Preoperative Risk Factors Predicting Postoperative Complications in Radical Cystectomy for Bladder Cancer
Abstract
BACKGROUND AND OBJECTIVE:
While radical cystectomy (RC) is the gold-standard treatment for patients with muscle-invasive bladder cancer, it is associated with a significant rate of complications. We aim to develop a prediction model to assess the risk of complications in the postoperative period using routinely collected data in the course of preoperative evaluation in patients undergoing RC for bladder cancer.
METHODS:
We retrospectively reviewed 508 patients who underwent RC for bladder cancer from January 2008 to October 2016. Potential preoperative risk predictors were collected. Postoperative complications were graded using the Clavien-Dindo scale. Prediction models were developed using variables with the highest predictive value for postoperative complications. The accuracy of themodels was assessed using the area under the receiver operating characteristic curve (AUROC) and cross-validation.
RESULTS:
Variables achieved the highest prediction accuracy for major postoperative complications in the 31 to 90-day postoperative period. In this period, 14 variables were predictive of major postoperative complications. The three most predictive individual variables were BMI, neoadjuvant chemotherapy, and creatinine with AUROC/odds ratios of 0.643/1.09, 0.609/2.43, and 0.597/1.22, respectively. This postoperative period also had the best performing prediction model for major complications, which utilized four variables to achieve an AUROC of 0.727.
CONCLUSION:
Routinely collected preoperative variables may be useful for determining patient risk for short-term postoperative complications after RC. Prediction models can help identify patients who may benefit from patient education, counseling and development of risk reduction strategies. Interactions between individual variables should be evaluated to further improve accuracy of the prediction models.
INTRODUCTION
Bladder cancer is the seventh leading cause of cancer mortality in the United States with an estimated 17,600 deaths in 2019 [1]. The number of new bladder cancer cases is estimated to be over 80,000 in 2019, constituting 4.6% of all new cancer cases [1]. Approximately 30% of incident cases will present as muscle-invasive bladder cancer (MIBC) [2]. Radical cystectomy (RC) based treatment remains the gold-standard for patients with MIBC (T2-T4a N0M0) [3]. Additionally, RC is also indicated in patients with recurrent or progressive non-muscle invasive bladder cancer [4].
Although RC is the standard procedure for patients with MIBC and is recommended for patients with recurrent or progressive non-muscle invasive bladder cancer, it is associated with a significant rate of complications, hospital readmission, and mortality. The high frequency of complications associated with RC likely result from performing a complex operation in an elderly patient population, typically with significant comorbid conditions. While RC morbidity and perioperative mortality rates have decreased significantly in the last few decades [5], an estimated 30% of patients experience postoperative complications within 30 days of surgery and an estimated 60% of patients experience complications within 90 days of the procedure [6]. Common complications include gastrointestinal (30%), infectious (25%), wound and stoma (15%), upper urinary tract (11%), cardiovascular (11%) and venous thromboembolism (8%) [7]. These complications prolong a patient’s length of stay in the hospital, increase total cost of treatment, and diminish quality of life [2]. Patient selection for RC is difficult and we often use the “eye ball test” as opposed to validated risk stratification tools that anchor on a patient’s risk factors. This underscores the need for preoperative tools to identify patients at high risk of complications and develop interventions that modify these factors. Currently, there is a lack of tools to assess risk in bladder cancer, in particular, utilizing only preoperative risk factors. Using patient data, we have created prediction models to predictmajor complications occurring in the timeframes of 30-day following RC, 31 to 90-day following RC and 90-day following RC.
MATERIALS AND METHODS
After Institutional Review Board approval (STUDY00140149), a retrospective review of the electronic medical record (EMR) was performed to identify 508 patients who had undergone radical cystectomy for bladder cancer at the University of Kansas Medical Center (KUMC) from January 2008 to January 2016. The data was collected retrospectively and entered into REDCap. The data collected from each patient were categorized into six categories: Demographic, Preoperative, Operative, Postoperative, Pathology, and Clinical Status. Demographics included a patient’s age, gender, race, and date of surgery. Preoperative information included date of diagnosis, smoking status, comorbidities, laboratory values, chemotherapy or radiation treatment, and clinical stage. Operative information included surgical approach, type of diversion, and estimated blood loss. Postoperative information included major and minor complications as defined by the Clavien-Dindo grading scale, need for blood transfusions, postoperative radiation or chemotherapy treatment, and postoperative laboratory values.
Using R Statistical Programming Language, univariable analysis of preoperative variables was performed to determine each variable’s association with postoperative complications in three timeframes: 30-day postoperative period, 31 to 90-day postoperative period, and 90-day postoperative period.Variables that were associated with an increased risk for major complications were selected in designing the prediction model for each postoperative timeframe. Prediction models were developed by testing all possible combinations of the selected variables. The prediction performance of each model is assessed by the average area under the receiver operating characteristic curve (AUROC) utilizing 10-fold cross-validation. This was repeated 100 times and averaged to achieve more consistent results. The variables with the highest predictive value for major complications in each postoperative period were then selected to develop prediction models for that postoperative timeframe. The 20 best performing models, along with the individual variables that make up each model, were determined for each of the three postoperative timeframes.
