Identifying glycolysis-related LncRNAs for predicting prognosis in breast cancer patients
Abstract
PURPOSE:
Functions associated with glycolysis could serve as targets or biomarkers for therapy cancer. Our purpose was to establish a prognostic model that could evaluate the importance of Glycolysis-related lncRNAs in breast cancer.
METHODS:
Gene expressions were evaluated for breast cancer through The Cancer Genome Atlas (TCGA) database, and we calculated Pearson correlations to discover potential related lncRNAs. Differentially expressed genes were identified via criteria of FDR
RESULTS:
Eighty-nine differentially expressed lncRNAs were identified from 420 Glycolysis-related lncRNAs. 14 lncRNAs were correlated with prognosis in training set and were selected to establish the prognostic model. Low risk group had better prognosis in both training (
CONCLUSION:
Our study indicated that glycolysis-related lncRNAs had a significant role to facilitate the individualized survival prediction in breast cancer patients, which would be a potential therapeutic target.
1.Introduction
Glycolysis was firstly described as a phenomenon that cancer cells metabolize glucose in a manner by Warburg[1, 2]. Glucose metabolism alteration is an important hallmark of tumor cells, which has been applied to the cancer diagnosis and assessment of cancer response. Interestingly, glycolysis is also a potential therapeutic strategy for cancers[3, 4]. It is increasingly evident that glycolysis is able to facilitate tumor growth and promote chemotherapy resistance[5, 6, 7].
Breast cancer is a highly heterogenous disease that is the most commonly diagnosed malignancies in women. Clinical tumor staging, lymph node status, histological grade and molecular characteristics are considered as prognostic factors by current guideline of AJCC (American Joint Committee on Cancer, https://cancerstaging.org). Investigation of prognostic biomarkers may provide new insight to develop effective therapeutic strategy.
Long noncoding RNAs (lncRNAs) were defined as a type of RNA with more than 200 nucleotides in length and barely encode proteins. LncRNAs are capable of regulating expressions of mRNA at various levels and take part in many critical biological processes. They have a correlation with the diagnosis, treatment and prognosis of breast cancer[8, 9]. However, the mechanism by which lncRNAs regulate gene transcription remains largely unknown. The prognosis of breast cancer patients has a correlation with the status of immune infiltrates [10]. This study aims to identify glycolysis-related lncRNAs and explore the status of immune infiltrates in breast cancer patients.
2.Materials and methods
2.1Datasets and study cohort
Data of RNA expressions and clinical information of tumor tissues and normal breast tissues (1053 tumor tissues and 111 normal tissues) were obtained from the TCGA database (http://www.cancergenome.nih.gov/). Patients with incomplete survival data or being followed up for less than 30 days were excluded. The genes of Glycolysis were identified from GESA Gene Set (http://www.gsea-msigdb.org/gsea/msigdb/cards/ KEGG_GLYCOLYSIS_GLUCONEOGENESIS).
2.2Bioinformatic analysis and statistical analysis
Genome Reference Consortium (GRCh38) was applied to identified lncRNAs Pearson’s correlation was calculated to screen out glycolysis-related lncRNAs identified as square of correlation coefficient
Entire samples were randomly separated into training and validating sets. In training set, univariate Cox regression analysis were performed again to identify prognostic lncRNAs among training set. We identified 14 prognostic lncRNAs in training set and each gene was compressed into a single score. Sankey diagram and co-expression network were depicted via R v4.02 and Cytoscape software 3.8.0. The risk score was calculated using the following formula to construct the prognostic model.
Kaplan-Meier curves were drawn to compare the survival differences. Clinicopathological variables (e.g., age, stage and TNM staging) and risk scores were compared through Cox univariate and multivariate analyses. The ROC curves were drawn according to clinicopathological variables and risk score. To test the feasibility of our model, the value was validated in validating set.
Data of tumor-infiltrating immune cells were downloaded from the Tumor Immune Estimation Resource (TIMER) database. The infiltrating status of neutrophils dendritic, macrophage T cells and B cells were evaluated to identify their correlation with risk score.
3.Results
3.1Identification of DEGs and prognostic glycolysis-related lncRNAs
There were 14142 lncRNAs in the breast cancer dataset downloaded in the TCGA database, and among them, 420 were glycolysis-related ones identified according to selection criteria (correlation coefficient
Table 1
The characteristics of training and validation set
Covariates | Type | Total | Test | Train | |
---|---|---|---|---|---|
Age | 520(54.28%) | 256(53.56%) | 264(55%) | 0.7013 | |
Age | 438(45.72%) | 222(46.44%) | 216(45%) | ||
Stage | Stage I–II | 725(75.68%) | 367(76.78%) | 358(74.58%) | 0.4737 |
Stage | Stage III–IV | 233(24.32%) | 111(23.22%) | 122(25.42%) | |
T | T1-2 | 811(84.66%) | 404(84.52%) | 407(84.79%) | 0.9781 |
T | T3-4 | 147(15.34%) | 74(15.48%) | 73(15.21%) | |
M | M0 | 943(98.43%) | 470(98.33%) | 473(98.54%) | 0.9935 |
M | M1 | 15(1.57%) | 8(1.67%) | 7(1.46%) | |
N | N0 | 451(47.08%) | 235(49.16%) | 216(45%) | 0.2202 |
N | N1-3 | 507(52.92%) | 243(50.84%) | 264(55%) |
Figure 1.
