The impact of the lung EDRN-CVC on Phase 1, 2, & 3 biomarker validation studies
Abstract
The Early Detection Research Network’s (EDRN) purpose is to discover, develop and validate biomarkers and imaging methods to detect early-stage cancers or at-risk individuals. The EDRN is composed of sites that fall into four categories: Biomarker Developmental Laboratories (BDL), Biomarker Reference Laboratories (BRL), Clinical Validation Centers (CVC) and Data Management and Coordinating Centers. Each component has a crucial role to play within the mission of the EDRN. The primary role of the CVCs is to support biomarker developers through validation trials on promising biomarkers discovered by both EDRN and non-EDRN investigators. The second round of funding for the EDRN Lung CVC at Vanderbilt University Medical Center (VUMC) was funded in October 2016 and we intended to accomplish the three missions of the CVCs: To conduct innovative research on the validation of candidate biomarkers for early cancer detection and risk assessment of lung cancer in an observational study; to compare biomarker performance; and to serve as a resource center for collaborative research within the Network and partner with established EDRN BDLs and BRLs, new laboratories and industry partners. This report outlines the impact of the VUMC EDRN Lung CVC and describes the role in promoting and validating biological and imaging biomarkers.
1.Introduction
1.1The Mission of the EDRN and the role of the CVC
The Early Detection Research Network’s (EDRN) purpose is to discover, develop and validate biomarkers and imaging methods to detect early-stage cancers or at-risk individuals. Furthermore, the EDRN seeks to translate these biomarkers and imaging methods into diagnostic tests. A consortium of more than 300 investigators that include teams from academic institutions, private sector and the National Cancer Institute work collaboratively to bring biomarkers and imaging methods to clinical use.
The EDRN is composed of sites that fall into four categories: Biomarker Developmental Laboratories, Biomarker Reference Laboratories, Clinical Validation Centers (CVC) and Data Management and Coordinating Centers. Each component has a crucial role to play within the mission of the EDRN. The primary role of the CVCs is to support biomarker developers through validation trials on promising biomarkers discovered by both EDRN and non-EDRN investigators. CVCs also provide high-quality, well-annotated biospecimens to the biomarker developers for biomarker discovery, assay development and pre-validation studies. The EDRN supports CVCs to create biorepositories utilizing rigorous standard operating procedures for recruitment and specimen handling which, in turn, helps minimize false discoveries arising from poor specimen quality.
Within the EDRN, the Lung Group CVC has three main missions: (1) To conduct innovative research on the validation of candidate biomarkers for early cancer detection and risk assessment of lung cancer in an observational study; (2) To compare biomarker performance, whether biological or imaging based, for eventual clinical utility trial; and (3) To serve as a resource center for collaborative research within the Network by partnering with established EDRN biomarker developers, reference laboratories, new laboratories and industry partners. This report outlines the impact of the Vanderbilt University Medical Center (VUMC) EDRN Lung CVC and describes the role in promoting and validating biological and imaging biomarkers.
1.2Clinical foundation of the VUMC EDRN lung CVC
Hospital and clinical infrastructure: Central to a strong clinical validation center is a quaternary interdisciplinary hospital system where excellent care is offered across a variety of disciplines. To this end, VUMC provides an ideal foundation for the EDRN Lung CVC and study participant recruitment. The adult hospital contains the region’s only Level I Trauma Center along with one of the largest transplant programs by volume in the United States. The free-standing Monroe Carell Jr. Children’s Hospital at Vanderbilt is the most comprehensive children’s hospital in the state and provides the highest level of neonatal intensive care in the state. The Vanderbilt Clinic comprises more than 95 outpatient specialty practices in several campus locations which collectively see about 1,000,000 patients per year. The Vanderbilt-Ingram Cancer Center (VICC) cares for about 4,500 new cancer patients and conducts more than 65,000 outpatient visits annually. According to the most recent figures available from the Tennessee Department of Health, Vanderbilt is the largest uncompensated care provider in the Nashville area, providing a safety network for the underserved.
Medical center catchment area and lung cancer prevalence: The medical center’s primary catchment area of Middle Tennessee, Northern Mississippi and Alabama and South-Central Kentucky has some of the highest lung cancer mortality rates in the United States. Overall, Tennessee had the 5th highest lung cancer mortality (51.3 compared to the national average of 38.5 per 100,000) and incidence (74.5 compared to the national average of 58.3 per 100,000) based upon the latest data 2014–2018 [1]. Similarly, Tennessee has the 5
Pulmonary nodule clinic, interventional pulmonary and thoracic surgery support: The Interventional Pulmonology Program and Pulmonary Nodule Clinic at Vanderbilt offer the full spectrum of diagnostic, therapeutic and palliative airway procedures. Currently the Vanderbilt Pulmonary Nodule Clinic evaluates over 750 patients annually with suspicious nodules. In patients with lung cancer, this extends from early detection and endoscopic treatment to palliative airway procedures for rapid symptom relief in patients with advanced lung cancer. The Interventional Pulmonary Program at Vanderbilt is staffed by three pulmonologists and an advanced practice nurse and manages a plethora of complex airway problems. The Department of Thoracic Surgery is staffed by five surgeons and over 800 thoracic operations are performed and over 400 patients with known or suspected lung cancer are evaluated annually.
Lung cancer screening program: VUMC participated as one of approximately thirty sites enrolling patients as part of the National Lung Screening Trial [3]. After the trial’s publication, the Vanderbilt Lung Screening Program (VLSP) was established. The program is a certified lung screening center and recognized as a Screening Center of Excellence by the GO2 Foundation. As of July 2020, over 2,500 unique patients have been screened for lung cancer. There have been over 4,000 low-dose computed tomography (CT) scans performed and over 80 cancers diagnosed. Imaging for the lung screening program is performed at three outpatient facilities, which are co-operated by VUMC and Vanderbilt Imaging Services (VIS). Imaging is performed in accordance with all American College of Radiology (ACR) standards and results are reported to the ACR registry. Low-dose CT scans and follow up imaging are interpreted solely by cardiothoracic trained radiologists. Reporting is performed in accordance with the ACR Lung-RADS system. The program is centralized with shared decision making performed by two dedicated lung screening nurse practitioners, both of whom are certified tobacco treatment specialists. A program patient navigator assists with scheduling of baseline and follow up examinations and with necessary referrals including to the Vanderbilt Lung Institute.
1.3Research infrastructure for the VUMC EDRN lung CVC
In addition to the clinical care, a CVC relies upon intimate integration of the research environment with the clinical setting, VUMC is an academically oriented institution that strongly supports basic and clinical research. Much institutional effort has been spent on developing an environment which nurtures such activities and provides high quality general support services through institutional cores and shared resources. These designated cores and shared resources offer cutting edge scientific services, enabling access to high-end equipment, advanced techniques and specialized expertise for all Vanderbilt investigators. Of the 39 core facilities and 15 centers in the VUMC, the most relevant to the EDRN CVC are the Advanced Computing Center for Research and Education (ACCRE), Clinical Trials Shared Resource, Digital Histology Shared Resource, Translational Pathology Shared Resource and Clinical Research Center. The CVC is housed within the Vanderbilt-Ingram Cancer Center (VICC) and the cancer center directly supports the CVC through information technology and computing support, laboratory space and administrative effort.
Other shared resources specific to VICC and utilized by the CVC or EDRN members include: 1) The Clinical Trials Shared Resource (CTSR) assists cancer investigators in developing, initiating and completing scientifically meritorious clinical trials in an efficient, cost-effective and technically sound manner. The CTSR service support includes protocol development, regulatory compliance, study start-up, data management, research nursing, auditing, and patient accrual. 2) Translational Pathology Shared Resource (TPSR) provides access to human tissue samples, histology services and specialized equipment to researchers. Human tissues are collected prospectively from routine surgical resections and autopsies for use by investigators in basic, translational, and clinical research studies. These tissues are available fresh, snap frozen or in a variety of fixatives as requested by the investigator. Normal, neoplastic, and other diseased tissues are available for study. 3) Digital Histology Shared Resource provides large scale digital archiving and quantitative analysis of histological, immunocytochemical and immunofluorescence staining for tissue sections and tissue microarrays. 4) Institute for Medicine and Public Health at Vanderbilt University Medical Center encompasses four centers and one training program — the Center for Health Services Research, the Institute for Community Health, the Center for Improving Patient Safety, the VA Geriatric Research, Education and Clinical Center (GRECC) and the Quality Scholars Program. 5) Vanderbilt Institute for Clinical and Translational Research (VICTR) is an institutional program that fosters development of innovative clinical and translational research opportunities. One major impediment to T1 and T2 translation is the difficulty to expeditiously determine whether a scientific idea is of real merit. The generation of preliminary data and pilot initiatives for clinical and translational studies is fundamental to addressing such an impediment. VICTR provides financial and content expertise (biostatistics, bioinformatics, health services, scientific writing, etc.) of early research through pilot funds which are available to all levels of academic status, undergraduate students through established independent investigators. Supported, in part, by the funding from Vanderbilt’s Clinical Translational Science Award (CTSA), the VICTR pilot funding program funds clinical and translational pilot and feasibility studies for projects that involve human tissue, human information (e.g., medical records) and/or application to human health.
