A computed tomography-based score indicative of lung cancer aggression (SILA) predicts lung adenocarcinomas with low malignant potential or vascular invasion
Abstract
BACKGROUND:
Histologic grading of lung adenocarcinoma (LUAD) is predictive of outcome but is only possible after surgical resection. A radiomic biomarker predictive of grade has the potential to improve preoperative management of early-stage LUAD.
OBJECTIVE:
Validate a prognostic radiomic score indicative of lung cancer aggression (SILA) in surgically resected stage I LUAD (
METHODS:
The SILA scores were generated from preoperative CT-scans using the previously validated Computer-Aided Nodule Assessment and Risk Yield (CANARY) software.
RESULTS:
Cox proportional regression showed significant association between the SILA and 7-year recurrence-free survival (RFS) in a univariate (
CONCLUSIONS:
The SILA scoring of preoperative CT scans was prognostic and predictive of resected pathologic grade.
1.Introduction
Lung cancer (LC) is the deadliest cancer in the United States (U.S.) with an estimated 238,340 new cases and 127,070 deaths in 2023 [1]. However, lung cancer mortality has begun to decline in part due to declining rates of cigarette smoking and more recently the widespread implementation of low-dose computed tomography (CT) screening programs that have led to detection at earlier stages where curative surgery is possible [1]. Despite the mortality reduction associated with LC screening, CT screening results in an increase in overdiagnosis leading to higher morbidity, financial burden, and stress among patients [2, 3]. Accurate pre-surgical prognostic markers are needed to personalize the management of early stage LC. Indolent tumors may be able to be treated with non-surgical approaches such as stereotactic body radiation therapy (SBRT), cryoablation, microwave ablation, or radiofrequency ablation (RFA) [4]. New clinical trials also indicate that a subset of early stage LC can be adequately managed with sublobar resection rather than standard of care lobectomy [5, 6]. On the other hand, patients with aggressive disease and high risk of recurrence may benefit from adjuvant or neoadjuvant systemic therapy, which is not standard of care for stage I disease [7, 8]. Tumor histopathology is highly prognostic, but it requires comprehensive histologic examination that is only possible after complete surgical excision [9]. Small biopsies, such as those obtained via bronchoscopy or CT-guided biopsy, are able to establish a diagnosis of LC and distinguish between LC subtypes, but cannot reliably provide the same level of prognostic information as resected specimens due to limited sampling, tumor heterogeneity, and crush artifact [10]. Widespread validation and clinical implementation of machine learning approaches that can predict prognostic histologic patterns and features from CT scans are an important approach to improve clinical management of early-stage tumors.
Lung adenocarcinoma (LUAD) is the most common subtype of LC overall, accounts for virtually all cases among light and never smokers, and is heterogeneous in its histologic patterns, features and prognosis [9]. In the National Lung Screening Trial (NLST), overdiagnosis was high (79%) among a subset of LUAD historically termed “bronchoalveolar carcinoma” (BAC), which comprised 27% of all LUAD detected by CT-screening [3, 11, 12]. Since the NLST, BAC has been discontinued as a diagnostic entity and replaced with adenocarcinoma in situ (AIS) and minimally invasive adenocarcinoma (MIA) which exhibit 100% disease-free survival (DFS) after excision, but together make up only
Recently, a proposed histopathology classification of stage I LUADs as low malignant potential (LMP) with 100% DFS that includes AIS and MIA, accounted for 23% of stage I LUAD, reflecting a similar proportion of cases as was reported as overdiagnosed stage I BAC in NLST [14]. In contrast to LMP, there are tumor invasive characteristics that are associated with poor prognosis. Vascular invasion (VI), a pathological hallmark of cancer pre-metastasis and a strong predictor of recurrence, cancer specific and overall mortality in patients with early-stage LUAD, even among tumors
We sought to evaluate the ability of a previously published CT scan-based method to distinguish between stage I LUAD classified as indolent (AIS/MIA/LMP), aggressive (VI), and intermediate grade (NST-no special type) at the time of resection. Computer Aided Nodule Assessment and Risk Yield (CANARY) is a software for automated risk assessment of adenocarcinoma based on of the clustering of voxel density histograms into nine clusters or exemplars named after colors [20]. Multidimensional scaling showed these nine exemplars clustered into three groups that visually corresponded to ground-glass appearance, solid appearance, and intermediate density. CANARY was originally designed and validated to distinguish invasive adenocarcinomas from AIS/MIA [20, 21]. Subsequently, three CANARY risk groups were defined and association with patient outcomes were validated, independent of histology, in two retrospective surgical lung adenocarcinoma cohorts, including the NLST [22, 23]. The good risk group among pathologic stage I adenocarcinoma was associated with 100% disease specific survival (DSS) in both cohorts. Interestingly, the good risk group represented 17% and 18% of pathologic stage I tumors in these cohorts, far exceeding the expected rate of AIS/MIA (
2.Materials and methods
2.1Clinical samples and pathology review
A retrospective cohort of 161 patients who were treated with surgery between 2005–2014 for pathologic stage I/0 LUAD were included in this study, representing a subset of a previously reported cohort [14, 18]. Tumors measuring
2.2CANARY analysis
CANARY Plus software version 1.0 was licensed from Mayo Clinic. CANARY has previously been demonstrated to have low inter-observer variability for segmenting and analyzing LUAD CT scans [28]. All CT scans were reviewed by an experienced board-certified thoracic radiologist at the time of clinical diagnosis. CT scans were acquired using a variety of scanners, with the majority (96.3%) acquired on one scanner. As part of this retrospective study, we collaborated with an experienced board-certified thoracic surgeon (KS) who confirmed that the nodule location on the CT scan matched the resected nodule on the original clinical report, and that adequate masking was performed by the CANARY nodule detection algorithm. The SILA and associated exemplars were generated by CANARY and exported for further analysis. The nine exemplars were previously named based on nine arbitrary colors: blue (B), cyan (C), green (G), yellow (Y), pink (P), violet (V), indigo (I), red (R), and orange (O) [20].
2.3Statistical analysis
All statistical analysis was performed with R version 4.2.1. Tables were created with the tableone package. Comparisons of distributions of count data were tested with chisq.test. Correlations were performed using spearman correlation with stat_cor or cor.test. Comparisons of distributions of continuous data were tested with wilcox.test or t.test, as specified.