RESULTS
Between January 2008 and January 2016, we identified 508 patients, 408 males (80.5%) and 100 females (19.5%), who underwent radical cystectomy for bladder cancer. Preoperative tumor biopsiesdemonstrated that 379 patients (74.6%) had disease stage T2 or higher, 85 patients (16.7%) had stage T1, 25 patients (4.9%) had stage Ta, and 19 patients (3.7%) had stage Tis. Neoadjuvant chemotherapy was administered to 198 patients (39%) (Table 1).
Table 1
Preoperative and Intraoperative Patient Characteristics
Preoperative Patient Characteristics | ||
Age median (range) | 68 | (33– 91) |
BMI average (range) | 28.8 | (16– 59.8) |
No. Pts | (%) | |
Male | 408 | (80.3) |
Female | 100 | (19.7) |
Race | ||
White/Caucasian not Hispanic | 484 | (95.2) |
Other | 24 | (4.8) |
Smoking Status | ||
Current or Former Smoker | 385 | (75.8) |
Never smoked | 123 | (24.2) |
Comorbidities | ||
Hypertension | 293 | (57.7) |
Hyperlipidemia | 214 | (42.1) |
Diabetes | 117 | (23.0) |
Coronary Artery Disease | 108 | (21.3) |
Asthma/COPD | 79 | (15.6) |
Obstructive Sleep Apnea | 25 | (4.9) |
Cerebrovascular Accident | 20 | (3.9) |
Congestive Heart Failure | 14 | (2.8) |
Preoperative Clinical Staging* | ||
Tis | 19 | (3.7) |
Ta | 25 | (4.9) |
T1 | 85 | (16.7) |
T2 or higher | 379 | (74.6) |
CIS present (any stage) | 101 | (19.9) |
Multifocal | 44 | (8.7) |
Neoadjuvant Chemotherapy | 198 | (39.0) |
Intraoperative Characteristics | ||
Open Surgical Approach | 387 | (76.2) |
Robotic Surgical Approach | 121 | (23.8) |
Diversion Type | ||
Ileal Conduit | 345 | (67.9) |
Neobladder | 157 | (30.9) |
Indiana Pouch | 3 | (0.6) |
No Diversion | 3 | (0.6) |
*Preoperative clinical staging is determined by highest stage found on pathology after TURBT/biopsy.
Complications within 90 days post-radical cystectomy were classified using the Clavien-Dindo scale with Clavien-Dindograde II or lower complications considered minor complications and grade III or higher complications classified as major complications. During the 90-day postoperative period, a total of 336 patients (66.1%) had at least one complication of any grade for a total of 470 complications. Of the total complications, 140 (29.8%) were major and 330 (70.2%) were minor complications. The Clavien-Dindo grade for all complications were 70 (14.9%) grade I, 260 (55.3%) grade II, 116 (24.7%) grade III, 17 (3.6%) grade IV and 7 (1.5%) grade V. We further divided postoperative complications to those occurring within 30 days of surgery and those occurring 31 to 90-days after surgery. When assessing the number of patients who experienced a major complication, we found 94 patients had a major complication within 30 days of surgery, 32 patients within 31 to 90 days, and 121 patients within 90 days. When evaluating the total number of complications that occurred in the 90-day postoperative period, we found that 407 complications occurred within 30 days of surgery and 63 complications occurred during the 31 to 90-day postoperative period. The Clavien-Dindo grade breakdown for complications occurring during these postoperative periods can be found in Table 2.
Table 2
Post-Radical Cystectomy Complications by Postoperative Period and Clavien-Dindo Classification
0– 90 d | <30 d | 31– 90 d | ||||
n | (%) | n | (%) | n | (%) | |
Total Complications | 470 | 100 | 407 | 100 | 63 | 100 |
Total Minor | 330 | 70.2 | 300 | 73.7 | 30 | 47.6 |
Grade I | 70 | 14.9 | 66 | 16.2 | 4 | 6.3 |
Grade II | 260 | 55.3 | 234 | 57.5 | 26 | 41.3 |
Total Major | 140 | 29.8 | 107 | 26.3 | 33 | 52.4 |
Grade III | 116 | 24.7 | 86 | 21.1 | 30 | 47.6 |
Grade IV | 17 | 3.6 | 15 | 3.7 | 2 | 3.2 |
Grade V | 7 | 1.5 | 6 | 1.5 | 1 | 1.6 |
Eight of the 28 variables were associated with increased risk for major complication in the 30-day postoperative period, 14 of the 28 variables were associated increased risk for major complication in the 31 to 90-day postoperative period, and 10 of the 28 were associated increased risk formajor complication in the 90-day postoperative period. Variables achieved the highest prediction accuracy for major complications within the 31 to 90-day postoperative period. In this postoperative period, the three mostpredictive variables were BMI, neoadjuvant chemotherapy, and creatinine with AUROC/odds ratios of 0.643/1.09, 0.609/2.43, and 0.597/1.22, respectively. The most predictive variables in the 30-day postoperative window were COPD, neobladder diversion, and neoadjuvant chemotherapy with AUROC/odds ratios of 0.529/1.49, 0.522/1.22, and 0.522/1.20, respectively. The most predictive variables in the 90-day postoperative period were BMI, neoadjuvant chemotherapy, and smoking pack years with AUROC/odds ratios of 0.552/1.04, 0.537/1.36, and 0.531/1.26, respectively. The results from the univariate analysis can be found in Tables 3–5.