Table 1
Nashville lung cancer screening cohort opened in 2011
Specimen type | # Patients | Samples collected | Samples | ||
CVC I | CVC II | Total | distributed | ||
Bronchial biopsy | 206 | 632 | 164 | 796 | 62 |
Bronchial brush | 11 | 7 | 4 | 11 | 9 |
Bronchial Brush DNA | 205 | 193 | 56 | 249 | 21 |
Bronchial Brush Protein | 197 | 187 | 59 | 246 | 33 |
Bronchial Brush RNA | 204 | 193 | 50 | 243 | 32 |
Bronchial Lavage Pellet | 34 | – | 53 | 53 | – |
Bronchial Lavage-Bal | 179 | 453 | 187 | 640 | 483 |
Buccal | 436 | 274 | 759 | 1,033 | – |
Nasal Brush | 428 | 274 | 727 | 1,001 | 28 |
Plasma | 444 | 3,570 | 11,640 | 15,210 | 229 |
Serum | 444 | 2,972 | 6,430 | 9,402 | 332 |
Sputum | 434 | 200 | 748 | 948 | 4 |
Streck Plasma | 406 | – | 7,094 | 7,094 | 752 |
Urine | 438 | 1,422 | 3,616 | 5,038 | – |
Total | 451 | 10,377 | 31,587 | 41,964 | 1985 |
1.4REDCap (research electronic data capture)
Translational research at this scale srequires a robust, easily modifiable, rapidly developed data input, storage, and retrieval system. The primary data repository and retrieval system that facilitates all translation research at VUMC is built upon REDCap. This system, developed and supported by Vanderbilt’s CTSA, provides HIPAA-compliant secure data management with very short development times. The REDCap consortium is an international group promoting the use and development of this open-source research tool. REDCap is in use by more than 1,500 institutions nationally and internationally, and recently received a $1.3M CTSA supplement grant for system enhancements. It is an example of the leveraging of CTSA-supported resources for cancer research. REDCap is the multi-center epidemiological data capture infrastructure used in the Cancer versus Fungus (CanVFun) consortium, the CVC’s clinical data capture and for some supplemental projects that require specific patient or tissue data. The EDRN’s clinical data survey is in REDCap and metadata for local database creation and data collection available to all EDRN members and the public. REDCap was recently implemented as a Veteran’s Affairs-wide data capture solution and is available with user support through the VA Information Resource Center (VIReC).
1.5Development of the VUMC EDRN CVC
The Vanderbilt-Ingram Cancer Center has a well-established Thoracic specimen biorepository dedicated to the support of lung cancer research. This biorepository was started by Dr. David Carbone in 2001 with the acquisition of a Lung SPORE grant that lasted until 2012. The biorepository follows the National Cancer Institute Best Practices for Biospecimen Resources (revised in 2011). Any specimens distributed are de-identified per HIPAA standards, as required by the Institutional Review Board (IRB). All specimens are collected and processed following a detailed standard operating procedure including low dose chest CT imaging data acquisition. Since 2009, protocols followed the EDRN tissue collection specifications as defined on the EDRN’s web portal (https://edrn.nci.nih.gov/archive/standard-operating-procedures/standard-operating-procedures). The development of the VUMC EDRN CVC can be understood as three main stages.
1.5.1Stage 1 – Infrastructure development
Biospecimen quality: Superlative biospecimen quality control is essential to the success of any research program. To this end, standard operating procedures (SOPs) for all tissue collection need to be developed and tested, and following implementation, adherence to these protocols must be regularly validated. Stage I of the EDRN CVC development process (2009 to 2015 at VUMC) was focused on defining the goals and procedures, implementing the workflows and ensuring compliance. Additionally, Stage I focused on integrating sample collection with a custom-built REDCap database that linked samples and de-identified clinical information and streamlining processes for requesting access to samples from outside institutions and internal collaborators while tracking sample disbursement.
To facilitate sample acquisition, Stage I focused on technical infrastructure development and procuring the requisite hardware to enable efficient and reproducible processing of all samples. This includes storage space, freezers, centrifuges, mass balances and barcoding systems. During this development stage, unique patient accrual averaged approximately 330 per year (Fig. 1). This enrollment volume does not represent the total number of longitudinal study encounters or specimen collections, as most patients were seen multiple times over the course of their evaluation and disease treatment, with multiple biospecimen and imaging collections (Table 1). Many patients who maintain VUMC as their primary clinic undergo continuing research and biorepository participation.
Figure 1.
(A) Patients consented for research over time in the VUMC Thoracic Biorepository. (B) Samples collected through the Nashville Lung Cancer Screening program.
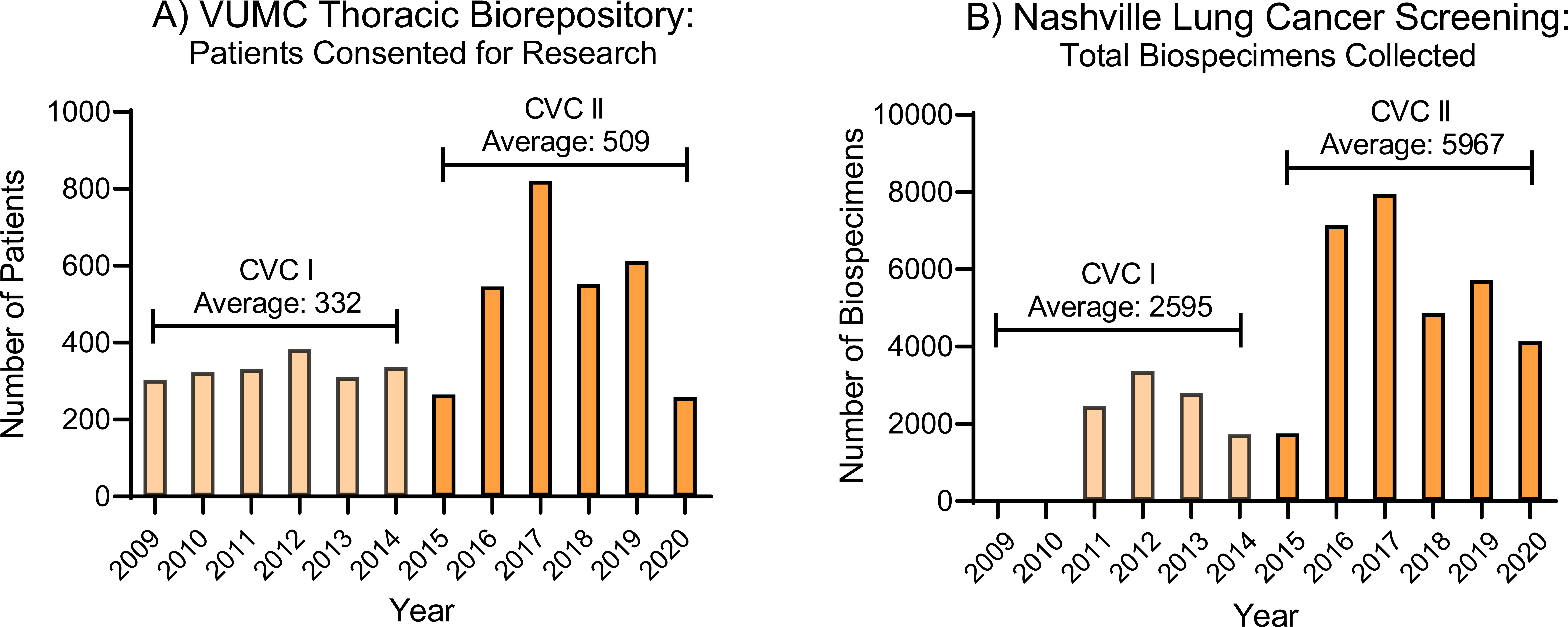
Following several iterations of sample collection and testing, several core sample processing SOPs were developed. Key SOPs are included in the supplementary material. All tissue acquisition is performed or supervised by a trained pathologist or certified pathologist assistant. Tumors with
1.5.2Stage 2 – Implementation
Stage 2 focused on scaling the patient accrual and sample collection efforts. During this stage from 2015 to 2020, patient accrual jumped to an average of approximately 500 per year. However, 2015 was a transition year, and the EDRN development Stage 2 did not begin until the end of the year, and 2020 saw decreased accrual due to a slowdown in clinical and research activities due to the COVID 19 pandemic. Therefore, when considering the time from 2016 to 2019, the average patient accrual was approximately 630 per year, nearly double the annual accrual from Stage 1 (Fig. 1).