Table 1
Clinical and pathologic characteristics of 161 patients with resected stage I LUAD included in the study
Overall | |
---|---|
| 161 |
Age (mean (SD)) | 67.30 (9.59) |
Gender | |
Female | 97 (60.2) |
Male | 64 (39.8) |
Race | |
Asian | 13 (8.1) |
Black/African American | 50 (31.1) |
Hispanic/Latino | 5 (3.1) |
Unknown | 8 (5.0) |
White | 85 (52.8) |
Pack years (mean (SD)) | 38.76 (33.67) |
Smoking Status | |
Never | 22 (14.2) |
Former | 73 (47.1) |
Current | 60 (38.7) |
Procedure | |
Lobe | 103 (64.0) |
Segment | 8 (5.0) |
Wedge | 50 (31.1) |
Invasive size (mean (SD)) | 1.39 (0.79) |
Total size (mean (SD)) | 1.86 (0.84) |
WHO 2021 grade | |
AIS/MIA | 4 (2.5) |
G1 | 28 (17.4) |
G2 | 43 (26.7) |
G3 | 80 (49.7) |
M | 6 (3.7) |
Novel grade | |
LMP | 27 (16.8) |
NST | 87 (54.0) |
VI | 47 (29.2) |
Recurrence | 20 (12.4) |
Follow-up years (mean (SD)) | 5.95 (3.42) |
Note: The data are shown as the number and (%) unless otherwise indicated. Abbreviations: AIS, adenocarcinoma in situ; MIA, minimally invasive adenocarcinoma; G1, grade 1; G2, grade 2; G3, grade 3; M, mucinous, LMP, low malignant potential; NST, no special type; VI, vascular invasion.
3.Results
3.1Patient and tumor characteristics
Table 1 shows the clinical and pathologic characteristics of 161 patients with resected stage I LUAD included in the study. The mean age was 67.3 years. Most patients were female (60%), self-identified as white (53%), were former (47%) smokers, and were treated with lobectomy (64%). The patients in the study had an overall 7-year RFS of 88% with a mean follow-up time of 5.95 years. Kaplan-Meier estimation showed a significant difference in both RFS and DSS among grades from both the WHO 2021 grading (
Figure 1.
The SILA is associated with recurrence-free survival in a cohort of resected stage I LUAD. (A) Distribution of the SILA by prognostic subgroup using previously established cutoffs (Varghese et al., 2019). (B) Kaplan Meier curve of the SILA prognostic subgroups with 7-year RFS. (C) Univariate cox proportional hazard model of the SILA predicting 7-year RFS. (D) Multivariate cox proportional hazard model of the SILA predicting 7-year RFS, with pack years, smoking status, gender, age, and surgical procedure as covariates.
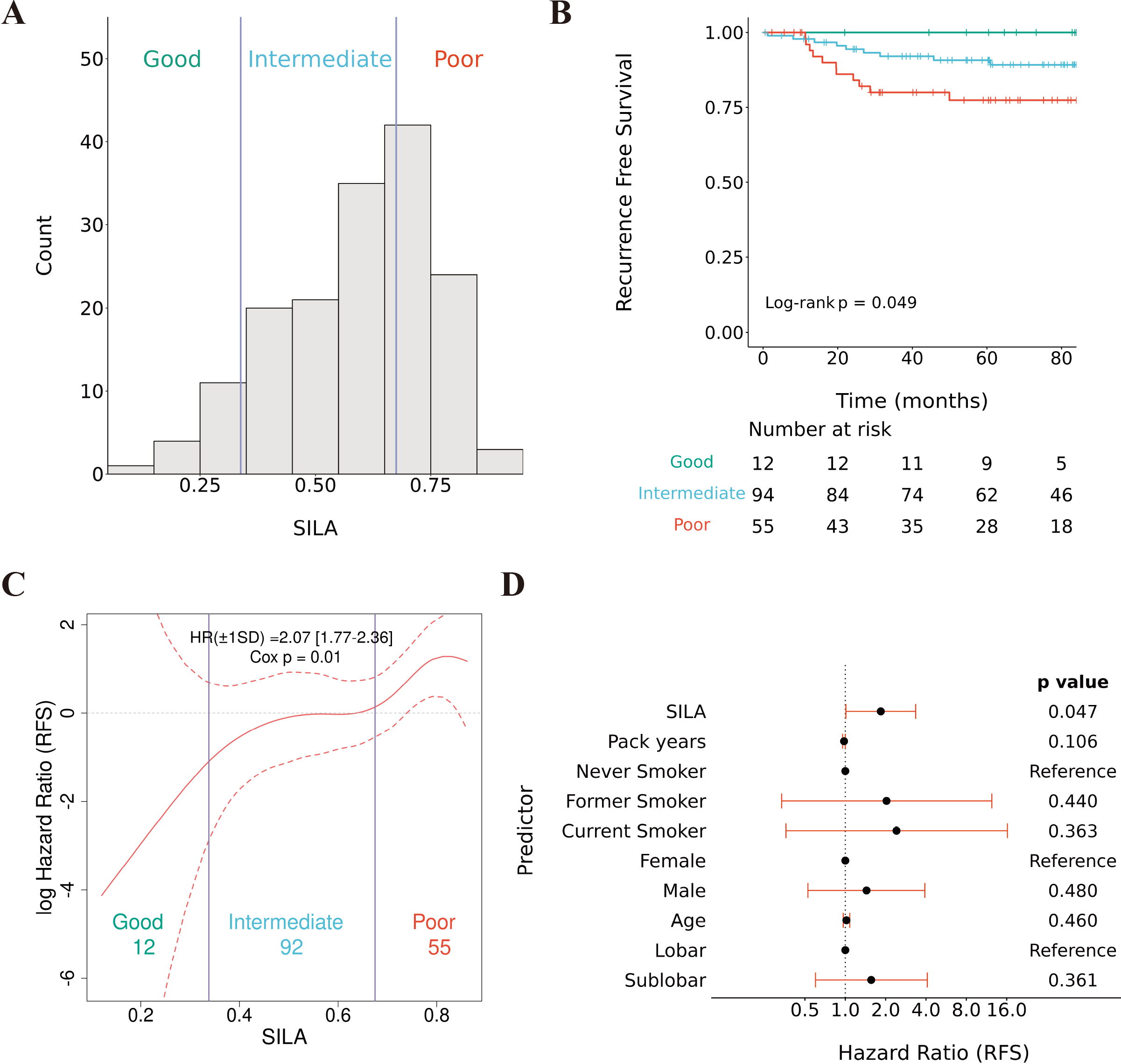
Table 2
Clinical and pathologic characteristics of resected stage I LUAD classified by the SILA prognostic subgroups
