Computational Approaches for Supporting Combination Therapy in the Post-Aducanumab Era in Alzheimer’s Disease
Abstract
With the approval of aducanumab on the “Accelerated Approval Pathway” and the recognition of amyloid load as a surrogate marker, new successful therapeutic approaches will be driven by combination therapy as was the case in oncology after the launch of immune checkpoint inhibitors. However, the sheer number of therapeutic combinations substantially complicates the search for optimal combinations. Data-driven approaches based on large databases or electronic health records can identify optimal combinations and often using artificial intelligence or machine learning to crunch through many possible combinations but are limited to the pharmacology of existing marketed drugs and are highly dependent upon the quality of the training sets. Knowledge-driven in silico modeling approaches use multi-scale biophysically realistic models of neuroanatomy, physiology, and pathology and can be personalized with individual patient comedications, disease state, and genotypes to create ‘virtual twin patients’. Such models simulate effects on action potential dynamics of anatomically informed neuronal circuits driving functional clinical readouts. Informed by data-driven approaches this knowledge-driven modeling could systematically and quantitatively simulate all possible target combinations for a maximal synergistic effect on a clinically relevant functional outcome. This approach seamlessly integrates pharmacokinetic modeling of different therapeutic modalities. A crucial requirement to constrain the parameters is the access to preferably anonymized individual patient data from completed clinical trials with various selective compounds. We believe that the combination of data- and knowledge driven modeling could be a game changer to find a cure for this devastating disease that affects the most complex organ of the universe.
INTRODUCTION: THE NEED FOR COMBINATION THERAPY
Comorbidities in the aging brain
Many comorbidities converge in the aging Alzheimer’s disease (AD) brain. Although defined by the presence of amyloid plaques and neurofibrillary tangles, by no means are these the only neuropathological findings documented in postmortem studies of AD patients. For instance, it has been reported that over 80% of AD brains show vascular pathology to some degree [1]. While minor vascular pathological signs are unlikely to contribute very much to the clinical phenotype of full-blown AD, it is conceivable that in earlier stages vascular pathology contributes to the cognitive deterioration and the appearance of amyloid and tau pathology. Lewy body pathology, a synucleinopathy is often associated not only with Parkinson’s disease, Parkinson’s disease with dementia, but also AD as they share common clinical features [2]. Recently TDP-43 aggregates have been detected in up to 30% of postmortem AD brain [3] leading to the concept of limbic-dominated age-related TDP-43 encephalopathy in the oldest-old where primary TDP-43 is often observed together with amyloid and tau pathology [4].
This leads to the conceptual question whether it would be possible to identify relevant biomarkers that could identify patient populations with a specific and unique pathology to which more targeted and highly selective therapies could be developed. For this concept to be successful, it then becomes crucial to determine the optimal window of treatment for the specific intervention that would address the single triggering factor. However, it is very well conceivable that even if specific forms of neurodegenerative diseases are triggered by a unique pathology, additional pathological factors play an increasingly important role in driving the pathology and clinical phenotypes.
Polypharmacy in other indications
In many other CNS indications, interventions aimed at restoring neuronal circuits by modulating multiple pathways have shown superior clinical benefit compared to interventions focused on single targets. For instance, successful antipsychotics have been discovered in phenotypic assays, long before even the targets were identified and almost all of them have a rich pharmacology [5]. In contrast, over the last 20 years, many selective drugs have failed in clinical trials for schizophrenia [6]. Similarly, anti-depressants whether based on serotonin or norepinephrine transport inhibition affect a number of neuronal circuits through their indirect effects on different subtypes of serotonin or noradrenergic receptors. Along the same lines, drugs discovered using target-agnostic phenotypic assays usually have pleiotropic effects and are likely to be more first-in-class [7]. As a prime example, the novel antipsychotic SEP-363856 [8] was recently identified in a phenotypic in vivo assay and showed clinical efficacy in a Phase II study in schizophrenia despite being devoid of D2R antagonism.
In other non-CNS indications, combination therapies are also common, such as in treatment of Helicobacter pylori [9], HIV [10], and hypertension [11].
De-risking novel R&D projects
An argument for de-risking new CNS R&D projects can be made for new combinations between a symptomatic and disease-modifying target. In such a hypothetical scenario, short-term trials (24 weeks) can be designed to test the symptomatic effect of the combination, While the regulatory approval if successful is ongoing, placebo patients can be switched to active medication for the longer 2–3 year trials in order to show benefit in a staggered trial design. If the combination is indeed disease-modifying, the patients who start active medication later are not supposed to catch up. This also allows large longitudinal data on biomarkers to be collected. In the most successful scenario, the combination will be approved and can be marketed before the long duration trials read out. If there is clear evidence of disease modification, the sponsor can then request a label change. Whether such a combination therapy ideally consists of a rationally designed single molecule or an actual combination of two drugs, will be discussed in the next section.
Amyloid modulating agents as background therapy
With the approval of Aduhelm and the recognition of amyloid load as a surrogate marker by the FDA, many future clinical trials will see patients already on these approved medications, raising important questions about pharmacodynamic interactions with novel investigative drugs. It is clear that amyloid reduction at best leads to modest changes in clinical deterioration and that there is room for additional therapeutics to improve patient quality of life. In addition, amyloid-related imaging abnormalities side-effects need to be taken into account opening the possibilities of dose-sparing with new combinations.
THE CHALLENGE OF COMBINATION THERAPY IN ALZHEIMER’S DISEASE
No shortage of possible targets
A large number of targets are being pursued by large and small companies (for a list, see the Alzforum database of therapeutics), broadly classified in amyloid, tau, neuroinflammation, synaptic function, neuroprotection, cholesterol, metals, dietary supplements, DNA/RNA based and symptomatic treatment. In addition, large public-private consortia such as Accelerating Medicines Partnership for AD (AMP-AD) have recently generated an additional list of nominated targets (https://agora.ampadportal.org/genes/(genes-router:genes-list)) with varying degrees of druggability. All these targets affect a specific pathway deemed to be on a critical path for the clinical phenotype, but their impact might differ dependent upon the disease state, the comorbidities, the patient genotypes and likely also the patient comedications. The challenge is to 1) prioritize the targets in a rational way for the limited amount of resources, 2) generate as much knowledge from small clinical studies, and 3) to identify possible synergies between different pathways for each patient subgroup.
An optimistic viewpoint is that each and every one of these therapeutic approaches can be considered as a unique pharmacological challenge of the human AD brain and therefore can teach us something different about the underlying response if we would have the appropriate tools for reverse engineer the response (see below).
Combinatorial explosion of possible combinations
The number of possible combinations rapidly increases to the point that performing actual experiments, even preclinical ones become prohibitive. Simple combinatorial mathematics suggest that there are already 11 combinations for four combinations. For 10 possible targets the number increases to 1,013 combinations and for 15 targets the number rapidly increases to over 37,500. With every week new hypotheses for reverting “Alzheimer” phenotypes in diverse model organisms being published, the challenge is to prioritize these interventions, not only in terms of impact on the human disease, but also in terms of druggability and side-effects. Obviously, the vast majority of the combinations will not be of interest but finding those combinations with a substantial degree of synergy is of utmost importance.