Table 3
Univariable Analysis for High Grade Postoperative Complications within 90 Days
Preoperative Variable | Odds Ratio | p-value | AUC |
BMI | 1.04 | 0.03 | 0.552 |
Neoadjuvant Chemotherapy | 1.36 | 0.14 | 0.537 |
Smoking (≤20 pack years) | 1.26 | 0.43 | 0.531 |
Coronary Artery Disease | 1.31 | 0.28 | 0.523 |
Robotic Approach | 1.27 | 0.31 | 0.523 |
Chronic Obstructive Pulmonary Disease | 1.29 | 0.36 | 0.517 |
Neobladder Diversion | 1.15 | 0.52 | 0.516 |
Obstructive Sleep Apnea | 1.54 | 0.33 | 0.511 |
CIS Presence | 1.14 | 0.61 | 0.511 |
Cerebrovascular Accident | 1.07 | 0.90 | 0.501 |
Table 4
Univariable Analysis for High Grade Postoperative Complications within 30 Days
Preoperative Variable | Odds Ratio | p-value | AUC |
Chronic Obstructive Pulmonary Disease | 1.49 | 0.17 | 0.529 |
Neobladder Diversion | 1.22 | 0.40 | 0.522 |
Neoadjuvant Chemotherapy | 1.20 | 0.43 | 0.522 |
BMI | 1.02 | 0.44 | 0.522 |
Obstructive Sleep Apnea | 1.77 | 0.22 | 0.515 |
CIS Presence | 1.20 | 0.51 | 0.515 |
Coronary Artery Disease | 1.08 | 0.78 | 0.507 |
Male | 1.04 | 0.88 | 0.503 |
Table 5
Univariable Analysis for High Grade Postoperative Complications within 31 to 90-Days
Preoperative Variable | Odds Ratio | p-value | AUC |
BMI | 1.09 | <0.01 | 0.643 |
Neoadjuvant Chemotherapy | 2.43 | 0.02 | 0.609 |
Creatinine | 1.22 | 0.41 | 0.597 |
Robotic Approach | 2.33 | 0.02 | 0.590 |
Smoking (≤20 pack years) | 1.87 | 0.24 | 0.588 |
Coronary Artery Disease | 1.75 | 0.16 | 0.553 |
Diabetes | 1.57 | 0.26 | 0.544 |
Hyperlipidemia | 1.40 | 0.35 | 0.542 |
Number of Comorbidities | 1.09 | 0.49 | 0.517 |
Neobladder Diversion | 1.15 | 0.72 | 0.515 |
Cerebrovascular Accident | 1.70 | 0.49 | 0.512 |
Obstructive Sleep Apnea | 1.31 | 0.72 | 0.507 |
Male | 1.07 | 0.89 | 0.505 |
Congestive Heart Failure | 1.15 | 0.90 | 0.502 |
Variables associated with increased risk for major complications were selected in designing the prediction modelin that postoperative timeframe. Therefore, eight variables were used forthe 30-day postoperative period, 14 forthe 31 to 90-day postoperative period, and 10 for the 90-day postoperative period. All combinations of the selected variables were used to design twenty of the best performing prediction models in each postoperative period. The AUROCs of the prediction models ranged from 0.551 to 0.563 in the 90-day postoperative period, 0.532 to 0.551 in the 30-day postoperative period, and 0.715 to 0.727 in the 31 to 90-day postoperative period. The best performing prediction model for major complications in the 31 to 90-day postoperative period utilized the variables of BMI, neoadjuvant chemotherapy, creatinine and robotic approach to achieve an AUROC of 0.727. Figures 1–3 illustrate the prediction models using a heatmap, while Tables 6–8 demonstrate the odds ratios and associated p-values for individual variables within the five top performing models in each postoperative timeframe. A small degree of variability exists in the values of the odds ratio and p-value across the models due to different composition of each model, though these values remain largely consistent across prediction models.
Fig. 1
Prediction Models for High Grade Post-Op Complications within 90 Days.