Good | Intermediate | Poor | ||
---|---|---|---|---|
| 12 | 94 | 55 | |
SILA (mean (SD)) | 0.26 (0.06) | 0.54 (0.10) | 0.75 (0.05) | |
Age (mean (SD)) | 65.58 (11.04) | 67.48 (9.43) | 67.36 (9.68) | 0.81 |
Gender (%) | 0.03 | |||
Female | 11 (91.7) | 58 (61.7) | 28 (50.9) | |
Male | 1 (8.3) | 36 (38.3) | 27 (49.1) | |
Race (%) | 0.646 | |||
Asian | 1 (8.3) | 10 (10.6) | 2 (3.6) | |
Black/African American | 2 (16.7) | 27 (28.7) | 21 (38.2) | |
Hispanic/Latino | 1 (8.3) | 2 (2.1) | 2 (3.6) | |
Unknown | 1 (8.3) | 4 (4.3) | 3 (5.5) | |
White | 7 (58.3) | 51 (54.3) | 27 (49.1) | |
Pack years (mean (SD)) | 24.58 (18.37) | 38.77 (36.78) | 42.01 (30.22) | 0.272 |
Smoking Status (%) | 0.009 | |||
Never | 3 (25.0) | 17 (18.9) | 2 (3.8) | |
Former | 2 (16.7) | 46 (51.1) | 25 (47.2) | |
Current | 7 (58.3) | 27 (30.0) | 26 (49.1) | |
Procedure (%) | 0.815 | |||
Lobe | 6 (50.0) | 60 (63.8) | 37 (67.3) | |
Segment | 1 (8.3) | 4 (4.3) | 3 (5.5) | |
Wedge | 5 (41.7) | 30 (31.9) | 15 (27.3) | |
Invasive size (mean (SD)) | 0.63 (0.39) | 1.21 (0.65) | 1.87 (0.82) | |
Total size (mean (SD)) | 1.33 (0.60) | 1.76 (0.82) | 2.14 (0.85) | 0.002 |
WHO 2021 grade (%) | ||||
AIS/MIA | 2 (16.7) | 2 (2.1) | 0 (0.0) | |
G1 | 3 (25.0) | 22 (23.4) | 3 (5.5) | |
G2 | 5 (41.7) | 19 (20.2) | 19 (34.5) | |
G3 | 1 (8.3) | 46 (48.9) | 33 (60.0) | |
M | 1 (8.3) | 5 (5.3) | 0 (0.0) | |
Novel grade (%) | ||||
LMP | 7 (58.3) | 17 (18.1) | 3 (5.5) | |
NST | 3 (25.0) | 61 (64.9) | 23 (41.8) | |
VI | 2 (16.7) | 16 (17.0) | 29 (52.7) |
Note: The data are shown as the number and (%) unless otherwise indicated. Abbreviations: SILA, score indicative of lung cancer aggression; AIS, adenocarcinoma in situ; MIA, minimally invasive adenocarcinoma; G1, grade 1; G2, grade 2; G3, grade 3; M, metachronous, LMP, low malignant potential; NST, no special type; VI, vascular invasion.
3.2The SILA is associated with recurrence-free survival
The SILA scores were binned into good (
Figure 2.
The SILA is associated with pathologic grade at resection. (A) The SILA correlation with invasive size at resection. (B) The SILA correlation with percentage of lepidic growth pattern, measured at resection. (C) The SILA association with WHO 2021 grading criteria. (D) The SILA association with novel pathology grading criteria.
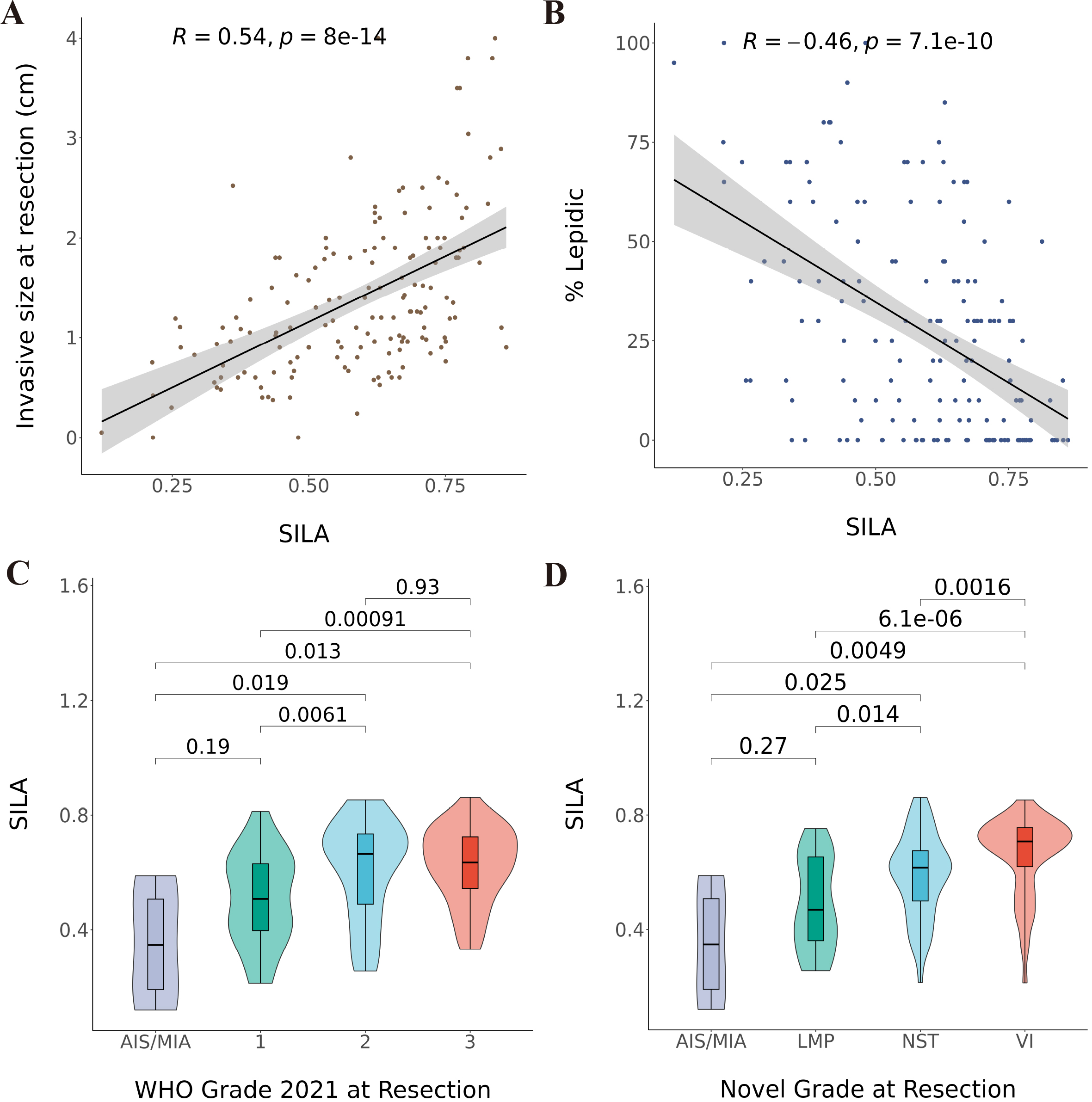
Figure 3.
