ||||||||
ADL | –0.09 | 0.357 | ||||||||
Strength | 0.04 | 0.69 | ||||||||
Fall Precaution | 0.01 | 0.922 | ||||||||
Functional Mobility | –0.25 | 0.011 | ||||||||
Observations | 165 | 176 | 176 | 176 | 176 | |||||
R2 R2adjusted | 0.146 0.096 | 0.159 0.113 | 0.155 0.110 | 0.155 0.109 | 0.187 0.143 | |||||
AIC | 420.67 | 451.63 | 452.36 | 452.52 | 445.64 |
ADL, Activities of Daily Living; AIC, Akaike information criterion.
DISCUSSION
This study assessed the relationships among falls, clinically informed composites of functioning, and neuroimaging AD biomarkers among CN older adults. We examined the relationships between these variables and AD biomarkers, and examined the relationships among clinically informed composites of functioning to identify the strongest predictor(s) of fall propensity and severity in a sample of CN older adults.
Of this cohort, 62% reported at least one fall during the year of fall monitoring (see Table 2). This is a higher percentage than expected; we believe this is due to our fall reporting methods. We use calendar-journals and ask participants about falls and details of any falls monthly, which captures a larger and more accurate prevalence of falls compared to longer self-report interval periods (such as the commonly used year recall period) [32–34].
Cognition was more closely associated with AD neuropathology (amyloid and neurodegeneration) compared to other clinical measures (rs –0.30 –0.25, ps < 0.01); functional mobility was associated with neurodegeneration to a smaller degree (rs 0.2, ps < 0.05). Clinical measures of ADL performance, strength, fall precautionary behaviors, and functional mobility were associated with the fall outcome measures. These findings suggest that, although subtle changes in cognition may be more closely associated with AD neuropathology, functional performance and mobility indicators better predict falls in cognitively normal older adults at risk of developing symptomatic AD.
Finally, multiple regression analyses indicated that functional mobility was the best predictor of fall propensity and severity above and beyond a host of covariates including age, gender, education, APOE4 status, polypharmacy, comorbidities (alcoholism, depression, pain), and sensation (see Tables 3 and 4). The cognitive composite did not significantly predict either of the fall outcome measures. Polypharmacy was a significant predictor of both fall propensity and fall severity in most models, standing out among many other fall risk covariates and stressing its importance in studying falls among this population. Polypharmacy is a well-known independent risk factor for falls [35, 36].
Because older adults with preclinical AD are at higher risk of falling compared to those without preclinical AD, a better understanding of the mechanisms underlying falls in older adults is needed to understand their relation to the progression of AD as well as to develop targeted fall prevention interventions. Measures of functional mobility may also help identify older adults at risk for falls and those who may benefit from further neurological or biomarker testing.
Even though motor abilities predicted falls while cognition did not in this sample, our results support recent findings that motor systems may become impaired prior to cognitive symptoms in the earliest stages of AD [11–13], and that these impairments may be the cause of increased risk of falls in this population. Among individuals with mild cognitive impairment, lower gait speed greatly increases the likelihood of progressing to dementia [13]. This may also be true in the preclinical stage of the disease, in that individuals with biomarkers consistent with AD and worse gait and balance may be more likely to progress to cognitive symptoms. Future longitudinal research should examine which factors best predict progression to symptomatic AD.
Limitations
The findings of this study should be considered in light of its limitations. First, we only analyzed a single time point for the clinical, cognitive, and biomarker measures in this initial examination. These relationships will be examined throughout the larger longitudinal observational study to better understand how these mechanisms impact fall risk and severity over time. Second, the data failed to produce significant relationships among AD biomarkers and fall measures as would be expected based on Keleman et al. [37]. As there are many ways to measure cognition and other clinical constructs of interest, it is possible that using more sensitive measures and other analytical procedures may capture preclinical AD changes and yield a different pattern of results. Finally, participants consisted of older adults who were comprehensively phenotyped and often engaged in imaging and fluid biomarker studies, and therefore are not representative of the general population. Although a primary goal of the Knight ADRC is to diversify enrollment to include underrepresented persons in aging and dementia research, the participants in this study were primarily White and highly educated (see Table 2).