1.5.3Stage 3 – Diversity and expansion
Our Stage 3 CVC development plan is focused on two main goals: 1) Increasing diversity through partnerships with clinics that primarily serve minority populations and clinics that serve large numbers of underinsured populations and 2) Expanding the reach of our accrual network through partnerships with other medical centers. Progress towards the first goal is presented in Section 2.3 which presents preliminary progress on a partnership with Meharry Medical College, a historically black college (HBC) in Nashville that serves a primarily minority population. Progress towards the second goal is presented in Section 2.4. Both projects leverage the EDRN-built CVC infrastructure at Vanderbilt to partner with regional clinics to aid the EDRN sample collection through increased overall sample collection and improved diversity of sample collection, but also benefit the regional centers as they empowers them to take advantage of the VUMC infrastructure to strengthen their own clinical research programs.
2.Patient enrollment and specimen acquisition for the lung CVC biospecimen repository
2.1VUMC institutionally supported biospecimen acquisition
VUMC has developed its own internal processes for biospecimen collections across a wide range of tissues that supplement these phase I/II/III studies across multiple lung cancer histological subtypes, which have benefitted the EDRN. Core to these studies are the protocols for obtaining biospecimens of the following types: fresh tissue, frozen tissues, paraffin block tissue, research pathology, linked tumor/normal tissue micro array, bronchioalveolar lavage, nasal rinse, sputum, serum, plasma, whole blood, buffy coat and urine. While the end goal of the EDRN is focused on easily accessible samples for non-invasive early detection, such as blood or urine, the availability of these other biospecimen types allows more complex studies such as the relation between the biology internal to the tumor and the circulating biomarkers. Matched tissue/tumor/blood sample sets are vital to this effort.
Table 2
Other sample accrual
# Patients | # Aliquots | # Aliquots distributed | |
CanVFun Consortium opened in 2018 | |||
Serum | 104 | 286 | 82 |
Plasma | 55 | 677 | 0 |
Buffy coat/EDTA | 34 | 34 | 0 |
Total Unique | 106 | 997 | 82 |
EDRN Collaborative Group Project 2 (LTP2) accrual 2013–2020 | |||
Serum | 118 | 2103 | |
Plasma | 118 | 2164 | |
Pax Gene | 118 | 236 | |
Nasal Brushing | 118 | 118 | |
Airway Brushings | 118 | 472 | |
Total | 118 | 3226 |
2.2EDRN nashville lung cancer screening cohort
The primary patient accrual instrument for the EDRN is an ongoing prospective longitudinal cohort of individuals at high-risk of developing lung cancer that was established to build a biorepository of carefully annotated biological specimens and low dose computed tomography (LDCT) chest images for derivation and validation of candidate biomarkers for early detection of lung cancer. The goal of this study is to characterize individuals with high risk for lung cancer, accumulating valuable biospecimens and LDCT chest scan longitudinally over five years. Participants 55–80 years of age and with a 6-year estimated risk of developing lung cancer greater than 1.5%, according to the PLCO model, were recruited and enrolled from clinics at Vanderbilt University Medical Center, the Veteran Affairs Medical Center, and Meharry Medical Center. Individual demographic characteristics were assessed via questionnaire at baseline. Participants underwent a LDCT scan, spirometry, sputum cytology and research bronchoscopy at the time of enrollment. Participants will be followed yearly for five years. Positive LDCT scans are followed up according to standard of care. The clinical, imaging and biospecimen data are collected prospectively and stored in the biorepository. Participants are offered smoking cessation counseling at each study visit. A total of 480 participants were enrolled at study baseline and consented to sharing of their data and biospecimens for research. Participants are followed with yearly clinic visits to collect imaging data and biospecimens. To date, a total of 19 lung cancers (13 adenocarcinomas, 4 squamous cell carcinoma, 1 large cell neuroendocrine and 1 small cell lung cancer) have been identified. We established a unique prospective cohort of individuals at high-risk for lung cancer, enrolled at three institutions for which full clinical data, well-annotated LDCT and biospecimens are being collected longitudinally. This repository will allow for the derivation of independent validation of clinical, imaging and molecular biomarkers of risk or diagnosis of lung cancer [4] (Table 1).
2.3Lung cancer screening in underrepresented populations
Developing a study population from an academic center runs the risk of under-representing certain populations, including those that may be at highest risk of disease and would most benefit from early detection. For this reason, VUMC has leveraged its CVC infrastructure to partner with Meharry Medical College through the Meharry-Vanderbilt Alliance and its affiliation with Nashville General Hospital, the city’s safety net hospital, to develop a cohort focused on underserved and underinsured persons. Study enrollment began at Meharry Medical College in September 2019. We have recruited and completed a baseline study visit for a total of 104 participants for our underserved (under-insured) protocol. Our population recruited from Meharry Medical College (
2.4Geographically diverse specimen collection (CanVFun consortium)
Another crucial role in the purview of the CVC is to identify collaborative centers that would improve population and sample diversity while benefitting from the infrastructure already in place at the CVC. In this approach, the CVC helps to collect biospecimens and clinical data for other institutions while organizing these extramural biospecimens into a cohesive, representative cohort. In this instance, VUMC serves as the hub for a firmly established 4 site consortium, the Cancer Versus Fungus (CanVFun) Consortium, with Washington University in St. Louis, the University of Kentucky, the University of Cincinnati and VUMC. This consortium is working to develop a diverse patient cohort representative of the indeterminate pulmonary nodule population within the central US to develop and validate both malignant and benign lung disease specific imaging and liquid biomarkers. Without the existing CVC infrastructure at VUMC for biospecimen collection and storage, image deidentification and storage and clinical data capture through REDCap using EDRN defined data dictionaries, it would be much more difficult for these external sites to develop study populations of their own. To date, CanVFun has prospectively consented 65 participants and collected biospecimens and clinical data based upon the EDRN’s standardized initial patient survey data collection form that was transcribed into a secure multi-site REDCap database. A separate set of 51 retrospectively acquired patients were contributed from Cincinnati with complete imaging and clinical information history. The goal for enrollment is 120 patients by July 2022. Specimen totals are included in Table 2.
2.5Non-EDRN collections facilitated by EDRN-developed infrastructure
Outside of the context of the EDRN specifically, several ongoing patient accrual studies at VUMC provide supplementary biospecimens that have been leveraged for both internal EDRN collaborative studies and external studies within the field of lung cancer. The EDRN-funded infrastructure has augmented and bolstered these accrual abilities and these specimens are available to EDRN investigators. For example, we have enrolled 98 patients into DECAMP studies [5].
2.5.1Lung Team Project 2 (LTP2)
Dr. Massion was the PI of the Lung Team Project 2 and was assisted by Drs Grogan and Deppen. The LTP2 study has brought collaborators (including new site Dr. Willey from U Toledo) to meet their accrual goals. The enrollment is closely monitored by the DMCC with the support of Tracey Marsh. COVID-19 limited 2020 enrollment but 15 additional samples from the CanVFun EDRN recruitment (see below) and 15 from Dr. Harvey Pass at NYU (EDRN collaborator) have completed the 300-patient reference set. The VUMC closed its accrual for being the lead accrual in LTP2 with over 1/3 of all patients accrued. All samples are being adjudicated and images transferred into XNAT for future image analysis and samples sent to the reference labs. These will be included in the EDRN reference set and sent to the NCI Frederick Biorepository Facility. Sample collections are included in Table 2.