The SILA performance for predicting LMP and VI stage I LUAD tumors. (A) ROC curve of the SILA predicting cases of LMP (including AIS/MIA) (Wilcoxon
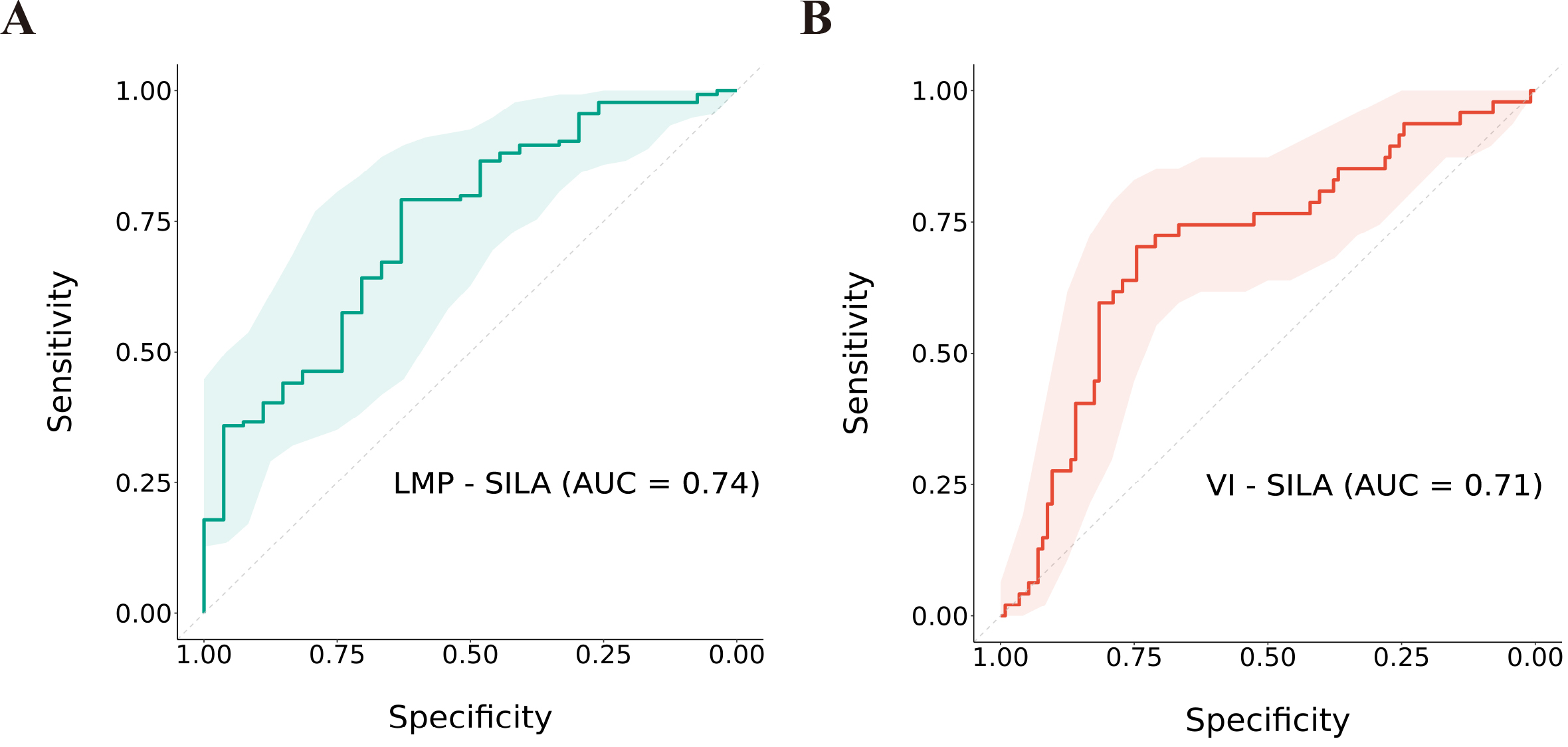
3.3The SILA is associated with pathologic grade at resection
Given that the SILA has previously been reported as linearly increasing with invasive size (non-lepidic tumor size) at resection [24], we sought to validate this in our cohort and examine associations with other pathology features observable in the resected tumor. The SILA was positively correlated with invasive size at resection (
Figure 4.
The CANARY red exemplar is associated with VI at resection. (A) Correlation matrix of CANARY exemplars with percentages of different LUAD histologic growth patterns (*indicates FDR
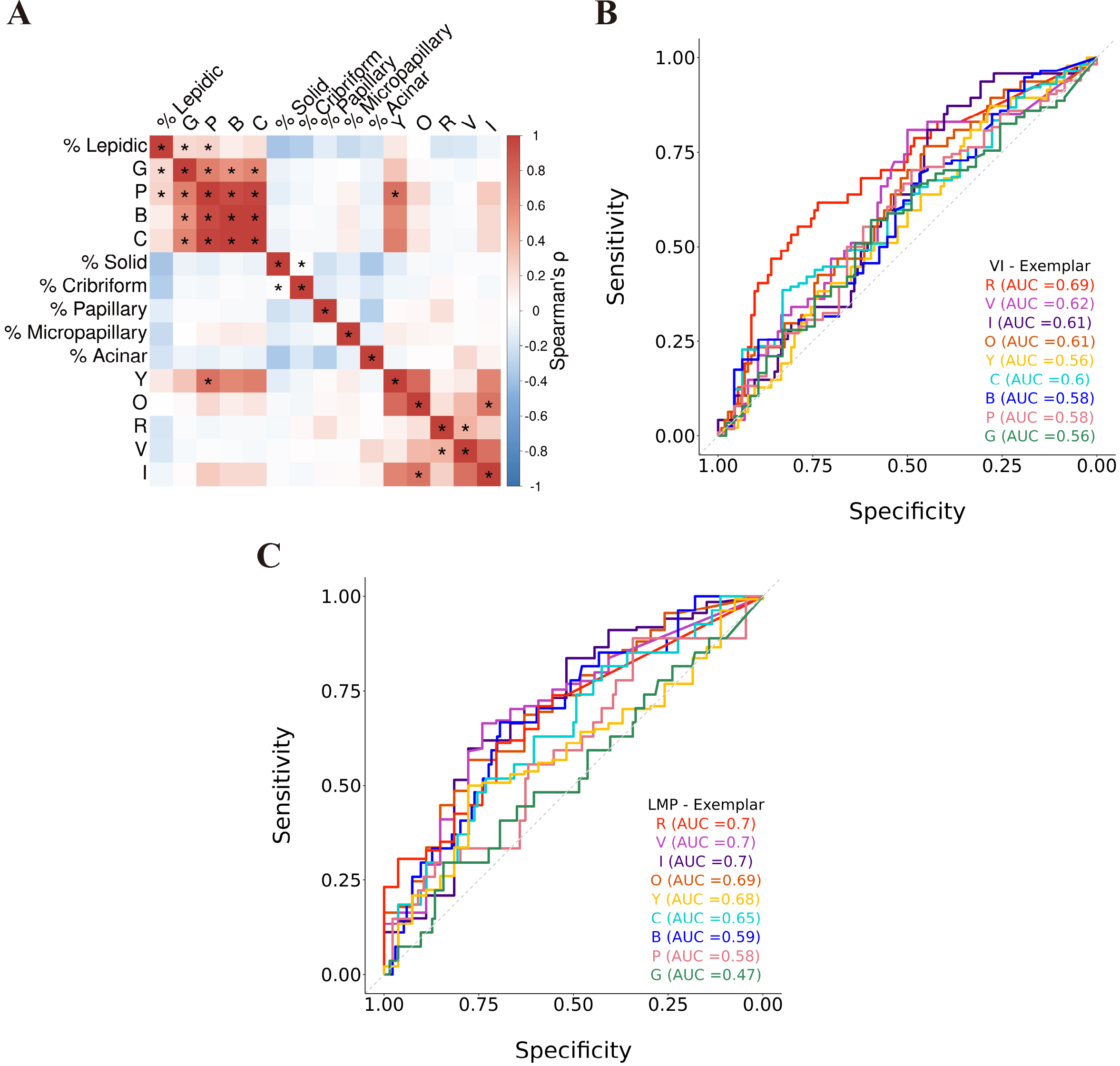
3.4The CANARY red exemplar is associated with VI at resection
Given that the SILA was weakly associated with WHO grade 3 tumors containing aggressive histologic patterns, we sought to determine whether any of the nine CANARY exemplars were associated with percentages of different growth patterns. Correlation analysis followed by unsupervised clustering revealed that non-lepidic patterns clustered separately from the exemplars (Fig. 4A), suggesting they are not major drivers of the SILA. The red exemplar had the highest performance for predicting VI (AUC of 0.69) (Fig. 4B). When all exemplars were included in a logistic regression model for predicting VI, only the red exemplar was significant (
4.Discussion
This study evaluated the association between CANARY, a well-described algorithm for preoperative prediction of indolent and aggressive LUAD [20, 22, 24, 25, 28], and histologic grade in an urban safety-net hospital for the first time. In this cohort, the low, medium, and high CANARY SILA prognostic groups were associated with 100%, 91%, and 73% 7-year RFS respectively, and the SILA was significantly associated with RFS even after correction for other clinical factors. The SILA prognostic subgroups were originally identified by association with linear extent of histologic invasion and showed prognostic stratification in both an internal and external cohort of predominantly (83%) clinical stage I LUAD; exhibiting 100%, 79%, and 58% 5-year DSS [24]. Our improved outcomes among intermediate and poor SILA risk groups likely reflect the restriction of our analysis to pathologic stage I LUAD. As the SILA has been previously validated in a cohort derived from a subset of the NLST containing 94% white patients, the validation of CANARY and the SILA for predicting prognosis in a cohort containing patients of diverse racial and ethnic identity (47% non-white) is encouraging given that both LUAD incidence and LUAD aggressiveness at diagnosis is higher for non-Hispanic black patients [18, 30, 31]. Additionally, our cohort captures the diverse etiology that is known about LUAD, with 14% of patients being never-smokers.
There remains no clinically accepted approach to preoperatively predict tumor aggressiveness among surgically operable LCs, which are managed uniformly by clinical stage, potentially resulting in over-treatment of indolent lesions. While the SILA has been shown to accurately predict AIS and MIA stage I LUAD, we have previously shown that tumors designated as LMP more closely match the proportion of overdiagnosed cases in the NLST [14] and Burks et al. in this edition. In our cohort, the SILA “Good” group (