Conclusions
This study extends upon previous work showing a relationship between preclinical AD biomarkers and falls in CN older adults. Functional mobility best predicted fall propensity and severity beyond a host of covariates, where other clinical measures such as strength and cognition did not. Cognition was more closely associated with AD neuropathology (amyloid and neurodegeneration) compared to other clinical measures, and functional mobility was associated with neurodegeneration but to a lesser extent. These findings suggest that, although subtle changes in cognition may be more closely associated with AD neuropathology, functional mobility indicators better predict falls in CN older adults. Together, these findings suggest that functional mobility plays an important role in fall risk among older adults at risk for AD and may be an ideal mechanism for future fall prevention strategies.
ACKNOWLEDGMENTS
We would like to thank the Knight Alzheimer’s Disease Research Center staff, dedicated participants, Clinical and Imaging Cores, and Megen Devine for her editorial assistance. We also acknowledge Avid Radiopharmaceuticals for access to the AV45 tracer; they were not involved in data analysis or interpretation.
FUNDING
This study was supported by the following sources: National Institutes of Health (NIH) R01AG057680, 5TL1TR002344-05, UL1 TR002345, P30AG066444, P01AG03991, and P01AG026276; the Daniel J. Brennan MD Fund; and the Paula and Rodger O. Riney Fund.
CONFLICT OF INTEREST
The authors have no conflict of interest to report.
DATA AVAILABILITY
The clinical data supporting the findings of this study are available on request from the corresponding author, and biomarker data on request from the Knight ADRC. The data are not publicly available due to privacy or ethical restrictions.
REFERENCES
[1] | Tinetti ME , Williams CS ((1997) ) Falls, injuries due to falls, and the risk of admission to a nursing home. N Engl J Med 337: , 1279–1284. |
[2] | Anstey KJ , Von Sanden C , Luszcz MA ((2006) ) An 8-year prospective study of the relationship between cognitive performance and falling in very old adults. J Am Geriatr Soc 54: , 1169–1176. |
[3] | Tinetti ME , Speechley M , Ginter SF ((1988) ) Risk factors for falls among elderly persons living in the community. N Engl J Med 319: , 1701–1707. |
[4] | Jack CR , Bennett DA , Blennow K , Carrillo MC , Feldman HH , Frisoni GB , Hampel H , Jagust WJ , Johnson KA , Knopman DS ((2016) ) A/T/N: An unbiased descriptive classification scheme for Alzheimer disease biomarkers. Neurology 87: , 539–547. |
[5] | Jack CR Jr , Bennett DA , Blennow K , Carrillo MC , Dunn B , Haeberlein SB , Holtzman DM , Jagust W , Jessen F , Karlawish J ((2018) ) NIA-AA research framework: Toward a biological definition of Alzheimer’s disease. Alzheimers Dement 14: , 535–562. |
[6] | Aschenbrenner AJ , Balota DA , Fagan AM , Duchek JM , Benzinger TL , Morris JC ((2015) ) Alzheimer disease cerebrospinal fluid biomarkers moderate baseline differences and predict longitudinal change in attentional control and episodic memory composites in the adult children study. J Int Neuropsychol Soc 21: , 573–583. |
[7] | Brookmeyer R , Abdalla N , Kawas CH , Corrada MM ((2018) ) Forecasting the prevalence of preclinical and clinical Alzheimer’s disease in the United States. Alzheimers Dement 14: , 121–129. |
[8] | Parnetti L , Chipi E , Salvadori N , D’Andrea K , Eusebi P ((2019) ) Prevalence and risk of progression of preclinical Alzheimer’s disease stages: A systematic review and meta-analysis. Alzheimers Res Ther 11: , 1–13. |
[9] | Stark SL , Roe CM , Grant EA , Hollingsworth H , Benzinger TL , Fagan AM , Buckles VD , Morris JC ((2013) ) Preclinical Alzheimer disease and risk of falls. Neurology 81: , 437–443. |