A: Heatmap of DEGs Blue and red indicate lower expression and higher expression. B: Volcano map of expression of Glycolysis-related lncRNAs. C: The Sankey diagram listed the relationship of 14 lncRNAs and 18 mRNAs. D: The co-expression network listed the relationship of 14 lncRNAs and 18 mRNAs.
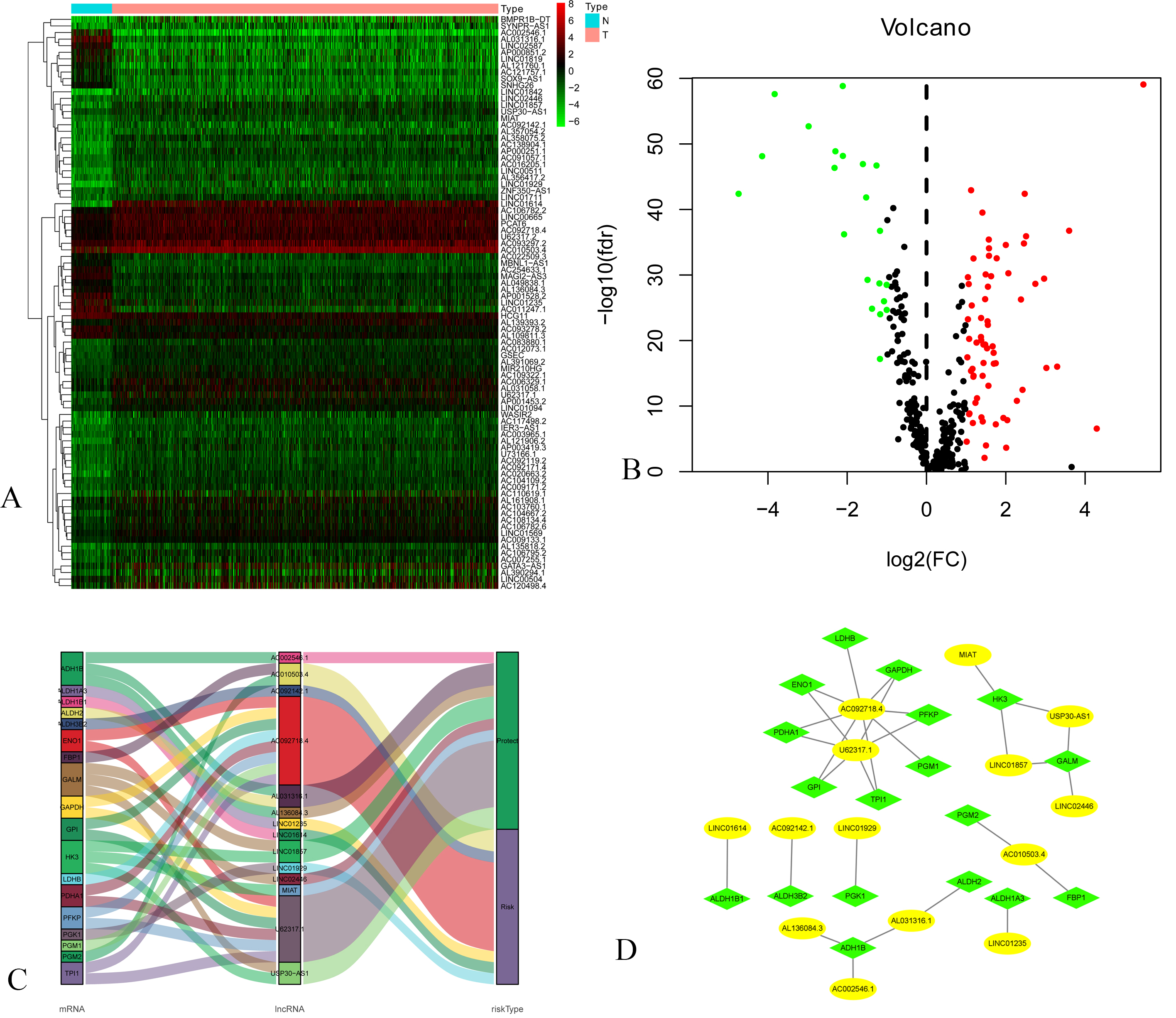
Table 2
Univariate Cox analysis of 14 prognostic glycolysis-related lncRNAs in training set
Id | HR | HR.95L | HR.95H | |
---|---|---|---|---|
LINC01614 | 1.3964652 | 1.1282007 | 1.7285178 | 0.0021532 |
U62317.1 | 0.7247198 | 0.5649086 | 0.9297412 | 0.0113057 |
AL031316.1 | 0.3779058 | 0.1869022 | 0.7641044 | 0.0067497 |
AC092142.1 | 1.7194702 | 1.1797157 | 2.5061782 | 0.0048056 |
AL136084.3 | 0.5839348 | 0.3437030 | 0.9920769 | 0.0466593 |
USP30-AS1 | 0.4388315 | 0.2645420 | 0.7279490 | 0.0014247 |
AC092718.4 | 1.3708613 | 1.0326176 | 1.8199001 | 0.0291104 |
LINC01235 | 1.3284102 | 1.0960503 | 1.6100298 | 0.0037931 |
AC010503.4 | 1.4792999 | 1.0081596 | 2.1706169 | 0.0453383 |
AC002546.1 | 0.2185639 | 0.0555115 | 0.8605451 | 0.0296486 |
LINC02446 | 0.5825388 | 0.3873295 | 0.8761311 | 0.0094582 |
MIAT | 0.4990005 | 0.2745908 | 0.9068095 | 0.0225518 |
LINC01929 | 1.7198242 | 1.2426473 | 2.3802372 | 0.0010748 |
LINC01857 | 0.5763394 | 0.3767619 | 0.8816366 | 0.0110597 |
Figure 2.