Tumor grading by microvascular invasion, the histologic representation of tumor intravasation, has been shown to be more strongly associated with post-surgical outcome in stage I LUAD than grading that takes into consideration the proportion of the aggressive LUAD histologic patterns – solid and micropapillary [18]. Retrospective analysis shows that patients with VI who undergo sublobar resection have poorer outcomes [34]. This underscores the growing importance of identifying individuals with more aggressive disease prior to surgery. Using the SILA to predict VI preoperatively, potentially in combination with other biomarker modalities, may therefore offer opportunities to guide precision surgery, but prospective studies are needed. Tumors exhibiting VI may also identify candidates who would benefit from adjuvant or neoadjuvant therapy. In this study, the SILA predicted VI with an AUC of 0.71 and was associated with VI independently of invasive size at resection. A previous study of stage IA LUAD nodules found that the ratio of the length of nodule consolidation to nodule diameter in preoperative CT scans predicted combined lymphatic and/or blood vessel invasion [35]. While lymph vessel invasion is often reported interchangeably with angioinvasion, it may not be as strong of an independent prognostic factor [18, 19]. Examination of the CANARY exemplars showed that the red exemplar, which corresponds to the most visually solid tumor areas on CT, [20] was most responsible for the performance of the SILA for predicting VI. This is the first analysis showing CANARY to be predictive of a specific type of pathologic invasion; prior studies assessed CANARY and/or the SILA for predicting the size of any type of invasion. Others have identified the violet, indigo, red, and orange CANARY exemplars, visually corresponding to varying degrees of solid tumor CT appearance, as being associated with a lower likelihood of EGFR-mutated LUAD, which might be expected since these tumors frequently are rich in lepidic histology and ground glass CT-appearance [36]. Additionally, other studies have shown a lower prevalence of EGFR-mutated cases among both LUAD and NSCLC with VI [37, 38].
Future efforts to improve the preoperative prediction of VI from CT images may take advantage of convolutional neural network-based extraction of perinodular features to add additional context from the surrounding lung microvascular architecture [39]. A study seeking to preoperatively predict VI positive hepatocellular carcinoma from CT scans achieved an AUC of 0.89 in a validation set using a radiomic model incorporating peritumoral features [40]. In contrast to our findings with VI, the SILA and the CANARY exemplars were not associated with aggressive LUAD histologic patterns, which may explain the poor predictive performance we reported for WHO grade 3 tumors. Other studies have demonstrated the feasibility of building radiomic classifiers that may predict solid and micropapillary histology, suggesting that other nodule features than those extracted by the SILA may be more representative of these high-grade patterns [41, 42].