3.Biomarker validation
As the science behind biomarker discovery and detection has evolved, so too have the focuses of the development and validation studies. As technology progresses, new avenues of research open, which also make previously traveled paths of research less optimal. For these reasons, a summary of studies will be presented approximately chronologically relative to their inception, rather than by any measure of relevance or completeness. The purpose of this section is not to compare the various biomarker techniques, which have been more thoroughly discussed elsewhere [6, 7, 8, 9], but to provide a summary of the breadth and depth of studies which the VUMC CVC has facilitated. The three phases of biomarker development are as follows:
Phase I – Preclinical/Exploratory Biomarker Discovery: This is the biomarker discovery phase. The role of the CVC in Phase I studies is primarily to provide biomarker development laboratories with high quality sample sets from the relevant patient populations. These samples can be either of the tissue of interest for final tests (such as serum or plasma) or bulk tumor/lung tissue which can be used for proteomic/genetic analysis. This phase also focuses on developing new technologies for either new or existing biomarkers. Samples from VUMC have been used in biomarker discovery for a range of biomarker technologies including mass spectroscopy-based methods [10, 11, 12, 13], enzyme-linke
Table 3
Biomarker studies facilitated by the EDRN lung CVC
Biomarker | Assay platform | Phase I | Phase II | Phase III | Status | Developed by |
---|---|---|---|---|---|---|
MALDI signature | MALDI MS | (12) | (10) | PP | Vanderbilt | |
MALDI signature | MALDI MS | (53) | Pittsburgh | |||
C4d fragment | ELISA | (16) | (15, 24) | U Navarra | ||
MRM signature of risk | PRM | PP (54) | Vanderbilt | |||
MRM signature of malignancy | MRM | (13) | (25, 55) | CA, ICT (Q4 2021) | Biodesix | |
Tumor Autoantibody Panel (6) | ELISA | (14) | CA, ICT (Q4 2021) | Oncimmune | ||
FXR1 | IHC | (26) | (26) | Vanderbilt | ||
SLC1A5 | IHC | (56) | Vanderbilt | |||
Free Solution Assay of CYFRA 21.1 | CIR | (20) | (57, 58) | (27, 28) | ICT (Q4 2021) | Vanderbilt |
Histoplasmosis IgG/IgM | ELISA | (29, 59) | ICT (Q4 2021) | Vanderbilt | ||
PRM signature | PRM | PP | Vanderbilt | |||
SIgA | ELISA | (60) | Vanderbilt | |||
MDM2 | FISH | (61) | PP | Vanderbilt | ||
MagArray Platform | MagArray | (18) | (18, 19) | (18, 19) | CA | MagArray |
SRM Mass Spec | Mass Spec | PP | Pacific Northwest National Labs & Vanderbilt | |||
Nucleic Acid Markers | ||||||
TP63, MYC, CEP3, CEP6 | FISH | (62) | Vanderbilt | |||
CAPP Seq | NGS | (22) | Stanford | |||
miRNA signature | RNA seq | (62) | (63) | Boston University | ||
miRNA signature | PCR | PP | Pending | Ohio State University | ||
RNA expression signature | PCR | (64) | University of Toledo | |||
miRNA | qRT-PCR | (65) | (17) | SUST, China | ||
DNA Methylation | (66) | PP | Vanderbilt | |||
Imaging | ||||||
Optellum Risk Predictor | Radiomics | (67) | (68) | CA | Optellum | |
Quantitative Radiomics | Radiomics | (52) | (27, 28) | (27, 28) | CA | HealthMyne |
CANARY | Radiomics | (69) | (43) | (69) | CA | CANARY |
VCAR | CT | (23) | Vanderbilt | |||
68Ga-DOTATATE PET/CT | PET Tracer | (70, 71) | Vanderbilt | |||
4-[18F]Fluoroglutamine | PET Tracer | (72) | Pending | Vanderbilt | ||
Models | ||||||
TREAT | (73) | Used clinically | Vanderbilt | |||
BRODERs | (74) | (74) | (74) | Used clinically | Mayo Clinic | |
Deep Path | (30) | (30) | Vanderbilt | |||
LSTM | (32) | Vanderbilt | ||||
Multi-Path | (31, 34) | Vanderbilt |
If a study has been published as part of a Phase I/II/III trial, the reference to the publication is listed. NP: Not published. PP: Publication Pending. CA: Commercially Available. ICT (XX): Interventional Clinical Trial, parenthesis indicates start date.
In addition to development of biomarkers themselves, the close interaction between the CVC and basic sciences has enabled investigation of new model building techniques to improve how biomarkers are incorporated into risk predictors and implemented into practice. For example, a new method to train predictive models on cohorts of patients using multiple biomarkers, when not all patients have all biomarkers measured, enables training models on larger populations resulting in more generalizable and robust models [30]. Additionally, novel techniques for incorporating longitudinal measurements at irregular intervals and using heterogeneous images allows for more robust treatment of messy, real world imaging data [31, 32, 33, 34].
Lastly, the CVC has provided the foundation required to train and validate new clinical risk models, including the TREAT model designed for use in surgical clinic cohorts [35, 36], validation of the BRODERs classifier [37] and validation of the currently used clinical risk models [35].
4.Imaging repository
Our validation center has been leading an effort in combining imaging and biomarker studies. We have now established a unique infrastructure to deposit large number of Chest CT series in an imaging repository. The EDRN supported Drs. Carr and Landman, who were instrumental in establishing a robust and reproducible infrastructure. The group uses the eXtensible Neuroimaging Toolkit (XNAT) to provide secure, multi-site access to data resources [38]. The EDRN has supported the infrastructure for additional programming and machine learning investigations and benefitted other areas of research. All projects make extensive use of PyTorch, Tensorflow and git and are tuned for scalable integration with XNAT [39, 40] and DAX, an open source extension of XNAT [41]. Our work [42, 43, 44, 45, 46] on machine learning with lung screening and more generally in body radiology and neuroimaging, with over 17 publications in non-lung cancer topics, relies heavily on this infrastructure. While this infrastructure forms the nucleus of our machine learning efforts, it supports external academic [37, 43] and industry [47] (resubmitted paper) imaging based machine learning model development and validation for the early diagnosis of lung cancer.
Vanderbilt University Institute of Image Science’s (VUIIS) mission is to support collaborative image processing research at Vanderbilt by (1) creating infrastructure to interface medical image processing with high performance computing, (2) supporting implementation and standardization of medical image processing and (3) facilitating collaboration on medical image processing. Today, all human research scans taken at VUIIS or consented participants in any EDRN related study are automatically routed to a long-term PACS archive. To date all CT and MRI scans are being processed and archived. PACS is the institutional distributed medical image management system. These scans are then mirrored in an eXtensible Neuroimaging Toolkit (XNAT) server and fully de-identified to provide secure, multi-site access to data resources. XNAT is an open source imaging informatics platform [48]. It facilitates common management, productivity, and quality assurance tasks for imaging and associated data. XNAT supports a wide range of imaging based processes with its the federated (locally hosted) and distributed infrastructure for the storage, retrieval, analysis and documentation of biomedical imaging data [49]. XNAT follows a three-tiered architecture that includes a data archive, user interface and middleware engine. The infrastructure consists of distributed data collections hosted on dedicated storage and computational resources located at each participating site. This approach to local hosting and data management in an open-source environment allows for rapid spread of this architecture to interested collaborators with a federated data management system and data integration environment, an extensible markup language (XML) schema for data exchange and analysis pipelines. Thus, it is designed to leverage both the distributed data management environment and the locally available grid computing resources.
Figure 2.
Organization of data processing pipeline within the VUMC IT infrastructure. (A) are the collective data streams arising from clinical care, they are replicated into the Research Derivative (RD, B) or Synthetic Derivative (SD, C) for use by researchers (D) while an independent set of processes extract imaging data (E) for clinical and research uses.
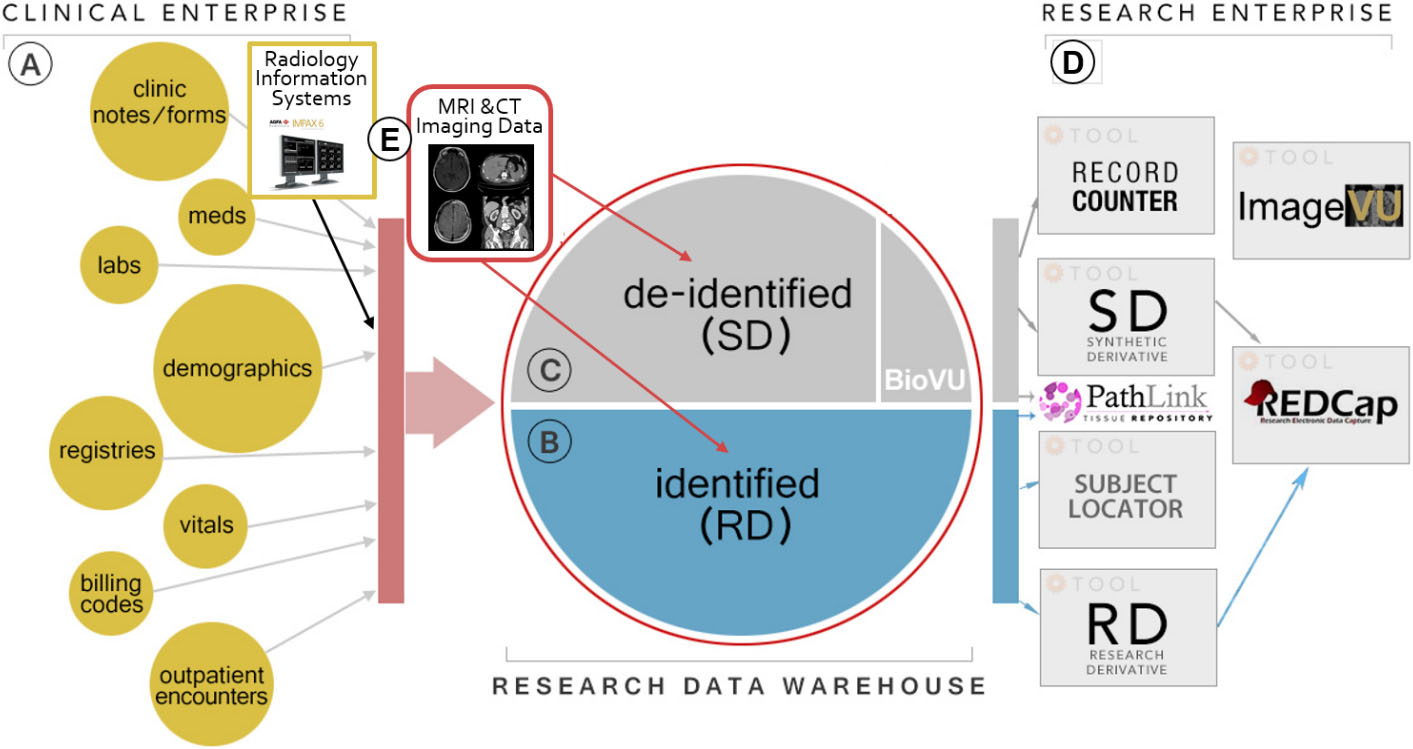
Demographics and non-imaging measures are reco-rded using Vanderbilt’s HIPAA compliant distributed REDCap database and matched with the XNAT archive (Fig. 2). To date over 251,000 patients, with 7.6 million imaging volume and 645 individual image files have been processed (E). These imaging data housed in the archive, called ImageVU, are then linked back to the patient clinical record either in the fully de-identified Synthetic Derivative (B), an identifier scrubbed version of the institutional electronic health record or in the Research Derivative (C), a nightly updated copy and searchable version of the electronic health record available to researchers as part of the Research Enterprise (D) with IRB oversight. Figure 2 shows the process workflow for these data streams.