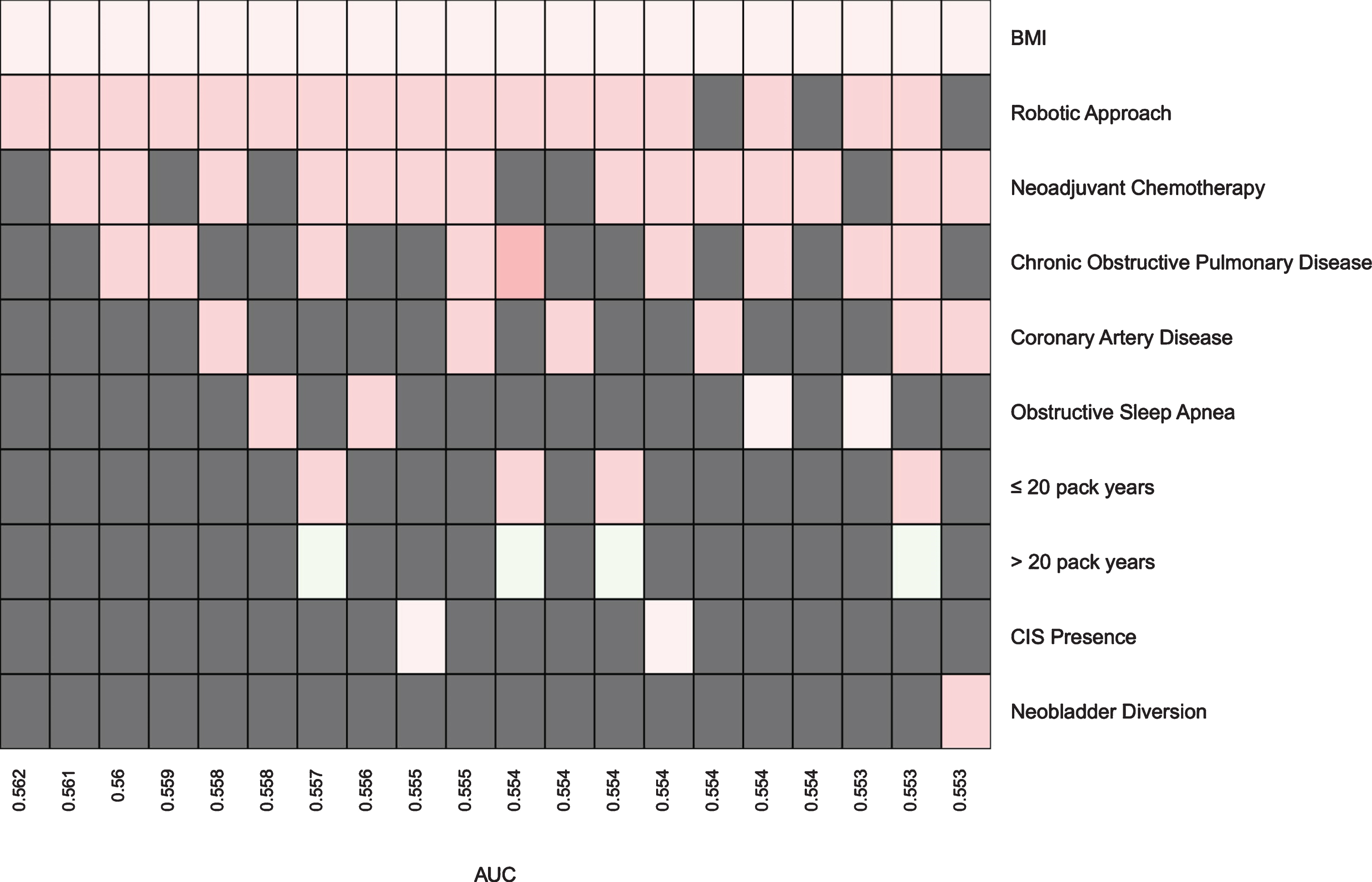
Fig. 2
Prediction Models for High Grade Post-Op Complications within 30 Days.
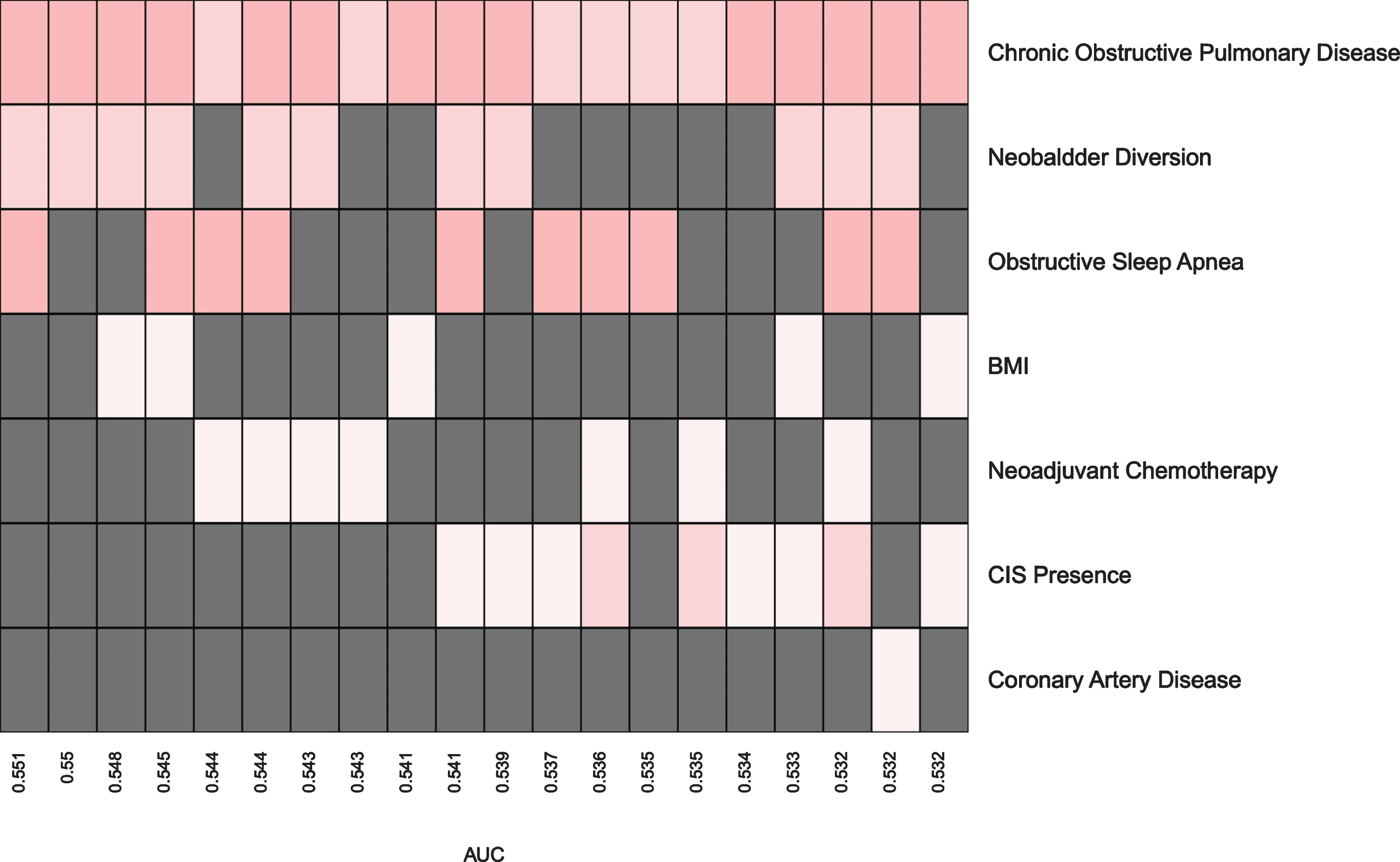
Fig. 3
Prediction Models for High Grade Post-Op Complications within 31 to 90-Days.