[10] | Deandrea S , Lucenteforte E , Bravi F , Foschi R , La Vecchia C , Negri E ((2010) ) Risk factors for falls in community-dwelling older people: A systematic review and meta-analysis. Epidemiology 21: , 658–668. |
[11] | Skillbäck T , Blennow K , Zetterberg H , Skoog J , Rydén L , Wetterberg H , Guo X , Sacuiu S , Mielke MM , Zettergren A ((2022) ) Slowing gait speed precedes cognitive decline by several years. Alzheimers Dement 18: , 1667–1676. |
[12] | Grande G , Triolo F , Nuara A , Welmer A-K , Fratiglioni L , Vetrano DL ((2019) ) Measuring gait speed to better identify prodromal dementia. Exp Gerontol 124: , 110625. |
[13] | Albers MW , Gilmore GC , Kaye J , Murphy C , Wingfield A , Bennett DA , Boxer AL , Buchman AS , Cruickshanks KJ , Devanand DP ((2015) ) At the interface of sensory and motor dysfunctions and Alzheimer’s disease. Alzheimers Dement 11: , 70–98. |
[14] | Morris JC ((1997) ) Clinical dementia rating: A reliable and valid diagnostic and staging measure for dementia of the Alzheimer type. Int Psychogeriatr 9: , 173–176. |
[15] | Bollinger RM , Keleman A , Thompson R , Westerhaus E , Fagan AM , Benzinger TL , Schindler SE , Xiong C , Balota D , Morris JC ((2021) ) Falls: A marker of preclinical Alzheimer disease: A cohort study protocol. BMJ Open 11: , e050820. |
[16] | Cruchaga C , Kauwe JS , Harari O , Jin SC , Cai Y , Karch CM , Benitez BA , Jeng AT , Skorupa T , Carrell D ((2013) ) GWAS of cerebrospinal fluid tau levels identifies risk variants for Alzheimer’s disease. Neuron 78: , 256–268. |
[17] | Lusardi MM , Fritz S , Middleton A , Allison L , Wingood M , Phillips E , Criss M , Verma S , Osborne J , Chui KK ((2017) ) Determining risk of falls in community dwelling older adults: A systematic review and meta-analysis using posttest probability. J Geriatr Phys Ther 40: , 1–36. |
[18] | Lamb SE , Jørstad-Stein EC , Hauer K , Becker C , Prevention of Falls Network Europe and Outcomes Consensus Group ((2005) ) Development of a common outcome data set for fall injury prevention trials: The Prevention of Falls Network Europe Consensus. J Am Geriatr Soc 53: , 1618–1622. |
[19] | Donohue MC , Sperling RA , Salmon DP , Rentz DM , Raman R , Thomas RG , Weiner M , Aisen PS ((2014) ) The preclinical Alzheimer cognitive composite: Measuring amyloid-related decline. JAMA Neurol 71: , 961–970. |
[20] | Papp KV , Rentz DM , Orlovsky I , Sperling RA , Mormino EC ((2017) ) Optimizing the preclinical Alzheimer’s cognitive composite with semantic processing: The PACC5. Alzheimers Dement 3: , 668–677. |
[21] | Weintraub S , Salmon D , Mercaldo N , Ferris S , Graff-Radford NR , Chui H , Cummings J , DeCarli C , Foster NL , Galasko D ((2009) ) The Alzheimer’s disease centers’ Uniform Data Set (UDS): The neuropsychological test battery. Alzheimer Dis Assoc Disord 23: , 91. |
[22] | Podsiadlo D , Richardson S ((1991) ) The “Timed Up & Go”: A test of basic functional mobility for frail elderly persons. J Am Geriatr Soc 39: , 142–148. |
[23] | Su Y , Blazey TM , Owen CJ , Christensen JJ , Friedrichsen K , Joseph-Mathurin N , Wang Q , Hornbeck RC , Ances BM , Snyder AZ ((2016) ) Quantitative amyloid imaging in autosomal dominant Alzheimer’s disease: Results from the DIAN study group. PLoS One 11: , e0152082. |
[24] | Mishra S , Gordon BA , Su Y , Christensen J , Friedrichsen K , Jackson K , Hornbeck R , Balota DA , Cairns NJ , Morris JC ((2017) ) AV-1451 PET imaging of tau pathology in preclinical Alzheimer disease: Defining a summary measure. Neuroimage 161: , 171–178. |
[25] | Su Y , D’Angelo GM , Vlassenko AG , Zhou G , Snyder AZ , Marcus DS , Blazey TM , Christensen JJ , Vora S , Morris JC ((2013) ) Quantitative analysis of PiB-PET with freesurfer ROIs. PLoS One 8: , e73377. |