5.VUMC EDRN lung CVC impact on biomarkers validation in industry
5.1Infrastructure available to industry
The EDRN Lung CVC has participated in industry-led and industry-supported biomarker development efforts across the range of biomarker development phases. Since the inception of the CVC, the VUMC CVC has collaborated with 13 biospecimen-focused industry partners and three imaging-focused partners. These collaborations are mutually beneficial, as industry support can offset some of the costs in maintaining sample and enrollee volumes while the specimens and independent validation offered to the industry collaborators ensure quality biomarker discovery and validation.
In contrast to pay-for-sample biobanks, the VUMC biospecimen repository offers well adjudicated quality sample sets that are de-identified, but the link to the patient’s medical record remains intact which allows further data acquisition if new endpoints or clinical data elements are desired which were not originally collected. Additionally, the biostatistics, clinical implementation infrastructure and epidemiological expertise at the CVC allows for true independent validation and replication of any industry-sponsored biomarkers. This independent analysis and validation is beneficial to the field overall, as any biomarkers that succeed in transition to commercial platforms are of the highest quality, but also beneficial to the industry partners as the data validation can then aide in submission for government regulatory approval. Lastly, the clinical trial infrastructure at the VUMC EDRN CVC enables easy transition from Phase I/II/III studies into interventional trials. This infrastructure, which is beneficial to the institution, the industry partners and the field as whole, would be unsustainable without the EDRN CVC foundation.
5.2Industry partnerships using biospecimens
These collaborations have facilitated biomarker development from Phase I through to commercially available products. For example, blood specimens from VUMC were included in the initial development and validation of an autoantibody panel that is now commercially available from Biodesix (Boulder, CA) in the US as the EarlyCDT test and from Oncimmune (Nottingham, UK) in Europe [14]. In addition to biospecimens, VUMC scientists contributed to the development effort, providing clinical, scientific and biostatistics expertise. Similarly, the multi-reaction-monitoring proteomic signature (MRM) that VUMC contributed specimens, clinical experience, scientific expertise and biostatistics validation for is also now available commercially from Biodesix as the NodifyXL2 test [25]. The magnetic nanosensor based biomarker detection platform for which VUMC provided a validation sample set and expertise is now commercially available from MagArray (Malpitas, CA) [18, 19, 50].
A collaboration between the EDRN CVC, Abbott Diagnostics, Lahey Medical Center and OneFlorida Clinical Research Consortium created a test case to assess “the economic feasibility, clinical utility, and medical validity of a blood-based in vitro diagnostic device (IVD) biomarker test panel (Class II/Class III) for risk stratification of suspicious lung nodules using Real-World Data (RWD)”. The test case was developed and overseen by the National Evaluation System for health Technology Coordinating Center (NESTcc), an FDA supported effort to generate real world evidence applicable to medical device development and surveillance. This project generated methods to define, collect and measure clinical utility data for the diagnosis of indeterminate pulmonary nodules (IPN). Clinical utility was defined as time to diagnosis and number of invasive or non-invasive diagnostic tests performed to achieve a diagnosis. This data collection methodology will be published and subsequently made available to the EDRN. Our approach allows for the retrospective evaluation of clinical utility among those with biological and imaging data in the CVC as their specimen and epidemiological survey data (see “Nodule Evaluation” in the supplementary material) are linked to their clinical data through the Research Derivative (Fig. 2, Section 4 above). Therefore, moving forward all future validation studies using EDRN CVC, CanVFun or other VUMC recruited participants will have the capability of measuring how the biomarker or combination of biomarkers might impact time to diagnosis and the healthcare effort necessary to achieve that diagnosis.
5.3Imaging industry partnerships
The VUMC imaging repository has collaborated with industry to develop and validate radiomic platforms that are in clinical use to improve the early detection of lung cancer. A cohort of patients undergoing CT scans at VUMC was used to validate the deep learning-based Lung Cancer Prediction-Convolutional Neural Network (LCP-CNN) which is now approved for use through the FDA and was developed and marketed by Optellum (Oxford, UK). In addition to the images, VUMC based
Table 4
Grant funding enabled by EDRN CVC
Title | PI | Grant ID or funding source | Funding period | Funding amount |
---|---|---|---|---|
Validation of biomarkers of Risk for the Early Detection of Lung Cancer | Grogan & Deppen(Massion) | 3U01CA152662-10S1 | 05/01/2016 – 03/31/2022 | $4,885,374.00 |
The Early Detection Research Network Data Management and Coordinating Center VUMC CORE: Validating an integrated biomarker and imaging approach in the evaluation of indeterminate pulmonary nodules | Grogan & Deppen | 5U24CA086368 (VUMC79673) | 04/01/2019 – 03/31/2022 | $262,288.00 |
Clinical Utility of Biomarkers Driven Management of Indeterminate Pulmonary Nodules | Grogan & Baron (Massion) | 1R01CA252964-01A1 | 05/01/2021 – 04/30/2026 | $3,399,733.00 |
Lavin Family Lung Cancer Research Fund | Grogan | Gift funding | 02/07/2019 – 02/06/2023 | $125,000.00 |
Decreasing Unnecessary Invasive Lung Cancer Diagnostic Procedures | Grogan | IK2HX000758 | 10/01/2011 – 09/30/2016 | $743,000.00 |
Improving Pathologic Nodal Staging of Resected Lung Cancer | Deppen | R01CA172253 (VUMC68849) | 08/22/2018 – 07/31/2021 | $28,651.00 |
Estimating and Validating Diagnostic Cancer Biomarker IVD Test Panel Characteristics and Clinical Utility for Indeterminate Pulmonary Nodule Risk Stratification in Patients with Lung Cancer | Deppen | NESTcc/Medical Device Innovation Consortium | 01/01/2020 – 03/31/2021 | $284,108.00 |
Cancer or Fungus? Avoiding Unnecessary Surgeries of Lung Nodules Suspicious for Cancer | Deppen | The Huff Project Lung Cancer Fund | 04/15/2019 – 03/15/2020 | $25,000.00 |
Improving Lung Cancer Screening and Biomarker Clinical Trial Enrollment in Underserved Communities | Deppen & Sandler | Precision Medicine and Health Disparities Collaborative Pilot Project Awards RFA | 04/01/2018 – 03/31/2019 | $50,000.00 |
Identifying racial disparities in population attributable risk for lung cancer | Aldrich | VICTR-Meharry Vanderbilt Alliance | 04/01/2019 – 02/29/2020 | $49,964.00 |
Understanding determinants of racial disparities in lung cancer incidence | Aldrich | U01CA253560 | 05/07/2020 – 04/30/2024 | $2,726,377.00 |
Understanding determinants of racial disparities in lung cancer stage | Aldrich | Lung Cancer Research Foundation | 10/01/2019 – 09/30/2021 | $147,780.00 |
Addressing racial disparities in lung cancer screening | Aldrich & Blume | R01CA251758-01A1 | 04/05/2021 – 03/31/2025 | $2,407,951.00 |