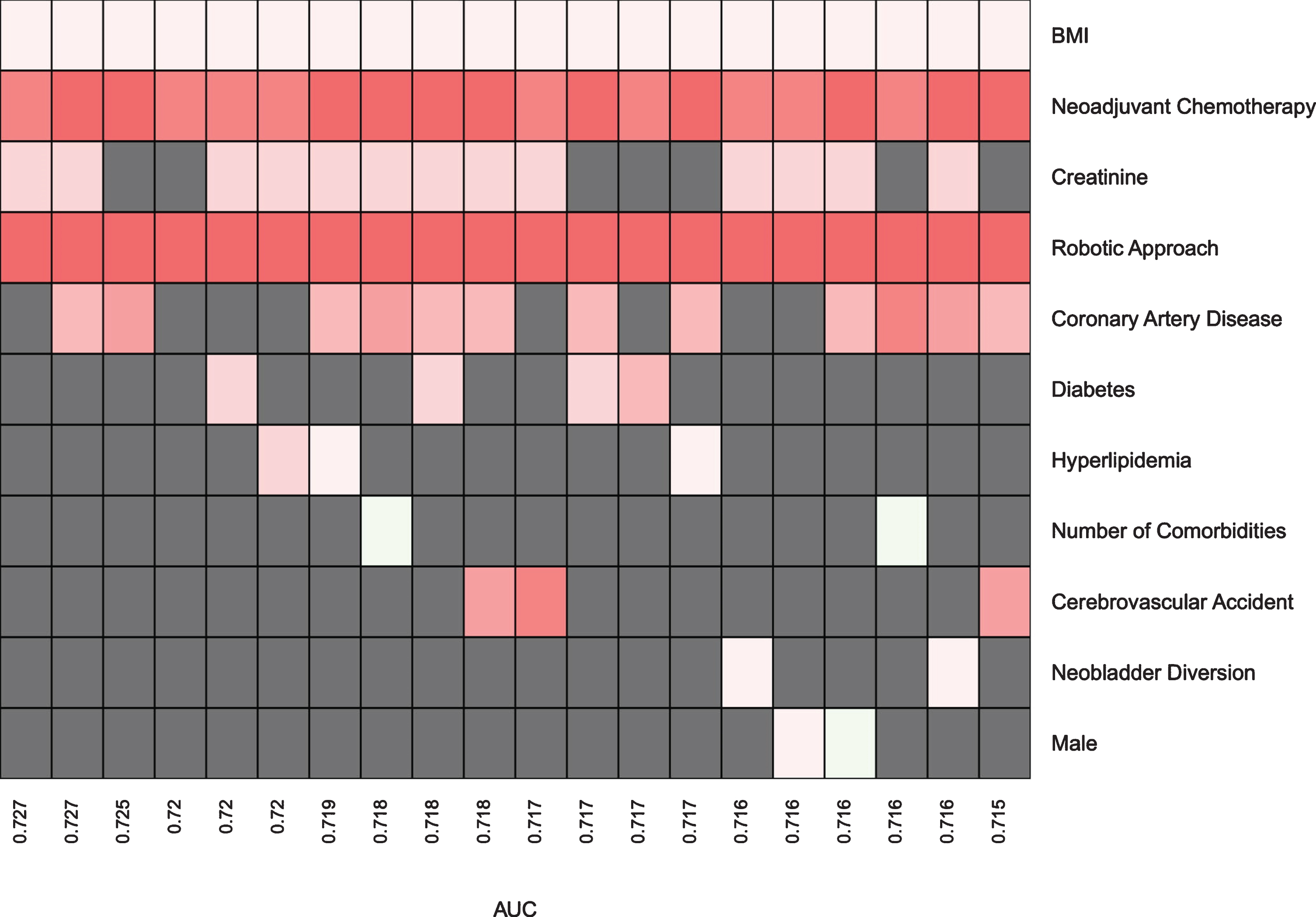
Table 6
Odds Ratios and p-values for the top 5 Performing Models for High Grade Post-Op Complications within 90 Days
Model 1 | Model 2 | Model 3 | Model 4 | Model 5 | |
BMI | 1.0 | 0.02 | 1.0 | 0.03 | 1.0 | 0.02 | 1.0 | 0.02 | 1.0 | 0.03 |
Robotic Approach | 1.3 | 0.24 | 1.3 | 0.25 | 1.3 | 0.21 | 1.4 | 0.20 | 1.3 | 0.26 |
Neoadjuvant Chemotherapy | – | 1.3 | 0.18 | 1.3 | 0.22 | – | 1.4 | 0.15 |
Chronic Obstructive Pulmonary Disease | – | – | 1.4 | 0.26 | 1.4 | 0.21 | – |
Coronary Artery Disease | – | – | – | – | 1.3 | 0.3 |
AUC | 0.562 | 0.561 | 0.56 | 0.559 | 0.558 |
x | y = odds ratio | p-value.