[26] | Head D , Rodrigue KM , Kennedy KM , Raz N ((2008) ) Neuroanatomical and cognitive mediators of age-related differences in episodic memory. Neuropsychology 22: , 491–507. |
[27] | Raz N , Lindenberger U , Ghisletta P , Rodrigue KM , Kennedy KM , Acker JD ((2008) ) Neuroanatomical correlates of fluid intelligence in healthy adults and persons with vascular risk factors. Cereb Cortex 18: , 718–726. |
[28] | Wang L , Benzinger TL , Hassenstab J , Blazey T , Owen C , Liu J , Fagan AM , Morris JC , Ances BM ((2015) ) Spatially distinct atrophy is linked to β-amyloid and tau in preclinical Alzheimer disease. Neurology 84: , 1254–1260. |
[29] | Fischl B , Dale AM ((2000) ) Measuring the thickness of the human cerebral cortex from magnetic resonance images. Proc Natl Acad Sci U S A 97: , 11050–11055. |
[30] | Cohen J ((1988) ) Statistical Power Analysis for the Behavioral Sciences. Lawrence Erlbaum Associates, Hillsdale, New Jersey. |
[31] | R Core Team (2013) R: A language and environment for statistical computing. R Foundation for Statistical Computing, Vienna, Austria. https://www.R-project.org/. |
[32] | Ganz DA , Higashi T , Rubenstein LZ ((2005) ) Monitoring falls in cohort studies of community-dwelling older people: Effect of the recall interval. J Am Geriatr Soc 53: , 2190–2194. |
[33] | Hannan MT , Gagnon MM , Aneja J , Jones RN , Cupples LA , Lipsitz LA , Samelson EJ , Leveille SG , Kiel DP ((2010) ) Optimizing the tracking of falls in studies of older participants: Comparison of quarterly telephone recall with monthly falls calendars in the MOBILIZE Boston Study. Am J Epidemiol 171: , 1031–1036. |
[34] | Hoffman GJ , Ha J , Alexander NB , Langa KM , Tinetti M , Min LC ((2018) ) Underreporting of fall injuries of older adults: Implications for wellness visit fall risk screening. J Am Geriatr Soc 66: , 1195–1200. |
[35] | Dhalwani NN , Fahami R , Sathanapally H , Seidu S , Davies MJ , Khunti K ((2017) ) Association between polypharmacy and falls in older adults: A longitudinal study from England. BMJ Open 7: , e016358. |
[36] | Hammond T , Wilson A ((2013) ) Polypharmacy and falls in the elderly: A literature review. Nurs Midwifery Stud 2: , 171–175. |
[37] | Keleman A , Wisch JK , Bollinger RM , Grant EA , Benzinger TL , Morris JC , Ances BM , Stark SL ((2020) ) Falls associate with neurodegenerative changes in ATN framework of Alzheimer’s disease. J Alzheimers Dis 77: , 745–752. |
[38] | Kästenbauer T , Sauseng S , Brath H , Abrahamian H , Irsigler K ((2004) ) The value of the Rydel-Seiffer tuning fork as a predictor of diabetic polyneuropathy compared with a neurothesiometer. Diabet Med 21: , 563–567. |
[39] | Deshpande N , Ferrucci L , Metter J , Faulkner KA , Strotmeyer E , Satterfield S , Schwartz A , Simonsick E ((2008) ) Association of lower limb cutaneous sensitivity with gait speed in the elderly: The health ABC study. Am J Phys Med Rehabil 87: , 921–928. |
[40] | Rubenstein LZ ((2006) ) Falls in older people: Epidemiology, risk factors and strategies for prevention. Age Ageing 35: , ii37–ii41. |
[41] | Lord SR , Menz HB , Tiedemann A ((2003) ) A physiological profile approach to falls risk assessment and prevention. Phys Ther 83: , 237–252. |
[42] | Blow F ((1991) ) Michigan Alcoholism Screening Test-Geriatric Version (MAST-G). University of Michigan Alcohol Research Center, Ann Arbor, Michigan. |
[43] | Spitzer RL , Kroenke K , Williams JB , the Patient Health Questionnaire Primary Case Study Group ((1999) ) Validation and utility of a self-report version of PRIME-MD: The PHQ primary care study. JAMA 282: , 1737–1744. |