There were several limitations present in this study. Despite the robust validation of the SILA in a new cohort, the cohort was collected over 11 years and there may be variability due to changes in standard of care and practice patterns. Additionally, the low number of AIS/MIA cases included (
5.Conclusion
The SILA derived from preoperative CT scans was prognostic and predictive of resected pathologic grade in stage I LUAD patients from a diverse cohort of patients. New strategies are necessary to minimize overdiagnosis in this clinical setting and identify aggressive tumors that may benefit from precision surgery, adjuvant and/or neoadjuvant treatment. Ultimately, the SILA should be prospectively validated and benchmarked against pathology review of biopsies to identify both LMP and VI tumors preoperatively.
Author contributions
Conception: Dylan Steiner, Eric Burks, Marc Lenburg.
Interpretation or analysis of data: Dylan Steiner, Ju Ae Park, Sarah Singh, Austin Potter, Jonathan Scalera.
Preparation of the manuscript: Dylan Steiner, Eric Burks.
Revision for important intellectual content: Jennifer Beane, Kei Suzuki, Marc Lenburg.
Supervision: Jennifer Beane, Kei Suzuki, Eric Burks, Marc Lenburg.
Supplementary data
The supplementary files are available to download from http://dx.doi.org/10.3233/CBM-230456.
Acknowledgments
This work was supported by 1R01CA275015-01A1 and the Ellison Foundation.
References
[1] | R.L. Siegel, K.D. Miller, N.S. Wagle and A. Jemal, Cancer statistics, CA. Cancer J. Clin. 73: ((2023) ), 17–48. |
[2] | L.J. Esserman, I.M. Thompson, B. Reid, P. Nelson, D.F. Ransohoff, H.G. Welch, S. Hwang, D.A. Berry, K.W. Kinzler, W.C. Black, M. Bissell, H. Parnes and S. Srivastava, Addressing overdiagnosis and overtreatment in cancer: a prescription for change, Lancet Oncol. 15: ((2014) ), e234–e242. |
[3] | E.F. Patz, P. Pinsky, C. Gatsonis, J.D. Sicks, B.S. Kramer, M.C. Tammemägi, C. Chiles, W.C. Black, D.R. Aberle and NLST Overdiagnosis Manuscript Writing Team, Overdiagnosis in low-dose computed tomography screening for lung cancer, JAMA Intern. Med. 174: ((2014) ), 269–274. |
[4] | R. Sroufe and F.-M. (Spring) Kong, Triaging early-stage lung cancer patients into non-surgical pathways: who, when, and what? Transl. Lung Cancer Res. 4: ((2015) ), 438–447. |
[5] | H. Saji, M. Okada, M. Tsuboi, R. Nakajima, K. Suzuki, K. Aokage, T. Aoki, J. Okami, I. Yoshino, H. Ito, N. Okumura, M. Yamaguchi, N. Ikeda, M. Wakabayashi, K. Nakamura, H. Fukuda, S. Nakamura, T. Mitsudomi, S.-I. Watanabe and H. Asamura, Segmentectomy versus lobectomy in small-sized peripheral non-small-cell lung cancer (JCOG0802/WJOG4607L): a multicentre, open-label, phase 3, randomised, controlled, non-inferiority trial, The Lancet. 399: ((2022) ), 1607–1617. |
[6] | N. Altorki, X. Wang, D. Kozono, C. Watt, R. Landrenau, D. Wigle, J. Port, D.R. Jones, M. Conti, A.S. Ashrafi, M. Liberman, K. Yasufuku, S. Yang, J.D. Mitchell, H. Pass, R. Keenan, T. Bauer, D. Miller, L.J. Kohman, T.E. Stinchcombe and E. Vokes, Lobar or Sublobar Resection for Peripheral Stage IA Non–Small-Cell Lung Cancer, N. Engl. J. Med. 388: ((2023) ), 489–498. |
[7] | P.M. Forde, J.E. Chaft, K.N. Smith, V. Anagnostou, T.R. Cottrell, M.D. Hellmann, M. Zahurak, S.C. Yang, D.R. Jones, S. Broderick, R.J. Battafarano, M.J. Velez, N. Rekhtman, Z. Olah, J. Naidoo, K.A. Marrone, F. Verde, H. Guo, J. Zhang, J.X. Caushi, H.Y. Chan, J.-W. Sidhom, R.B. Scharpf, J. White, E. Gabrielson, H. Wang, G.L. Rosner, V. Rusch, J.D. Wolchok, T. Merghoub, J.M. Taube, V.E. Velculescu, S.L. Topalian, J.R. Brahmer and D.M. Pardoll, Neoadjuvant PD-1 Blockade in Resectable Lung Cancer, N. Engl. J. Med. 378: ((2018) ), 1976–1986. |
[8] | N.K. Altorki, T.E. McGraw, A.C. Borczuk, A. Saxena, J.L. Port, B.M. Stiles, B.E. Lee, N.J. Sanfilippo, R.J. Scheff, B.B. Pua, J.F. Gruden, P.J. Christos, C. Spinelli, J. Gakuria, M. Uppal, B. Binder, O. Elemento, K.V. Ballman and S.C. Formenti, Neoadjuvant durvalumab with or without stereotactic body radiotherapy in patients with early-stage non-small-cell lung cancer: a single-centre, randomised phase 2 trial, Lancet Oncol. 22: ((2021) ), 824–835. |
[9] | W.D. Travis, E. Brambilla, A.G. Nicholson, Y. Yatabe, J.H.M. Austin, M.B. Beasley, L.R. Chirieac, S. Dacic, E. Duhig, D.B. Flieder, K. Geisinger, F.R. Hirsch, Y. Ishikawa, K.M. Kerr, M. Noguchi, G. Pelosi, C.A. Powell, M.S. Tsao and I. Wistuba, The 2015 World Health Organization Classification of Lung Tumors: Impact of Genetic, Clinical and Radiologic Advances Since the 2004 Classification, J. Thorac. Oncol. 10: ((2015) ), 1243–1260. |
[10] | T.H. Kim, D. Buonocore, E.N. Petre, J.C. Durack, M. Maybody, R.P. Johnston, W.D. Travis, P.S. Adusumilli, S.B. Solomon and E. Ziv, Utility of Core Biopsy Specimen to Identify Histologic Subtype and Predict Outcome for Lung Adenocarcinoma, Ann. Thorac. Surg. 108: ((2019) ), 392–398. |
[11] | Reduced Lung-Cancer Mortality with Low-Dose Computed Tomographic Screening, N. Engl. J. Med. 365: ((2011) ), 395–409. |