A: Kaplan-Meier Curve of low-risk group and high-risk groups according to the risk score in training set. B: Distribution of prognostic index in training set. C: Survival status of patients in training set D: Forest plot of Cox univariate analysis in training set E: Forest plot of Cox multivariate analysis in training set. F: Multi-parameter ROC curves for risk score, age, stage, lymph node statue metastasis statue and tumor size in training set.
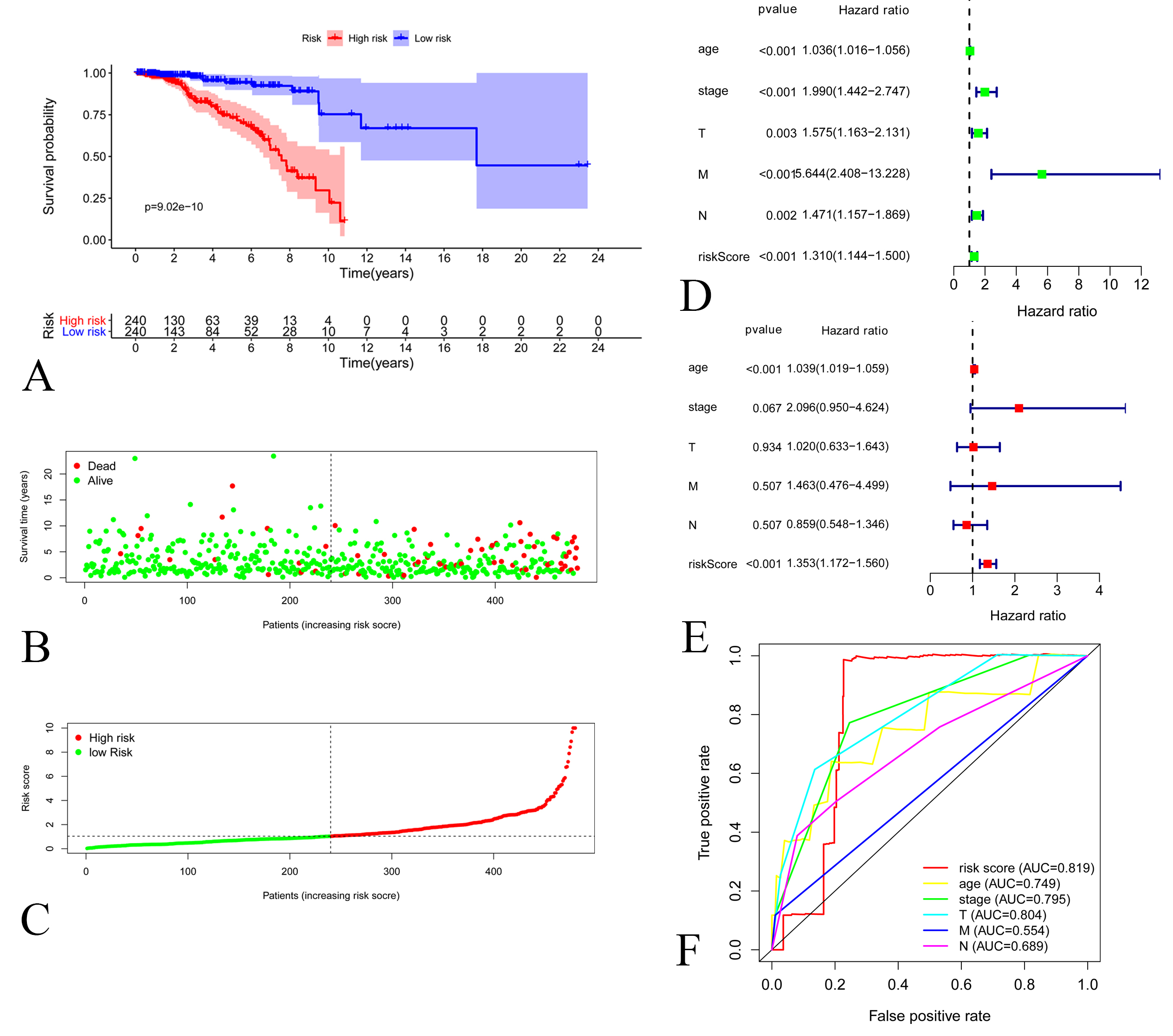
Figure 3.