Polypharmacy is already prominent in current clinical practice
AD patients are treated with a number of comedications not only to address cognitive impairment (standard-of-care) but also other comorbidities with an estimated 40% on antidepressants and 20% on antipsychotics for behavioral disturbances [12]. Current guidelines have been established for pharmacokinetic (PK-PK) interactions whereby one drug affects the metabolism of the other drugs or where a specific genotype of the metabolizing enzymes determines individual drug dose. However, the often underappreciated pharmacodynamic interactions (PD-PD) where drugs interact on the same neuronal circuit are not very well studied in detail, which often can lead to a less than optimal treatment paradigm. For instance, the use of drugs with direct or an indirect anticholinergic activity leads to a higher risk of AD [13, 14]. The number of medications in elderly patients is correlated with higher incidence of unplanned hospitalization [15] and polypharmacy is associated with lower cognitive performance [16]. In some cases neurocognitive effects of comedications have been linked to the failure of clinical trials [17]. This significantly complicates the clinical development of combination treatment, as many pharmacokinetic and pharmacodynamic interactions need to be controlled and mitigated.
Developing single drug with rational polypharmacy or combination of different drugs?
An important question relates to the decision to develop a single molecule with different pharmacological activities or developing a combination of different single highly-specific drugs. In view of the polypharmacy already present in clinical practice (see above), it might make more sense to develop a single drug as this would limit the number of PK-PK interactions. However, the medicinal chemistry is more complex and the objective of a rational polypharmacy is in conflict with the usual highly selective, highly potent strategy used for many decades in pharmaceutical drug discovery [6].
Recent developments in artificial intelligence and machine learning [18] are being applied to large chemical databases and can speed up the discovery of interesting hits, even with balanced polypharmacy. The availability of many protein structures in the Protein Database (PDB) (https://www.wwpdb.org/) [19], together with sophisticated and advanced computational docking molecular modeling techniques [20] allows to screen large virtual libraries and speed up the discovery of putative hits.
Further challenges abound with developing a cocktail of individual drugs, including 1) complex PK-PK interaction studies, 2) demonstrating that all components of such a combination are needed for the clinical effect, or 3) providing evidence that the combination has a synergistic effect.
Regulatory considerations
Traditionally, development of a fixed combination of two medications A + B necessitates the proof that the combination is superior to placebo, drug A in isolation and drug B in isolation resulting in substantial costs and resources. However, in 2013 the FDA has issued an updated guidance for co-development of two or more medications under strict conditions without the need for an expensive and resourceful 2×2 design (https://www.fda.gov/media/80100/download). Direct development of a combination is to be discussed on a case-by case basis but can be approved when each of the following conditions is met: 1) the combination is intended to treat a serious disease or condition; 2) there is a compelling biological rationale for the use of the combination; 3) a preclinical model or short-term clinical study on an established biomarker suggests that the combination has substantial activity and provides greater than additive activity or a more durable response (e.g., delayed resistance) compared to the individual agents alone; and 4) there is a compelling reason why the agents cannot be developed individually. While this is still a high threshold, at least in principle it allows for an accelerated and relatively straightforward clinical development plan.
Given the fact that preclinical animal models do not recapitulate the complete pathology of AD but only one or two aspects, it will be highly challenging to demonstrate the superiority of a combination over the two individual drugs in these animal model systems. On the other hand, well-validated human induced pluripotent stem cells (IPSC) and 3D organoid models might provide experimental evidence for a synergistic effect of a combination therapy focused on intracellular pathways. For all other combinations, more indirect evidence from predictive computer models (see below) might provide a basis for discussion.
KNOWLEDGE-DRIVEN COMPUTATIONAL APPROACH FOR UNDERSTANDING THE BIOLOGY
In order to identify the optimal combination of new drugs, one has to better understand the interactions of the many biological pathways driving the clinical trajectory. While animal models still can provide ample information, they suffer from a substantial lack of translationability to the clinical situation [21]. The new technology of neuronally differentiated human IPSC partially and certainly the development of brain organoids partially addresses this issue but suffers from low throughput and issues with differentiation and standardization. The previous discussion has highlighted the absolute need for a better understanding of the complex pathology in clinical AD, preferentially by using the information gathered from the many clinical trials over the past decades.
We propose a novel simulation approach, called quantitative systems pharmacology (QSP) that expands upon data-driven systems biology by combining the properties of a large number of pharmaceutical interventions with a model of the biology in a quantitative way, so that clinical data on disease trajectory can be fully integrated.
Basically, this multi-level modeling approach [22] integrates information about the underlying biology and pathology in an actionable computer model allowing for modeling biomarkers such as amyloid and tau load. This is combined with a biophysical realistic simulation of neuronal activity using Hodgkin-Huxley equations where action potential dynamics is driven by changes in conductances of voltage-gated ion channels [23]. The action potential dynamics in these specific brain regions can drive human behavior and is a proxy for clinical outcomes. In particular, the stability of a memory trace in a working memory paradigm in cortical neuronal networks after implementation of AD pathology has been calibrated with changes on clinically relevant scales such as ADAS-Cog [24].
The current version of the QSP model for AD includes a neuronal excitation-inhibition network based on multicompartment model of pyramidal excitatory and inhibitory GABA neurons with over 30 G-protein coupled receptors implemented, the differential effect of various amyloid-β (Aβ) forms on glutamatergic and nicotinic neurotransmission [25, 26] and the effect of oligomeric tau on voltage-gated and compartment-specific Na + and K + channels [27]. Amyloid aggregation dynamics, from synthesis, enzymatic removal of monomers, primary and secondary nucleation, breakdown of large aggregates, formation of protofibrils along an off-aggregation pathway, and finally deposition into plaques together with the clearance by activated microglia cells is included [28]. Spatio-temporal progression of misfolded tau protein along neuronal projections is implemented using preclinical and clinical data. Especially the impact of amyloid modulating antibodies is calibrated using clinical observations on the surrogate cerebrospinal fluid biomarkers Aβ42 levels and standardized uptake value ratio. The impact of medications is calculated using the appropriate pharmacology and level of target exposure often derived from PET displacement studies [29]. Genotypes such as the COMTVal158Met [29] and 5-HTTLPR rs23351 [26, 30] can be included as well as they impact neuronal firing and functional outcome.
AD pathology is implemented using a cholinergic deficit and a progressive loss of neurons and synapses over time, and the in silico readout of memory trace stability is used as a proxy for cognitive performance. The platform is calibrated with ADAS-Cog readouts of 28 different drug-dose-duration clinical trials outcomes, not only from standard-of-care procholinergic medication but also with serotonergic interventions [24].