Table 7
Odds Ratios and p-values for the top 5 Performing Models for High Grade Post-Op Complications within 30 Days
Model 1 | Model 2 | Model 3 | Model 4 | Model 5 | |
Chronic Obstructive Pulmonary Disease | 1.5 | 0.15 | 1.6 | 0.13 | 1.6 | 0.10 | 1.6 | 0.13 | 1.4 | 0.22 |
Neobladder Diversion | 1.3 | 0.27 | 1.3 | 0.29 | 1.3 | 0.25 | 1.3 | 0.25 | – |
Obstructive Sleep Apnea | 1.7 | 0.25 | – | – | 1.6 | 0.34 | 1.7 | 0.27 |
BMI | – | – | 1.0 | 0.31 | 1.0 | 0.43 | – |
Neoadjuvant Chemotherapy | – | – | – | – | 1.2 | 0.53 |
AUC | 0.551 | 0.55 | 0.548 | 0.545 | 0.544 |
x | y = odds ratio | p-value.
Table 8
Odds Ratios and p-values for the top 5 Performing Models for High Grade Post-Op Complications within 31 to 90-Days
Model 1 | Model 2 | Model 3 | Model 4 | Model 5 | |
BMI | 1.1 | <0.01 | 1.1 | <0.01 | 1.1 | <0.01 | 1.1 | <0.01 | 1.1 | <0.01 |
Neoadjuvant Chemotherapy | 2.5 | 0.02 | 2.8 | <0.01 | 2.7 | 0.01 | 2.5 | 0.02 | 2.7 | 0.01 |
Creatinine | 1.5 | 0.15 | 1.4 | 0.20 | – | – | 1.4 | 0.17 |
Robotic Approach | 3.1 | <0.01 | 3.0 | <0.01 | 2.8 | <0.01 | 2.9 | <0.01 | 3.1 | <0.01 |
Coronary Artery Disease | – | 1.8 | 0.17 | 1.9 | 0.14 | – | – |
Diabetes | – | – | – | – | 1.5 | 0.35 |
AUC | 0.727 | 0.727 | 0.725 | 0.720 | 0.720 |
x | y = odds ratio | p-value.
DISCUSSION
Radical cystectomy has been the standard treatment for muscle-invasive bladder cancer for decades but is often associated with highly variable risks of potential complications and perioperative mortality [8– 10]. Shabsigh et al. reported that 64% (735/1142) of patients experienced a complication within 90 days of surgery [7]. Furthermore, Clavien-Dindo Grade V complication, perioperative mortality in radical cystectomy patients ranges from 0.3% to 4.5% [11– 14]. However, some studies have suggested that complication rates after radical cystectomy may be significantly underestimated compared to reality [7, 15]. A model capable of estimating postoperative morbidity and mortality would provide valuable information to both the patient and the surgeon in making an informed decision regarding whether or not to pursue surgical treatment with a next step of working to modify risk factors.
Our study is one of the first attempts to develop a predictive model based on preoperative risk factors. We examined numerous preoperative risk factors and selected the most predictive factors to be included in the development of an individualized preoperative assessment tool. Previous studies have shown variables such as patient age, comorbidities, albumin level, diversion choice, pathological information (stage, grade and histology), ASA score, surgeon experience, and hospital volume are associated with postoperative mortality based on multivariate analysis [9, 16– 19]. This is consistent with the variables we found to be the most predictive of postoperative complications in our study, though our emphasis was on preoperative patient factors in order to develop a useful preoperative assessment tool. Since our prediction models are developed using the Clavien-Dindo grading system for complications, they inherently predict for morbidity and mortality. We wanted to target risk factors that are potentially modifiable during our variable selection so that there was a greater benefit for physician counseling and preoperative interventions. While neoadjuvant chemotherapy was found to be predictive of post-operative complications in the 31 to 90-day postoperative period, robust level one evidence supports the use of neoadjuvant chemotherapy and it should be considered in eligible patients [20]. Our results suggest that neoadjuvant chemotherapy may contribute to postoperative complication and this should be discussed in the preoperative counseling with patients pursuing radical cystectomy. In cases when there are several non-modifiable preoperative factors present in a patient, the prediction models can still play an important role in the decision-making process by revealing the higher risks of surgery and the potential need for an alternative treatment approach. Furthermore, a surgeon can elect closer postoperative surveillance of patients identified with possible higher risks for later complications, resulting in decreased costs, morbidity and mortality.