[12] | National Lung Screening Trial Research Team, Lung Cancer Incidence and Mortality with Extended Follow-up in the National Lung Screening Trial, J. Thorac. Oncol. Off. Publ. Int. Assoc. Study Lung Cancer. 14: ((2019) ), 1732–1742. |
[13] | W.D. Travis, E. Brambilla, M. Noguchi, A.G. Nicholson, K.R. Geisinger, Y. Yatabe, D.G. Beer, C.A. Powell, G.J. Riely, P.E. Van Schil, K. Garg, J.H.M. Austin, H. Asamura, V.W. Rusch, F.R. Hirsch, G. Scagliotti, T. Mitsudomi, R.M. Huber, Y. Ishikawa, J. Jett, M. Sanchez-Cespedes, J.-P. Sculier, T. Takahashi, M. Tsuboi, J. Vansteenkiste, I. Wistuba, P.-C. Yang, D. Aberle, C. Brambilla, D. Flieder, W. Franklin, A. Gazdar, M. Gould, P. Hasleton, D. Henderson, B. Johnson, D. Johnson, K. Kerr, K. Kuriyama, J.S. Lee, V.A. Miller, I. Petersen, V. Roggli, R. Rosell, N. Saijo, E. Thunnissen, M. Tsao and D. Yankelewitz, International Association for the Study of Lung Cancer/American Thoracic Society/European Respiratory Society International Multidisciplinary Classification of Lung Adenocarcinoma, J. Thorac. Oncol. Off. Publ. Int. Assoc. Study Lung Cancer. 6: ((2011) ), 244–285. |
[14] | I. Yambayev, T.B. Sullivan, K. Suzuki, Q. Zhao, S.E. Higgins, O.H. Yilmaz, V.R. Litle, P. Moreira, E.L. Servais, C.T. Stock, S.M. Quadri, C. Williamson, K.M. Rieger-Christ and E.J. Burks, Pulmonary Adenocarcinomas of Low Malignant Potential, Am J Surg Pathol. 45: ((2020) ), 567–576. |
[15] | Y. Shimada, H. Saji, K. Yoshida, M. Kakihana, H. Honda, M. Nomura, J. Usuda, N. Kajiwara, T. Ohira and N. Ikeda, Pathological Vascular Invasion and Tumor Differentiation Predict Cancer Recurrence in Stage ia Non–Small-Cell Lung Cancer After Complete Surgical Resection, J. Thorac. Oncol. 7: ((2012) ), 1263–1270. |
[16] | A. Kagimoto, Y. Tsutani, T. Kambara, Y. Handa, T. Kumada, T. Mimae, K. Kushitani, Y. Miyata, Y. Takeshima and M. Okada, Utility of Newly Proposed Grading System From International Association for the Study of Lung Cancer for Invasive Lung Adenocarcinoma, JTO Clin. Res. Rep. 2: ((2021) ), 100126. |
[17] | G. Lee, S. Yoon, B. Ahn, H.-R. Kim, S.J. Jang and H.S. Hwang, Blood Vessel Invasion Predicts Postoperative Survival Outcomes and Systemic Recurrence Regardless of Location or Blood Vessel Type in Patients with Lung Adenocarcinoma, Ann. Surg. Oncol. 28: ((2021) ), 7279–7290. |
[18] | I. Yambayev, T.B. Sullivan, K.M. Rieger-Christ, E.L. Servais, C.T. Stock, S.M. Quadri, J.M. Sands, K. Suzuki and E.J. Burks, Vascular invasion identifies the most aggressive histologic subset of stage I lung adenocarcinoma: Implications for adjuvant therapy, Lung Cancer. 171: ((2022) ), 82–89. |
[19] | L. Suaiti, T.B. Sullivan, K.M. Rieger-Christ, E.L. Servais, K. Suzuki and E.J. Burks, Vascular Invasion Predicts Recurrence in Stage IA2-IB Lung Adenocarcinoma but not Squamous Cell Carcinoma, Clin. Lung Cancer. 24: ((2023) ), e126–e133. |
[20] | F. Maldonado, J.M. Boland, S. Raghunath, M.C. Aubry, B.J. Bartholmai, M. de Andrade, T.E. Hartman, R.A. Karwoski, S. Rajagopalan, A.-M. Sykes, P. Yang, E.S. Yi, R.A. Robb and T. Peikert, Noninvasive Characterization of the Histopathologic Features of Pulmonary Nodules of the Lung Adenocarcinoma Spectrum using Computer-Aided Nodule Assessment and Risk Yield (CANARY) – A Pilot Study, J. Thorac. Oncol. 8: ((2013) ), 452–460. |
[21] | U. Nemec, B.H. Heidinger, K.R. Anderson, M.S. Westmore, P.A. VanderLaan and A.A. Bankier, Software-based risk stratification of pulmonary adenocarcinomas manifesting as pure ground glass nodules on computed tomography, Eur. Radiol. 28: ((2018) ), 235–242. |
[22] | S. Raghunath, F. Maldonado, S. Rajagopalan, R.A. Karwoski, Z.S. DePew, B.J. Bartholmai, T. Peikert and R.A. Robb, Noninvasive Risk Stratification of Lung Adenocarcinoma using Quantitative Computed Tomography, J. Thorac. Oncol. 9: ((2014) ), 1698–1703. |
[23] | F. Maldonado, F. Duan, S.M. Raghunath, S. Rajagopalan, R.A. Karwoski, K. Garg, E. Greco, H. Nath, R.A. Robb, B.J. Bartholmai and T. Peikert, Noninvasive Computed Tomography – based Risk Stratification of Lung Adenocarcinomas in the National Lung Screening Trial, Am. J. Respir. Crit. Care Med. 192: ((2015) ), 737–744. |
[24] | C. Varghese, S. Rajagopalan, R.A. Karwoski, B.J. Bartholmai, F. Maldonado, J.M. Boland and T. Peikert, Computed Tomography – Based Score Indicative of Lung Cancer Aggression (SILA) Predicts the Degree of Histologic Tissue Invasion and Patient Survival in Lung Adenocarcinoma Spectrum, J. Thorac. Oncol. 14: ((2019) ), 1419–1429. |
[25] | J. Lee, B. Bartholmai, T. Peikert, J. Chun, H. Kim, J.S. Kim and S.Y. Park, Evaluation of Computer-Aided Nodule Assessment and Risk Yield (CANARY) in Korean patients for prediction of invasiveness of ground-glass opacity nodule, PLOS ONE. 16: ((2021) ), e0253204. |
[26] | WHO Classification of Tumours Editorial Board. Thoracic Tumours. Vol 5. 5th ed. Lyon (France): International Agency for Research on Cancer; (2021) . https://publications.iarc.fr/595. |