The strategy is to use this platform to simulate the outcome of a number of clinical trials with highly selective drugs and to consider each of them as perturbing a single pathway. By combining the insights of a number of these trials, a more complete picture of the complex underlying biology can be formed. Figure 1 illustrates this strategy, which is somewhat analogous to well-established seismology approaches in geology to identify subterranean oil fields. Here the properties of the reflected waves are determined by the constitution of the different layers. In the case of clinical AD, the “seismology waves” consist of the different therapeutic approaches, each one with their own very selective target that perturbates part of the system at a particular point along the disease trajectory of an individual patient. The “reflection waves” can be defined as the clinical outcome which is a complex combination of the pharmacodynamics and pharmacokinetics of the therapeutic intervention and the reaction of the underlying pathways or circuits to this therapeutic perturbation. The aim is to use the QSP platform to generate information about the underlying pathology by “deconvoluting” this complex response using quantitative information about the drug and the underlying biology.
Fig. 1
Schematic representation of the ‘seismology’ strategy for elucidating key pathways driving the AD phenotype. Using the concept of AD pathology as a black box, each clinical trial (1 ... N) with a drug acting on a specific target (here a few examples are given for which clinical data in principle are available) can be considered a highly localized challenge that generates a perturbation inside the system and generating a reaction captured as the clinical outcome of that intervention. This response is a highly complex combination of the underlying pathology and the pharmacodynamic activity and pharmacokinetic profile of the drug. The challenge is to “deconvolute” this response so as to generate actionable knowledge about the internal pathways and circuits triggered by this perturbation. Modeling these “interventions” in an actionable computer model using available information about the pharmacology of the drug together with extensive biological and genetic knowledge can significantly speed up this process. In principle, this would allow us to gradually document more and more complex pathways and their interactions in the human patients.
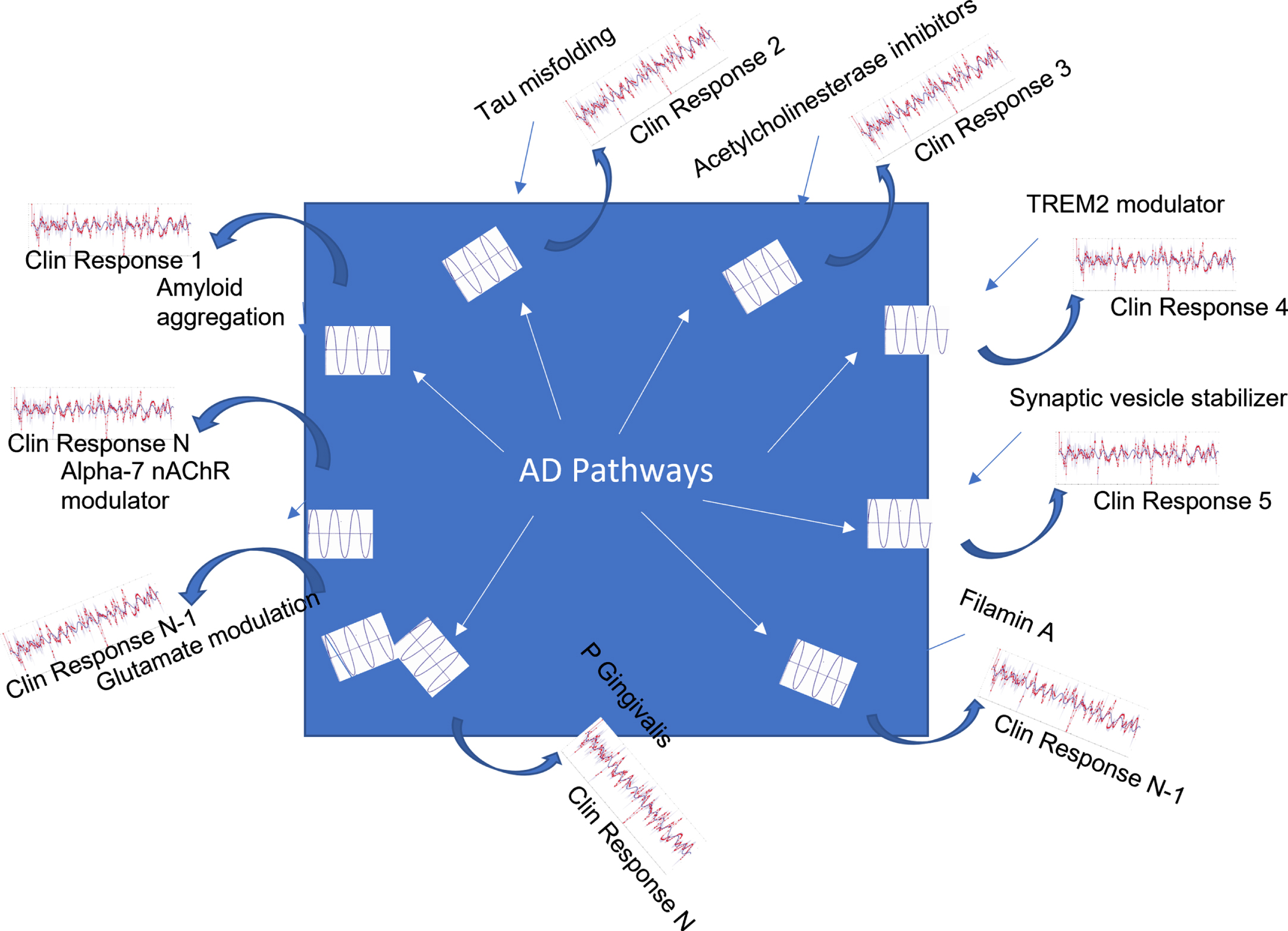
Group average outcomes for clinical trials are a good starting point, but the scope and dynamic range of possibilities can be drastically extended by studying individual patient responses. Physiology-based pharmacokinetic modeling can simulate the effect of comedications and genotypes in metabolizing enzymes on plasma levels and drug exposure. By taking into account specific drug exposure and pharmacodynamic interactions with comedications and genotypes, the possible range of interventions and outcomes can be drastically increased. Quantitative systems pharmacology also allows to simulate the impact of comedications (based on their known pharmacology), common genotypes variants (based on imaging) and disease status on a clinically relevant cognitive readout [30]. This allows to optimally use as many aspects of the actual trial design as possible for reverse engineering, including some “confounding” factors at the individual patient level to maximize the information for deconvolution.
It has to be noted that this modeling approach is not limited to AD but can be applied to other neurodegenerative disorders, as they might share pathological processes, such as spatio-temporal progression of misfolded protein (for example alpha-synuclein in Parkinson’s disease or tau protein in primary tauopathies), neuroinflammation, oxidative stress, mitochondrial dysfunction, and deficient protein degradation.
STRATEGIES FOR SELECTING BEST COMBINATIONS
It is evident from the previous discussion that well validated approaches with a large capacity are needed to systematically screen and prioritize the many possible combinations. The most efficient approach is to focus on pathways and circuits rather than single targets, as some drugs might affect the same pathway or a very similar mode of action.