Our study demonstrated that prediction models can be created for major complications following radical cystectomy using a combination of individual preoperative variables. The 20 best performing models in each postoperative period were created using different combinations of the best performing individual variables from univariable analysis. In some cases, an individual variable did not appear in the final model design because it did not contribute to the 20 best performing models for that postoperative period. Interestingly, we saw an improved AUC and fitting of our prediction models in the 31 to 90-day postoperative period when compared to the two other postoperative periods. We speculate that this is because complications occurring in the 31 to 90-day period are more dependent upon intrinsic patient factors as opposed to surgical factors in the first 30 days immediate after surgery. Several studies have reported that most issues within the first 30 days of surgery are associated gastrointestinal and septic complications related to bowel or urine leakage [10, 21]. It is possible that complications such as bowel leaks, urine leaks, and the need for postoperative transfusions are more likely due to surgeon or intraoperative factors and therefore occur in an earlier time period.
While tools like the American College of Surgeons’ National Surgical Quality Improvement Program (ACS NSQIP) Surgical Risk Calculator have been designed to estimate patients’ risk of postoperative complications, these calculators are not specific to radical cystectomy and their prediction algorithms include a large variety of risk factors without certainty in which variables are most strongly associated with postoperative complications. This makes these calculators too cumbersome to navigate, and the results generated may become too extensive to be clinically useful. Furthermore, studies have shown that the level of standardization in reporting of outcomes differs between expert series and NSQIP, making comparisons difficult [21]. In this study, we have developed accessible radical cystectomy prediction models with a reasonable number of inputs for preoperative risk factors in order to help physicians identify patients with an increased risk for radical cystectomy postoperative complications and provide a tool for risk stratifying patients.
Our study is not without limitations and we acknowledge the limited nature of retrospective cohort reviews. Selection bias is likely present as we inherently selected patients who were healthy enough to receive radical cystectomy based on their overall health status, which is not applicable to all patients who present with bladder cancer requiring surgical treatment. As with many studies of this type, our data comes from a large single-institution series, which is less generalizable when compared to population-based representative data. We acknowledge that the robotic approach learning curve occurred during the timeframe of the study cohort, and this may have contributed to the variable’s ability to predict postoperative complications during certain postoperative periods. Several investigators were involved in the retrospective chart review, which is more prone to data acquisition errors when compared to a standardized prospective data abstraction approach. However, we tried to minimize this limitation by assigning each investigator with reviewing a specific portion of patients’ charts so that data collection and interpretation were less variable. In developing the prediction model, we realized that some risk factors are interconnected with others (e.g. hypertension, hyperlipidemia, diabetes) and may contribute to a cumulative comorbidity status instead of being an individual specific predictive factor. This may weaken the prediction power of the model and more effort needs to be put towards identifying unique, unrelated preoperative variables.
To reduce the computation burden during statistical analysis, promising univariate variables were initially selected to be considered in the model building process. However, it is possible that univariate variables with negligible individual effects can potentially result in a strong prediction performance when combined. Therefore, the exclusion of variables from the model building process involves a risk of failing to identify an optimal set of variables. An additional limitation of the feature selection approach is the consideration of main effects solely. Including interactions between variables can possibly further enhance the ability to correctly predict complications.
Our models provide a novel framework to design an individualized assessment tool focusing on selective preoperative patient factors. This provides the foundation for developing future radical cystectomy nomograms with higher predictive powers. Future designs can improve the strength of prediction by incorporating frailty score and other predictive factors not included in our study and minimize data acquisition discrepanciesby utilizing standardized prospective data abstraction. By bridging the gap between statistical analysis and clinical practice, a preoperative assessment tool can inform patients of post-cystectomy risks on an individual basis and offer tailored advice based on a patient’s unique medical composition.
Our findings suggest routinely collected preoperative patient-level clinical variables may be useful for determining patient risk for short-term postoperative complications, especially in a postoperative period that reflects a patient’s intrinsic factors which may contribute to the development of a complication. Our prediction models have flexible number of predictor inputs, allowing users to tailor the degree of risk assessment based on a patient’s baseline heath status. A simple and accessible prediction model with selective predictors may help risk stratify patients, allowing a surgeon to identify at risk patients who may benefit from patient education, counseling and development of risk reduction strategies. Furthermore, the prediction model may give patients additional information to assist in the shared decision-making process of whether to pursue surgical treatment given their preoperative risk factors.
ACKNOWLEDGMENTS
The authors have no acknowledgments.
FUNDING
The authors report no funding.
AUTHOR CONTRIBUTIONS
SN: Protocol Development, Data Entry, Data Analysis, Manuscript Writing and Editing; SG: Statistical Analysis, Manuscript Writing and Editing; DJ: Data Entry, Data Analysis, Manuscript Editing; VG: Data Entry, Data Analysis, Manuscript Editing; HW: Protocol Development, Manuscript Editing; JMH: Protocol Development, Manuscript Editing; DK: Protocol Development, Statistical Analysis, Manuscript Editing; EL: Protocol Development, Data Analysis, Manuscript Writing and Editing.
CONFLICT OF INTEREST
The authors have no conflict of interest, financial or otherwise.