Studying Trial Determinants of Success (STRIDES): Understanding barriers and facilitators to clinical trial accrual among patients with lung cancer in the Deep South | Aldrich | Genentech, Health Equity Innovations Fund; Bristol Meyers Squibb | 01/01/2021 – 12/31/2022 | $100,000.00 |
EDRN Core Funds | Aldrich (Massion) | U01CA086402 | 07/01/2014 – 06/30/2019 | $213,472.00 |
Novel Integrative Approach for the Early Detection of Lung Cancer using Repeated Measures | Maldonado & Landman (Massion) | R01 CA253923-01 | 01/01/2021 – 12/31/2025 | $3,326,740.00 |
Noninvasive Characterization of Indeterminate Pulmonary Nodules Detected on Chest High-Resolution Computed Tomography | Maldonado | W81XWH-15-1-0110 | 09/30/2015 – 09/20/2021 | $399,497.00 |
Liquid biopsy of the lung to profile lung cancer | Maldonado | U01CA253166 (VUMC88362) | 09/15/2020 – 08/30/2025 | $85,000.00 |
Cellular, Molecular and Quantitative Imaging Analysis of Screening-Detected Lung Adenocarcinoma | Maldonado (Massion) | 5U01CA196405-06 | 09/24/2015 – 08/31/2021 | $5,359,710.00 |
Multimodal Intervention Strategies to Improve Lung Cancer Screening for Women Undergoing Breast Screening | Sandler & Thomson | American Cancer Society | 05/01/2021 – 12/31/2021 | $95,743.63 |
Utilization of Machine Learning to Predict Incidence Lung Cancer in a Screening Population | Sandler & Landman | Vanderbilt-Ingram Cancer Center | 08/01/2019 – 07/31/2020 | $40,000.00 |
Table 4, continued | ||||
---|---|---|---|---|
Title | PI | Grant ID or funding source | Funding period | Funding amount |
Saving Lives with Early Detection of Lung Cancer: Promoting enrollment of women engaged in breast screening in a lung screening program | Sandler | Vanderbilt-Ingram Cancer Center | 01/01/2019 – 12/31/2019 | $50,000.00 |
SPORE Pilot Project: Machine Learning for Prognosis Assessment | Sandler | University of Colorado and Vanderbilt-Ingram Cancer Center | 05/01/2018 – 04/30/2019 | $50,000.00 |
A Randomized Evaluation of Strategies to Increase Lung Cancer Screening Participation in Women who are Engaged in Breast Cancer Screening | Sandler | Vanderbilt-Ingram Cancer Center | 11/01/2017 – 10/31/2018 | $50,000.00 |
DECAMP | Massion (Site PI) | LC 100017 | 09/01/2013 – 09/29/2017 | $1,084,916.00 |
ECOG | Lovly (Site PI starting 03/01/2017) | 1U10CA180950 | 06/19/2014 – 02/28/2019 | $364,727.00 |
Non invasive evaluation of indeterminate pulmonary nodules | Massion | 1R01CA186145-01A1 | 08/03/2015 – 07/31/2020 | $1,843,200.00 |
Phenotypic heterogeneity in small cell lung cancer | Massion | 1I01CX001425-01 | 04/01/2016 – 03/31/2020 | $668,000.00 |
Mechanisms driving airway inflammation in chronic lung disease | Blackwell | 1I01BX002378-01A1 | 04/01/2014 – 06/20/2024 | $1,425,000.00 |
Total | $30,291,231.63 |
scientists, biostatisticians and radiologists collaborated in the development process to ensure proper implementation of the LCP-CNN for IPN management. Similar studies were performed for Phase I, II and III studies with Dr. Gillies that resulted in the HealthMyne radiomic platform [51, 52]. A Phase 4 study (R01CA252964 Grogan/Baron) using the HealthMyne radiomics combined with hsCYFRA 21.1 is now funded as a randomized clinical utility trial.
5.4Grants secured
The impact on lung cancer research at VUMC has been substantial. Multiple additional grants have been secured, in part due to efforts related to this EDRN CVC grant (Table 4). These include only grants obtained or pending at this institution. Most if not every collaborator has active funding applications related to this work and has benefited from our CVC infrastructure, but are not listed.
Summary and conclusions
The EDRN CVC funding mechanism has been highly successful in bringing biomarkers closer to the bedside in the field of lung cancer. The VUMC EDRN Lung CVC is an international resource for the validation of biospecimen and imaging biomarkers for the risk assessment and early detection of lung cancer. The next generation CVC will provide an infrastructure for multicenter Phase 4 adaptive clinical utility trials and expand the biorepository resource for additional validation studies with diverse populations.
Supplementary materials
EDRN Sample collection Standard Operating Procedures
CanVFun Recap intake form
Nodule Evaluation
Author contributions
Conception: MNK, SAD, ELG
Interpretation or analysis of data: SA, JR
Preparation of the manuscript: MNK, SAD, ELG
Revision for important intellectual content: BL, KS
Supervision: ELG
Acknowledgments
This work was funded by U01CA152662 to PPM. This work was built by and empowered through the bottomless energy, effort and hope of our dear colleague, mentor and friend Pierre Massion, MD.
References
[1] | Centers for Disease Control and Prevention. Rate of Cancer Deaths in the United States 2014–2018 2021 [updated 6/1/2021. Available from: https://gis.cdc.gov/Cancer/USCS/#/AtAGlance/. |
[2] | Centers for Disease Control and Prevention. Map of Current Cigarette Use Among Adults 2020 [updated 9/14/2020. Available from: https://www.cdc.gov/statesystem/cigaretteuseadult.html. |
[3] | D.R. Aberle, A.M. Adams, C.D. Berg, W.C. Black, J.D. Clapp, R.M. Fagerstrom et al., Reduced lung-cancer mortality with low-dose computed tomographic screening, New Engl J Med 365: (5) ((2011) ), 395–409. |
[4] | D.A. Lakhani, S.C. Chen, S. Antic, A. Muterspaugh, C. Cook, N. Liu et al., Establishing a Cohort and a Biorepository to Identify Biomarkers for Early Detection of Lung Cancer: The Nashville Lung Cancer Screening Trial Cohort, Ann Am Thorac Soc 18: (7) ((2021) ), 1227–34. |
[5] | E. Billatos, F. Duan, E. Moses, H. Marques, I. Mahon, L. Dymond et al., Detection of early lung cancer among military personnel (DECAMP) consortium: study protocols, BMC Pulm Med 19: (1) ((2019) ), 59. |
[6] | R. Paez, M.N. Kammer and P. Massion, Risk stratification of indeterminate pulmonary nodules, Curr Opin Pulm Med 27: (4) ((2021) ), 240–8. |
[7] | M.N. Kammer and P.P. Massion, Noninvasive biomarkers for lung cancer diagnosis, where do we stand? J Thorac Dis 12: (6) ((2020) ), 3317–30. |
[8] | L.M. Seijo, N. Peled, D. Ajona, M. Boeri, J.K. Field, G. Sozzi et al., Biomarkers in lung cancer screening: achievements, promises, and challenges, Journal of Thoracic Oncology: Official Publication of the International Association for the Study of Lung Cancer 14: (3) ((2019) ), 343–57. |
[9] | N. Al Nasrallah and C.R. Sears, Biomarkers in pulmonary nodule diagnosis: Is it time to put away the biopsy needle? Chest 154: (3) ((2018) ), 467–8. |
[10] | S.M. Rahman, A.L. Gonzalez, M. Li, E.H. Seeley, L.J. Zimmerman, X.J. Zhang et al., Lung cancer diagnosis from proteomic analysis of preinvasive lesions, Cancer Res 71: (8) ((2011) ), 3009–17. |
[11] | T. Kikuchi, M. Hassanein, J.M. Amann, Q.F. Liu, R.J.C. Slebos, S.M.J. Rahman et al., In-depth proteomic analysis of nonsmall cell lung cancer to discover molecular targets and candidate biomarkers, Mol Cell Proteomics 11: (10) ((2012) ), 916–32. |
[12] | C.V. Pecot, M. Li, X.J. Zhang, R. Rajanbabu, C. Calitri, A. Bungum et al., Added value of a serum proteomic signature in the diagnostic evaluation of lung nodules, Cancer Epidemiol Biomarkers Prev 21: (5) ((2012) ), 786–92. |
[13] | X.J. Li, C. Hayward, P.Y. Fong, M. Dominguez, S.W. Hunsucker, L.W. Lee et al., A blood-based proteomic classifier for the molecular characterization of pulmonary nodules, Sci Transl Med 5: (207) ((2013) ), 207ra142. |