Experimental approaches
High-throughput screening approaches based on high-content imaging [31] can possibly handle the numerous combinations with some companies, like CombinatorX, (now Zalicus) offering this service as a Contract Research Organization. A freely available software platform allows for visualization and quantification of synergistic effects [32]. Human IPSC cell cultures can provide a strong translational avenue for testing neuroprotective compounds. Such an approach is basically target-agnostic and does not rely on assumptions of the pharmacology of the drugs to be tested. Drawbacks include the challenge to differentiate the induced neuronal-type cells, the need to physically acquire reference compounds for all the pathways involved in the disease (which might be partially mitigated by CRISPRi/a genetic approaches), and the challenge of reproducing the right balance between different cell types present in the human brain, although 3D cultures can provide a more relevant environment [33]. Another challenge is the capacity and reproducibility of these cellular assays. For instance, the expression of 3R and 4R tau isoforms is highly dependent upon maturation stage and it may take several months with specific disease associated mutations to achieve the right balance of 3R:4R tau [34]. Finally, these experimental approaches at this point in their development are not yet able to fully reproduce the complex activity of neuronal circuits in the human brain that is more directly associated with clinical phenotype.
Other approaches using mouse chimeric models with human IPSC cells [35], non-human primates, especially marmosets [36] and sophisticated transgene mouse models based on late-onset AD genes [37] do reflect the complex interaction of many processes involved and bridge some of the translational gap, but are notoriously low-throughput and have limited capacity.
Data-driven computational approaches
The availability of large longitudinal database cohorts together with increased hardware capacity and software sophistication has allowed for pattern recognition type analytics and identification of drivers for protection against cognitive worsening. An innovative approach combined clinical data from the Rush database with a computer model of the microglia-dependent contribution to AD [38] and resulted in a number of possible combinations of existing and marketed drugs suggesting synergy.
A similar study used the National Alzheimer Coordination Center data on 35,000 patients with 118,000 evaluations in clinical trials [39] and collapsing all medications in 53 mechanism groups to address the questions what interventions in healthy subjects could delay the onset of dementia. While none of the individual groups was found to have a major effect, combinations of up to five active compounds were found to be highly correlated with cognitive performance and protection against dementia. The analysis also identified medications that negatively affected this trajectory.
An interesting approach [40] uses multi-model imaging data (structural MRI, tau and amyloid PET imaging, vascular imaging, functional BOLD fMRI) from individualized patients to create a multi-parameter relationship with cognition. Subsequently a “cost function” is calculated to revert the “pathological” fingerprint back to the healthy case which leads to the identification of combination of optimal high-level interventions. By using imaging from individual subjects this approach allows for individual fingerprint of interventions best suited to the particular patient [41]. However, the lack of concrete examples of drugs affecting these high-level pathways limits the usefulness of this approach.
Knowledge-driven computational approaches for repurposed drug combinations
Repurposing drugs is a fast and relatively inexpensive way for hypothesis testing in a clinical trial. By identifying various pathways documented to be relevant for AD, a combination of 8 available and marketed drugs was proposed to reduce the transition of amnestic MCI to AD [42], although no interaction between the various pathways was assumed and no specific dose or PK-PK interactions were explored. The advantage of considering combinations of existing drugs is that there is substantial knowledge about side-effects, PK, and the corresponding pharmacology. As an example a QSP model of the basal ganglia motor circuit identified four non-dopaminergic drugs from the Prestwick library, a collection of off-patent FDA approved drugs for tremor reduction in Parkinson’s disease [43].
Combining PBPK and QSP (Fig. 2) as described above then allows to account for pharmacokinetic and pharmacodynamic interactions of any new combination and to ensure that all properties of putative combinations are aligned for a synergistic effect. For instance, it is of little use to combine two drugs that have synergistic effects on their pharmacodynamic readout but where one drug significantly reduces exposure of the other drug.
Fig. 2
Possible flow-chart of knowledge-driven QSP identification of optimal treatment combinations. An existing QSP model that includes amyloid-tau pathology modeling, neuroinflammation, and a functional ADAS-Cog calibrated neuronal circuit is informed by preclinical, clinical observational and bio-informatics data. Target exposure of drugs currently tested in clinical trials is implemented using PBPK modeling to derive the functional impact in the QSP model. Calibration and validation are then performed with available clinical data, preferentially on individual patient outcomes. The validated QSP platform can then be used to systematically search all possible drug combinations and rank orders the outcomes for an AD patient with pre-specified baseline conditions. This process can then be repeated for subjects with varying baseline biomarkers conditions, allowing for more “targeted treatment” paradigm for certain baseline biomarkers signatures.
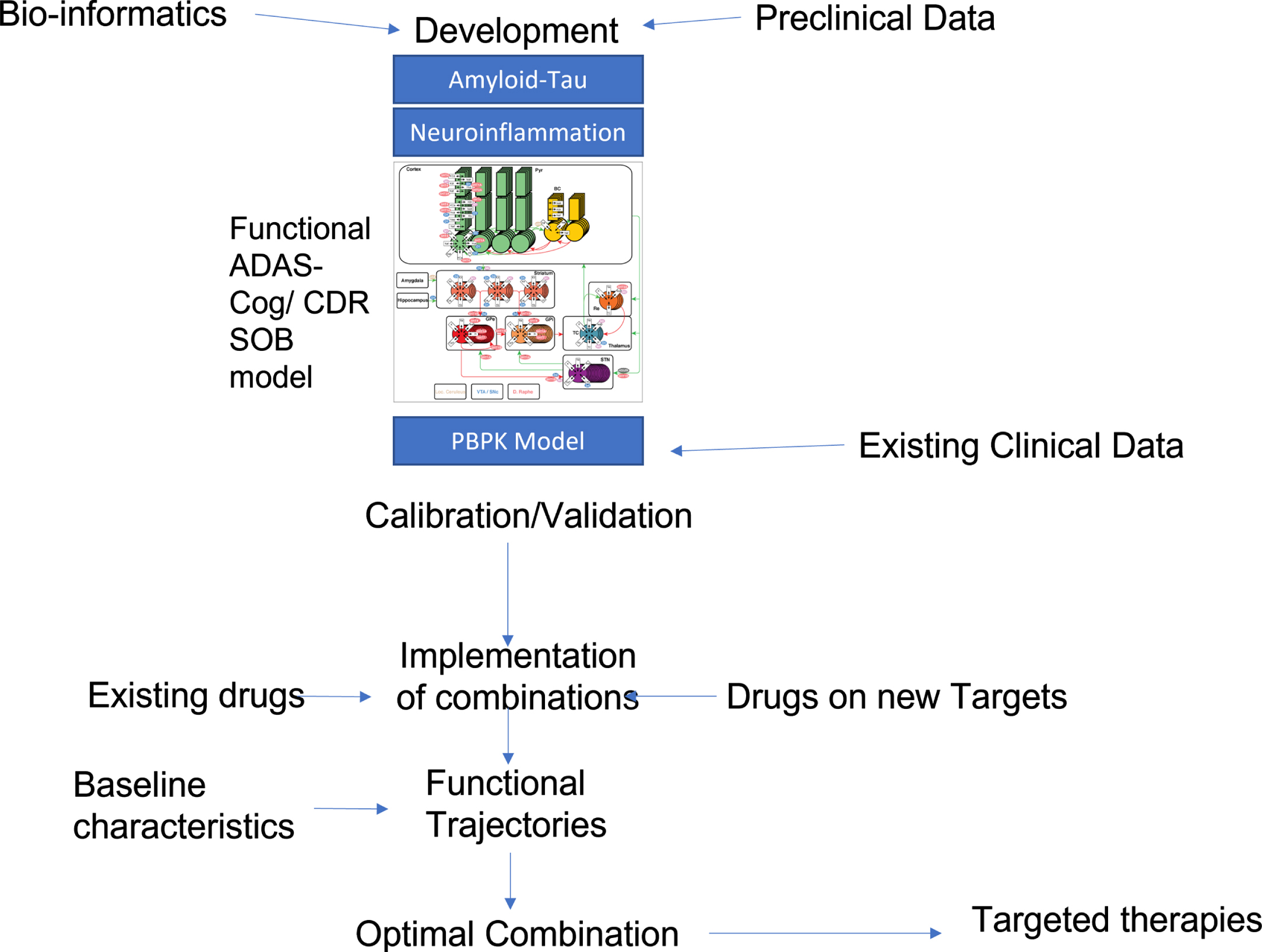
Knowledge-driven computational approaches for rationally designed polypharmacy drug discovery