REFERENCES
[1] | Bladder Cancer - Cancer Stat Facts [Internet]. [cited 2018 Sep 19]. Available from: https://seer.cancer.gov/statfacts/html/urinb.html. |
[2] | Witjes JA , Compérat E , Cowan NC , De Santis M , Gakis G , Lebret T , et al. EAU guidelines on muscle-invasive and metastatic bladder cancer: summary of the guidelines. Eur Urol. (2014) ;65: (4):778–92. |
[3] | Chedgy ECP , Black PC . Radical Cystectomy and the Multidisciplinary Management of Muscle-Invasive Bladder Cancer. JAMA Oncol. (2016) ;2: (7):855–6. |
[4] | Chang SS , Boorjian SA , Chou R , Clark PE , Daneshmand S , Konety BR , et al. Diagnosis and Treatment of Non-Muscle Invasive Bladder Cancer: AUA/SUO Guideline. J Urol. (2016) ;196: (4):1021–9. |
[5] | Lawrentschuk N , Colombo R , Hakenberg OW , Lerner SP , Månsson W , Sagalowsky A , et al. Prevention and management of complications following radical cystectomy for bladder cancer. Eur Urol. (2010) ;57: (6):983–1001. |
[6] | Krajewski W , Zdrojowy R , Tupikowski K , Małkiewicz B , Kołodziej A . How to lower postoperative complications after radical cystectomy – a review. Cent European J Urol. (2016) ;69: (4):370–6. |
[7] | Shabsigh A , Korets R , Vora KC , Brooks CM , Cronin AM , Savage C , et al. Defining early morbidity of radical cystectomy for patients with bladder cancer using a standardized reporting methodology. Eur Urol. (2009) ;55: (1):164–74. |
[8] | Konety BR , Allareddy V , Herr H . Complications after radical cystectomy: analysis of population-based data. Urology. (2006) ;68: (1):58–64. |
[9] | Novara G , De Marco V , Aragona M , Boscolo-Berto R , Cavalleri S , Artibani W , et al. Complications and mortality after radical cystectomy for bladder transitional cell cancer. J Urol. (2009) ;182: (3):914–21. |
[10] | Quek ML , Stein JP , Daneshmand S , Miranda G , Thangathurai D , Roffey P , et al. A critical analysis of perioperative mortality from radical cystectomy. J Urol.886-9; discussion. (2006) ;175: (3 Pt 1):889–890. |
[11] | Stein JP , Lieskovsky G , Cote R , Groshen S , Feng AC , Boyd S , et al. Radical cystectomy in the treatment of invasive bladder cancer: long-term results in 1,054 patients. J Clin Oncol. (2001) ;19: (3):666–75. |
[12] | Chang SS , Cookson MS , Baumgartner RG , Wells N , Smith JA . Analysis of early complications after radical cystectomy: results of a collaborative care pathway. J Urol. (2002) ;167: (5):2012–6. |
[13] | Figueroa AJ , Stein JP , Dickinson M , Skinner EC , Thangathurai D , Mikhail MS , et al. Radical cystectomy for elderly patients with bladder carcinoma: an updated experience with 404 patients. Cancer. (1998) ;83: (1):141–7. |
[14] | Stroumbakis N , Herr HW , Cookson MS , Fair WR . Radical cystectomy in the octogenarian. J Urol. (1997) ;158: (6):2113–7. |
[15] | Hollenbeck BK , Miller DC , Taub DA , Dunn RL , Khuri SF , Henderson WG , et al. The effects of adjusting for case mix on mortality and length of stay following radical cystectomy. J Urol. (2006) ;176: (4 Pt 1):1363–8. |
[16] | Hollenbeck BK , Miller DC , Taub D , Dunn RL , Khuri SF , Henderson WG , et al. Identifying risk factors for potentially avoidable complications following radical cystectomy. J Urol. (2005) ;174: (4 Pt 1):1231–7; discussion 1237. |
[17] | Elting LS , Pettaway C , Bekele BN , Grossman HB , Cooksley C , Avritscher EBC , et al. Correlation between annual volume of cystectomy, professional staffing, and outcomes: a statewide, population-based study. Cancer. (2005) ;104: (5):975–84. |
[18] | Taylor JM , Feifer A , Savage CJ , Maschino AC , Bernstein M , Herr HW , et al. Evaluating the utility of a preoperative nomogram for predicting 90-day mortality following radical cystectomy for bladder cancer. BJU Int. (2012) ;109: (6):855–9. |
[19] | Knap MM , Lundbeck F , Overgaard J . Early and late treatment-related morbidity following radical cystectomy. Scand J Urol Nephrol. (2004) ;38: (2):153–60. |
[20] | Grossman HB , Natale RB , Tangen CM , Speights VO , Vogelzang NJ , Trump DL , et al. Neoadjuvant chemotherapy plus cystectomy compared with cystectomy alone for locally advanced bladder cancer. N Engl J Med. (2003) ;349: (9):859–66. |
[21] | Chahal R , Sundaram SK , Iddenden R , Forman DF , Weston PMT , Harrison SCW . A study of the morbidity, mortality and long-term survival following radical cystectomy and radical radiotherapy in the treatment of invasive bladder cancer in Yorkshire. Eur Urol. (2003) ;43: (3):246–57. |
[22] | Knight BA , Potretzke AM , Larson JA , Bhayani SB . Comparing Expert Reported Outcomes to National Surgical Quality Improvement Program Risk Calculator-Predicted Outcomes: Do Reporting Standards Differ? Journal of Endourology. (2015) ;29: (9):1091–9. |