A: Kaplan-Meier Curve of low-risk group and high-risk groups according to the risk score in validating set. B: Distribution of prognostic index in validating set. C: Survival status of patients in validating set D: Forest plot of Cox univariate analysis in validating set E: Forest plot of Cox multivariate analysis in validating set. F: Multi-parameter ROC curves for risk score, age, stage, lymph node statue metastasis statue and tumor size in validating set.
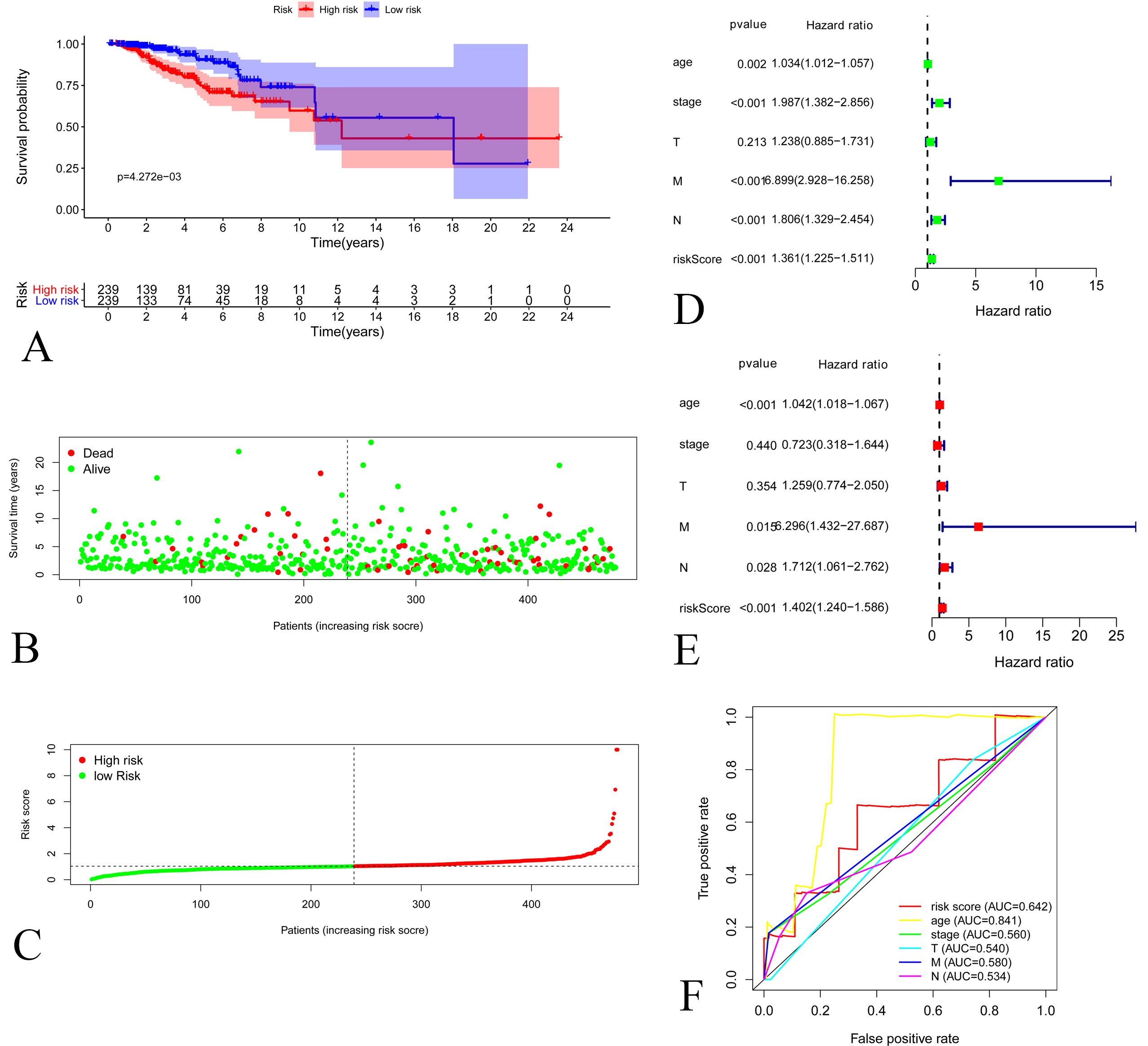
3.2Construction of the prognostic model
We randomly divided the entire samples into a training set (480 patient samples) and a validating set (478 patient samples) at a 1:1 ratio. The characteristics of two sets were presented in Table 1. There were no differences in clinicopathological variables between the two sets. We identified 14 prognostic lncRNAs via univariate Cox regression analysis in the training set (Table 2). Sankey diagram (Fig. 1C) and co-expression network (Fig. 1D) listed the relationship of 14 lncRNAs and 18 mRNAs. Among 14 prognostic lncRNAs, 8 lncRNAs have a positive (HRs
Figure 4.