[27] | A.L. Moreira, P.S.S. Ocampo, Xia Y, et al. A Grading System for Invasive Pulmonary Adenocarcinoma: A Proposal From the International Association for the Study of Lung Cancer Pathology Committee. J Thorac Oncol Off Publ Int Assoc Study Lung Cancer. 15: ((2020) ), 1599–1610. doi: 10.1016/j.jtho.2020.06.001. |
[28] | E.C. Nakajima, M.P. Frankland, T.F. Johnson, S.L. Antic, H. Chen, S.-C. Chen, R.A. Karwoski, R. Walker, B.A. Landman, R.D. Clay, B.J. Bartholmai, S. Rajagopalan, T. Peikert, P.P. Massion and F. Maldonado, Assessing the inter-observer variability of Computer-Aided Nodule Assessment and Risk Yield (CANARY) to characterize lung adenocarcinomas, PLOS ONE. 13: ((2018) ), e0198118. |
[29] | X. Robin, N. Turck, A. Hainard, N. Tiberti, F. Lisacek, J.-C. Sanchez and M. Müller, pROC: an open-source package for R and S+ to analyze and compare ROC curves, BMC Bioinformatics. 12: ((2011) ), 77. |
[30] | R. Meza, C. Meernik, J. Jeon and M.L. Cote, Lung Cancer Incidence Trends by Gender, Race and Histology in the United States, 1973–2010, PLOS ONE. 10: ((2015) ), e0121323. |
[31] | K.A. Houston, K.A. Mitchell, J. King, A. White and B.M. Ryan, Histologic Lung Cancer Incidence Rates and Trends Vary by Race/Ethnicity and Residential County, J. Thorac. Oncol. 13: ((2018) ), 497–509. |
[32] | M.N. Kammer, D.A. Lakhani, A.B. Balar, S.L. Antic, A.K. Kussrow, R.L. Webster, S. Mahapatra, U. Barad, C. Shah, T. Atwater, B. Diergaarde, J. Qian, A. Kaizer, M. New, E. Hirsch, W.J. Feser, J. Strong, M. Rioth, Y. Miller, Y. Balagurunathan, D.J. Rowe, S. Helmey, S.-C. Chen, J. Bauza, S.A. Deppen, K. Sandler, F. Maldonado, A. Spira, E. Billatos, M.B. Schabath, R.J. Gillies, D.O. Wilson, R.C. Walker, B. Landman, H. Chen, E.L. Grogan, A.E. Barón, D.J. Bornhop and P.P. Massion, Integrated Biomarkers for the Management of Indeterminate Pulmonary Nodules, Am. J. Respir. Crit. Care Med. 204: ((2021) ), 1306–1316. |
[33] | M.-F. Senosain, Y. Zou, K. Patel, S. Zhao, A. Coullomb, D.J. Rowe, J.M. Lehman, J.M. Irish, F. Maldonado, M.N. Kammer, V. Pancaldi and C.F. Lopez, Integrated Multi-omics Analysis of Early Lung Adenocarcinoma Links Tumor Biological Features with Predicted Indolence or Aggressiveness, Cancer Res. Commun. 3: ((2023) ), 1350–1365. |
[34] | L. Ma, T.B. Sullivan, K.M. Rieger-Christ, I. Yambayev, Q. Zhao, S.E. Higgins, O.H. Yilmaz, L. Sultan, E.L. Servais, K. Suzuki and E.J. Burks, Vascular invasion predicts the subgroup of lung adenocarcinomas = 2.0 cm at risk of poor outcome treated by wedge resection compared to lobectomy, JTCVS Open. 16: ((2023) ), 938–947. |
[35] | T. Ito, T. Murakawa, H. Sato, A. Tanabe, M. Maekawa, Y. Yoshida, M. Fukayama and J. Nakajima, Simple preoperative computed tomography image analysis shows good predictive performance for pathological vessel invasion in clinical stage IA non-small cell lung cancer? Interact. Cardiovasc. Thorac. Surg. 15: ((2012) ), 633–638. |
[36] | R. Clay, B.R. Kipp, S. Jenkins, R.A. Karwoski, F. Maldonado, S. Rajagopalan, J.S. Voss, B.J. Bartholmai, M.C. Aubry and T. Peikert, Computer-Aided Nodule Assessment and Risk Yield (CANARY) may facilitate non-invasive prediction of EGFR mutation status in lung adenocarcinomas, Sci. Rep. 7: ((2017) ), 17620. |
[37] | A. Alwithenani, D. Bethune, M. Castonguay, A. Drucker, G. Flowerdew, M. Forsythe, D. French, J. Fris, W. Greer, H. Henteleff, M. MacNeil, P. Marignani, W. Morzycki, M. Plourde, S. Snow, P. Marcato and Z. Xu, Profiling non-small cell lung cancer reveals that PD-L1 is associated with wild type EGFR and vascular invasion, and immunohistochemistry quantification of PD-L1 correlates weakly with RT-qPCR, PLOS ONE. 16: ((2021) ), e0251080. |
[38] | M. Ito, Y. Miyata, K. Kushitani, D. Ueda, Y. Takeshima and M. Okada, Distribution and prognostic impact of EGFR and KRAS mutations according to histological subtype and tumor invasion status in pTis-3N0M0 lung adenocarcinoma, BMC Cancer. 23: ((2023) ), 248. |
[39] | R. Gao, T. Li, Y. Tang, K. Xu, M. Khan, M. Kammer, S.L. Antic, S. Deppen, Y. Huo, T.A. Lasko, K.L. Sandler, F. Maldonado and B.A. Landman, Reducing uncertainty in cancer risk estimation for patients with indeterminate pulmonary nodules using an integrated deep learning model, Comput. Biol. Med. 150: ((2022) ), 106113. |
[40] | X. Xu, H.-L. Zhang, Q.-P. Liu, S.-W. Sun, J. Zhang, F.-P. Zhu, G. Yang, X. Yan, Y.-D. Zhang and X.-S. Liu, Radiomic analysis of contrast-enhanced CT predicts microvascular invasion and outcome in hepatocellular carcinoma, J. Hepatol. 70: ((2019) ), 1133–1144. |
[41] | S.H. Song, H. Park, G. Lee, H.Y. Lee, I. Sohn, H.S. Kim, S.H. Lee, J.Y. Jeong, J. Kim, K.S. Lee and Y.M. Shim, Imaging Phenotyping Using Radiomics to Predict Micropapillary Pattern within Lung Adenocarcinoma, J. Thorac. Oncol. 12: ((2017) ), 624–632. |
[42] | B. He, Y. Song, L. Wang, T. Wang, Y. She, L. Hou, L. Zhang, C. Wu, B.A. Babu, U. Bagci, T. Waseem, M. Yang, D. Xie and C. Chen, A machine learning-based prediction of the micro- papillary/solid growth pattern in invasive lung adenocarcinoma with radiomics, Transl. Lung Cancer Res. 10: ((2021) ), 955–964. |