[14] | P.P. Massion, G.F. Healey, L.J. Peek, L. Fredericks, H.F. Sewell, A. Murray et al., Autoantibody signature enhances the positive predictive power of computed tomography and nodule-based risk models for detection of lung cancer, Journal of Thoracic Oncology: Official Publication of the International Association for the Study of Lung Cancer 12: (3) ((2017) ), 578–84. |
[15] | D. Ajona, M. Okrój, M.J. Pajares, J. Agorreta, M.D. Lozano, J.J. Zulueta et al., Complement C4d-specific antibodies for the diagnosis of lung cancer, Oncotarget 9: (5) ((2018) ), 6346–55. |
[16] | D. Ajona, M.J. Pajares, L. Corrales, J.L. Perez-Gracia, J. Agorreta, M.D. Lozano et al., Investigation of complement activation product c4d as a diagnostic and prognostic biomarker for lung cancer, J Natl Cancer Inst 105: (18) ((2013) ), 1385–93. |
[17] | X. Yang, W. Su, X. Chen, Q. Geng, J. Zhai, H. Shan et al., Validation of a serum 4-microRNA signature for the detection of lung cancer, Transl Lung Cancer Res 8: (5) ((2019) ), 636–48. |
[18] | N.N. Trivedi, M. Arjomandi, J.K. Brown, T. Rubenstein, A.D. Rostykus, S. Esposito et al., Risk assessment for indeterminate pulmonary nodules using a novel, plasma-protein based biomarker assay, Biomed Res Clin Pract 3: (4) ((2018) ). |
[19] | A. Fish, A. Vachani, P. Massion, S. Antic, N. Trivedi, J. Brown et al., Novel multiplexed plasma biomarkers and clinical factors augment risk assessment for indeterminate pulmonary nodules in former smokers, American Journal of Respiratory and Critical Care Medicine 199: ((2019) ). |
[20] | I.R. Olmsted, M. Hassanein, A. Kussrow, M. Hoeksema, M. Li, P.P. Massion et al., Toward rapid, high-sensitivity, volume-constrained biomarker quantification and validation using backscattering interferometry, Analytical Chemistry 86: (15) ((2014) ), 7566–74. |
[21] | M.N. Kammer, A.K. Kussrow, R.L. Webster, H. Chen, M. Hoeksema, R. Christenson et al., Compensated Interferometry Measures of CYFRA 21-1 Improve Diagnosis of Lung Cancer, ACS Comb Sci 21: (6) ((2019) ), 465–72. |
[22] | J.J. Chabon, E.G. Hamilton, D.M. Kurtz, M.S. Esfahani, E.J. Moding, H. Stehr et al., Integrating genomic features for non-invasive early lung cancer detection, Nature 580: (7802) ((2020) ), 245–51. |
[23] | G. Smith, A. Rahman, M. Li, B. Moore, H. Gietema, G. Veronesi et al., Reproducibility of volumetric computed tomography of stable small pulmonary nodules with implications on estimated growth rate and optimal scan interval, Journal of Thoracic Imaging (2015) ; submitted. |
[24] | D. Ajona, A. Remirez, C. Sainz, C. Bertolo, A. Gonzalez, N. Varo et al., A model based on the quantification of complement C4c, CYFRA 21-1 and CRP exhibits high specificity for the early diagnosis of lung cancer, Transl Res 233: ((2021) ), 77–91. |
[25] | G.A. Silvestri, N.T. Tanner, P. Kearney, A. Vachani, P.P. Massion, A. Porter et al., Assessment of plasma proteomics biomarker’s ability to distinguish benign from malignant lung nodules: Results of the PANOPTIC (Pulmonary Nodule Plasma Proteomic Classifier) Trial, Chest 154: (3) ((2018) ), 491–500. |
[26] | J. Qian, M. Hassanein, M.D. Hoeksema, B.K. Harris, Y. Zou, H. Chen et al., The RNA binding protein FXR1 is a new driver in the 3q26-29 amplicon and predicts poor prognosis in human cancers, PNAS, (2015) ; Submitted. |
[27] | M. Kammer, D. Lakhani, A. Balar, S. Antic, A. Kussrow, R. Webster et al., Biomarker informed management of indeterminate pulmonary nodules with a combined clinical, blood and imaging-based biomarker strategy, D99 ADVANCING RISK ASSESSEMENT FOR PULMONARY NODULES: American Thoracic Society, (2020) . p. A7694-A. |
[28] | S. Mahapatra, M. Kammer, S. Antic, D. Lakhani, A. Balar, A. Miller et al., Clinical Utility of Combined Biomarker Model to Manage Indeterminate Pulmonary Nodules, B110 IMAGING AND MOLECULAR BIOMARKERS FOR LUNG CANCER: American Thoracic Society, (2020) . p. A4443-A. |
[29] | M.E. Shipe, S.A. Deppen, S. Sullivan, M. Kammer, S.L. Starnes, D.O. Wilson et al., Validation of histoplasmosis enzyme immunoassay to evaluate suspicious lung nodules, The Annals of Thoracic Surgery 111: (2) ((2021) ), 416–20. |
[30] | R. Gao, Y. Tang, K. Xu, M.N. Kammer, S.L. Antic, S. Deppen et al., editors. Deep multi-path network integrating incomplete biomarker and chest CT data for evaluating lung cancer risk, Medical Imaging 2021: Image Processing; 2021: International Society for Optics and Photonics. |
[31] | R. Gao, Y. Huo, S. Bao, Y. Tang, S.L. Antic, E.S. Epstein et al., Multi-path x-D Recurrent Neural Networks for Collaborative Image Classification, Neurocomputing 397: ((2020) ), 48–59. |
[32] | R. Gao, Y. Tang, K. Xu, Y. Huo, S. Bao, S.L. Antic et al., Time-distanced gates in long short-term memory networks, Medical Image Analysis 65: ((2020) ), 101785. |
[33] | R. Gao, L. Li, Y. Tang, S.L. Antic, A.B. Paulson, Y. Huo et al., Deep multi-task prediction of lung cancer and cancer-free progression from censored heterogenous clinical imaging, Proc SPIE Int Soc Opt Eng ((2020) ), 11313. |
[34] | Y. Yang, R. Gao, Y. Tang, S.L. Antic, S. Deppen, Y. Huo et al., Internal-transfer Weighting of Multi-task Learning for Lung Cancer Detection, Proc SPIE Int Soc Opt Eng ((2020) ), 11313. |
[35] | S.A. Deppen and E.L. Grogan, Using clinical risk models for lung nodule classification, Seminars in Thoracic and Cardiovascular Surgery 27: (1) ((2015) ), 30–5. |
[36] | S.A. Deppen, J.D. Blume, M.C. Aldrich, S.A. Fletcher, P.P. Massion, R.C. Walker et al., Predicting lung cancer prior to surgical resection in patients with lung nodules, Journal of Thoracic Oncology: Official Publication of the International Association for the Study of Lung Cancer 9: (10) ((2014) ), 1477–84. |
[37] | F. Maldonado, C. Varghese, S. Rajagopalan, F. Duan, A.B. Balar, D.A. Lakhani et al., Validation of the BRODERS classifier (Benign versus aggRessive nODule Evaluation using Radiomic Stratification), a novel HRCT-based radiomic classifier for indeterminate pulmonary nodules, Eur Respir J 57: (4) ((2021) ). |
[38] | R.L. Harrigan, B.C. Yvernault, B.D. Boyd, S.M. Damon, K.D. Gibney, B.N. Conrad et al., Vanderbilt University Institute of Imaging Science Center for Computational Imaging XNAT: A multimodal data archive and processing environment, NeuroImage (2015) . |
[39] | R. Herrick, W. Horton, T. Olsen, M. McKay, K.A. Archie, D.S. Marcus, XNAT Central: Open sourcing imaging research data, Neuroimage 124: (Pt B) ((2016) ), 1093–6. |
[40] | Y. Schwartz, A. Barbot, B. Thyreau, V. Frouin, G. Varoquaux, A. Siram et al., PyXNAT: XNAT in python, Front Neuroinform 6: ((2012) ), 12. |
[41] | R.L. Harrigan, B.C. Yvernault, B.D. Boyd, S.M. Damon, K.D. Gibney, B.N. Conrad et al., Vanderbilt university institute of imaging science center for computational imaging XNAT: A multimodal data archive and processing environment, Neuroimage 124: (Pt B) ((2016) ), 1097–101. |
[42] | J. Wang, R. Gao, Y. Huo, S. Bao, Y. Xiong, S.L. Antic et al., Lung cancer detection using co-learning from chest CT images and clinical demographics, Proc SPIE Int Soc Opt Eng 10949: ((2019) ). |
[43] | E.C. Nakajima, M.P. Frankland, T.F. Johnson, S.L. Antic, H. Chen, S.C. Chen et al., Assessing the inter-observer variability of Computer-Aided Nodule Assessment and Risk Yield (CANARY) to characterize lung adenocarcinomas, PLoS One 13: (6) ((2018) ), e0198118. |
[44] | R. Gao, L. Li, Y. Tang, S.L. Antic, A.B. Paulson, Y. Huo et al., editors. Deep Multi-task Prediction of Lung Cancer and Cancer-free Progression from Censored Heterogenous Clinical Imaging, SPIE MI:IP 2020 2020; Houston, TX. |