The correlation between neutrophils, CD4+ T cells CD8+ T cells B cells macrophage, dendritic cells and risk score.
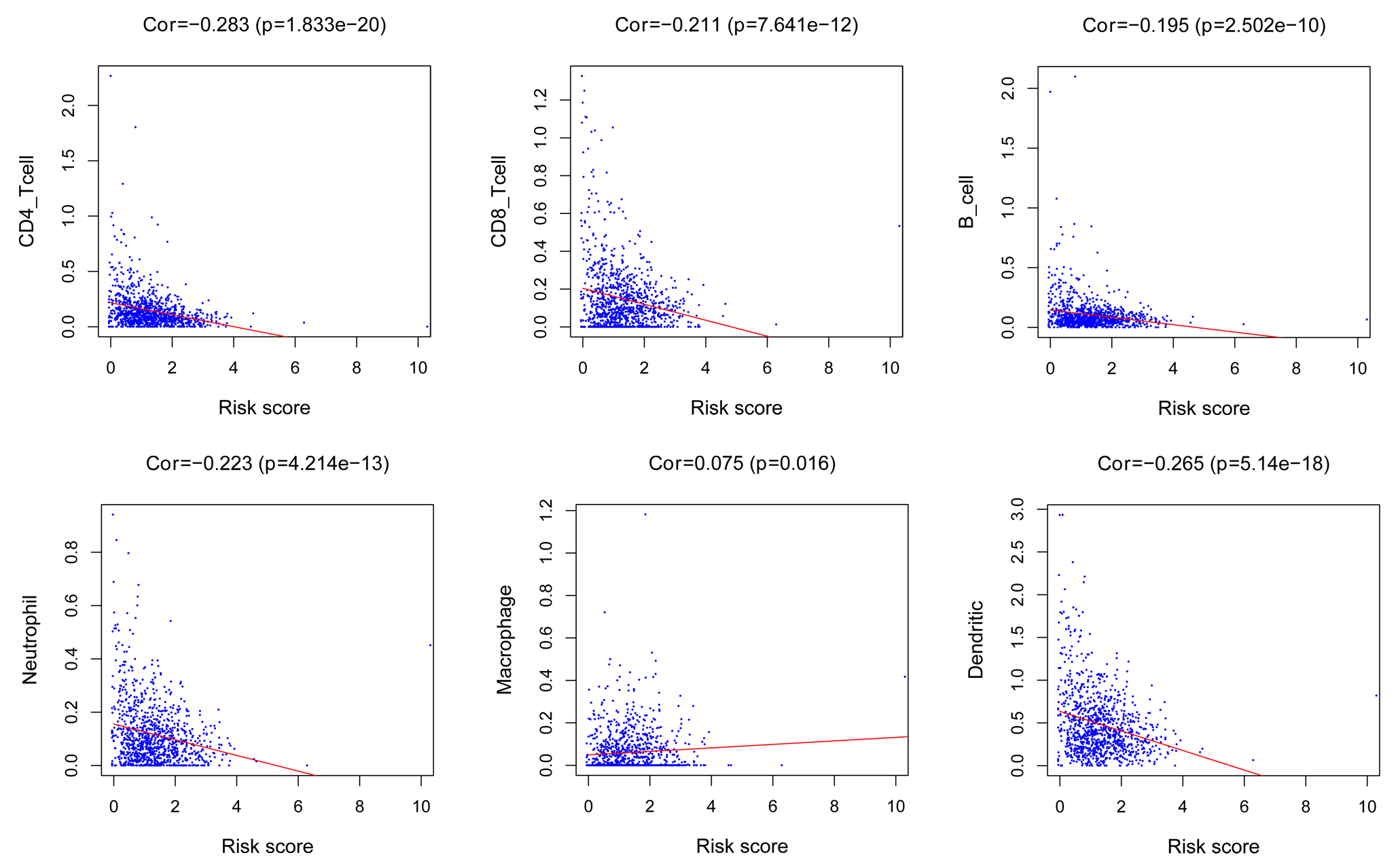
Each prognostic lncRNA obtained a risk score, and thus a prognostic model was constructed according to the formula. Finally, breast cancer patients were separated into high risk group and low risk group according to the median risk score for survival analysis. Kaplan-Meier curves indicated that low-risk patients were associated with better prognosis (
3.3The infiltrating status of immune cells
The neutrophils (
4.Discussion
Breast cancer is a heterogeneous disease that is commonly detected in clinical practice. Although multiple therapeutic strategies for breast cancer are optional, there were half a million deaths of breast cancer in 2012 [11]. In the future, cancer treatment based on tumor biology and early therapy response is the trend to enhance clinical outcomes of cancer patients [12]. Numerous studies have found that lncRNAs are critical regulators of tumorigenesis, growth, tumorigenesis and drug resistance of breast cancer[13, 14, 15, 16]. In our analysis, we investigated expression levels of 420 lncRNAs in breast cancer, narrowing down to 87 DEGs. We divided the entire samples into a training set (480 patient samples) and a validating set randomly. In training set, we identified 6 oncogenic lncRNAs and 8 tumor-suppressor lncRNAs. We constructed a prognostic model according to prognostic genes for breast cancer. We found that glycolysis-related lncRNAs were independent prognostic factors for breast cancer via Cox regression analyses. This result was validated in validating set Lactic acid is a key production induced by glycolysis. The accumulation of lactic acid may promote biological processes via tumor microenvironment [17]. On the other hand, the differentiation of CD4+ and CD8+ T cells relies on glycolysis[18]. The status of immune infiltrates was evaluated to identify their correlation with risk score of glycolysis-related LncRNAs. We observed that risk score was negatively correlated with neutrophils, CD4+ T cells, CD8+ T cells, B cells and dendritic cells. Previous studies found that CD8+ T cells was predictive factor for pathological complete remission to primary systemic therapy and was associated with better clinical outcomes[19, 20, 21]. Although CD4+ T cell may serve as an antitumor factor [22]. Recent study found that high CD4+ T cells had a non-favorable effect on breast cancer patient[21]. The main mechanism of anti-tumor immune response of CD4+ T cells is still being explored.