A possible limitation of drug repurposing strategies is the reliance on existing approved medications that might not have the optimal pharmacology or potency to affect the different pathways or come with dose limitations based on their side-effect profiles. Therefore, there is also a great need for in silico screening approaches for identifying novel molecules that act on the optimal combination of specific key pathways with a distinct synergistic effect. Such an approach is based on a sensitivity analysis or a reverse engineering strategy to identify the pathways with the biggest impact on the functional in silico outcome. As an example, the QSP model for schizophrenia efficacy (PANSS Total) used Design of Experiment (DOE) strategy [44] to identify the pathways that most importantly generate the clinical response of the novel antipsychotic iloperidone. In this case, the most important biological process was identified as the interaction between D4 and AMPA-R on pyramidal cells, corresponding to one of the five SNPs found using a traditional pharmacogenomics analysis on responders versus non-responders [45].
Validation of predicted synergistic interactions
Validation of any assay system is crucial for wider acceptance of these results. Many preclinical transgene mouse models lack translationability to the clinical situation for a number of reasons, including incomplete pathology, mismatched drug exposure, differences in key targes or pathways, and the lack of comedications and genotypes. For computer models, an interesting strategy is to create virtual “mirror” patients with the same characteristics as real patients in longitudinal studies or clinical trials, predict the functional clinical trajectory and compare the outcome with the actual real data on an individual patient basis. While ambitious. this ensures the model platform will be ultimately constrained by clinical data on real patients.
EXAMPLES OF RATIONAL COMBINATION THERAPY
A large number of combination therapies have been tested in clinical studies (for an excellent and comprehensive review, see [46]). Most of the combinations were based upon ad-hoc arguments and so far none have shown substantial synergy. The following lists a few non-obvious combinations that are currently in clinical trials.
Amyloid-amyloid combinations. Because of the availability of different modalities for amyloid modulation, the first combination therapy included an antibody LY3002813, or N3pG mAb against aggregated amyloid and a BACE inhibitor LY3202626. In Nov 2018, this trial was halted for undisclosed reasons, although the single treatment arm with N3pG mAb continued. However, the interest in combining antibody treatment with small-molecule BACE or GSI was significantly decreased after a number of trials reported a transient worsening when interfering with the synthesis of Aβ.
Amyloid-tau modulation. With the approval of aducanumab, a combination with tau-directed drugs should in principle address the two major hallmarks of AD. There are already a number of tau molecules in clinical trial, most of them based on antibodies against specific tau forms and the DIAN-TU study now gears up to include a tau therapeutic arm [47].
Cross-sectional clinical evidence suggests an interaction between amyloid and reduced pathology, a relationship that has been further confirmed in intervention trials reducing amyloid load (for an overview see [48]), On the other hand, therapeutic anti-amyloid antibodies have been documented to activate microglia cells [49] which is necessary to clear substantial amounts of Aβ. The impact of activated microglia cells on tau pathology is complex as preclinical studies suggest a beneficial effect of TREM2 mediated activation on tau pathology only in the presence of amyloid pathology [50] and TREM2 activation can exacerbate tau pathology in a pure tauopathy model [51]. This complicates the timing of combination therapy as microglia can switch to a deleterious phenotype during the decrease in amyloid load.
Other challenges remain as to the specific spatio-temporal progression of tau pathology that might be affected in a complex brain-region dependent way by Aβ load [52] with specific implications on functional outcome. Recent imaging studies suggest four different tau pathology trajectories with varying degrees of interaction with Aβ [53].
It is likely that qualitative arguments on the different course of these two pathologies need to be complemented by more quantitative modeling to account for the complex non-linear relationships between these two processes.
PXT864 is a combination of baclofen, a derivative of GABA and acamprosate a compound used for alcohol dependence currently in Phase II for AD. The rationale is to restore the balance between excitation and inhibition.
ALZT-OPT1 is a combination of dry power inhaled cromolyn and oral ibuprofen that affects neuroinflammation. Cromolyn is a mast-cell stabilizer that has been documented to reduce Aβ plaque formation, while ibuprofen is a non-steroidal anti-inflammatory medication.
AVP-923 combines dextromethorphan, an opioid-type painkiller used in cough syrup with quinidine. Dextrometorphan has weak NMDA antagonism and agonism at the sigma-1 receptor, while quinidine blocks the peripheral metabolism of dextromethorphan therefore increasing the brain permeability. The drug is currently marketed as Nuedexta for pseudobulbar affect and is currently in clinical trials for behavioral problems such as agitation associated with dementia.
AVP-786 is a second generation form of Nuedexta with a deuterated (d6) version of dextromethorphan/quinidine combination. The deuteration is supposed to make dextromethorphan more resistant against CY2D6 mediated metabolization therefore increasing the brain permeability. Initial data from a Phase III study were encouraging and the compound is in further development.
AMX0035 is a combination of two repurposed drugs (sodium phenylbutyrate and taurursodiol) that has shown great promise in a Phase II study in ALS patents [54] resulting in a 6.5 month longer median survival.
CONCLUSION
Despite the increased interest for combination therapy in neurodegenerative diseases, the pharmaceutical challenges remain substantial. Not only is there a need for identifying synergy in drug-drug interactions, at the level of both pharmacokinetics and pharmacodynamics, but the sheer number of possible combinations preclude any systematic experimental approach. This paper reports on a number of computational modeling approaches that could support this process in three ways: 1) prefiltering and rank ordering possible combinations with the highest synergistic potential, 2) identify patient subgroups by generating possible scientific insights related to these potential synergies, and 3) identify the optimal clinical trial design for testing this hypothesis.