[45] | Y. Yang, R. Gao, Y. Tang, S.L. Antic, S. Deppen, Y. Huo et al., editors. Internal-transfer Weighting of Multi-task Learning for Lung Cancer Detection, SPIE IP:MI 2020; 2020; Houston, TX. |
[46] | R. Gao, Y. Huo, S. Bao, Y. Tang, S.L. Antic, E.S. Epstein et al., editors. Distanced LSTM: Time-Distanced Gates in Long Short-Term Memory Models for Lung Cancer Detection, MICCAI Workshop on Machine learning in medical imaging; 2020; Shenzhen, China. |
[47] | P.P. Massion, S. Antic, S. Ather, C. Arteta, J. Brabec, H. Chen et al., Assessing the accuracy of a deep learning method to risk stratify indeterminate pulmonary nodules, American Journal of Respiratory and Critical Care Medicine 202: (2) ((2020) ), 241–9. |
[48] | D.S. Marcus, K.A. Archie, T.R. Olsen and M. Ramaratnam, The open-source neuroimaging research enterprise, Journal of Digital Imaging 20: (1) ((2007) ), 130–8. |
[49] | D.S. Marcus, T.R. Olsen, M. Ramaratnam and R.L. Buckner, The Extensible Neuroimaging Archive Toolkit: an informatics platform for managing, exploring, and sharing neuroimaging data, Neuroinformatics 5: (1) ((2007) ), 11–34. |
[50] | A. Fish, A. Vachani, M. Beggs, L. Carbonell, Z. Haddad, A. Juang et al., Risk assessment for indeterminate pulmonary nodules using a novel, plasma-protein based biomarker assay, Journal of Thoracic Oncology 13: (10) ((2018) ), S439-S. |
[51] | Y. Balagurunathan, M.B. Schabath, H. Wang, Y. Liu and R.J. Gillies, Quantitative Imaging features Improve Discrimination of Malignancy in Pulmonary nodules, Scientific Reports 9: (1) ((2019) ), 8528. |
[52] | Y. Liu, Y. Balagurunathan, T. Atwater, S. Antic, Q. Li, R.C. Walker et al., Radiological image traits predictive of cancer status in pulmonary nodules, Clinical Cancer Research: An Official Journal of the American Association for Cancer Research 23: (6) ((2017) ), 1442–9. |
[53] | P.B. Yildiz, Y. Shyr, J.S. Rahman, N.R. Wardwell, L.J. Zimmerman, B. Shakhtour et al., Diagnostic accuracy of MALDI mass spectrometric analysis of unfractionated serum in lung cancer, Journal of Thoracic Oncology: Official Publication of the International Association for the Study of Lung Cancer 2: (10) ((2007) ), 893–901. |
[54] | S.M.J. Rahman, X. Ji, L.J. Zimmerman, M. Li, B.K. Harris, M.D. Hoeksema et al., The airway epithelium undergoes metabolic reprogramming in individuals at high risk for lung cancer, JCI Insight 1: (19) ((2016) ), e88814. |
[55] | A. Vachani, H.I. Pass, W.N. Rom, D.E. Midthun, E.S. Edell, M. Laviolette et al., Validation of a multi-protein plasma classifier to identify benign lung nodules, Journal of Thoracic Oncology: Official Publication of the International Association for the Study of Lung Cancer, (2015) . |
[56] | M. Hassanein, J. Qian, M.D. Hoeksema, J. Wang, M. Jacobovitz, X. Ji et al., Targeting SLC1A5-mediated glutamine dependence in non-small cell lung cancer, Int J Cancer ((2014) ); submitted. |
[57] | M. Kammer, A. Kussrow, R. Webster, H. Chen, M. Hoeksemas, R. Christenson et al., Compensated interferometry measures of CYFRA 21-1 improve diagnosis of lung cancer, Acs Combinatorial Science 21: (6) ((2019) ), 465–72. |
[58] | M. Kammer, A. Kussrow, S. Antic, R. Nguyen, H. Chen, D. Bornhop et al., Validation of a new blood based biomarker strategy for the early detection of lung cancer, Cancer Res 79: (13) ((2019) ). |
[59] | S.A. Deppen, P.P. Massion, J. Blume, R.C. Walker, S. Antic, H. Chen et al., Accuracy of a novel histoplasmosis enzyme immunoassay to evaluate suspicious lung nodules, Cancer Epidemiol Biomarkers Prev 28: (2) ((2019) ), 321–6. |
[60] | V.V. Polosukhin, B.W. Richmond, R.H. Du, J.M. Cates, P. Wu, H. Nian et al., Secretory IgA deficiency in individual small airways is associated with persistent inflammation and remodeling, American Journal of Respiratory and Critical Care Medicine 195: (8) ((2017) ), 1010–21. |
[61] | Y. Zou, A. Borczuk, C.A. Powell and P.P. Massion, D30 NOVEL CLINICAL APPROACHES TO THORACIC MALIGNANCIES: Mdm2 gene copy number gain distinguishes aggressive from indolent lung adenocarcinomas, American Journal of Respiratory and Critical Care Medicine 193: ((2016) ), 1. |
[62] | P.P. Massion, Y. Zou, H. Uner, P. Kiatsimkul, H.J. Wolf, A.E. Baron et al., Recurrent genomic gains in preinvasive lesions as a biomarker of risk for lung cancer, PloS One 4: (6) ((2009) ), e5611. |
[63] | J. Beane, A. Spira and M.E. Lenburg, Clinical impact of high-throughput gene expression studies in lung cancer, Journal of Thoracic Oncology: Official Publication of the International Association for the Study of Lung Cancer 4: (1) ((2009) ), 109–18. |
[64] | J. Yeo, D.A. Morales, T. Chen, E.L. Crawford, X. Zhang, T.M. Blomquist et al., RNAseq analysis of bronchial epithelial cells to identify COPD-associated genes and SNPs, BMC Pulmonary Medicine 18: (1) ((2018) ), 42. |
[65] | E. Nadal, A. Truini, A. Nakata, J. Lin, R.M. Reddy, A.C. Chang et al., A Novel Serum 4-microRNA signature for lung cancer detection, Scientific Reports 5: ((2015) ), 12464. |
[66] | X. Ji, J. Qian, S.M.J. Rahman, P.J. Siska, Y. Zou, B.K. Harris et al., xCT (SLC7A11)-mediated metabolic reprogramming promotes non-small cell lung cancer progression, Oncogene 37: (36) ((2018) ), 5007–19. |
[67] | P. Massion, S. Ather, C. Arteta, J. Brabec, H. Chen, J. Declerck et al., Assessing the accuracy of a deep learning method to risk stratify indeterminate pulmonary nodules, American Journal of Respiratory and Critical Care Medicine ((2020) ). |
[68] | D.R. Baldwin, J. Gustafson, L. Pickup, C. Arteta, P. Novotnys, J. Declerck et al., External validation of a convolutional neural network artificial intelligence tool to predict malignancy in pulmonary nodules, Thorax 75: (4) ((2020) ), 306–12. |
[69] | A. Balar, D. Lakhani, L. Roller, S. Antic, H. Chen, M. Kammer et al., Can Longitudinal Analysis of Computer Tomography-Based Score Indicative of Lung Cancer Aggression (SILA) Help in the Early Characterization of Lung Adenocarcinoma? D99 ADVANCING RISK ASSESSEMENT FOR PULMONARY NODULES: American Thoracic Society, (2020) . pp. A7692-A. |
[70] | S.A. Deppen, E. Liu, J.D. Blume, J. Clanton, C. Shi, L.B. Jones-Jackson et al., Safety and Efficacy of 68Ga-DOTATATE PET/CT for Diagnosis, Staging, and Treatment Management of Neuroendocrine Tumors, J Nucl Med 57: (5) ((2016) ), 708–14. |
[71] | S.A. Deppen, J. Blume, A.J. Bobbey, C. Shah, M.M. Graham, P. Lee et al., 68Ga-DOTATATE Compared with 111In-DTPA-octreotide and conventional imaging for pulmonary and gastroenteropancreatic neuroendocrine tumors: a systematic review and meta-analysis, J Nucl Med 57: (6) ((2016) ), 872–8. |
[72] | M. Hassanein, M.R. Hight, J.R. Buck, M.N. Tantawy, M.L. Nickels, M.D. Hoeksema et al., Preclinical Evaluation of 4-[18F]Fluoroglutamine PET to Assess ASCT2 Expression in Lung Cancer, Molecular imaging and biology: MIB: the official publication of the Academy of Molecular Imaging 18: (1) ((2016) ), 18–23. |
[73] | S.A. Deppen, J.D. Blume, C.D. Kensinger, A.M. Morgan, M.C. Aldrich, P.P. Massion et al., Accuracy of FDG-PET to Diagnose Lung Cancer in Areas With Infectious Lung Disease: A Meta-analysis, JAMA: the journal of the American Medical Association 312: (12) ((2014) ), 1227–36. |
[74] | F. Maldonado, C. Varghese, S. Rajagopalan, F. Duan, A.B. Balar, D.A. Lakhani et al., Validation of the BRODERS classifier (Benign ersus aggRessive nODule Evaluation using Radiomic Stratification), a novel HRCT-based radiomic classifier for indeterminate pulmonary nodules, Eur Respir J 57: (4) ((2021) ), 2002485. |