So far, among 14 prognostic lncRNAs, the function AC002546.1, AC010503.4, AC092142.1, AC092718.4, AL031316.1, AL136084.3 and U62317.1 have not been previously explored in breast cancer or other cancers. LINC01929 has been found positively correlated with tumor progression in liver cancer, oral squamous cell carcinoma and lung cancer[23, 24, 25]. Che et al. indicated that LINC01929 promotes tumor progression through miR-137-3p/FOXC1 axis in oral squamous cell carcinoma [23]. So far, LINC01929 has not been reported in breast cancer yet. We found that LINC01929 acted as a carcinogenic lncRNA in breast cancer, and its specific oncogenic mechanism remains unclear and is worth exploring. In the present study, LINC02446 was consistently identified as a prognostic gene and might serve as a promising therapeutic biomarker. LINC01614 was identified as a non-favorable prognostic biomarker in breast cancer[26, 27], which is consistent with our analysis. Previous studies have indicated that the oncogenic LINC01614 stimulated the development of osteosarcoma, gastric cancer cells, non-small cell lung cancer and lung adenocarcinoma, which provide a new insight into the development of cancer treatment[28, 29, 31]. MIAT was firstly discovered as a susceptible locus for myocardial infarction[32]. In recent years, a growing number of evidence has indicated the vital effect of MIAT in regulating the development of human cancers[33]. Previous study indicated that silence of MIAT inhibits proliferation of MCF-7 cells, and overexpression of it yields the opposite trend[34]. In addition, MIAT has a correlation with 5-fluorouracil (5-FU) resistance of MCF-7 cell. Yao et al. found that the expression level of GRP78, MIAT, OCT4 and AKT were dysregulated in 5-FU resistant MCF cells. These genes contributed to drug resistance [35]. However, we found that upregulation of MIAT was associated with a better overall survival. It is reported that the MIAT is lower-expressed in hormone receptor-negative tumor tissues than that in hormone receptor-positive ones. Hormone receptor-positive subtypes account for 60% of breast cancers and their prognosis is better than that of hormone receptor-negative subtypes[11, 36]. In addition, expression level of MIAT increases in early-stage breast cancer, rather than stage III and IV[34, 37]. These may attribute to the inconsistent findings.
Our findings suggested that glycolysis-related lncRNAs may serve as therapeutic targets against breast cancer progression. Several limitations existed in our analysis Our data was downloaded from the TCGA database, and most of breast cancer patients were primarily Americans. Breast cancer patients from other countries should be explored. On the other hand, all samples only record the breast cancer related events and we are limited in discussing the event of other organ sites. Experiments of in vitro and in vivo were lacked to validate our data mining results, which are needed in the future.
In summary, we have identified transcripts corresponding to 14 genes that may serve as prognostic markers in training set and constructed a prognostic model of breast cancer. This analysis provides a promising method in predicting individualized survival of breast cancer. In the prognostic model, risk score were correlated with infiltrating status of neutrophils dendritic, macrophage T cells and B cells. Our results might provide potential therapeutic targets for breast cancer.
Conflict of interest
None.
Author contributions
Data curation: Qi Zhu, Yanlin Gu.
Data analysis and statistical analysis: Yanlin Gu, Jiayue Zou and Xiaohua Li.
Figure constuction: Qi Zhu, Yanlin Gu.
Bioinformatic analysis: Jiayue Zou.
Manuscript draft: Jiayue Zou.
Review and Supervise: Lei Qin.