By using such in silico models to systematically examine all possible combinations (including dose) in different disease states, combinations can be ranked according to the highest potential for positive synergy before being tested in experimental assays and clinical studies. These approaches can be applied both to repurposing combinations of existing drugs and to supporting rational polypharmacology novel drug discovery.
In contrast to experimental high-throughput approaches, this computational approach is heavily based on clinical data, both with regard to therapeutic interventions as well as imaging and biomarker studies. These in silico approaches might therefore not cover the complete biology; however, they have the advantage to take into account different comorbidities and pathological processes, such as the interaction between amyloid, tau and neuro-immunology in a humanized context which are often difficult to achieve in experimental in vivo models. At the same time, they aim to optimally integrate the biological knowledge from these preclinical models.
Obviously, these in silico models do not claim to capture the full human biology and are based on a vast number of assumptions and simplifications. Other long-term academic endeavors are aimed at developing very comprehensive computer models of human brain circuits with multiple cell types and complex connections [55]. The long-term objective of the QSP approach present here, is to develop relatively simple models of subcircuits that are relevant to clinical outcomes and can be applied to actual clinical questions such as dosing, relative start of the selected treatments, patient selection and trial duration. In that sense there is always a trade-off between scope and granularity on one hand and feasibility and speed on the other hand to generate actionable outcomes that can be useful in the real world.
Ultimately, a trade-off between theoretical optimal outcome and realistic approaches that take into account the full pharmacological and clinical constraints of the various proposed combinations often needs to be made. For instance, combinations with more than four components, while maybe better in theory are much more difficult to test in clinical trials. In this case, a possible alternative strategy would be to identify subtypes of patients that can respond to one of the “leaner” pharmacological profile (i.e., with less targets).
The real challenge of these approaches resides in the validation of the models, and it is likely that a number of iterations will be needed before achieving sufficient confidence. As explained above, focusing on comparing virtual “mirror” patients with actual real clinical data will optimally constrain the model for the systematic screening of relevant combination therapies.
It might be worthwhile to look on how the oncology field is addressing the issue of combination therapy after the “gold standard” of check point inhibition was approved. Many combination therapies are now in clinical trials. Interestingly, a pre-competitive consortium on advanced quantitative systems pharmacology models with 6 pharmaceutical companies [56, 57] was successful in generating useful insights in combination trials. Such an approach could be a template for neuroscience in general and AD in particular.
These strategies that includes biological principles, provide a scientific framework hypothesizing the anticipated synergy and can be extremely helpful to convince stakeholders, such as sponsors, patients, and regulatory agencies. In addition, they can be used to optimize the clinical trial design, especially with regard to possible negative pharmacokinetic and pharmacodynamic interactions between the selected combination and the allowed comedications.
In summary, this report provides a strategy using advanced in silico computer models, not only to better understand the complex neuropathology, but also to address the major challenge of identifying the optimal combination therapy for AD. Quantitative systems pharmacology modeling, informed by a combination of biological and clinical imaging data, allows to integrate a large number of clinically relevant biological processes and might be a powerful tool to support actual combination trials.
ACKNOWLEDGMENTS
HG wants to thank Prof. Lon Schneider for interesting discussions on this topic.
FUNDING
The authors have no funding to report.
CONFLICT OF INTEREST
The authors have no conflict of interest to report.
REFERENCES
[1] | Attems J , Jellinger KA ((2014) ) The overlap between vascular disease and Alzheimer’s disease–lessons from pathology. BMC Med 12: , 206. |
[2] | Foguem C , Manckoundia P ((2018) ) Lewy body disease: clinical and pathological “overlap syndrome” between synucleinopathies (Parkinson disease) and tauopathies (Alzheimer disease). Curr Neurol Neurosci Rep 18: , 24. |
[3] | Katsumata Y , Fardo DW , Kukull WA , Nelson PT ((2018) ) Dichotomous scoring of TDP-43 proteinopathy from specific brain regions in 27 academic research centers: associations with Alzheimer’s disease and cerebrovascular disease pathologies. Acta Neuropathol Commun 6: , 142. |
[4] | Nelson PT , Dickson DW , Trojanowski JQ , Jack CR , Boyle PA , Arfanakis K , Rademakers R , Alafuzoff I , Attems J , Brayne C , Coyle-Gilchrist ITS , Chui HC , Fardo DW , Flanagan ME , Halliday G , Hokkanen SRK , Hunter S , Jicha GA , Katsumata Y , Kawas CH , Keene CD , Kovacs GG , Kukull WA , Levey AI , Makkinejad N , Montine TJ , Murayama S , Murray ME , Nag S , Rissman RA , Seeley WW , Sperling RA , White Iii CL , Yu L , Schneider JA ((2019) ) Limbic-predominant age-related TDP-43 encephalopathy (LATE): consensus working group report. Brain 142: , 1503–1527. |
[5] | Geerts H , Barrett JE ((2019) ) Neuronal circuit-based computer modeling as a phenotypic strategy for CNS R&D. Front Neurosci 13: , 723. |
[6] | Geerts H , Kennis L ((2014) ) Multitarget drug discovery projects in CNS diseases: quantitative systems pharmacology as a possible path forward. Future Med Chem 6: , 1757–1769. |
[7] | Swinney DC , Anthony J ((2011) ) How were new medicines discovered? Nat Rev Drug Discov 10: , 507–519. |
[8] | Dedic N , Jones PG , Hopkins SC , Lew R , Shao L , Campbell JE , Spear KL , Large TH , Campbell UC , Hanania T , Leahy E , Koblan KS ((2019) ) SEP-363856, a novel psychotropic agent with a unique, non-D2 receptor mechanism of action. J Pharmacol Exp Ther 371: , 1–14. |