[44] | Gandek B , Ware JE , Aaronson NK , Apolone G , Bjorner JB , Brazier JE , Bullinger M , Kaasa S , Leplege A , Prieto L ((1998) ) Cross-validation of item selection and scoring for the SF-12 Health Survey in nine countries: Results from the IQOLA Project. J Clin Epidemiol 51: , 1171–1178. |
[45] | Ferris III FL , Kassoff A , Bresnick GH , Bailey I ((1982) ) New visual acuity charts for clinical research. Am J Ophthalmol 94: , 91–96. |
[46] | Pelli D , Robson J ((1988) ) The design of a new letter chart for measuring contrast sensitivity. Clin Vision Sci 2: , 187–199. |
[47] | Grober E , Buschke H ((1987) ) Genuine memory deficits in dementia. Dev Neuropsychol 3: , 13–36. |
[48] | Rosen WG ((1980) ) Verbal fluency in aging and dementia. J Clin Exp Neuropsychol 2: , 135–146. |
[49] | Craft S , Newcomer J , Kanne S , Dagogo-Jack S , Cryer P , Sheline Y , Luby J , Dagogo-Jack A , Alderson A ((1996) ) Memory improvement following induced hyperinsulinemia in Alzheimer’s disease. Neurobiol Aging 17: , 123–130. |
[50] | Weintraub S , Besser L , Dodge HH , Teylan M , Ferris S , Goldstein FC , Giordani B , Kramer J , Loewenstein D , Marson D ((2018) ) Version 3 of the Alzheimer Disease Centers’ neuropsychological test battery in the Uniform Data Set (UDS). Alzheimer Dis Assoc Disord 32: , 10–17. |
[51] | Hassenstab J , Aschenbrenner AJ , Balota DA , McDade E , Lim YY , Fagan AM , Benzinger TL , Cruchaga C , Goate AM , Morris JC ((2020) ) Remote cognitive assessment approaches in the Dominantly Inherited Alzheimer Network (DIAN) using digital technology to drive clinical innovation in brain-behavior relationships: A new era in neuropsychology. Alzheimers Dement 16: , e038144. |
[52] | Fillenbaum G ((1988) ) Multidimensional Functional Assessment of Older Adults. Erlbaum Associates, Hillsdale, New Jersey. |
[53] | Holm MB , Rogers JC , Hemphill-Pearson B ((2008) ) The performance assessment of self-care skills (PASS). Assess Occup Ther Mental Health 2: , 101–110. |
[54] | Clemson L , Roland M , Cumming RG ((1997) ) Types of hazards in the homes of elderly people. OTJR (Thorofare N J) 17: , 200–213. |
[55] | Bohannon RW ((2008) ) Hand-grip dynamometry predicts future outcomes in aging adults. J Geriatr Phys Ther 31: , 3–10. |
[56] | Rikli RE , Jones CJ ((1999) ) Development and validation of a functional fitness test for community-residing older adults. J Aging Phys Act 7: , 129–161. |
[57] | Ward RE , Leveille SG , Beauchamp MK , Travison T , Alexander N , Jette AM , Bean JF ((2015) ) Functional performance as a predictor of injurious falls in older adults. J Am Geriatr Soc 63: , 315–320. |
[58] | Clemson L , Cumming RG , Heard R ((2003) ) The development of an assessment to evaluate behavioral factors associated with falling. Am J Occup Ther 57: , 380–388. |
[59] | Kempen GI , Yardley L , Van Haastregt JC , Zijlstra GR , Beyer N , Hauer K , Todd C ((2008) ) The Short FES-I: A shortened version of the falls efficacy scale-international to assess fear of falling. Age Ageing 37: , 45–50. |
[60] | O’Connor SM , Baweja HS , Goble DJ ((2016) ) Validating the BTrackS Balance Plate as a low cost alternative for the measurement of sway-induced center of pressure. J Biomech 49: , 4142–4145. |
[61] | Levy SS , Thralls KJ , Kviatkovsky SA ((2018) ) Validity and reliability of a portable balance tracking system, BTrackS, in older adults. J Geriatr Phys Ther 41: , 102–107. |
[62] | Tinetti ME ((1986) ) Performance-oriented assessment of mobility problems in elderly patients. J Am Geriatr Soc 34: , 119–126. |
[63] | Viccaro LJ , Perera S , Studenski SA ((2011) ) Is Timed Up and Go better than gait speed in predicting health, function, and falls in older adults? J Am Geriatr Soc 59: , 887–892. |