References
[1] | O. Warburg, On the origin of cancer cells, Science (New York, NY) 123: ((1956) ), 309–14. |
[2] | O. Warburg, On respiratory impairment in cancer cells, Science (New York, NY) 124: ((1956) ), 269–70. |
[3] | S. Ganapathy-Kanniappan and J.F. Geschwind, Tumor glycolysis as a target for cancer therapy: progress and prospects, Molecular Cancer 12: ((2013) ), 152. |
[4] | M. Ghanavat, M. Shahrouzian, Z. Deris Zayeri, S. Banihashemi, S.M. Kazemi and N. Saki, Digging deeper through glucose metabolism and its regulators in cancer and metastasis, Life Sciences 264: ((2021) ), 118603. |
[5] | S. Bonnet, S.L. Archer, J. Allalunis-Turner, A. Haromy, C. Beaulieu, R. Thompson, C.T. Lee, G.D. Lopaschuk, L. Puttagunta, S. Bonnet, G. Harry, K. Hashimoto, C.J. Porter, M.A. Andrade, B. Thebaud and E.D. Michelakis, A mitochondria-K+ channel axis is suppressed in cancer and its normalization promotes apoptosis and inhibits cancer growth, Cancer Cell 11: ((2007) ), 37–51. |
[6] | A.G. Al-Ziaydi and A.M. Al-Shammari, Newcastle disease virus suppress glycolysis pathway and induce breast cancer cells death, 31: ((2020) ), 341–348. |
[7] | C.W. Lu, S.C. Lin, K.F. Chen, Y.Y. Lai and S.J. Tsai, Induction of pyruvate dehydrogenase kinase-3 by hypoxia-inducible factor-1 promotes metabolic switch and drug resistance, The Journal of Biological Chemistry 283: ((2008) ), 28106–14. |
[8] | Z. Xing, P.K. Park, C. Lin and L. Yang, LncRNA BCAR4 wires up signaling transduction in breast cancer, RNA Biology 12: ((2015) ), 681–9. |
[9] | H. Ke, L. Zhao, X. Feng, H. Xu, L. Zou, Q. Yang, X. Su, L. Peng and B. Jiao, NEAT1 is Required for Survival of Breast Cancer Cells Through FUS and miR-548, Gene Regulation and Systems Biology 10: ((2016) ), 11–7. |
[10] | Y. Shen, X. Peng and C. Shen, Identification and validation of immune-related lncRNA prognostic signature for breast cancer, Genomics 112: ((2020) ), 2640–2646. |
[11] | N. Harbeck and M. Gnant, Breast cancer, Lancet (London, England) 389: ((2017) ), 1134–1150. |
[12] | Y.H. Lin, Crosstalk of lncRNA and cellular metabolism and their regulatory mechanism in cancer, International Journal of Molecular Sciences 21: ((2020) ). |
[13] | Z. Li, P. Hou, D. Fan, M. Dong, M. Ma, H. Li, R. Yao, Y. Li, G. Wang, P. Geng, A. Mihretab, D. Liu, Y. Zhang, B. Huang and J. Lu, The degradation of EZH2 mediated by lncRNA ANCR attenuated the invasion and metastasis of breast cancer, Cell Death and Differentiation 24: ((2017) ), 59–71. |
[14] | A. Lin, C. Li, Z. Xing, Q. Hu, K. Liang, L. Han, C. Wang and D.H. Hawke, The LINK-A lncRNA activates normoxic HIF1α signalling in triple-negative breast cancer, Nature Cell Biology 18: ((2016) ), 213–24. |
[15] | P. Koirala, J. Huang, T.T. Ho, F. Wu, X. Ding and Y.Y. Mo, LncRNA AK023948 is a positive regulator of AKT, Nature Communications 8: ((2017) ), 14422. |
[16] | Q. Shi, Y. Li, S. Li and L. Jin, LncRNA DILA1 inhibits Cyclin D1 degradation and contributes to tamoxifen resistance in breast cancer, Nature Communications 11: ((2020) ), 5513. |
[17] | J.X. Wang, S.Y.C. Choi, X. Niu, N. Kang, H. Xue, J. Killam and Y. Wang, Lactic acid and an acidic tumor microenvironment suppress anticancer immunity, 21: ((2020) ). |
[18] | Z. Hu, G. Qu, X. Yu, H. Jiang, X.L. Teng, L. Ding, Q. Hu, X. Guo, Y. Zhou, F. Wang, H.B. Li, L. Chen, J. Jiang, B. Su, J. Liu and Q. Zou, Acylglycerol kinase maintains metabolic state and immune responses of CD8(+) T Cells, Cell Metabolism 30: ((2019) ), 290–302e5.. |
[19] | H.R. Ali, E. Provenzano, S.J. Dawson, F.M. Blows, B. Liu, M. Shah, H.M. Earl, C.J. Poole, L. Hiller, J.A. Dunn, S.J. Bowden, C. Twelves, J.M. Bartlett, S.M. Mahmoud, E. Rakha, I.O. Ellis, S. Liu, D. Gao, T.O. Nielsen, P.D. Pharoah and C. Caldas, Association between CD8+ T-cell infiltration and breast cancer survival in 12,439 patients, Annals of Oncology: Official Journal of the European Society for Medical Oncology 25: ((2014) ), 1536–43. |
[20] | A.N. Seo, H.J. Lee, E.J. Kim, H.J. Kim, M.H. Jang, H.E. Lee, Y.J. Kim, J.H. Kim and S.Y. Park, Tumour-infiltrating CD8+ lymphocytes as an independent predictive factor for pathological complete response to primary systemic therapy in breast cancer, British Journal of Cancer 109: ((2013) ), 2705–13. |