[9] | Graham DY , Fischbach L ((2010) ) Helicobacter pylori treatment in the era of increasing antibiotic resistance. Gut 59: , 1143–1153. |
[10] | Mbuagbaw L , Mursleen S , Irlam JH , Spaulding AB , Rutherford GW , Siegfried N ((2016) ) Efavirenz or nevirapine in three-drug combination therapy with two nucleoside or nucleotide-reverse transcriptase inhibitors for initial treatment of HIV infection in antiretroviral-naive individuals. Cochrane Database Syst Rev 12: , CD004246. |
[11] | Bakris G , Ali W , Parati G ((2019) ) ACC/AHA versus ESC/ESH on hypertension guidelines: JACC Guideline Comparison. J Am Coll Cardiol 73: , 3018–3026. |
[12] | Clague F , Mercer SW , McLean G , Reynish E , Guthrie B ((2017) ) Comorbidity and polypharmacy in people with dementia: insights from a large, population-based cross-sectional analysis of primary care data. Age Ageing 46: , 33–39. |
[13] | Gray SL , Anderson ML , Dublin S , Hanlon JT , Hubbard R , Walker R , Yu O , Crane PK , Larson EB ((2015) ) Cumulative use of strong anticholinergics and incident dementia: a prospective cohort study. JAMA Intern Med 175: , 401–407. |
[14] | Risacher SL , McDonald BC , Tallman EF , West JD , Farlow MR , Unverzagt FW , Gao S , Boustani M , Crane PK , Petersen RC , Jack CR Jr. , Jagust WJ , Aisen PS , Weiner MW , Saykin AJ ((2016) ) Association between anticholinergic medication use and cognition, brain metabolism, and brain atrophy in cognitively normal older adults. JAMA Neurol 73: , 721–732. |
[15] | Wimmer BC , Bell JS , Fastbom J , Wiese MD , Johnell K ((2016) ) Medication regimen complexity and number of medications as factors associated with unplanned hospitalizations in older people: a population-based cohort study. J Gerontol A Biol Sci Med Sci 71: , 831–837. |
[16] | Lau DT , Mercaldo ND , Shega JW , Rademaker A , Weintraub S ((2011) ) Functional decline associated with polypharmacy and potentially inappropriate medications in community-dwelling older adults with dementia. Am J Alzheimers Dis Other Demen 26: , 606–615. |
[17] | Amanatkar HR , Papagiannopoulos B , Grossberg GT ((2017) ) Analysis of recent failures of disease modifying therapies in Alzheimer’s disease suggesting a new methodology for future studies. Expert Rev Neurother 17: , 7–16. |
[18] | Yang X , Wang Y , Byrne R , Schneider G , Yang S ((2019) ) Concepts of artificial intelligence for computer-assisted drug discovery. Chem Rev 119: , 10520–10594. |
[19] | Berman H , Henrick K , Nakamura H ((2003) ) Announcing the worldwide Protein Data Bank. Nat Struct Biol 10: , 980. |
[20] | Kellenberger E , Rodrigo J , Muller P , Rognan D ((2004) ) Comparative evaluation of eight docking tools for docking and virtual screening accuracy. Proteins 57: , 225–242. |
[21] | Geerts H ((2009) ) Of mice and men: bridging the translational disconnect in CNS drug discovery. CNS Drugs 23: , 915–926. |
[22] | Geerts H , Wikswo J , van der Graaf PH , Bai JPF , Gaiteri C , Bennett D , Swalley SE , Schuck E , Kaddurah-Daouk R , Tsaioun K , Pelleymounter M ((2020) ) Quantitative systems pharmacology for neuroscience drug discovery and development: current status, opportunities, and challenges. CPT Pharmacometrics Syst Pharmacol 9: , 5–20. |
[23] | Catterall WA , Raman IM , Robinson HP , Sejnowski TJ , Paulsen O ((2012) ) The Hodgkin-Huxley heritage: from channels to circuits. J Neurosci 32: , 14064–14073. |
[24] | Roberts PD , Spiros A , Geerts H ((2012) ) Simulations of symptomatic treatments for Alzheimer’s disease: computational analysis of pathology and mechanisms of drug action. Alzheimers Res Ther 4: , 50. |
[25] | Geerts H , Spiros A , Roberts P ((2018) ) Impact of amyloid-beta changes on cognitive outcomes in Alzheimer’s disease: analysis of clinical trials using a quantitative systems pharmacology model. Alzheimers Res Ther 10: , 14. |
[26] | Geerts H , Spiros A ((2020) ) Learning from amyloid trials in Alzheimer’s disease. A virtual patient analysis using a quantitative systems pharmacology approach. Alzheimers Dement 16: , 862–872. |
[27] | Diaz K , Spiros A , Jeromin A , Haas M , Geerts H ((2019) ) A computer model simulating the effect of tau antibodies on the inter-cellular spread of tau. Alzheimers Dement 15: , P1289. |
[28] | Spiros A , Geerts H ((2014) ) New insights into brain amyloid kinetics using a human computational modeling study. Alzheimers Dement 10: , P314. |
[29] | Spiros A , Geerts H ((2012) ) A quantitative way to estimate clinical off-target effects for human membrane brain targets in CNS research and development. J Exp Pharmacol 4: , 53–61. |
[30] | Geerts H , Spiros A ((2020) ) Simulating the effects of common comedications and genotypes on Alzheimer’s cognitive trajectory using a quantitative systems pharmacology approach. J Alzheimers Dis 78: , 413–424. |
[31] | Cheng C , Fass DM , Folz-Donahue K , MacDonald ME , Haggarty SJ ((2017) ) Highly expandable human iPS cell-derived neural progenitor cells (NPC) and neurons for central nervous system disease modeling and high-throughput screening. Curr Protoc Hum Genet 92: , 21.8.1–21.8.21. |
[32] | Di Veroli GY , Fornari C , Wang D , Mollard S , Bramhall JL , Richards FM , Jodrell DI ((2016) ) Combenefit: an interactive platform for the analysis and visualization of drug combinations. Bioinformatics 32: , 2866–2868. |
[33] | Park J , Wetzel I , Marriott I , Dreau D , D’Avanzo C , Kim DY , Tanzi RE , Cho H ((2018) ) A 3D human triculture system modeling neurodegeneration and neuroinflammation in Alzheimer’s disease. Nat Neurosci 21: , 941–951. |
[34] | Iovino M , Agathou S , Gonzalez-Rueda A , Del Castillo Velasco-Herrera M , Borroni B , Alberici A , Lynch T , O’Dowd S , Geti I , Gaffney D , Vallier L , Paulsen O , Karadottir RT , Spillantini MG ((2015) ) Early maturation and distinct tau pathology in induced pluripotent stem cell-derived neurons from patients with MAPT mutations. Brain 138: , 3345–3359. |
[35] | Tong G , Izquierdo P , Raashid RA ((2017) ) Human induced pluripotent stem cells and the modelling of Alzheimer’s disease: the human brain outside the dish. n Neurol J 11: , 27–38. |
[36] | Freire-Cobo C , Edler MK , Varghese M , Munger E , Laffey J , Raia S , In SS , Wicinski B , Medalla M , Perez SE , Mufson EJ , Erwin JM , Guevara EE , Sherwood CC , Luebke JI , Lacreuse A , Raghanti MA , Hof PR ((2021) ) Comparative neuropathology in aging primates: A perspective. Am J Primatol 83: , e23299. |
[37] | Oblak AL , Forner S , Territo PR , Sasner M , Carter GW , Howell GR , Sukoff-Rizzo SJ , Logsdon BA , Mangravite LM , Mortazavi A , Baglietto-Vargas D , Green KN , MacGregor GR , Wood MA , Tenner AJ , LaFerla FM , Lamb BT ((2020) ) Model organism development and evaluation for late-onset Alzheimer’s disease: MODEL-AD. Alzheimers Dement 6: , e12110. |
[38] | Anastasio TJ ((2019) ) Exploring the correlation between the cognitive benefits of drug combinations in a clinical database and the efficacies of the same drug combinations predicted from a computational model. J Alzheimers Dis 70: , 287–302. |
[39] | Mayburd AL , Koivogui M , Baranova A ((2019) ) Pharmacological signatures of the reduced incidence and the progression of cognitive decline in ageing populations suggest the protective role of beneficial polypharmacy. PLoS One 14: , e0224315. |
[40] | Iturria-Medina Y , Carbonell FM , Sotero RC , Chouinard-Decorte F , Evans AC ((2017) ) Multifactorial causal model of brain (dis)organization and therapeutic intervention: Application to Alzheimer’s disease. Neuroimage 152: , 60–77. |
[41] | Iturria-Medina Y , Carbonell FM , Evans AC ((2018) ) Multimodal imaging-based therapeutic fingerprints for optimizing personalized interventions: Application to neurodegeneration. Neuroimage 179: , 40–50. |
[42] | Fessel J ((2019) ) Prevention of Alzheimer’s disease by treating mild cognitive impairment with combinations chosen from eight available drugs. Alzheimers Dement 5: , 780–788. |
[43] | Spiros A , Roberts P , Geerts H ((2013) ) Phenotypic screening of the Prestwick library for treatment of Parkinson’s tremor symptoms using a humanized quantitative systems pharmacology platform. J Parkinsons Dis 3: , 569–580. |
[44] | Geerts H , Roberts P , Spiros A , Potkin S ((2015) ) Understanding responder neurobiology in schizophrenia using a quantitative systems pharmacology model: application to iloperidone. J Psychopharmacol 29: , 372–382. |
[45] | Lavedan C , Licamele L , Volpi S , Hamilton J , Heaton C , Mack K , Lannan R , Thompson A , Wolfgang CD , Polymeropoulos MH ((2008) ) Association of the NPAS3 gene and five other loci with response to the antipsychotic iloperidone identified in a whole genome association study. Mol Psychiatry 14: , 804–819. |
[46] | Kabir MT , Uddin MS , Mamun AA , Jeandet P , Aleya L , Mansouri RA , Ashraf GM , Mathew B , Bin-Jumah MN , Abdel-Daim MM ((2020) ) Combination drug therapy for the management of Alzheimer’s disease. Int J Mol Sci 21: , 3272. |
[47] | Aisen PS , Bateman RJ , Carrillo M , Doody R , Johnson K , Sims JR , Sperling R , Vellas B ((2021) ) Platform trials to expedite drug development in Alzheimer’s disease: a report from the EU/US CTAD Task Force. J Prev Alzheimers Dis 8: , 306–312. |
[48] | Avgerinos KI , Ferrucci L , Kapogiannis D ((2021) ) Effects of monoclonal antibodies against amyloid-beta on clinical and biomarker outcomes and adverse event risks: A systematic review and meta-analysis of phase III RCTs in Alzheimer’s disease. Ageing Res Rev 68: , 101339. |
[49] | Crehan H , Liu B , Kleinschmidt M , Rahfeld JU , Le KX , Caldarone BJ , Frost JL , Hettmann T , Hutter-Paier B , O’Nuallain B , Park MA , DiCarli MF , Lues I , Schilling S , Lemere CA ((2020) ) Effector function of anti-pyroglutamate-3 Abeta antibodies affects cognitive benefit, glial activation and amyloid clearance in Alzheimer’s-like mice. Alzheimers Res Ther 12: , 12. |
[50] | Lee SH , Meilandt WJ , Xie L , Gandham VD , Ngu H , Barck KH , Rezzonico MG , Imperio J , Lalehzadeh G , Huntley MA , Stark KL , Foreman O , Carano RAD , Friedman BA , Sheng M , Easton A , Bohlen CJ , Hansen DV ((2021) ) Trem2 restrains the enhancement of tau accumulation and neurodegeneration by beta-amyloid pathology. Neuron 109: , 1283–1301.e1286. |
[51] | Leyns CEG , Ulrich JD , Finn MB , Stewart FR , Koscal LJ , Remolina Serrano J , Robinson GO , Anderson E , Colonna M , Holtzman DM ((2017) ) TREM2 deficiency attenuates neuroinflammation and protects against neurodegeneration in a mouse model of tauopathy. Proc Natl Acad Sci U S A 114: , 11524–11529. |
[52] | Villemagne VL , Dore V , Burnham SC , Masters CL , Rowe CC ((2018) ) Imaging tau and amyloid-beta proteinopathies in Alzheimer disease and other conditions. Nat Rev Neurol 14: , 225–236. |
[53] | Vogel JW , Young AL , Oxtoby NP , Smith R , Ossenkoppele R , Strandberg OT , La Joie R , Aksman LM , Grothe MJ , Iturria-Medina Y , Pontecorvo MJ , Devous MD , Rabinovici GD , Alexander DC , Lyoo CH , Evans AC , Hansson O ((2021) ) Four distinct trajectories of tau deposition identified in Alzheimer’s disease. Nat Med 27: , 871–881. |
[54] | Paganoni S , Hendrix S , Dickson SP , Knowlton N , Macklin EA , Berry JD , Elliott MA , Maiser S , Karam C , Caress JB , Owegi MA , Quick A , Wymer J , Goutman SA , Heitzman D , Heiman-Patterson TD , Jackson CE , Quinn C , Rothstein JD , Kasarskis EJ , Katz J , Jenkins L , Ladha S , Miller TM , Scelsa SN , Vu TH , Fournier CN , Glass JD , Johnson KM , Swenson A , Goyal NA , Pattee GL , Andres PL , Babu S , Chase M , Dagostino D , Hall M , Kittle G , Eydinov M , McGovern M , Ostrow J , Pothier L , Randall R , Shefner JM , Sherman AV , St Pierre ME , Tustison E , Vigneswaran P , Walker J , Yu H , Chan J , Wittes J , Yu ZF , Cohen J , Klee J , Leslie K , Tanzi RE , Gilbert W , Yeramian PD , Schoenfeld D , Cudkowicz ME ((2021) ) Long-term survival of participants in the CENTAUR trial of sodium phenylbutyrate-taurursodiol in amyotrophic lateral sclerosis. Muscle Nerve 63: , 31–39. |
[55] | Markram H ((2006) ) The blue brain project. Nat Rev Neurosci 7: , 153–160. |
[56] | Chelliah V , Lazarou G , Bhatnagar S , Gibbs JP , Nijsen M , Ray A , Stoll B , Thompson RA , Gulati A , Soukharev S , Yamada A , Weddell J , Sayama H , Oishi M , Wittemer-Rump S , Patel C , Niederalt C , Burghaus R , Scheerans C , Lippert J , Kabilan S , Kareva I , Belousova N , Rolfe A , Zutshi A , Chenel M , Venezia F , Fouliard S , Oberwittler H , Scholer-Dahirel A , Lelievre H , Bottino D , Collins SC , Nguyen HQ , Wang H , Yoneyama T , Zhu AZX , van der Graaf PH , Kierzek AM ((2021) ) Quantitative systems pharmacology approaches for immuno-oncology: adding virtual patients to the development paradigm. Clin Pharmacol Ther 109: , 605–618. |
[57] | Lazarou G , Chelliah V , Small BG , Walker M , van der Graaf PH , Kierzek AM ((2020) ) Integration of omics data sources to inform mechanistic modeling of immune-oncology therapies: a tutorial for clinical pharmacologists. Clin Pharmacol Ther 107: , 858–870. |