[21] | Y. Huang, C. Ma, Q. Zhang, J. Ye, F. Wang, Y. Zhang, P. Hunborg, M.A. Varvares, D.F. Hoft, E.C. Hsueh and G. Peng, CD4+ and CD8+ T cells have opposing roles in breast cancer progression and outcome, Oncotarget 6: ((2015) ), 17462–78. |
[22] | M.P. Protti, L. De Monte and G. Di Lullo, Tumor antigen-specific CD4+ T cells in cancer immunity: from antigen identification to tumor prognosis and development of therapeutic strategies, Tissue Antigens 83: ((2014) ), 237–46. |
[23] | H. Che, Y. Che, Z. Zhang and Q. Lu, Long Non-Coding RNA LINC01929 accelerates progression of oral squamous cell carcinoma by targeting the miR-137-3p/FOXC1 Axis, Frontiers in Oncology 11: ((2021) ), 657876. |
[24] | A. Acha-Sagredo, B. Uko, P. Pantazi, N.G. Bediaga, C. Moschandrea, L. Rainbow, M.W. Marcus, M.P.A. Davies, J.K. Field and T. Liloglou, Long non-coding RNA dysregulation is a frequent event in non-small cell lung carcinoma pathogenesis, British Journal of Cancer 122: ((2020) ), 1050–1058. |
[25] | J. Ye, S. Wu, S. Pan, J. Huang and L. Ge, Risk scoring based on expression of long non-coding RNAs can effectively predict survival in hepatocellular carcinoma patients with or without fibrosis, Oncol Rep 43: ((2020) ), 1451–1466. |
[26] | Y. Wang, B. Song, L. Zhu and X. Zhang, Long non-coding RNA, LINC01614 as a potential biomarker for prognostic prediction in breast cancer, PeerJ 7: ((2019) ), e7976. |
[27] | R. Vishnubalaji, H. Shaath, E. Elkord and N.M. Alajez, Long non-coding RNA (lncRNA) transcriptional landscape in breast cancer identifies LINC01614 as non-favorable prognostic biomarker regulated by TGFβ and focal adhesion kinase (FAK) signaling, Cell Death Discovery 5: ((2019) ), 109. |
[28] | Y. Chen, W.Y. Cheng, H. Shi, S. Huang and H. Chen, Classifying gastric cancer using FLORA reveals clinically relevant molecular subtypes and highlights LINC01614 as a biomarker for patient prognosis, 40: ((2021) ), 2898–2909. |
[29] | Q. Cai, X. Zhao, Y. Wang, S. Li, J. Wang, Z. Xin and F. Li, LINC01614 promotes osteosarcoma progression via miR-520a-3p/SNX3 axis, Cellular Signalling 83: ((2021) ), 109985. |
[30] | Y. Sun and C. Ling, Analysis of the long non-coding RNA LINC01614 in non-small cell lung cancer, Medicine 98: ((2019) ), e16437. |
[31] | A.N. Liu, H.J. Qu, C.Y. Yu and P. Sun, Knockdown of LINC01614 inhibits lung adenocarcinoma cell progression by up-regulating miR-217 and down-regulating FOXP1, 22: ((2018) ), 4034–4044. |
[32] | N. Ishii, K. Ozaki, H. Sato, H. Mizuno, S. Susumu, A. Takahashi, Y. Miyamoto, S. Ikegawa, N. Kamatani, M. Hori, S. Satoshi, Y. Nakamura and T. Tanaka, Identification of a novel non-coding RNA, MIAT, that confers risk of myocardial infarction, Journal of Human Genetics 51: ((2006) ), 1087–1099. |
[33] | C.M. Da, C.Y. Gong, W. Nan, K.S. Zhou, Z.L. Wu and H.H. Zhang, The role of long non-coding RNA MIAT in cancers, Biomedicine & Pharmacotherapy = Biomedecine & Pharmacotherapie 129: ((2020) ), 110359. |
[34] | Y. Li, B. Jiang, X. Wu, Q. Huang, W. Chen, H. Zhu, X. Qu, L. Xie, X. Ma and G. Huang, Long non-coding RNA MIAT is estrogen-responsive and promotes estrogen-induced proliferation in ER-positive breast cancer cells, Biochemical and Biophysical Research Communications 503: ((2018) ), 45–50. |
[35] | X. Yao, Y. Tu, Y. Xu, Y. Guo, F. Yao and X. Zhang, Endoplasmic reticulum stress confers 5-fluorouracil resistance in breast cancer cell via the GRP78/OCT4/lncRNA MIAT/AKT pathway, American Journal of Cancer Research 10: ((2020) ), 838–855. |
[36] | I. Widodo, E.K. Dwianingsih, T. Utoro, S.L. Anwar, T. Aryandono and S. Soeripto, Prognostic value of lymphangiogenesis determinants in luminal and non-luminal breast carcinomas, Asian Pacific Journal of Cancer Prevention: APJCP 19: ((2018) ), 2461–2467. |
[37] | Z.A. Almnaseer and M. Mourtada-Maarabouni, Long noncoding RNA MIAT regulates apoptosis and the apoptotic response to chemotherapeutic agents in breast cancer cell lines, Bioscience Reports 38: ((2018) ). |